Key Takeaways
- Understanding AI Software: AI software replicates human intelligence to automate tasks, analyze data, and make decisions, with applications across various industries.
- Key Technologies Driving AI: Machine learning, deep learning, natural language processing, and computer vision are the core technologies enabling AI systems to function and evolve.
- Benefits and Challenges: While AI software offers efficiency, automation, and personalization, challenges like data privacy, bias, and implementation costs must be addressed for successful integration.
Artificial Intelligence (AI) has evolved from a futuristic concept into a transformative force that is reshaping industries and daily life.
From voice assistants like Siri and Alexa to recommendation algorithms on streaming platforms, AI software is becoming an integral part of modern technology.
But what exactly is AI software, and how does it work?
This question has become increasingly important as businesses and individuals alike adopt AI to streamline processes, enhance decision-making, and create innovative solutions across various sectors.
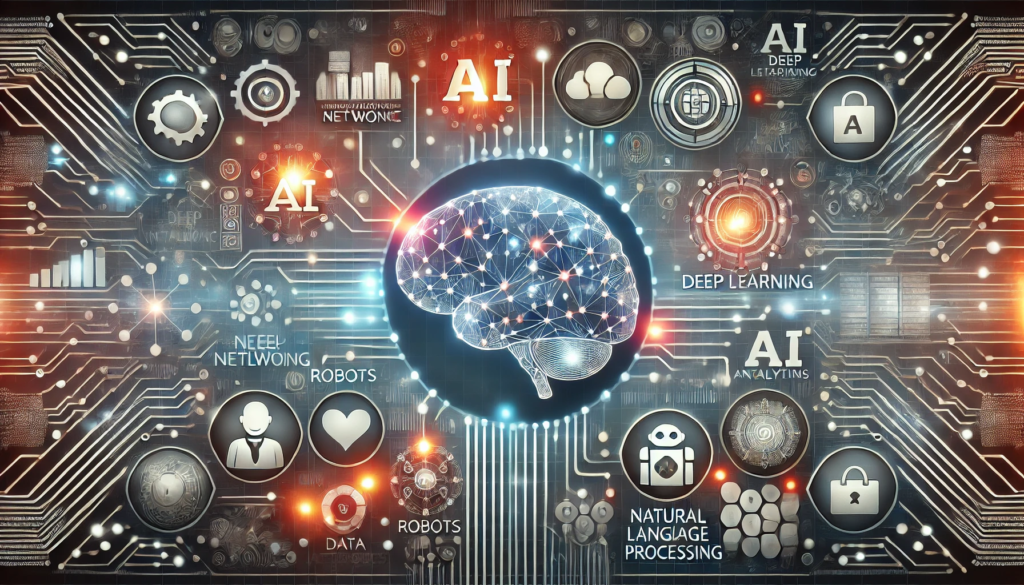
AI software refers to systems that use algorithms, data, and computing power to mimic human intelligence.
Unlike traditional software that follows predefined rules to complete tasks, AI software has the capability to learn from data, adapt to new information, and improve its performance over time.
This ability to evolve makes AI incredibly powerful, capable of solving complex problems and performing tasks that were once reserved for human brains.
Whether it’s analyzing massive datasets, driving autonomous vehicles, or understanding and generating human language, AI software is at the forefront of technological advancements.
In this blog, we will explore the fundamentals of AI software—what it is, how it works, and the technologies that drive it.
We’ll look at the different types of AI, such as machine learning, deep learning, and natural language processing, and how these technologies work together to create intelligent systems.
Moreover, we will discuss the real-world applications of AI software in fields like healthcare, finance, marketing, and everyday consumer products.
As AI continues to expand and integrate into various industries, understanding its core principles and mechanics is crucial for businesses, tech enthusiasts, and anyone interested in the future of technology.
AI is not just a passing trend—it’s a revolution in the making. As businesses seek to leverage AI for increased efficiency, enhanced personalization, and smarter decision-making, its impact on the global economy and everyday life continues to grow.
In this detailed exploration, we will also touch on the benefits and challenges that come with adopting AI software, as well as the ethical considerations surrounding its use.
By the end of this blog, you will have a comprehensive understanding of AI software, its inner workings, and why it is considered one of the most significant technological breakthroughs of the 21st century.
Prepare to dive into the world of AI software, where intelligence meets innovation and endless possibilities.
Before we venture further into this article, we would like to share who we are and what we do.
About 9cv9
9cv9 is a business tech startup based in Singapore and Asia, with a strong presence all over the world.
With over eight years of startup and business experience, and being highly involved in connecting with thousands of companies and startups, the 9cv9 team has listed some important learning points in this overview of What is Artificial Intelligence (AI) Software and How It Works.
If your company needs recruitment and headhunting services to hire top-quality employees, you can use 9cv9 headhunting and recruitment services to hire top talents and candidates. Find out more here, or send over an email to [email protected].
Or just post 1 free job posting here at 9cv9 Hiring Portal in under 10 minutes.
What is Artificial Intelligence (AI) Software and How It Works
- What is Artificial Intelligence (AI) Software?
- How AI Software Works
- Key Technologies Behind AI Software
- The Benefits of AI Software
- Challenges of AI Software
- Future of AI Software
1. What is Artificial Intelligence (AI) Software?
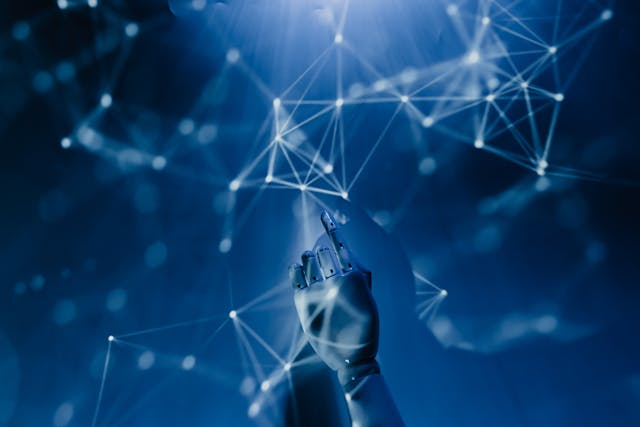
Artificial Intelligence (AI) software refers to the collection of tools, algorithms, and models that enable machines and computers to perform tasks that typically require human intelligence. Unlike traditional software, which follows a set of programmed rules to complete tasks, AI software is designed to “learn” from experience, improve over time, and make decisions based on data. This ability to mimic cognitive functions such as problem-solving, learning, and decision-making has made AI one of the most transformative technologies of the 21st century.
AI software can be applied in a variety of fields, from healthcare and finance to marketing and entertainment. In this section, we will break down what AI software is, its core components, and its key features. Additionally, we’ll explore real-world examples to illustrate the diverse applications of AI software.
Key Components of AI Software
AI software is built around several fundamental technologies that enable it to replicate human intelligence. These technologies allow AI systems to process large volumes of data, recognize patterns, and improve decision-making. The key components of AI software include:
- Machine Learning (ML):
- The core of most AI software. ML algorithms allow AI systems to learn from data without explicit programming.
- AI models improve over time as they are exposed to more data, enabling them to make better predictions and decisions.
- Examples: Google’s search engine ranking algorithm, Netflix’s recommendation engine.
- Natural Language Processing (NLP):
- Enables AI software to understand, interpret, and generate human language.
- Key in applications such as chatbots, virtual assistants, and text analysis.
