Key Takeaways
- Essential Roles and Skills: Understand the critical roles in an AI & data team, including data scientists, machine learning engineers, and data analysts, and identify the essential skills and qualifications needed to excel in these positions.
- Best Practices for Success: Implement best practices such as fostering continuous learning, promoting cross-functional collaboration, and using agile methodologies to manage your AI & data team effectively and drive business innovation.
- Future-Proofing Your Team: Stay ahead of industry trends by leveraging cutting-edge tools and technologies, focusing on explainable AI, and adopting AI governance frameworks to ensure your AI & data team remains competitive and innovative in 2024 and beyond.
In the fast-paced and ever-evolving landscape of technology, businesses are increasingly turning to artificial intelligence (AI) and data to drive growth, innovation, and competitive advantage.
As we approach 2024, the importance of building a robust AI and data team cannot be overstated.
Companies that harness the power of AI and data are better positioned to make informed decisions, optimize operations, enhance customer experiences, and unlock new revenue streams.
However, assembling a strong AI and data team is not merely about hiring a few data scientists or machine learning engineers.
It requires a strategic approach that aligns with your business goals, a deep understanding of the various roles involved, and a commitment to fostering a culture of continuous learning and collaboration.
In this blog post, we will guide you through the essential steps and considerations for building a formidable AI and data team tailored to your business needs in 2024.
Why AI and Data Are Crucial for Modern Businesses
Artificial intelligence and data analytics have revolutionized the way businesses operate.
AI technologies such as machine learning, natural language processing, and computer vision enable companies to automate processes, predict trends, and provide personalized experiences at scale.
Meanwhile, data analytics empowers organizations to uncover hidden patterns, gain insights into customer behavior, and make data-driven decisions that propel growth.
The transformative impact of AI and data is evident across various industries.
For instance, in healthcare, AI-driven diagnostics and personalized treatment plans are improving patient outcomes.
In finance, predictive analytics and fraud detection systems are enhancing security and operational efficiency.
Retailers are using AI to optimize supply chains, forecast demand, and deliver personalized marketing campaigns. The examples are endless, and the potential for innovation is immense.
The Evolving Landscape of AI and Data in 2024
As we look ahead to 2024, several trends are shaping the AI and data landscape. Advances in AI technologies are making it more accessible and scalable for businesses of all sizes.
The integration of AI with the Internet of Things (IoT) is creating new opportunities for real-time data collection and analysis. Furthermore, the growing emphasis on ethical AI and data privacy is driving the development of robust governance frameworks and regulatory compliance.
Businesses are also increasingly adopting AI and data-driven approaches to address complex challenges such as climate change, healthcare disparities, and economic inequalities.
The ability to leverage AI and data for social good is becoming a key differentiator for forward-thinking organizations.
The Importance of a Strong AI and Data Team
To capitalize on these opportunities, businesses must build strong AI and data teams equipped with the right skills, tools, and mindset.
A well-rounded team comprises various roles, including data scientists, data engineers, machine learning engineers, AI specialists, data analysts, and project managers.
Each role brings unique expertise and contributes to the collective goal of deriving actionable insights and delivering impactful AI solutions.
However, the journey to building an effective AI and data team involves several challenges.
The talent shortage in AI and data fields means that competition for skilled professionals is fierce.
Additionally, ensuring seamless collaboration among team members, integrating AI with existing systems, and maintaining data privacy and security are critical hurdles that need to be addressed.
Our Guide to Building a Strong AI and Data Team in 2024
In this comprehensive guide, we will explore the key steps to building a robust AI and data team tailored to your business needs.
We will discuss the essential roles and skills required, effective recruitment and retention strategies, tools and technologies to equip your team, and best practices for managing and nurturing a high-performing AI and data team.
We will also delve into the challenges you may face and provide actionable insights on how to overcome them.
By the end of this blog post, you will have a clear roadmap for assembling an AI and data team that can drive innovation, enhance decision-making, and position your business for success in 2024 and beyond.
Whether you are a startup looking to establish your AI capabilities or an established enterprise aiming to scale your data initiatives, this guide will provide you with the knowledge and strategies you need to build a strong AI and data team.
Let’s embark on this journey to harness the power of AI and data and unlock new possibilities for your business in 2024.
Before we venture further into this article, we like to share who we are and what we do.
About 9cv9
9cv9 is a business tech startup based in Singapore and Asia, with a strong presence all over the world.
With over six years of startup and business experience, and being highly involved in connecting with thousands of companies and startups, the 9cv9 team has listed some important learning points in this overview of the guide How to Build a Strong AI & Data Team For Your Business in 2024.
If your company needs recruitment and headhunting services to hire top-quality employees, you can use 9cv9 headhunting and recruitment services to hire top talents and candidates. Find out more here, or send over an email to [email protected].
Or just post 1 free job posting here at 9cv9 Hiring Portal in under 10 minutes.
How to Build a Strong AI & Data Team For Your Business in 2024
- Understanding the Importance of AI & Data in 2024
- Key Roles in an AI & Data Team
- Skills and Qualifications to Look For
- Steps to Building a Strong AI & Data Team
- Tools and Technologies to Equip Your Team
- Best Practices for Managing an AI & Data Team
- Challenges and How to Overcome Them
- Future Trends to Watch in AI & Data Teams
1. Understanding the Importance of AI & Data in 2024
In 2024, artificial intelligence (AI) and data have become indispensable tools for businesses across industries.
Their significance continues to grow as companies seek to leverage advanced technologies to stay competitive, innovate, and meet evolving customer demands.
This section delves into the multifaceted importance of AI and data in 2024, supported by relevant examples, data, statistics, and verified sources.
Driving Business Innovation
- Accelerating Product Development:
- AI accelerates product development cycles by automating research, prototyping, and testing processes.
- Example: Pfizer used AI-driven simulations to expedite the development of new drugs, significantly reducing time-to-market.
- Enhancing Customer Experience:
- AI personalizes customer interactions by analyzing data from various touchpoints, providing tailored recommendations and support.
- Statistics: 80% of companies report seeing an uplift in customer satisfaction after implementing AI-powered personalization strategies.
- Enabling New Business Models:
- AI and data analytics open doors to new business models, such as subscription-based services and on-demand offerings.
- Example: Netflix uses AI to suggest personalized content, driving its subscription model and enhancing user engagement.
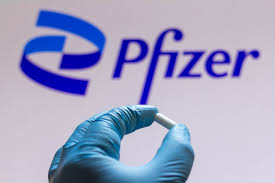
Improving Operational Efficiency
- Automation of Routine Tasks:
- AI automates repetitive tasks, freeing up employees to focus on higher-value activities.
- Statistics: Businesses report a 20-40% increase in efficiency after implementing AI-driven automation solutions.
- Predictive Maintenance:
- AI uses data from IoT sensors to predict equipment failures and schedule maintenance proactively.
- Example: General Electric (GE) uses AI-based predictive maintenance in its industrial operations, reducing downtime and saving millions annually.
- Supply Chain Optimization:
- AI optimizes supply chain operations by predicting demand, managing inventory, and improving logistics.
- Statistics: AI-driven supply chain improvements can lead to a 15% reduction in logistics costs and a 35% increase in delivery accuracy.