- Example: Apple’s Siri and Amazon Alexa rely heavily on NLP to understand and respond to user commands.
- Computer Vision:
- Allows AI software to interpret and process visual information, such as images and videos.
- Used in facial recognition, object detection, and autonomous vehicles.
- Example: AI-powered facial recognition systems in security cameras, Tesla’s self-driving cars.
- Robotics:
- A branch of AI focused on designing machines that can perform tasks autonomously or semi-autonomously.
- Robotics integrates AI to enable machines to learn from their environment and perform complex tasks.
- Example: Boston Dynamics’ robots that can navigate complex environments, iRobot’s Roomba vacuum.
Types of AI Software
AI software can be categorized based on its capabilities and the type of tasks it can perform. The primary categories of AI software include:
- Narrow AI (Weak AI):
- Designed to perform a specific task or set of tasks.
- Narrow AI is the most common form of AI in use today.
- Example: Virtual assistants like Siri and Alexa, recommendation systems like those used by Spotify and YouTube.
- General AI (Strong AI):
- A theoretical concept where AI systems can perform any intellectual task that a human can do.
- General AI does not currently exist but represents the ultimate goal of AI research.
- Superintelligent AI:
- A form of AI that surpasses human intelligence across all domains.
- While still speculative, superintelligent AI could revolutionize every aspect of life once developed.
- Example: Speculated in science fiction works like The Terminator or Her, but not yet realized in the real world.
How AI Software Works
AI software works through a combination of algorithms, data, and computing power. The learning and decision-making process within AI can be broken down into several stages:
- Data Collection and Preprocessing:
- AI systems require vast amounts of data to learn from. This data is gathered and cleaned to ensure accuracy and relevance.
- Example: In healthcare, AI software uses medical records, images, and patient data to learn how to diagnose diseases.
- Model Training:
- AI systems use machine learning algorithms to process the data and identify patterns.
- Models are trained on labeled data (supervised learning), unlabeled data (unsupervised learning), or a combination of both.
- Example: In the finance industry, AI models are trained on historical data to predict stock market trends.
- Decision Making and Prediction:
- After training, AI software can make predictions or decisions based on new input data.
- AI models can continuously improve as more data is fed into the system.
- Example: AI systems in customer service software can automatically recommend products based on past purchases and browsing history.
Real-World Examples of AI Software
AI software is already being used in a variety of applications, both in businesses and consumer products. Here are some key examples of AI software in action:
- AI in Healthcare:
- Diagnosis and Treatment Recommendations: AI software is used to analyze medical images, patient records, and genetic data to identify diseases and recommend treatments.
- Example: IBM Watson Health helps doctors diagnose diseases such as cancer by analyzing vast amounts of medical literature and patient data.
- Predictive Analytics: AI models can predict disease outbreaks and patient deterioration, allowing healthcare providers to take preventive measures.
- Example: Predictive models in hospitals help track patient conditions and improve resource allocation.
- AI in Marketing and Customer Service:
- Personalization: AI algorithms are used to provide personalized recommendations to customers based on their behavior and preferences.
- Example: Amazon uses AI to recommend products based on previous purchases and browsing history.
- Chatbots and Virtual Assistants: AI-powered chatbots interact with customers, answering queries, resolving issues, and guiding them through processes.
- Example: H&M uses AI chatbots to assist customers in selecting clothing styles, sizes, and completing purchases.
- AI in Autonomous Vehicles:
- Self-Driving Cars: AI software is crucial in enabling autonomous vehicles to make real-time decisions based on sensory data (e.g., cameras, LIDAR, and radar).
- Example: Tesla’s Autopilot system uses AI to navigate roads, detect obstacles, and respond to traffic conditions.
- AI in Finance:
- Fraud Detection: AI models analyze transaction data to identify patterns of fraudulent activity and flag suspicious transactions.
- Example: AI-powered fraud detection systems used by banks like JPMorgan Chase and Mastercard.
- Algorithmic Trading: AI software is used by financial institutions to make high-frequency trading decisions based on market data and trends.
- Example: Hedge funds and investment firms use AI to perform algorithmic trading, optimizing profits by executing trades at the right time.
Conclusion
AI software is a rapidly advancing field that is reshaping industries and our everyday lives. From healthcare to entertainment, businesses are harnessing the power of AI to enhance productivity, personalize experiences, and create smarter solutions. By understanding what AI software is, how it works, and the key technologies that enable it, we can appreciate its far-reaching impact and its potential to revolutionize even more sectors in the future.
2. How AI Software Works
AI software operates by utilizing advanced algorithms, vast datasets, and powerful computing resources to simulate human-like intelligence. At its core, AI software is designed to learn from data, make predictions, solve problems, and continuously improve its performance over time. In this section, we will break down the key processes that enable AI software to function, explore the different types of learning techniques used, and provide real-world examples to illustrate these mechanisms in action.
1. Data Collection and Preprocessing
The first step in how AI software works is gathering data. Since AI learns from data, the quality and quantity of the data play a crucial role in determining the accuracy and efficiency of AI models. Here’s how it works:
- Data Collection:
- AI systems require large volumes of structured and unstructured data to identify patterns and make predictions. This data could be in the form of text, images, videos, sensor data, or even transactional data.
- Example: In healthcare, AI software uses patient medical records, diagnostic images, and historical data to train models for predicting diseases.
- Data Preprocessing:
- Raw data is often messy and incomplete. Preprocessing involves cleaning, transforming, and structuring the data to ensure it’s ready for analysis.
- This step includes removing duplicates, handling missing values, and normalizing or scaling the data.
- Example: In e-commerce, product reviews and customer feedback are cleaned to remove irrelevant content before being used to train recommendation models.
2. Model Training
Once the data is preprocessed, it is used to train AI models. During the training phase, the AI learns from the data and builds predictive models. There are several different types of machine learning models that AI software can use:
- Supervised Learning:
- In supervised learning, the model is trained on a labeled dataset, meaning the input data is paired with the correct output (the “ground truth”).
- The model learns to map inputs to correct outputs and refines itself over time to minimize errors.
- Example: In image recognition, supervised learning is used to train AI to recognize objects (e.g., dogs, cats) based on labeled images.
- Unsupervised Learning:
- Unsupervised learning is used when the data does not have labels, and the AI needs to identify hidden patterns or structures within the data.
- This technique is typically used for clustering, anomaly detection, and dimensionality reduction.
- Example: In marketing, unsupervised learning can be used to segment customers based on purchasing behavior without predefined categories.
- Reinforcement Learning:
- Reinforcement learning involves an agent that interacts with an environment and learns to make decisions by receiving feedback (rewards or penalties) based on its actions.
- This learning technique is often used in situations requiring sequential decision-making, such as robotics or game playing.
- Example: AI in self-driving cars uses reinforcement learning to optimize driving behavior by receiving feedback on its actions in different traffic conditions.
3. Algorithm Selection and Application
Once a model is trained, AI software applies various algorithms to process the data and make predictions or decisions. The choice of algorithm depends on the specific task the AI is being trained for. Some common algorithms used in AI software include:
- Linear Regression:
- A simple algorithm used for predicting numerical values based on a linear relationship between variables.
- Example: In finance, linear regression is used to predict stock prices based on historical data.
- Decision Trees:
- Decision trees model decisions and their possible consequences in a tree-like structure, used for classification and regression tasks.