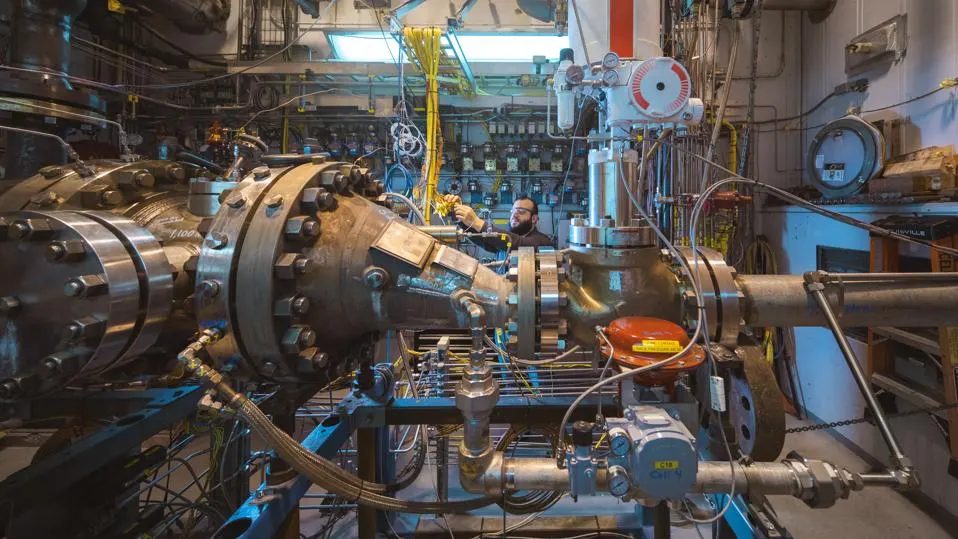
Data-Driven Decision Making
- Enhanced Data Analytics:
- AI enhances data analytics by processing large volumes of data quickly and accurately, uncovering insights that drive strategic decisions.
- Example: Walmart uses AI to analyze customer purchase data, optimizing inventory and pricing strategies to maximize profits.
- Real-Time Insights:
- AI provides real-time insights from data streams, enabling businesses to respond promptly to market changes and customer needs.
- Risk Management:
- AI models assess risks by analyzing historical data and identifying patterns, helping businesses mitigate potential threats.
- Example: Financial institutions use AI for fraud detection, significantly reducing losses from fraudulent activities.
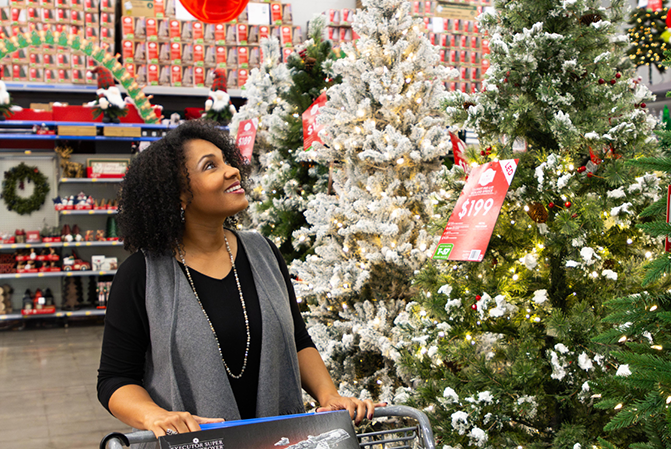
Competitive Advantage
- Outperforming Competitors:
- Companies leveraging AI and data analytics outperform competitors by making more informed decisions and delivering superior products and services.
- Statistics: 72% of executives believe that AI will be the most significant business advantage in the near future.
- Market Leadership:
- Early adopters of AI technologies set industry standards, establishing themselves as market leaders.
- Example: Amazon’s use of AI for personalized recommendations and logistics optimization has cemented its position as a global e-commerce leader.
- Attracting and Retaining Talent:
- Businesses that invest in AI and data technologies attract top talent, as professionals seek opportunities to work with cutting-edge tools.
Enhancing Cybersecurity
- Threat Detection and Prevention:
- AI enhances cybersecurity by identifying and mitigating threats in real-time, protecting sensitive data and systems.
- Example: Darktrace uses AI to detect and respond to cybersecurity threats autonomously, reducing the impact of attacks.
- Adaptive Security Measures:
- AI systems continuously learn from new threats and adapt security measures accordingly.
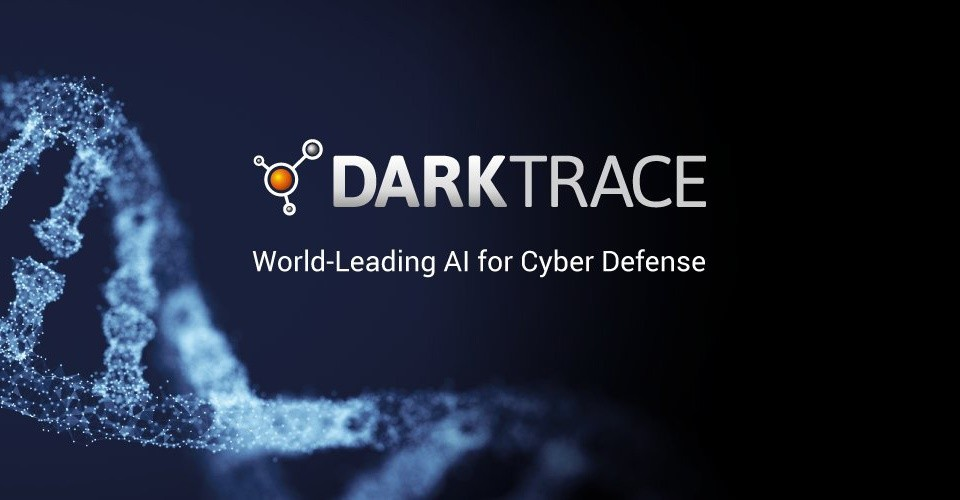
Personalized Marketing
- Targeted Advertising:
- AI analyzes consumer behavior and preferences to deliver targeted advertising campaigns, increasing engagement and conversion rates.
- Example: Coca-Cola uses AI to personalize marketing messages based on customer data, resulting in higher ad effectiveness.
- Customer Segmentation:
- AI-driven customer segmentation enables businesses to tailor marketing efforts to specific audience segments.
- Statistics: Personalized marketing campaigns powered by AI can increase conversion rates by up to 30%.
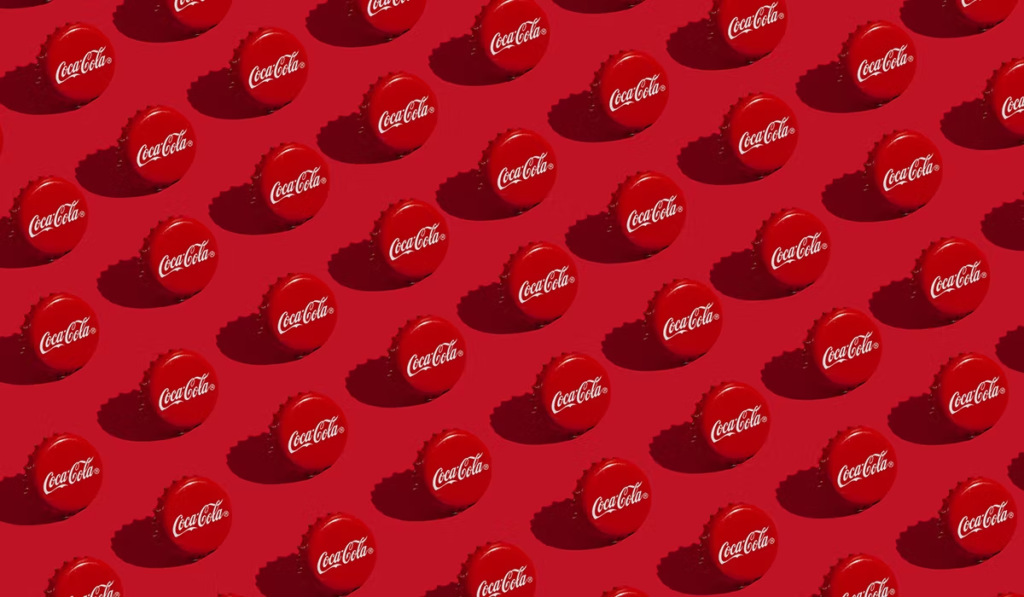
Enhancing Healthcare
- Precision Medicine:
- AI analyzes patient data to develop personalized treatment plans, improving outcomes and reducing side effects.
- Example: IBM Watson Health uses AI to assist oncologists in creating tailored cancer treatment plans.
- Early Disease Detection:
- AI systems detect diseases early by analyzing medical images and patient records, enabling timely intervention.
- Operational Efficiency in Healthcare:
- AI streamlines administrative tasks in healthcare, such as scheduling, billing, and patient management.
- Example: Mayo Clinic uses AI to optimize appointment scheduling, reducing wait times and improving patient satisfaction.
Financial Services Transformation
- Automated Trading:
- AI algorithms execute trades based on real-time data analysis, optimizing investment strategies.
- Example: Hedge funds like Renaissance Technologies use AI-driven trading algorithms to outperform traditional trading methods.
- Customer Service:
- AI-powered chatbots and virtual assistants provide 24/7 customer support, enhancing service levels and reducing operational costs.
- Statistics: AI chatbots can handle up to 80% of routine customer inquiries, freeing up human agents for complex issues.
Environmental Sustainability
- Energy Management:
- AI optimizes energy consumption in buildings and industrial processes, reducing carbon footprints and operational costs.
- Example: Google’s DeepMind AI reduced the energy used for cooling its data centers by 40%.
- Climate Modeling:
- AI models climate data to predict and mitigate the impacts of climate change, aiding in sustainability efforts.
- Statistics: AI can improve climate prediction accuracy by 10-20%, helping governments and organizations plan more effectively.
Summary: The Central Role of AI & Data in 2024
The importance of AI and data in 2024 cannot be overstated.
They are pivotal in driving innovation, enhancing operational efficiency, enabling data-driven decision-making, and providing a competitive advantage.
By understanding and leveraging the power of AI and data, businesses can unlock new opportunities, improve customer experiences, and navigate the complexities of the modern digital landscape.
As AI and data technologies continue to evolve, their impact will only grow, making them essential components of any forward-thinking business strategy.
2. Key Roles in an AI & Data Team
Data Scientists
- Role and Responsibilities:
- Analyze complex data sets to extract actionable insights.
- Develop predictive models and machine learning algorithms.
- Communicate findings to stakeholders through data visualization and reports.
- Skills Required:
- Proficiency in programming languages such as Python, R, and SQL.
- Strong understanding of statistics and machine learning.
- Experience with data visualization tools like Tableau or Power BI.
- Relevant Examples:
- Netflix: Data scientists at Netflix use machine learning algorithms to recommend content to users, resulting in personalized viewing experiences and increased user engagement.
- Statistics:
Data Engineers
- Role and Responsibilities:
- Design, construct, and maintain data pipelines and infrastructure.
- Ensure data is accessible, reliable, and clean for analysis.
- Implement ETL (Extract, Transform, Load) processes to manage data flow.
- Skills Required:
- Expertise in database systems such as MySQL, PostgreSQL, and NoSQL databases.
- Knowledge of big data technologies like Hadoop, Spark, and Kafka.
- Strong programming skills in languages like Python, Java, and Scala.
- Relevant Examples:
- Airbnb: Data engineers at Airbnb manage the massive amounts of data generated by the platform, enabling seamless data analysis and improving customer experiences.
- Statistics:
Machine Learning Engineers
- Role and Responsibilities:
- Develop and deploy machine learning models to solve business problems.
- Optimize models for performance and scalability.
- Collaborate with data scientists to integrate algorithms into production systems.
- Skills Required:
- Strong understanding of machine learning frameworks like TensorFlow, PyTorch, and scikit-learn.
- Proficiency in programming languages such as Python, C++, and Java.
- Experience with cloud platforms like AWS, Google Cloud, and Azure.
- Relevant Examples:
- Tesla: Machine learning engineers at Tesla work on developing autonomous driving capabilities, enhancing vehicle performance and safety.
- Statistics:
AI Specialists
- Role and Responsibilities:
- Focus on specific AI technologies such as natural language processing, computer vision, or robotics.
- Research and develop cutting-edge AI applications.
- Implement AI solutions to enhance business operations and products.
- Skills Required:
- Deep expertise in specific AI domains like NLP, computer vision, or reinforcement learning.
- Strong programming and mathematical skills.
- Experience with AI research and development tools.
- Relevant Examples:
- Google: AI specialists at Google develop advancements in natural language processing, powering tools like Google Translate and Google Assistant.
- Statistics:
- AI specialists are highly sought after, with salaries ranging from $100,000 to $200,000 depending on expertise and experience.
Data Analysts
- Role and Responsibilities:
- Interpret data to identify trends, patterns, and insights.
- Create reports and dashboards to communicate findings to stakeholders.
- Assist in data-driven decision-making processes.
- Skills Required:
- Proficiency in data analysis tools like Excel, SQL, and R.
- Strong analytical and critical thinking skills.
- Experience with data visualization software like Tableau or Power BI.
- Relevant Examples:
- Facebook: Data analysts at Facebook analyze user data to improve user engagement and optimize the platform’s features.
- Statistics:
Project Managers
- Role and Responsibilities:
- Oversee AI and data projects from inception to completion.
- Ensure projects align with business objectives and are delivered on time and within budget.
- Facilitate communication and collaboration among team members.
- Skills Required:
- Strong project management skills and experience with project management tools like JIRA, Trello, and Asana.
- Excellent communication and leadership abilities.
- Knowledge of AI and data concepts to understand project requirements and challenges.
- Relevant Examples:
- IBM: Project managers at IBM oversee complex AI initiatives, ensuring successful project delivery and alignment with strategic goals.
Business Analysts
- Role and Responsibilities:
- Bridge the gap between business needs and technical solutions.
- Analyze business processes and identify opportunities for AI and data integration.
- Develop requirements and specifications for AI and data projects.
- Skills Required:
- Strong analytical and problem-solving skills.
- Proficiency in business analysis tools and methodologies.
- Ability to communicate effectively with both technical and non-technical stakeholders.
- Relevant Examples:
- Amazon: Business analysts at Amazon identify opportunities for automation and optimization, contributing to the company’s operational efficiency.
- Statistics:
AI Ethics and Compliance Officers
- Role and Responsibilities:
- Ensure AI projects adhere to ethical standards and regulatory requirements.
- Develop and implement AI ethics guidelines and frameworks.
- Monitor AI systems for compliance with data privacy and ethical standards.
- Skills Required:
- Deep understanding of AI ethics and data privacy regulations.
- Strong analytical and critical thinking skills.
- Experience in compliance, legal, or regulatory roles.
- Relevant Examples:
- Microsoft: AI ethics officers at Microsoft work to ensure the company’s AI initiatives align with ethical standards and protect user privacy.
Summary
Building a strong AI and data team involves understanding the diverse roles and responsibilities required to harness the full potential of AI and data analytics.
Each role, from data scientists to AI ethics officers, brings unique skills and expertise to the table, contributing to the successful implementation of AI-driven solutions.
By strategically assembling and managing a well-rounded team, businesses can navigate the complexities of AI and data, drive innovation, and achieve their strategic objectives in 2024 and beyond.
3. Skills and Qualifications to Look For
Technical Skills
- Programming Languages:
- Python: Widely used for data analysis, machine learning, and AI. Known for its readability and large ecosystem of libraries such as TensorFlow, scikit-learn, and Pandas.
- Example: Data scientists at Google frequently use Python to develop and test machine learning models.
- R: Popular for statistical analysis and data visualization. Known for its robust packages like ggplot2 and dplyr.