- Example: In customer support, decision trees are used to automate customer queries by guiding AI to the right response based on user input.
- Neural Networks:
- Neural networks mimic the structure of the human brain by processing information in layers of interconnected nodes (neurons). Deep learning is a subset of machine learning that uses multi-layered neural networks.
- Example: In speech recognition, neural networks are used to convert spoken words into text (e.g., Google Assistant or Amazon Alexa).
4. Model Evaluation and Testing
After an AI model is trained and algorithms are applied, it must be evaluated to ensure its effectiveness. This evaluation process is critical for determining how well the AI system can generalize to new, unseen data.
- Training vs. Test Data:
- To evaluate an AI model, the data is typically divided into two sets: a training set and a test set. The training set is used to train the model, while the test set is used to assess its performance on new data.
- Example: In fraud detection systems, the model is trained on historical transaction data and then tested on recent transactions to predict fraudulent activities.
- Performance Metrics:
- Common metrics used to evaluate AI models include accuracy, precision, recall, F1-score, and area under the curve (AUC).
- Example: In medical AI, the accuracy metric would evaluate how well an AI system predicts diseases based on test data.
5. Decision Making and Prediction
Once the AI model is trained and tested, it can begin to make decisions or predictions based on real-time input data. The decision-making process is a crucial step in AI’s ability to perform complex tasks autonomously.
- Input Data:
- AI software receives real-time input data from various sources, such as sensors, user interactions, or databases.
- Example: In self-driving cars, AI receives data from cameras, LIDAR, and radar sensors to make real-time driving decisions.
- Data Analysis:
- The AI model processes the input data using the algorithms and patterns learned during the training phase. The system evaluates the data and identifies the most likely outcome.
- Example: In e-commerce, an AI recommendation system analyzes browsing and purchase history to suggest relevant products to users.
- Output and Actions:
- Based on the analysis, the AI software generates an output or takes action. This output could be a decision, a recommendation, a classification, or a prediction.
- Example: In customer service, AI chatbots use NLP to understand customer queries and respond with appropriate answers or actions.
6. Continuous Learning and Improvement
One of the defining characteristics of AI software is its ability to learn and improve over time. As AI systems encounter more data and new situations, they can adapt and refine their models to improve accuracy and performance.
- Model Retraining:
- AI software can retrain its models with new data to ensure it stays up-to-date and relevant to current trends and patterns.
- Example: In fraud detection, AI models are retrained with new data to adapt to evolving fraudulent tactics.
- Feedback Loops:
- AI systems can incorporate feedback from users or the environment to continuously improve their performance.
- Example: In voice recognition, systems like Siri and Alexa improve their accuracy by learning from user interactions and speech patterns over time.
7. Real-World Examples of How AI Software Works
To further illustrate how AI software works, here are a few examples of real-world applications:
- AI in Healthcare:
- Diagnostic Tools: AI software analyzes medical images (e.g., X-rays, MRIs) to diagnose conditions like cancer and heart disease.
- Example: Google Health’s AI system can detect breast cancer in mammograms with higher accuracy than human radiologists.
- AI in Autonomous Vehicles:
- Self-Driving Cars: AI processes sensor data to make decisions about navigation, speed, and safety.
- Example: Tesla’s Autopilot uses AI to control the car’s movement, making real-time driving decisions based on road conditions.
- AI in E-Commerce:
- Personalization Engines: AI analyzes user behavior and preferences to recommend products and improve user experiences.
- Example: Amazon uses AI to suggest products based on past browsing and purchasing history.
- AI in Finance:
- Algorithmic Trading: AI algorithms analyze market data to make fast, high-frequency trading decisions.
- Example: Hedge funds and investment firms use AI to automate trading strategies and maximize profit opportunities.
Conclusion
Understanding how AI software works is essential for grasping its potential and impact on various industries. Through data collection, preprocessing, model training, and algorithm application, AI systems can perform complex tasks that traditionally required human intelligence. As AI technology continues to evolve, its ability to learn, adapt, and improve ensures that it will remain a powerful tool in transforming industries and enhancing human capabilities.
3. Key Technologies Behind AI Software
Artificial Intelligence (AI) software is powered by various advanced technologies that enable it to simulate human-like intelligence, learn from data, and make decisions autonomously. These technologies form the backbone of AI systems, driving their ability to perform tasks that traditionally required human intelligence, such as speech recognition, image analysis, and decision-making. In this section, we will explore the key technologies behind AI software, examining how they work and their practical applications.
1. Machine Learning (ML)
Machine Learning (ML) is one of the most critical components of AI. ML allows systems to learn from data without being explicitly programmed. By identifying patterns in large datasets, ML algorithms can make predictions, recognize objects, and even detect anomalies.
- How ML Works:
- ML algorithms use statistical methods to analyze data and “learn” from it, improving their predictions over time.
- Models are trained using labeled data (supervised learning) or unlabeled data (unsupervised learning) to develop an understanding of the patterns within the dataset.
- Types of Machine Learning:
- Supervised Learning:
- Involves training a model on labeled data to predict outcomes.
- Example: In finance, supervised learning is used to predict stock prices based on historical market data.
- Unsupervised Learning:
- Involves training a model on unlabeled data to identify hidden patterns or groupings.
- Example: In marketing, unsupervised learning is used to segment customers based on purchasing behavior.
- Reinforcement Learning:
- Involves training an agent to make decisions by rewarding or penalizing its actions in a given environment.
- Example: Self-driving cars use reinforcement learning to navigate streets by learning from rewards and penalties based on driving behavior.
- Supervised Learning:
- Applications of Machine Learning:
- Spam Filtering: AI systems learn to identify spam emails based on characteristics and past examples.
- Recommendation Systems: Platforms like Netflix and YouTube use ML to recommend content based on user preferences.
2. Deep Learning
Deep Learning is a subfield of Machine Learning that uses neural networks with multiple layers to process complex data structures. Deep learning is especially powerful in tasks like image recognition, natural language processing (NLP), and speech recognition.
- How Deep Learning Works:
- Deep learning models are designed with several layers of neurons (artificial neurons modeled after the human brain), each layer learning different features of the data.
- The more layers in the neural network, the “deeper” the learning process becomes, allowing the system to learn more intricate patterns and make more accurate predictions.
- Types of Deep Learning Architectures:
- Convolutional Neural Networks (CNNs):
- Primarily used for image and video recognition tasks, CNNs process pixel data and can detect patterns such as edges, shapes, and textures.
- Example: AI-powered medical imaging systems like IBM Watson Health use CNNs to identify anomalies in X-rays and MRIs.
- Recurrent Neural Networks (RNNs):
- Designed for processing sequential data, such as time-series data or natural language.
- Example: AI chatbots use RNNs to generate responses based on the context of the conversation.
- Generative Adversarial Networks (GANs):
- Used for generating new data by pitting two neural networks against each other: one generates data (the generator), and the other evaluates it (the discriminator).
- Example: AI-generated art and realistic deepfakes are created using GANs to generate new, realistic images or videos.
- Convolutional Neural Networks (CNNs):
- Applications of Deep Learning:
- Autonomous Vehicles: AI systems in self-driving cars use deep learning to process visual and sensor data to navigate and make decisions.
- Voice Assistants: Virtual assistants like Siri and Alexa use deep learning to understand and process spoken commands.
3. Natural Language Processing (NLP)
Natural Language Processing (NLP) is the AI technology that enables machines to understand, interpret, and generate human language. NLP allows AI systems to process and analyze large amounts of natural language data, enabling applications like machine translation, sentiment analysis, and chatbots.