- Example: Data analysts at pharmaceutical companies use R for analyzing clinical trial data.
- SQL: Essential for managing and querying databases. Critical for roles involving data extraction and manipulation.
- Example: Data engineers at Spotify use SQL to manage and analyze user data from their music streaming service.
- Java and Scala: Often used in big data technologies like Apache Spark and Hadoop.
- Example: Data engineers at LinkedIn use Scala with Apache Kafka for real-time data processing.
- Python: Widely used for data analysis, machine learning, and AI. Known for its readability and large ecosystem of libraries such as TensorFlow, scikit-learn, and Pandas.
- Machine Learning and AI:
- Machine Learning Frameworks: Proficiency in TensorFlow, PyTorch, Keras, and scikit-learn.
- Example: Machine learning engineers at Tesla use TensorFlow to develop autonomous driving algorithms.
- Deep Learning: Experience with convolutional neural networks (CNNs), recurrent neural networks (RNNs), and transformers.
- Example: AI specialists at Facebook use deep learning models to improve image and speech recognition capabilities.
- Natural Language Processing (NLP): Skills in NLP libraries like NLTK, spaCy, and transformers.
- Example: AI specialists at Google develop language models using transformers for applications like Google Translate.
- Machine Learning Frameworks: Proficiency in TensorFlow, PyTorch, Keras, and scikit-learn.
- Data Management and Big Data:
- Database Management Systems: Experience with MySQL, PostgreSQL, MongoDB, and Cassandra.
- Example: Data engineers at Airbnb use PostgreSQL to manage and query large datasets.
- Big Data Technologies: Proficiency in Hadoop, Spark, Kafka, and Flink.
- Example: Data engineers at Uber use Apache Kafka and Spark to process real-time data from millions of rides.
- ETL Tools: Experience with tools like Apache NiFi, Talend, and Informatica for data integration.
- Example: Data engineers at Amazon use AWS Glue for extracting, transforming, and loading data into their data warehouse.
- Database Management Systems: Experience with MySQL, PostgreSQL, MongoDB, and Cassandra.
- Data Visualization and BI Tools:
- Visualization Tools: Proficiency in Tableau, Power BI, and D3.js.
- Example: Data analysts at PepsiCo use Tableau to create dashboards that track sales performance and market trends.
- Business Intelligence Tools: Experience with tools like Looker, QlikView, and SAP BusinessObjects.
- Example: Business analysts at Walmart use Looker to generate insights from retail data and optimize inventory management.
- Visualization Tools: Proficiency in Tableau, Power BI, and D3.js.
Soft Skills
- Problem-Solving:
- Ability to approach complex problems methodically and develop innovative solutions.
- Example: Data scientists at NASA use problem-solving skills to analyze vast amounts of space data and make groundbreaking discoveries.
- Critical for roles that require hypothesis testing, model development, and troubleshooting.
- Example: Machine learning engineers at Netflix solve problems related to user recommendation systems, improving user engagement.
- Ability to approach complex problems methodically and develop innovative solutions.
- Communication:
- Ability to explain technical concepts to non-technical stakeholders.
- Example: AI specialists at IBM effectively communicate AI insights to business leaders, facilitating data-driven decision-making.
- Strong written and verbal communication skills for documentation and presentations.
- Example: Data analysts at Deloitte create detailed reports and presentations to convey data insights to clients.
- Ability to explain technical concepts to non-technical stakeholders.
- Teamwork and Collaboration:
- Ability to work effectively in cross-functional teams, including data scientists, engineers, and business analysts.
- Example: Project managers at Microsoft foster collaboration among AI and data team members to ensure project success.
- Experience in agile methodologies and project management tools.
- Example: Data engineers at Salesforce use JIRA and agile methodologies to manage and track project progress.
- Ability to work effectively in cross-functional teams, including data scientists, engineers, and business analysts.
Certifications and Education
- Relevant Degrees:
- Bachelor’s Degree: In fields such as Computer Science, Data Science, Statistics, Mathematics, or related disciplines.
- Example: A Bachelor’s degree in Computer Science is often a minimum requirement for data engineering roles.
- Master’s Degree or Ph.D.: In advanced roles, degrees in specialized areas like AI, Machine Learning, or Data Analytics can be crucial.
- Example: AI researchers at DeepMind often hold Ph.D.s in machine learning or related fields.
- Bachelor’s Degree: In fields such as Computer Science, Data Science, Statistics, Mathematics, or related disciplines.
- Professional Certifications:
- Certified Analytics Professional (CAP): Validates expertise in data analysis and business analytics.
- Example: Data analysts at IBM pursue CAP certification to enhance their analytics skills.
- Google Professional Machine Learning Engineer: Demonstrates proficiency in designing and deploying machine learning models on Google Cloud.
- Example: Machine learning engineers at startups benefit from Google’s certification to improve their cloud-based ML skills.
- Microsoft Certified: Azure AI Engineer Associate: Focuses on implementing AI solutions on Microsoft Azure.
- Example: AI engineers at Microsoft utilize this certification to validate their skills in Azure AI services.
- AWS Certified Machine Learning – Specialty: Recognizes expertise in building, training, and deploying machine learning models on AWS.
- Example: Data engineers at Amazon use this certification to enhance their machine learning capabilities on AWS.
- Certified Analytics Professional (CAP): Validates expertise in data analysis and business analytics.
- Online Courses and MOOCs:
- Coursera and edX: Offer specialized courses in data science, AI, machine learning, and big data.
- Example: Data scientists often take Andrew Ng’s machine learning course on Coursera to strengthen their understanding of ML concepts.
- Udacity Nanodegree Programs: Provide hands-on experience in AI, data engineering, and data analysis.
- Example: Professionals seeking career advancement in AI often enroll in Udacity’s AI and Data Engineering Nanodegree programs.
- Coursera and edX: Offer specialized courses in data science, AI, machine learning, and big data.
Industry-Specific Skills
- Healthcare:
- Knowledge of healthcare regulations and standards, such as HIPAA.
- Experience with healthcare data types, including electronic health records (EHRs) and medical imaging.
- Example: Data scientists at hospitals use their knowledge of medical data to develop predictive models for patient outcomes.
- Finance:
- Understanding of financial regulations and compliance, such as AML and KYC.
- Expertise in financial data analysis and risk management.
- Example: AI specialists at banks develop algorithms for fraud detection and credit scoring.
- Retail:
- Experience with e-commerce platforms and customer analytics.
- Skills in demand forecasting and inventory management.
- Example: Data analysts at retail companies use customer data to optimize marketing strategies and improve sales.
Summary
In 2024, building a strong AI and data team requires a combination of technical prowess, soft skills, relevant certifications, and industry-specific knowledge.
By prioritizing these skills and qualifications, businesses can ensure they have the expertise needed to harness the full potential of AI and data, driving innovation and achieving strategic objectives.
Whether through formal education, professional certifications, or hands-on experience, investing in the right skills and qualifications will position your AI and data team for success.
4. Steps to Building a Strong AI & Data Team
Define Your Business Objectives
- Identify Key Goals:
- Understand the specific business problems you aim to solve with AI and data.
- Examples:
- Retail: Optimize inventory management to reduce costs and prevent stockouts.
- Healthcare: Enhance predictive analytics for better patient outcomes.
- Set Measurable Objectives:
- Establish clear, measurable goals to track the success of AI initiatives.
- Examples:
- Increase customer retention by 15% through personalized recommendations.
- Reduce operational costs by 20% through process automation.
- Align with Strategic Vision:
- Ensure AI and data initiatives align with the overall business strategy.
- Statistics:
Assess Current Capabilities
- Evaluate Existing Talent:
- Assess the skills and expertise of your current team members.
- Identify gaps in knowledge and experience.