- How NLP Works:
- NLP systems use algorithms to break down text into smaller parts (such as words or phrases), understand their meaning, and then generate appropriate responses.
- Techniques like tokenization, named entity recognition (NER), part-of-speech tagging, and dependency parsing are used to process and analyze language.
- Key Tasks in NLP:
- Speech Recognition:
- AI systems convert spoken language into text, allowing voice-controlled applications to function.
- Example: Google’s speech-to-text software and voice assistants like Alexa use NLP for accurate voice recognition.
- Text Classification:
- Categorizing text into predefined categories, such as spam vs. non-spam emails or positive vs. negative sentiment.
- Example: Sentiment analysis in social media monitoring tools helps companies understand public opinion about their brand.
- Machine Translation:
- NLP enables the automatic translation of text between languages.
- Example: Google Translate uses NLP algorithms to offer real-time translations for text and speech.
- Speech Recognition:
- Applications of NLP:
- Chatbots and Virtual Assistants: AI-powered customer service agents use NLP to interact with users in natural language.
- Text Summarization: AI tools use NLP to generate concise summaries of long articles or documents.
4. Computer Vision
Computer Vision enables AI systems to interpret and understand visual data, such as images and videos. It mimics the human ability to recognize objects, scenes, and activities, allowing AI to perform tasks like image classification, facial recognition, and motion detection.
- How Computer Vision Works:
- Computer vision algorithms extract features from visual data and use machine learning or deep learning models to recognize patterns and make predictions.
- The models are trained on large datasets of labeled images to learn how to identify objects, textures, and shapes.
- Key Tasks in Computer Vision:
- Object Detection:
- Identifying and locating objects within an image or video.
- Example: AI-powered surveillance cameras use object detection to identify people or vehicles in real-time.
- Facial Recognition:
- Identifying and verifying individuals based on facial features.
- Example: Smartphones like iPhone use facial recognition for secure device unlocking.
- Image Segmentation:
- Dividing an image into segments to identify and analyze different parts of it.
- Example: AI in autonomous vehicles uses image segmentation to understand road lanes and obstacles.
- Object Detection:
- Applications of Computer Vision:
- Medical Imaging: AI uses computer vision to assist doctors in detecting diseases from X-rays, MRIs, and CT scans.
- Retail: AI-driven visual recognition systems help automate inventory tracking and checkout processes in stores.
5. Robotics and Automation
Robotics involves the use of AI to control physical robots that can perform tasks autonomously or semi-autonomously. These robots are equipped with sensors, actuators, and algorithms to interact with their environment and perform tasks.
- How Robotics Works:
- Robots use sensors (e.g., cameras, LIDAR, accelerometers) to perceive their environment, process the data using AI algorithms, and then take appropriate actions using actuators (motors, arms, etc.).
- Key Technologies in Robotics:
- Computer Vision: Robots use computer vision to navigate and interact with objects in their environment.
- Sensor Fusion: Combining data from multiple sensors to create a comprehensive understanding of the environment.
- Applications of Robotics:
- Manufacturing: AI-powered robots automate tasks such as assembly, welding, and packaging.
- Healthcare: Robotic surgery systems, like the da Vinci Surgical System, assist surgeons in performing precise operations with minimal invasiveness.
6. Neural Networks and Cognitive Computing
Neural networks and cognitive computing simulate the processes of the human brain, enabling AI systems to think, reason, and make decisions. Cognitive computing involves systems that can process vast amounts of data to simulate human thought processes.
- How Neural Networks and Cognitive Computing Work:
- Neural networks are designed with interconnected layers of nodes, where each node mimics the functioning of a neuron. The network learns patterns from data by adjusting the weights of these connections during training.
- Applications of Neural Networks:
- Image and Speech Recognition: Neural networks are used in image recognition (e.g., facial recognition systems) and speech recognition (e.g., voice assistants).
- Financial Trading: AI models use neural networks to analyze market data and make real-time trading decisions.
Conclusion
The technologies behind AI software are vast and interconnected, each playing a critical role in enabling AI systems to process, analyze, and make decisions based on data. Machine learning, deep learning, natural language processing, computer vision, robotics, and cognitive computing are just a few of the key technologies that empower AI to perform complex tasks with increasing efficiency. As these technologies continue to evolve, AI will become even more capable of transforming industries and improving the way we live and work.
4. The Benefits of AI Software
AI software offers transformative benefits across a wide range of industries. By simulating human intelligence, AI systems can perform complex tasks with higher efficiency and accuracy than traditional methods. As businesses and organizations adopt AI software, they experience numerous advantages, from cost savings and improved productivity to enhanced customer experiences and data-driven decision-making. This section explores the key benefits of AI software and how these advantages are reshaping the modern landscape.
1. Increased Efficiency and Productivity
AI software significantly enhances operational efficiency and productivity by automating repetitive tasks, streamlining processes, and minimizing human error. This allows employees to focus on more creative and strategic tasks, which ultimately drives business growth.
- Automation of Routine Tasks:
- AI systems can handle mundane, time-consuming tasks such as data entry, customer inquiries, and document processing.
- Example: Robotic Process Automation (RPA) tools powered by AI can automate invoice processing in accounting departments, freeing up employees to work on more complex financial analysis.
- Faster Decision-Making:
- AI software analyzes data in real time, helping businesses make faster decisions based on the most relevant information available.
- Example: AI-driven financial trading platforms can analyze market conditions in milliseconds and execute trades based on real-time data, providing an edge in high-stakes markets.
- Optimized Workflow and Operations:
- AI systems optimize workflow by identifying bottlenecks and inefficiencies in existing processes and suggesting improvements.
- Example: AI-powered supply chain management software can predict demand, automate inventory restocking, and optimize routes for delivery drivers, resulting in smoother operations and reduced operational costs.
2. Cost Reduction
One of the primary drivers behind the adoption of AI software is its potential to reduce costs. By automating tasks, improving accuracy, and enhancing productivity, businesses can achieve significant cost savings in various aspects of their operations.
- Reduction in Labor Costs:
- AI can perform tasks traditionally done by human employees, reducing the need for extensive human labor and allowing businesses to reallocate resources to other areas.
- Example: AI-powered customer support chatbots can handle customer inquiries, reducing the need for large customer service teams and cutting labor costs.
- Lower Error Rates:
- AI software reduces human error, ensuring higher accuracy in tasks such as data entry, calculations, and decision-making.
- Example: In the healthcare sector, AI software used in diagnostics can minimize the chances of errors in reading medical images, leading to fewer misdiagnoses and, ultimately, lower costs associated with malpractice.
- Operational Cost Optimization:
- AI can identify inefficiencies in operations and suggest ways to minimize resource usage, reducing operational costs over time.
- Example: AI-driven energy management systems can optimize power consumption in large facilities, significantly lowering energy costs while maintaining optimal performance.
3. Enhanced Customer Experience
AI software is revolutionizing customer service and experience by providing personalized interactions, improving response times, and offering consistent support across channels.
- Personalization:
- AI tools can analyze customer data to offer personalized recommendations, product suggestions, and tailored experiences, enhancing customer satisfaction and loyalty.
- Example: E-commerce platforms like Amazon and Netflix use AI algorithms to suggest products and content based on individual user preferences and browsing history, increasing engagement and sales.