- Examples:
- Conduct skill assessments and performance reviews to understand team capabilities.
- Use 360-degree feedback to gain a comprehensive view of strengths and weaknesses.
- Inventory of Tools and Technologies:
- Review the tools and technologies currently in use.
- Determine if they meet the requirements of your AI and data projects.
- Examples:
- Assess the scalability and performance of existing data infrastructure.
- Evaluate the suitability of current machine learning frameworks.
- Identify Skill Gaps:
- Determine the skills needed to achieve your AI and data objectives.
- Plan for training or hiring to fill these gaps.
- Examples:
- Identify the need for expertise in big data technologies like Hadoop and Spark.
- Recognize the importance of advanced machine learning skills.
Define Key Roles and Responsibilities
- Data Scientists:
- Focus on extracting insights from complex data sets.
- Develop predictive models and machine learning algorithms.
- Examples:
- At Netflix, data scientists create recommendation algorithms to enhance user experience.
- Data Engineers:
- Design and maintain data pipelines and infrastructure.
- Ensure data accessibility, reliability, and cleanliness.
- Examples:
- Data engineers at Airbnb manage data flow from various sources to enable real-time analytics.
- Machine Learning Engineers:
- Develop and deploy machine learning models.
- Optimize models for performance and scalability.
- Examples:
- Machine learning engineers at Tesla develop autonomous driving algorithms.
- AI Specialists:
- Focus on specific AI technologies such as NLP, computer vision, or robotics.
- Implement AI solutions to enhance business operations.
- Examples:
- AI specialists at Google work on NLP advancements for tools like Google Assistant.
- Data Analysts:
- Interpret data to identify trends and insights.
- Create reports and dashboards for stakeholders.
- Examples:
- Data analysts at Facebook analyze user data to improve platform features.
Recruit and Retain Top Talent
- Develop a Recruitment Strategy:
- Define clear job descriptions and requirements.
- Use multiple channels to reach potential candidates.
- Examples:
- Post job openings on platforms like 9cv9, Indeed, and specialized AI job boards.
- Partner with universities and coding bootcamps to access emerging talent.
- Offer Competitive Compensation:
- Provide competitive salaries and benefits to attract top talent.
- Foster a Positive Work Environment:
- Create a culture that values innovation, collaboration, and continuous learning.
- Examples:
- Implement flexible working arrangements and opportunities for professional development.
- Encourage team-building activities and open communication.
- Provide Continuous Learning Opportunities:
- Invest in ongoing training and development programs.
- Examples:
- Offer access to online courses, certifications, and workshops.
- Encourage attendance at industry conferences and seminars.
Implement the Right Tools and Technologies
- Data Management Platforms:
- Choose robust data management solutions to handle large volumes of data.
- Examples:
- Implement databases like MySQL, PostgreSQL, and NoSQL databases.
- Use big data technologies like Hadoop and Spark for large-scale data processing.
- Machine Learning and AI Frameworks:
- Select appropriate machine learning frameworks and libraries.
- Examples:
- Use TensorFlow, PyTorch, and scikit-learn for developing machine learning models.
- Implement deep learning frameworks for advanced AI applications.
- Data Visualization Tools:
- Utilize data visualization tools to communicate insights effectively.
- Examples:
- Use Tableau, Power BI, and D3.js for creating interactive dashboards and reports.
Foster Collaboration and Communication
- Cross-Functional Teams:
- Promote collaboration between AI specialists, data scientists, engineers, and business analysts.
- Examples:
- Establish regular team meetings and collaborative projects to enhance teamwork.
- Agile Methodologies:
- Implement agile methodologies to manage AI and data projects efficiently.
- Examples:
- Use Scrum or Kanban frameworks to improve project management and delivery.
- Effective Communication Tools:
- Utilize communication tools to ensure seamless collaboration.
- Examples:
- Use Slack, Microsoft Teams, and project management tools like JIRA and Trello.
Monitor and Evaluate Performance
- Key Performance Indicators (KPIs):
- Define KPIs to measure the success of AI and data initiatives.
- Examples:
- Track metrics like model accuracy, data processing speed, and cost savings.
- Regular Reviews and Feedback:
- Conduct regular performance reviews and provide constructive feedback.
- Examples:
- Schedule quarterly reviews to assess progress and address challenges.
- Continuous Improvement:
- Encourage a culture of continuous improvement and innovation.
- Examples:
- Implement a feedback loop to learn from past projects and improve future initiatives.
Case Studies and Success Stories
- Healthcare:
- IBM Watson Health: Leveraging AI to analyze medical data, providing doctors with evidence-based treatment options.
- Example: A study found Watson for Oncology recommended treatment plans aligned with experts’ recommendations 85% of the time.
- Finance:
- JPMorgan Chase: Using AI to analyze legal documents and contracts, saving over 360,000 hours of work annually.
- Retail:
- Amazon: Utilizing AI for product recommendations, inventory management, and dynamic pricing.
- Example: Amazon’s recommendation engine accounts for 35% of the company’s total sales.
Summary
Building a strong AI and data team involves a strategic approach that starts with defining business objectives and assessing current capabilities.
By recruiting top talent, implementing the right tools and technologies, fostering collaboration, and continuously monitoring performance, businesses can create a high-performing AI and data team.
This team will be well-equipped to drive innovation, optimize operations, and achieve strategic goals in 2024 and beyond.
5. Tools and Technologies to Equip Your Team
Data Management and Storage Solutions
- Relational Databases:
- MySQL:
- Widely used open-source relational database management system.
- Suitable for structured data and transactional systems.
- Examples:
- Facebook: Uses MySQL to manage large volumes of user data.
- Statistics:
- PostgreSQL:
- Advanced open-source relational database with support for complex queries and data types.
- Known for its reliability and robust feature set.
- Examples:
- Instagram: Utilizes PostgreSQL to manage its large-scale data needs.
- MySQL:
- NoSQL Databases:
- MongoDB:
- Document-oriented database ideal for unstructured data.
- Supports horizontal scaling and high performance.
- Examples:
- eBay: Uses MongoDB for managing complex data sets in real-time.
- Statistics:
- Cassandra:
- Distributed NoSQL database designed for handling large amounts of data across multiple servers.
- Known for its fault tolerance and scalability.
- Examples:
- Netflix: Relies on Cassandra for streaming data and user activity logs.
- Statistics:
- MongoDB:
- Data Warehousing:
- Amazon Redshift:
- Fully managed data warehouse service.
- Scales to petabytes of data and integrates seamlessly with other AWS services.
- Examples:
- Yelp: Uses Amazon Redshift to analyze user reviews and engagement.
- Statistics:
- Google BigQuery:
- Serverless, highly scalable data warehouse.
- Enables fast SQL queries using the processing power of Google’s infrastructure.
- Examples:
- Spotify: Uses BigQuery for large-scale data analysis and reporting.
- Amazon Redshift:
Data Processing and Integration Tools
- ETL (Extract, Transform, Load) Tools:
- Apache NiFi:
- Open-source tool for automating data flow between systems.
- Supports data ingestion, transformation, and routing.
- Examples:
- Cloudera: Uses NiFi to handle real-time data processing tasks.
- Statistics:
- Talend:
- Data integration platform offering ETL, data quality, and governance capabilities.
- Known for its ease of use and comprehensive feature set.
- Examples:
- Domino’s: Uses Talend for integrating customer data and improving marketing strategies.
- Apache NiFi:
- Big Data Processing:
- Apache Hadoop:
- Framework for distributed storage and processing of large data sets.
- Comprises Hadoop Distributed File System (HDFS) and MapReduce programming model.
- Examples:
- LinkedIn: Uses Hadoop for member data analysis and recommendation systems.
- Apache Spark:
- Unified analytics engine for big data processing with built-in modules for SQL, streaming, machine learning, and graph processing.
- Known for its speed and ease of use.
- Examples:
- Uber: Uses Spark for real-time data analytics and decision-making.
- Statistics:
- Apache Hadoop:
Machine Learning and AI Frameworks
- TensorFlow:
- Open-source machine learning framework developed by Google.
- Widely used for building and deploying machine learning models.
- Examples:
- DeepMind: Uses TensorFlow for its AI research, including AlphaGo.
- Statistics:
- PyTorch:
- Open-source machine learning library developed by Facebook’s AI Research lab.
- Known for its flexibility and ease of use in research and production.
- Examples:
- Tesla: Uses PyTorch for developing autonomous driving technology.
- scikit-learn:
- Simple and efficient tools for data mining and data analysis built on NumPy, SciPy, and matplotlib.
- Suitable for both research and production.
- Examples:
- Spotify: Uses scikit-learn for its recommendation engine.
Data Visualization and Business Intelligence (BI) Tools
- Tableau:
- Interactive data visualization software.
- Helps create a wide range of visualizations to understand data better.
- Examples:
- PepsiCo: Uses Tableau to track sales performance and market trends.
- Statistics:
- Tableau has over 86,000 customer accounts and is a leader in the BI market.
- Power BI:
- Business analytics service by Microsoft.
- Provides interactive visualizations and business intelligence capabilities.
- Examples:
- Heineken: Uses Power BI to analyze and visualize marketing data.
- Statistics:
- D3.js:
- JavaScript library for producing dynamic, interactive data visualizations in web browsers.
- Known for its flexibility and power in creating custom visualizations.
- Examples:
- The New York Times: Uses D3.js for interactive graphics and data journalism.
Collaboration and Project Management Tools
- JIRA:
- Project management tool for agile teams.
- Helps track tasks, issues, and project progress.
- Examples:
- Atlassian: Uses JIRA to manage its internal development projects.
- Statistics:
- Trello:
- Visual collaboration tool for organizing tasks and projects.
- Uses boards, lists, and cards to manage project workflows.
- Examples:
- Google: Teams at Google use Trello for project management and collaboration.
- Statistics:
- Slack:
- Collaboration platform that connects teams through channels, direct messages, and integrations with other tools.
- Examples:
- IBM: Uses Slack to enhance team communication and collaboration.
- Statistics:
Cloud Platforms and Services
- Amazon Web Services (AWS):
- Comprehensive cloud computing platform offering a wide range of services including compute, storage, and AI.
- Examples:
- Netflix: Uses AWS for its entire infrastructure, including data storage, processing, and machine learning.
- Statistics:
- Google Cloud Platform (GCP):
- Cloud services platform offering computing, data storage, data analytics, and machine learning.
- Examples:
- Twitter: Uses GCP for data analytics and machine learning.
- Statistics:
- Microsoft Azure:
- Cloud computing service providing a variety of services including analytics, virtual computing, storage, and networking.
- Examples:
- Adobe: Uses Azure for its cloud-based services and analytics.
- Statistics:
Continuous Integration and Deployment (CI/CD) Tools
- Jenkins:
- Open-source automation server for continuous integration and delivery.
- Automates the build, test, and deployment process.
- Examples:
- Airbnb: Uses Jenkins for continuous integration and delivery of its services.
- Statistics:
- CircleCI:
- Continuous integration and delivery platform for automating development workflows.
- Supports a wide range of languages and environments.
- Examples:
- Instacart: Uses CircleCI for automated testing and deployment.
- Statistics:
Summary
Equipping your AI and data team with the right tools and technologies is crucial for maximizing their productivity and effectiveness.
By leveraging advanced data management solutions, machine learning frameworks, data visualization tools, collaboration platforms, cloud services, and CI/CD tools, businesses can ensure their AI and data initiatives are successful.
Investing in these tools not only streamlines processes but also enhances the team’s ability to deliver actionable insights, drive innovation, and achieve strategic objectives.
6. Best Practices for Managing an AI & Data Team
Managing an AI & data team requires a blend of technical knowledge, strategic vision, and effective leadership.
By implementing best practices, you can foster a productive environment that maximizes the potential of your AI and data initiatives.
Below are key strategies to help you manage your AI & data team successfully.
Establish Clear Objectives and Goals
- Define Clear Objectives:
- Set specific, measurable, achievable, relevant, and time-bound (SMART) goals.
- Align team objectives with overall business goals.
- Examples:
- Increase predictive model accuracy by 10% in the next quarter.
- Implement a data-driven marketing strategy to boost conversion rates by 15%.
- Communicate Goals Effectively:
- Ensure all team members understand the objectives and their roles in achieving them.
- Use regular meetings, emails, and project management tools to keep everyone informed.
Foster a Collaborative Environment
- Promote Cross-Functional Collaboration:
- Encourage collaboration between data scientists, engineers, business analysts, and other departments.
- Use cross-functional teams to tackle complex problems.
- Examples:
- At Spotify, data scientists and engineers collaborate with product managers to improve the recommendation system.
- Leverage Collaboration Tools:
- Use tools like Slack, Microsoft Teams, and Trello to facilitate communication and project management.
- Ensure all team members have access to the same information and resources.
- Statistics:
Invest in Continuous Learning and Development
- Provide Training and Development Opportunities:
- Offer access to online courses, workshops, and conferences.
- Encourage team members to pursue certifications in relevant fields.
- Examples:
- Google provides its employees with access to Udacity courses and encourages participation in AI conferences.
- Statistics:
- Encourage Knowledge Sharing:
- Foster a culture of continuous learning by encouraging team members to share their knowledge and expertise.
- Organize regular knowledge-sharing sessions and internal workshops.
- Examples:
- Airbnb hosts regular tech talks and hackathons to promote knowledge sharing among its data teams.
Implement Agile Methodologies
- Adopt Agile Practices:
- Use agile methodologies like Scrum or Kanban to manage projects.
- Break projects into smaller tasks and use iterative development cycles.
- Examples:
- IBM uses agile practices to manage its AI projects, ensuring flexibility and adaptability.
- Statistics:
- Conduct Regular Stand-Up Meetings:
- Hold daily or weekly stand-up meetings to discuss progress, challenges, and next steps.
- Ensure all team members are on the same page and address any roadblocks.
- Examples:
- At Atlassian, daily stand-up meetings help keep the team aligned and focused on their goals.
Monitor Performance and Provide Feedback
- Track Key Performance Indicators (KPIs):
- Define and monitor KPIs to measure the performance of AI and data initiatives.
- Examples:
- Model accuracy, data processing speed, project completion time, and cost savings.
- Statistics:
- Conduct Regular Performance Reviews:
- Provide constructive feedback through regular performance reviews.
- Use the feedback to help team members improve and develop their skills.
- Examples:
- Netflix conducts regular 360-degree feedback sessions to ensure continuous improvement.
Encourage Innovation and Experimentation
- Foster a Culture of Innovation:
- Encourage team members to experiment with new ideas and technologies.
- Provide resources and time for innovation projects.
- Examples:
- Amazon encourages its teams to innovate by allowing them to spend 20% of their time on experimental projects.
- Statistics:
- Companies that foster a culture of innovation see a 30% increase in enterprise value.
- Implement a Fail-Fast Approach:
- Encourage a fail-fast mentality where team members are not afraid to fail and learn from their mistakes.
- Promote a safe environment for experimentation.
- Examples:
- Google’s X lab embraces a fail-fast approach to drive innovation in its projects.
Ensure Data Security and Compliance
- Implement Robust Security Measures:
- Protect data with encryption, access controls, and regular security audits.
- Ensure compliance with relevant data protection regulations (e.g., GDPR, CCPA).