- 24/7 Availability:
- AI-powered chatbots and virtual assistants provide round-the-clock customer support, ensuring that customers receive timely responses to their inquiries, regardless of the time zone.
- Example: AI chatbots used by banks or telecom companies handle customer queries at any hour, significantly reducing wait times and enhancing the overall customer experience.
- Faster Issue Resolution:
- AI systems can quickly identify issues and provide solutions, improving service quality and customer satisfaction.
- Example: AI-powered CRM systems can track customer interactions and instantly retrieve the most relevant data, enabling customer service representatives to resolve issues quickly and efficiently.
4. Better Decision-Making Through Data Analytics
AI software’s ability to analyze vast amounts of data enables businesses to make data-driven decisions that improve outcomes and drive success. The insights derived from AI analytics can help organizations identify trends, predict future events, and optimize their strategies.
- Predictive Analytics:
- AI software can analyze historical data and predict future trends, helping businesses anticipate market changes, customer behavior, and potential risks.
- Example: In retail, AI algorithms predict consumer buying patterns, allowing companies to plan inventory levels and marketing strategies accordingly, thus minimizing stockouts or overstocking.
- Data-Driven Insights:
- AI software can analyze complex datasets and uncover hidden patterns that humans might overlook, providing actionable insights that inform business strategies.
- Example: AI tools in the healthcare sector analyze patient data to predict potential health conditions, helping doctors make more accurate diagnoses and treatment plans.
- Improved Forecasting:
- AI models can forecast market conditions, demand patterns, and economic indicators with higher accuracy than traditional methods, aiding businesses in long-term planning.
- Example: AI-powered financial forecasting tools predict stock price movements, helping investors make informed decisions in volatile markets.
5. Scalability and Flexibility
AI software enables businesses to scale their operations effectively and adapt quickly to changes in the market or operational environment. Whether it is handling an increased volume of data, expanding customer support operations, or managing more complex tasks, AI provides the flexibility needed to grow without significant additional investment.
- Handling Large Volumes of Data:
- AI systems can process and analyze vast amounts of data quickly and accurately, enabling businesses to handle large-scale operations with ease.
- Example: Social media platforms like Facebook and Twitter use AI to process billions of user interactions daily, extracting valuable insights for advertisers and content creators.
- Adaptability to Market Changes:
- AI software can adapt to new data and conditions in real time, ensuring that businesses can respond quickly to market shifts.
- Example: In the automotive industry, AI-powered systems in self-driving cars can instantly adjust to changing road conditions or unexpected obstacles, ensuring safe and efficient navigation.
- Automated Scaling:
- AI systems enable automatic scaling of operations, such as customer support, production lines, and data processing capabilities, without manual intervention.
- Example: Cloud-based AI services such as AWS and Google Cloud allow businesses to scale up their infrastructure as needed, handling surges in demand without downtime.
6. Innovation and Competitive Advantage
By adopting AI software, businesses gain a competitive edge by unlocking new opportunities for innovation and growth. AI enables companies to stay ahead of the curve by enabling faster product development, creating new business models, and optimizing existing products and services.
- Accelerating Product Development:
- AI-driven simulations and prototyping tools can accelerate the process of product development by testing designs and iterations quickly and accurately.
- Example: In the automotive industry, AI is used to design more fuel-efficient engines, improving performance while reducing environmental impact.
- Innovation in New Products and Services:
- AI software enables the creation of innovative products and services that would not be possible without AI capabilities, opening up new revenue streams.
- Example: AI-powered voice assistants, such as Google Assistant and Amazon Alexa, have created new opportunities for smart home devices and applications, revolutionizing the way consumers interact with technology.
- Enhanced Competitive Position:
- By leveraging AI, businesses can optimize processes, reduce costs, and offer enhanced products or services, improving their market position and differentiation from competitors.
- Example: AI-driven customer insights and targeted marketing strategies allow companies like Spotify and Facebook to deliver highly personalized user experiences, keeping customers engaged and loyal.
7. Improved Security
AI software enhances security by identifying and responding to threats more effectively than traditional methods. Its ability to monitor data and detect patterns in real time makes it a powerful tool for protecting sensitive information and mitigating risks.
- Threat Detection and Prevention:
- AI systems can analyze network traffic and identify unusual patterns that may signal potential cyberattacks or data breaches.
- Example: AI-powered cybersecurity tools use anomaly detection to identify phishing attempts, malware, and other threats before they can cause significant damage.
- Fraud Detection:
- AI software analyzes transactional data in real time to detect fraudulent activities and prevent financial losses.
- Example: Banks and financial institutions use AI to detect unusual account activity, such as unauthorized transactions, which helps prevent fraud.
- Predictive Security Measures:
- AI can predict potential security breaches by analyzing historical data and identifying vulnerabilities in systems or processes.
- Example: AI systems used by governments and defense agencies can predict potential cyberattacks based on patterns of past threats and suggest preemptive measures.
Conclusion
The benefits of AI software extend across all aspects of business operations, from increasing efficiency and reducing costs to enhancing customer experiences and providing data-driven insights. As AI technology continues to evolve, its potential to drive innovation and competitive advantage grows, enabling businesses to stay agile and thrive in an increasingly dynamic marketplace. Whether it’s improving decision-making, enhancing security, or scaling operations, AI offers unprecedented opportunities for organizations to improve their bottom line and deliver better results for customers and stakeholders alike.
5. Challenges of AI Software
While AI software brings transformative benefits to various industries, its implementation and use also present several challenges. These challenges can range from technical and ethical issues to financial and operational obstacles. It’s important for businesses and developers to understand these challenges to mitigate risks and ensure the responsible deployment of AI systems. In this section, we will explore the key challenges of AI software and provide relevant examples to highlight their impact.
1. Data Privacy and Security
One of the major concerns surrounding AI software is the handling of sensitive data. AI systems often rely on large datasets to learn and make decisions. If this data is not handled properly, it can lead to privacy breaches, data theft, and other security issues.
- Sensitive Data Handling:
- AI systems require access to vast amounts of data, including personally identifiable information (PII), which raises concerns about how that data is stored, processed, and protected.
- Example: Health data used by AI-powered diagnostic tools needs to be encrypted and anonymized to avoid privacy violations and meet regulatory standards such as HIPAA.
- Data Breaches:
- Hackers targeting AI systems may steal critical data or inject malicious data to manipulate AI’s decision-making capabilities.
- Example: In 2020, AI-based facial recognition systems were compromised in various security breaches, exposing sensitive biometric data.
- Compliance with Regulations:
- Strict regulations, such as GDPR (General Data Protection Regulation) in Europe, impose legal requirements on how AI systems must handle data privacy, creating compliance challenges for businesses.
- Example: Companies using AI to analyze consumer data for personalized marketing must ensure that their practices align with data protection laws to avoid penalties.
2. Ethical Issues and Bias
AI systems are only as unbiased and ethical as the data they are trained on and the algorithms that drive them. Bias in AI models can lead to unfair or discriminatory outcomes, raising concerns about the ethical implications of AI.
- Algorithmic Bias:
- If AI systems are trained on biased datasets, the resulting decisions or predictions can reflect societal inequalities, amplifying issues like racial or gender discrimination.
- Example: In 2018, Amazon scrapped an AI-powered recruitment tool that showed a preference for male candidates, as the system was trained on resumes that were predominantly male.