- Examples:
- Facebook invests heavily in data security measures to protect user data.
- Statistics:
- Educate Team Members on Data Security:
- Conduct regular training sessions on data security best practices.
- Ensure all team members understand the importance of data protection.
- Examples:
- Microsoft provides regular security training to its employees to prevent data breaches.
Leverage the Right Tools and Technologies
- Use Advanced Analytics Tools:
- Equip your team with tools like Tableau, Power BI, and D3.js for data visualization and analysis.
- Examples:
- Uber uses Tableau to visualize and analyze large datasets for better decision-making.
- Adopt Scalable Data Infrastructure:
- Use cloud platforms like AWS, Google Cloud, and Microsoft Azure for scalable data storage and processing.
- Examples:
- Netflix relies on AWS for its scalable and reliable data infrastructure.
- Invest in Machine Learning Frameworks:
- Use frameworks like TensorFlow, PyTorch, and scikit-learn for developing and deploying machine learning models.
- Examples:
- Airbnb uses PyTorch to build and deploy machine learning models for personalized user experiences.
Promote Diversity and Inclusion
- Foster a Diverse Team:
- Encourage diversity in hiring to bring different perspectives and ideas.
- Create an inclusive environment where everyone feels valued and respected.
- Examples:
- IBM has initiatives to promote diversity and inclusion within its AI teams.
- Statistics:
- Implement Inclusive Policies:
- Ensure your workplace policies promote inclusivity and equality.
- Provide support for underrepresented groups in the tech industry.
- Examples:
- Google has programs to support women and minorities in tech through mentorship and professional development.
Summary
Managing an AI & data team effectively requires a strategic approach that encompasses clear goal setting, fostering collaboration, continuous learning, agile methodologies, performance monitoring, innovation, data security, leveraging the right tools, and promoting diversity.
By following these best practices, you can create a high-performing AI & data team capable of driving significant business value and staying ahead in the competitive landscape of 2024 and beyond.
7. Challenges and How to Overcome Them
Managing an AI & data team comes with its unique set of challenges.
Overcoming these obstacles requires strategic planning, effective leadership, and a deep understanding of the technical and organizational landscape.
Here, we explore common challenges and provide actionable solutions to help you navigate them successfully.
Talent Acquisition and Retention
- Challenge: Shortage of Skilled Professionals
- Explanation:
- The demand for AI and data professionals far exceeds the supply, making it difficult to find and hire qualified candidates.
- Statistics:
- According to LinkedIn, the demand for AI specialists grew by 74% annually over the past four years.
- Solutions:
- Invest in Training Programs:
- Develop internal training programs to upskill existing employees.
- Partner with educational institutions to create talent pipelines.
- Examples:
- Amazon invests in upskilling programs like AWS Training and Certification to address talent shortages.
- Leverage Remote Work:
- Expand the talent pool by offering remote work options.
- Use collaborative tools to manage remote teams effectively.
- Statistics:
- Invest in Training Programs:
- Explanation:
- Challenge: High Turnover Rates
- Explanation:
- High turnover rates in the tech industry can disrupt projects and lead to loss of valuable knowledge.
- Statistics:
- The tech industry experiences an average turnover rate of 13.2%, higher than many other sectors.
- Solutions:
- Create a Positive Work Environment:
- Foster a supportive and inclusive culture.
- Recognize and reward employee contributions.
- Examples:
- Google’s employee-friendly policies and perks have helped it maintain low turnover rates.
- Offer Competitive Compensation:
- Provide competitive salaries and benefits to attract and retain top talent.
- Examples:
- Facebook offers lucrative compensation packages, including stock options and bonuses, to retain its AI experts.
- Create a Positive Work Environment:
- Explanation:
Managing Interdisciplinary Teams
- Challenge: Communication Barriers
- Explanation:
- Differences in technical jargon and expertise can lead to misunderstandings and inefficiencies.
- Statistics:
- Solutions:
- Foster Open Communication:
- Encourage regular meetings and open channels for discussion.
- Use clear and concise language to bridge the gap between technical and non-technical team members.
- Examples:
- Atlassian uses Confluence to facilitate communication and documentation across interdisciplinary teams.
- Provide Cross-Training:
- Offer training sessions to help team members understand each other’s roles and responsibilities.
- Examples:
- Spotify conducts cross-functional workshops to enhance collaboration between data scientists and engineers.
- Foster Open Communication:
- Explanation:
- Challenge: Aligning Goals and Priorities
- Explanation:
- Different departments may have conflicting goals, leading to misalignment and inefficiency.
- Statistics:
- Only 29% of employees feel that their goals are aligned with the company’s strategic objectives.
- Solutions:
- Set Unified Objectives:
- Define common goals that align with the organization’s overall strategy.
- Ensure all team members understand their roles in achieving these goals.
- Examples:
- At IBM, regular strategy sessions ensure that all teams are aligned with the company’s AI vision.
- Use OKRs (Objectives and Key Results):
- Implement OKRs to align team objectives with business outcomes.
- Regularly review and adjust OKRs to stay on track.
- Examples:
- Google’s use of OKRs has been instrumental in aligning its diverse teams towards common objectives.
- Set Unified Objectives:
- Explanation:
Ensuring Data Quality and Security
- Challenge: Maintaining Data Quality
- Explanation:
- Poor data quality can lead to inaccurate models and flawed insights.
- Statistics:
- Solutions:
- Implement Data Governance:
- Establish policies and procedures for data management.
- Assign data stewards to oversee data quality.
- Examples:
- Microsoft has a robust data governance framework to ensure high data quality across its AI projects.
- Use Data Quality Tools:
- Deploy tools for data cleaning, validation, and monitoring.
- Examples:
- Companies like Talend and Informatica offer comprehensive data quality solutions.
- Implement Data Governance:
- Explanation:
- Challenge: Ensuring Data Security
- Explanation:
- Protecting sensitive data from breaches and unauthorized access is critical.
- Statistics:
- Solutions:
- Adopt Robust Security Measures:
- Use encryption, access controls, and regular security audits.
- Ensure compliance with regulations like GDPR and CCPA.
- Examples:
- Facebook employs advanced encryption and multi-factor authentication to secure user data.
- Educate Employees on Security Practices:
- Conduct regular training sessions on data security best practices.
- Examples:
- IBM regularly trains its employees on the latest security protocols to prevent data breaches.
- Adopt Robust Security Measures:
- Explanation:
Managing Project Timelines and Expectations
- Challenge: Scope Creep
- Explanation:
- Uncontrolled changes or continuous growth in project scope can lead to delays and cost overruns.
- Statistics:
- Solutions:
- Define Clear Project Scope:
- Establish detailed project plans with clearly defined deliverables.
- Use change management processes to handle scope adjustments.
- Examples:
- Atlassian uses Jira to manage project scope and track changes effectively.
- Set Realistic Deadlines:
- Ensure deadlines are achievable and account for potential obstacles.
- Regularly review progress and adjust timelines as necessary.
- Examples:
- Google’s AI projects often use phased milestones to manage timelines effectively.
- Define Clear Project Scope:
- Explanation:
- Challenge: Managing Stakeholder Expectations
- Explanation:
- Misaligned expectations between the AI team and stakeholders can lead to dissatisfaction and project failure.
- Statistics:
- Solutions:
- Engage Stakeholders Early:
- Involve stakeholders in the planning and decision-making process.
- Clearly communicate project goals, timelines, and potential risks.
- Examples:
- Amazon Web Services (AWS) involves stakeholders from the beginning to ensure alignment on AI initiatives.
- Provide Regular Updates:
- Use progress reports and meetings to keep stakeholders informed.
- Address concerns and adjust plans based on feedback.
- Examples:
- Slack uses regular update meetings to manage stakeholder expectations and ensure transparency.