- Lack of Transparency (Black Box Problem):
- Many AI algorithms, especially deep learning models, operate as “black boxes,” meaning it is difficult to understand how they arrive at decisions. This lack of transparency raises concerns about accountability.
- Example: AI in criminal justice systems, such as risk assessment tools used in sentencing, often provides decisions that are difficult to explain, making it hard to hold the system accountable for biased outcomes.
- Unintended Consequences:
- AI models may make decisions that are unintended or harmful, especially if they are not properly tested or monitored.
- Example: A self-driving car might make a decision that prioritizes the safety of the driver over pedestrians in an accident scenario, leading to ethical dilemmas about whose safety should be prioritized.
3. High Costs of Development and Implementation
While AI software offers significant benefits, the development, deployment, and maintenance of AI systems can be expensive. The costs involved may limit access to AI technology for smaller businesses or organizations with fewer resources.
- Initial Investment:
- Developing AI solutions requires significant investment in infrastructure, including high-performance hardware, specialized software, and skilled personnel.
- Example: To train AI models, companies often need access to expensive computing resources, such as cloud infrastructure or powerful GPUs (Graphics Processing Units), which can drive up the initial cost.
- Ongoing Maintenance and Updates:
- AI models require continuous monitoring, retraining, and updating to remain effective and accurate over time, which can add substantial operational costs.
- Example: A recommendation system for an e-commerce site needs to be updated frequently with new data to ensure its recommendations remain relevant to customers, leading to ongoing expenses.
- Talent Shortage:
- AI development requires specialized knowledge and expertise in areas such as machine learning, data science, and natural language processing, leading to a shortage of qualified professionals.
- Example: Many tech companies struggle to find skilled AI engineers and data scientists to meet the demand for AI software development, driving up labor costs.
4. Integration with Existing Systems
Integrating AI software into legacy systems can be a complex process. Many businesses operate with outdated infrastructure that may not be compatible with advanced AI technologies, requiring substantial rework or new system architecture.
- Legacy Systems Compatibility:
- Older systems may not be able to support the data processing needs of AI software, making integration time-consuming and costly.
- Example: A bank with legacy IT systems may find it difficult to integrate AI-powered fraud detection tools with its outdated transaction processing systems.
- Data Silos:
- AI models often require data from multiple sources, but many organizations have data stored in silos, making it difficult to consolidate and share that data for AI analysis.
- Example: In manufacturing, siloed data from different machines or departments can hinder the ability to apply AI-driven predictive maintenance solutions effectively.
- Customization and Adaptation:
- AI solutions may need to be customized to suit the specific needs of an organization, which can require additional development work.
- Example: A healthcare provider implementing an AI-powered patient management system may need to tailor the software to accommodate specific workflows and patient care processes.
5. Limited Generalization Across Industries
AI software tends to be highly specialized, meaning that models trained in one industry or domain may not be easily transferable to another without significant retraining and adjustment. This limits AI’s effectiveness when businesses seek to adopt AI across various sectors.
- Domain-Specific Models:
- AI models are often tailored to specific industries or tasks, which means they may not generalize well to new applications or sectors.
- Example: An AI system trained for medical image analysis may not perform effectively when used in other industries, such as retail or manufacturing, without substantial retraining.
- Data Dependency:
- AI software relies heavily on data specific to its intended use case. Without sufficient quality data in a new domain, AI models may underperform or fail altogether.
- Example: AI models trained on large consumer datasets may struggle to make accurate predictions in niche industries like agriculture or forestry, where data is less abundant.
6. Dependency on High-Quality Data
AI systems are highly dependent on the quality of the data they are trained on. Poor or incomplete data can lead to inaccurate or biased predictions, which compromises the value of AI software.
- Data Quality Issues:
- Inaccurate, incomplete, or biased data can result in AI models that produce unreliable outcomes or reinforce societal biases.
- Example: An AI algorithm used in recruitment may perform poorly if it is trained on historical hiring data that includes biases based on gender, ethnicity, or age.
- Data Cleaning and Preprocessing:
- Before AI systems can be trained, large datasets often need to be cleaned and preprocessed to remove noise and irrelevant information. This process can be time-consuming and resource-intensive.
- Example: In the finance industry, AI models used for credit scoring may require extensive preprocessing of financial transaction data to ensure that it is clean and accurate.
- Data Imbalance:
- In some cases, datasets used to train AI systems may suffer from imbalances, where certain classes of data are overrepresented or underrepresented, leading to skewed outcomes.
- Example: An AI system trained to detect fraudulent transactions might be biased toward detecting fraud in high-volume transactions, underperforming on low-frequency, high-risk fraud scenarios.
7. Lack of Trust and Adoption
Despite the many advantages AI offers, there is often reluctance to trust and adopt AI technologies. This lack of trust stems from concerns about reliability, transparency, and accountability, especially in mission-critical applications.
- Fear of Job Losses:
- One of the major concerns with AI is its potential to replace human workers, leading to job displacement. This fear can create resistance to AI adoption, particularly in industries reliant on manual labor.
- Example: Manufacturing workers may resist the adoption of AI-powered robots and automation tools that threaten their jobs, even if these tools ultimately improve efficiency and reduce costs.
- Trust in AI Decision-Making:
- AI systems that operate as “black boxes” may struggle to gain trust from users, especially when it is difficult to explain how decisions are made.
- Example: In healthcare, doctors may hesitate to rely on AI software for diagnoses if they cannot understand how the system arrived at a particular conclusion, even if the AI’s accuracy is high.
- Ethical Concerns:
- The ethical implications of AI, including concerns over fairness, accountability, and transparency, can slow down its adoption.
- Example: Public opposition to AI-based surveillance systems in cities often stems from concerns about privacy and potential misuse of collected data.
Conclusion
The challenges of AI software are substantial and multifaceted, ranging from data privacy and security issues to ethical dilemmas and high development costs. Addressing these challenges is essential for ensuring that AI systems are deployed responsibly and effectively. While overcoming these hurdles requires significant effort and resources, the benefits of AI software—when used appropriately—can far outweigh the risks. By understanding these challenges and implementing strategies to address them, businesses can unlock the full potential of AI and drive innovation across industries.
6. Future of AI Software
Artificial Intelligence (AI) software continues to evolve at an unprecedented pace, revolutionizing industries and transforming human lives. As the capabilities of AI expand, its future promises more advanced applications, deeper integration into daily life, and groundbreaking innovations.
1. Advancements in Machine Learning and Deep Learning
- Improved Algorithms
- Future AI software will feature more efficient machine learning (ML) algorithms capable of processing vast amounts of data faster.
- Examples include advancements in reinforcement learning for autonomous vehicles and robotics.
- Greater Personalization
- AI systems will increasingly use deep learning to offer hyper-personalized recommendations in areas like healthcare, e-commerce, and entertainment.
- For instance, streaming platforms like Netflix could refine content suggestions using deeper contextual understanding.
- Explainable AI (XAI)
- Development of AI models that can explain their decision-making processes will enhance transparency and trust.
- Example: AI diagnostic tools in healthcare offering rationale for medical decisions.
2. Integration with Emerging Technologies
- AI and IoT Convergence
- AI software will power smart devices in the Internet of Things (IoT), enabling predictive maintenance, enhanced automation, and real-time analytics.
- Example: Smart homes using AI to optimize energy consumption and improve security.
- AI in Blockchain
- Combining AI with blockchain can lead to more secure and efficient systems for data management and verification.
- Example: AI-driven blockchain solutions for fraud detection in financial services.
- Quantum Computing
- AI software leveraging quantum computing will solve complex problems much faster, particularly in drug discovery and climate modeling.
- Example: AI-accelerated simulations to find potential treatments for rare diseases.
3. AI in Ethical and Sustainable Innovation
- Focus on Ethical AI
- Governments and organizations will prioritize ethical guidelines to ensure responsible AI development.
- Example: Bias-reduction tools in hiring software to promote diversity.
- Sustainability Initiatives
- AI will play a significant role in addressing global challenges like climate change and resource optimization.
- Example: AI-powered agricultural tools improving crop yields while reducing environmental impact.
4. Expansion in Real-World Applications
- AI in Healthcare
- AI software will enhance precision medicine, telehealth, and early disease detection.
- Example: AI algorithms identifying cancer at earlier stages through imaging analysis.
- Education Revolution
- AI-driven educational platforms will offer personalized learning experiences, catering to diverse learning styles and needs.
- Example: Adaptive learning software like Duolingo providing tailored language lessons.
- AI in Entertainment
- AI will redefine content creation in films, music, and video games through generative AI tools.
- Example: AI generating entire movie scripts or designing game levels autonomously.
5. Increased Accessibility and Affordability
- Democratization of AI Tools
- AI software will become more accessible to small businesses and individual users.
- Example: Low-code or no-code AI platforms allowing non-technical users to create applications.
- Reduced Costs
- As hardware costs decline and cloud-based AI platforms expand, implementing AI will become more affordable.
- Example: Startups using cloud AI services to automate customer support.
6. Advanced Human-AI Collaboration
- AI as a Co-Creator
- AI will increasingly collaborate with humans in creative fields like design, art, and writing.
- Example: Designers using AI tools like DALL-E for generating initial concepts.
- Enhanced Decision-Making
- AI software will act as decision-support systems in sectors such as finance, law, and policy-making.
- Example: Predictive analytics tools helping businesses anticipate market trends.
7. Potential Challenges in AI’s Future
- Job Displacement
- Automation driven by AI could lead to job losses in certain industries, prompting the need for reskilling programs.
- Example: AI automating repetitive tasks in manufacturing.
- Cybersecurity Risks
- As AI becomes more advanced, cyber threats leveraging AI will also rise, necessitating robust defense mechanisms.
- Example: AI detecting phishing scams in real time.
Final Thoughts
The future of AI software is rich with opportunities to transform industries, solve global problems, and enhance human capabilities. However, it is essential to address challenges responsibly, ensuring AI benefits society while fostering ethical development.
Conclusion
Artificial Intelligence (AI) software has emerged as one of the most transformative technologies in the modern world, revolutionizing industries and reshaping how businesses operate. From enhancing customer experiences to driving innovation in sectors like healthcare, finance, and retail, AI software has vast potential. Understanding what AI software is, how it works, and the key technologies that power it is crucial for businesses and individuals who wish to leverage its capabilities effectively.
A Recap of AI Software: Its Definition and Working Mechanisms
AI software encompasses a broad range of tools and technologies designed to replicate human intelligence in machines. These systems can analyze large datasets, recognize patterns, and make decisions or predictions based on this data. The working of AI software is powered by machine learning (ML), deep learning (DL), natural language processing (NLP), and computer vision, among other technologies. These core technologies enable AI systems to “learn” from data and improve their performance over time, allowing them to carry out tasks such as image recognition, speech analysis, and predictive analytics with remarkable accuracy.
The Technological Backbone of AI Software
The benefits of AI are deeply tied to the technologies that underpin it. AI software thrives on advancements in deep learning algorithms, neural networks, and advanced data processing techniques. Key technologies, such as neural networks mimicking the human brain and large-scale data processing through cloud computing, provide the foundation for AI’s remarkable capabilities. AI’s reliance on vast amounts of data ensures that it continually improves, often outperforming traditional systems that rely on static algorithms or pre-programmed logic.
AI-powered tools also benefit from natural language processing, which enables machines to interact with humans in more human-like ways, as seen in virtual assistants like Siri or Alexa. Similarly, computer vision allows AI systems to interpret visual data, making it possible for industries such as automotive (through autonomous vehicles) and healthcare (with AI diagnostic tools) to advance rapidly.
Key Benefits of AI Software: Efficiency, Automation, and Personalization
The benefits of AI software cannot be overstated. By automating repetitive tasks, AI significantly boosts productivity, reduces human error, and streamlines business operations. In customer service, AI-powered chatbots can handle customer inquiries 24/7, providing instant responses and freeing up human agents for more complex tasks. In the manufacturing sector, AI-driven automation is enhancing production lines, improving both speed and quality.
AI also excels at personalizing experiences. In the retail sector, AI algorithms analyze consumer behavior to deliver targeted recommendations, driving higher conversion rates. AI’s role in predictive analytics further contributes to business success by enabling companies to forecast market trends and consumer needs, ensuring that they remain competitive in an ever-evolving landscape.
Moreover, AI has revolutionized sectors like healthcare, where AI-powered diagnostic tools assist doctors in identifying diseases earlier, leading to better patient outcomes. In finance, AI is used for fraud detection, optimizing trading strategies, and managing risk, providing businesses with better decision-making capabilities.
The Challenges AI Software Faces: Data Privacy, Bias, and Costs
However, despite the immense promise AI holds, it is not without its challenges. Data privacy remains a key concern, as AI software often requires access to large sets of sensitive data. If this data is mishandled or exposed, it could lead to breaches of privacy and undermine trust in AI applications. Moreover, ethical issues such as algorithmic bias and lack of transparency in AI decision-making processes have raised alarms across industries, particularly in sectors like hiring and criminal justice.
The financial cost of developing and implementing AI software also cannot be ignored. For small and medium-sized businesses, the resources required to integrate AI solutions into existing systems can be prohibitive. Furthermore, ongoing maintenance, retraining, and adaptation of AI models require sustained investment, posing financial hurdles for organizations with limited budgets.
Additionally, the limited generalization of AI systems across different domains means that businesses must invest considerable time and effort into customizing AI models to suit their specific needs. Despite its potential, the technology is still not universally adaptable across all industries without significant adjustments.
Moving Forward: The Future of AI Software
As AI software continues to evolve, so too do the opportunities and challenges associated with it. In the coming years, advancements in AI technologies, coupled with better data governance practices, are expected to mitigate many of the current challenges. For example, enhanced data encryption methods and privacy standards could help address concerns related to data security. Similarly, the development of more transparent and interpretable AI models is likely to improve trust in AI decision-making, particularly in sensitive fields such as healthcare and finance.
The future of AI also holds significant promise as it integrates more deeply with emerging technologies such as quantum computing and 5G. These innovations will likely drive even more powerful AI systems capable of solving complex problems at previously unimaginable speeds.
Moreover, AI’s role in augmenting human capabilities, rather than replacing them, will continue to grow. For instance, AI may enable workers to focus on more strategic, creative tasks while automating mundane operations, enhancing job satisfaction and productivity.
Conclusion: AI Software’s Potential and the Path Forward
In conclusion, AI software stands at the forefront of technological innovation, capable of revolutionizing industries, enhancing decision-making, and improving efficiency across sectors. While challenges related to privacy, bias, and costs remain, AI’s transformative potential cannot be ignored. The key to unlocking AI’s true potential lies in addressing these challenges through responsible development, ethical guidelines, and continuous advancements in technology.
For businesses looking to stay competitive, investing in AI software is no longer optional—it’s a necessity. By understanding how AI works, the key technologies that power it, and the potential benefits it offers, organizations can unlock new levels of efficiency, innovation, and growth. As AI continues to evolve, its applications will only become more widespread, shaping the future of technology and society as a whole.
If you find this article useful, why not share it with your hiring manager and C-level suite friends and also leave a nice comment below?
We, at the 9cv9 Research Team, strive to bring the latest and most meaningful data, guides, and statistics to your doorstep.
To get access to top-quality guides, click over to 9cv9 Blog.
People Also Ask
What is Artificial Intelligence (AI) software?
AI software refers to systems designed to perform tasks that typically require human intelligence, such as decision-making, speech recognition, and problem-solving, by processing data and learning from patterns.
How does AI software work?
AI software uses algorithms, machine learning models, and vast datasets to recognize patterns, make predictions, and improve its performance over time without human intervention, learning from each interaction.
What are the key components of AI software?
The key components include machine learning algorithms, neural networks, natural language processing (NLP), and computer vision, which enable AI to perform tasks like analyzing data, understanding language, and recognizing images.
What are the benefits of using AI software?
AI software offers increased efficiency, automation of repetitive tasks, data analysis for better decision-making, personalized customer experiences, and the ability to work continuously without human fatigue.
What are some examples of AI software?
Examples include virtual assistants like Siri and Alexa, recommendation systems on platforms like Netflix, AI-driven diagnostic tools in healthcare, and autonomous vehicles powered by computer vision and machine learning.
How is AI used in businesses?
Businesses use AI for automating processes, improving customer service (via chatbots), predicting trends, analyzing data, enhancing personalization, and optimizing operations like inventory management and marketing campaigns.
What industries benefit the most from AI software?
AI is transforming industries such as healthcare, finance, automotive, retail, and manufacturing by enhancing decision-making, improving efficiency, and enabling innovations like predictive maintenance and personalized recommendations.
What is machine learning in AI?
Machine learning is a subset of AI where systems learn from data and improve their performance over time. It involves training algorithms to identify patterns and make predictions without explicit programming.
How does deep learning differ from machine learning?
Deep learning is a subset of machine learning that uses neural networks with many layers to analyze complex data like images and voice, while machine learning typically uses simpler algorithms to analyze smaller datasets.
What is natural language processing (NLP) in AI?
NLP enables AI software to understand, interpret, and generate human language. It powers virtual assistants, chatbots, translation tools, and sentiment analysis, allowing machines to communicate with humans more effectively.
What is computer vision in AI?
Computer vision allows AI systems to interpret and process visual data from the world, enabling applications like facial recognition, object detection, and self-driving cars to navigate environments based on images and video.
Can AI software think like humans?
While AI software can simulate human thinking by processing large amounts of data and identifying patterns, it does not have consciousness or emotions. It operates based on algorithms and learned data, not human reasoning.
How does AI improve customer service?
AI improves customer service by automating responses via chatbots, providing personalized recommendations, analyzing customer behavior to predict needs, and offering 24/7 support without human agents being required.
What is the role of data in AI software?
Data plays a crucial role in AI software, as it serves as the foundation for training algorithms and machine learning models. The more high-quality data AI systems process, the better their performance and accuracy.
What are the challenges of using AI software?
Challenges include data privacy concerns, ethical issues like bias in AI algorithms, the high cost of development and implementation, and the complexity of integrating AI into existing systems.
How does AI software learn and improve over time?
AI software learns through continuous exposure to data. It uses algorithms to identify patterns, make predictions, and adjust its behavior based on feedback, improving accuracy and efficiency with each iteration.
How does AI impact job roles and employment?
AI automation may replace repetitive jobs but also creates new opportunities in fields like AI development, data science, and cybersecurity. It enables workers to focus on more strategic, creative tasks by automating routine processes.
Can AI software make decisions on its own?
AI software can make decisions based on the data it processes. However, these decisions are rooted in patterns, statistical probabilities, and programmed algorithms, rather than independent reasoning or judgment.
How can AI be used for predictive analytics?
AI uses historical data to make predictions about future trends. In industries like finance or marketing, AI models forecast sales, customer behavior, and market movements, helping businesses make data-driven decisions.
What are neural networks in AI?
Neural networks are a key part of deep learning that simulate the human brain’s neurons. They consist of interconnected layers of algorithms designed to recognize patterns, making them essential for tasks like image and speech recognition.
What is reinforcement learning in AI?
Reinforcement learning is a type of machine learning where AI agents learn by interacting with their environment. They receive feedback in the form of rewards or penalties based on their actions, which helps improve decision-making.
How does AI handle large volumes of data?
AI software processes large volumes of data using advanced algorithms and cloud computing, enabling it to analyze massive datasets quickly and efficiently, finding patterns and insights that would be impossible for humans to extract manually.
What is AI’s role in autonomous vehicles?
AI enables autonomous vehicles to navigate and make decisions by using sensors, computer vision, and machine learning algorithms to interpret their surroundings, avoid obstacles, and drive safely without human input.
How does AI improve healthcare?
AI software assists healthcare professionals by analyzing medical images, predicting patient outcomes, diagnosing diseases early, and personalizing treatment plans based on data-driven insights, enhancing patient care and efficiency.
What is the difference between narrow AI and general AI?
Narrow AI is designed for specific tasks, such as language translation or image recognition, while general AI refers to a theoretical machine capable of performing any intellectual task that a human can do, which is still under development.
Can AI software replace human creativity?
AI software can assist with creative tasks, such as generating art or music, but it does not possess true creativity or emotional depth. It replicates patterns based on data and existing human work, lacking independent artistic vision.
What role does AI play in cybersecurity?
AI enhances cybersecurity by detecting anomalies in network traffic, identifying potential threats, and automating responses to security breaches. AI can quickly analyze large datasets to identify patterns indicative of malicious activities.
What ethical concerns are associated with AI?
Ethical concerns include algorithmic bias, where AI systems may unintentionally favor certain groups, lack of transparency in decision-making, data privacy issues, and the potential for AI to be used for malicious purposes.
How is AI software used in finance?
AI is used in finance for fraud detection, optimizing investment strategies, algorithmic trading, and customer service. It can analyze vast amounts of financial data in real-time, making predictions and providing valuable insights.
What is the future of AI software?
The future of AI software includes advancements in machine learning, natural language processing, and deep learning, leading to more intelligent systems that can adapt to a wider range of tasks, offering even more personalized and accurate solutions.
How does AI improve marketing strategies?
AI enhances marketing by analyzing customer data to identify patterns, creating personalized campaigns, automating content creation, and optimizing advertising strategies in real-time to improve engagement and ROI.
Can AI software be self-aware?
No, AI software is not self-aware. While it can simulate human-like responses and adapt based on data, it does not possess consciousness or emotions. AI operates through algorithms and learned patterns, not independent thought.
How is AI integrated into everyday life?
AI is integrated into everyday life through applications like voice assistants (Siri, Alexa), recommendation systems on streaming platforms (Netflix, Spotify), autonomous vehicles, personalized marketing, and AI-based customer service chatbots.
Is AI software expensive to implement?
Yes, AI software can be expensive to implement, especially for small businesses. Costs include the development of custom solutions, acquiring large datasets, integrating AI with existing systems, and ongoing maintenance and updates.