- Engage Stakeholders Early:
- Explanation:
Keeping Up with Rapid Technological Advancements
- Challenge: Staying Current with AI Trends
- Explanation:
- The rapid pace of AI advancements can make it challenging to stay updated with the latest tools and technologies.
- Statistics:
- Solutions:
- Encourage Continuous Learning:
- Provide resources for ongoing education and professional development.
- Encourage participation in AI conferences, webinars, and online courses.
- Examples:
- Google encourages its AI team members to attend industry conferences and engage in continuous learning.
- Leverage AI Communities:
- Participate in AI forums, online communities, and professional networks.
- Share knowledge and learn from industry peers.
- Examples:
- OpenAI’s active participation in AI research communities helps it stay at the forefront of technological advancements.
- Encourage Continuous Learning:
- Explanation:
- Challenge: Integrating New Technologies
- Explanation:
- Integrating new AI technologies with existing systems can be complex and resource-intensive.
- Statistics:
- Solutions:
- Adopt Modular Architectures:
- Use modular and scalable architectures to facilitate integration.
- Examples:
- Netflix’s microservices architecture allows for seamless integration of new AI technologies.
- Pilot New Technologies:
- Conduct pilot projects to test new technologies before full-scale implementation.
- Use feedback to refine integration strategies.
- Examples:
- Uber conducts pilot programs to evaluate new AI tools and technologies.
- Adopt Modular Architectures:
- Explanation:
Summary
Managing an AI & data team involves navigating a variety of challenges, from talent acquisition to technological advancements.
By implementing best practices such as investing in training, fostering collaboration, ensuring data quality and security, managing project timelines, and staying current with AI trends, organizations can overcome these obstacles and harness the full potential of their AI initiatives.
With strategic planning and effective leadership, your AI & data team can drive innovation, achieve business goals, and maintain a competitive edge in the rapidly evolving tech landscape.
8. Future Trends to Watch in AI & Data Teams
As the field of artificial intelligence (AI) and data science continues to evolve at a rapid pace, staying ahead of emerging trends is crucial for businesses aiming to maintain a competitive edge.
The following sections explore key trends that are expected to shape AI & data teams in the coming years, supported by relevant examples, data, and statistics.
Increased Adoption of Automated Machine Learning (AutoML)
- Trend: Rise of AutoML Tools
- Explanation:
- Automated Machine Learning (AutoML) tools simplify the process of building, deploying, and managing machine learning models.
- These tools enable non-experts to create high-quality models, democratizing access to AI technologies.
- Examples:
- Google Cloud AutoML allows developers with limited machine learning expertise to train high-quality models tailored to their needs.
- DataRobot offers an enterprise AI platform that automates the end-to-end process of building, deploying, and maintaining AI models.
- Explanation:
- Implications for AI & Data Teams:
- Efficiency Gains:
- AutoML reduces the time and effort required to develop machine learning models, allowing teams to focus on more complex tasks.
- It can lead to faster deployment of AI solutions, accelerating time-to-market for new products and services.
- Broader Involvement:
- With AutoML, more team members, including those without deep AI expertise, can participate in the model-building process.
- Efficiency Gains:
Expansion of Explainable AI (XAI)
- Trend: Focus on Explainability and Transparency
- Explanation:
- Explainable AI (XAI) aims to make AI models more transparent and interpretable, addressing the “black box” nature of many algorithms.
- XAI is crucial for gaining trust from stakeholders and ensuring regulatory compliance.
- Examples:
- IBM’s AI Explainability 360 toolkit provides a suite of algorithms to help users understand and interpret AI models.
- DARPA’s XAI program focuses on creating AI systems that can explain their decisions in a way that humans can understand.
- Explanation:
- Implications for AI & Data Teams:
- Enhanced Trust and Adoption:
- Explainable models can increase trust among users and stakeholders, facilitating broader adoption of AI solutions.
- Regulatory Compliance:
- Teams must ensure their models comply with emerging regulations that require transparency and accountability in AI systems.
- Enhanced Trust and Adoption:
Growth of Edge AI
- Trend: Deployment of AI at the Edge
- Explanation:
- Edge AI involves running AI algorithms directly on devices such as smartphones, IoT devices, and sensors, rather than relying on cloud-based systems.
- This trend is driven by the need for real-time processing, reduced latency, and enhanced data privacy.
- Examples:
- Apple’s A14 Bionic chip includes a neural engine that supports on-device machine learning for applications like image recognition and natural language processing.
- NVIDIA’s Jetson platform provides edge AI capabilities for robotics, smart cities, and autonomous machines.
- Explanation:
- Implications for AI & Data Teams:
- Real-Time Analytics:
- Teams can develop applications that require real-time data processing and immediate insights, such as autonomous vehicles and industrial automation.
- Data Privacy and Security:
- Edge AI enhances data privacy by processing data locally, reducing the need to transmit sensitive information to the cloud.
- Real-Time Analytics:
Integration of AI with Internet of Things (IoT)
- Trend: AI-Driven IoT Solutions
- Explanation:
- The integration of AI with IoT (AIoT) enables smart devices to make autonomous decisions based on real-time data analysis.
- This synergy enhances the capabilities of IoT systems, making them more intelligent and responsive.
- Examples:
- Siemens uses AIoT to optimize industrial operations, leveraging AI to analyze data from IoT sensors in real-time.
- Smart home devices like Amazon Echo and Google Nest use AI to learn user preferences and automate tasks.
- Explanation:
- Implications for AI & Data Teams:
- Enhanced Decision-Making:
- AIoT systems can provide actionable insights and automated responses, improving decision-making processes in various industries.
- Interdisciplinary Collaboration:
- Building AIoT solutions requires collaboration between AI experts, IoT specialists, and domain-specific engineers.
- Enhanced Decision-Making:
Emphasis on Ethical AI
- Trend: Focus on Ethics and Fairness in AI
- Explanation:
- Ethical AI involves ensuring that AI systems are developed and used in a manner that is fair, unbiased, and respects user privacy.
- Addressing ethical concerns is crucial for gaining public trust and avoiding potential legal issues.
- Examples:
- Microsoft’s AI Ethics Committee oversees the development and deployment of AI technologies to ensure they align with ethical standards.
- Google’s AI Principles guide the company’s AI research and development efforts, emphasizing fairness, accountability, and privacy.
- Explanation:
- Implications for AI & Data Teams:
- Bias Mitigation:
- Teams must implement strategies to identify and mitigate biases in AI models, ensuring fair outcomes for all users.
- Transparency and Accountability:
- Developing transparent AI systems and maintaining accountability throughout the AI lifecycle is essential.
- Bias Mitigation:
Adoption of Quantum Computing
- Trend: Quantum Computing in AI
- Explanation:
- Quantum computing promises to revolutionize AI by providing unprecedented computational power to solve complex problems.
- It can significantly enhance machine learning algorithms, optimization problems, and data analysis.
- Examples:
- IBM’s Quantum Experience platform allows researchers and developers to explore quantum computing applications in AI.
- Google’s Quantum AI lab focuses on advancing quantum computing technologies for AI research.
- Explanation:
- Implications for AI & Data Teams:
- Advanced Problem-Solving:
- Quantum computing can tackle problems that are currently intractable for classical computers, opening new avenues for AI applications.
- Skill Development:
- Teams will need to develop expertise in quantum computing and its integration with AI systems.
- Advanced Problem-Solving:
Rise of Federated Learning
- Trend: Distributed Machine Learning
- Explanation:
- Federated learning enables the training of machine learning models across decentralized devices or servers without sharing raw data.
- This approach enhances data privacy and security while leveraging the computational power of distributed systems.
- Statistics:
- Federated learning is projected to grow significantly, with applications in healthcare, finance, and IoT.
- Examples:
- Google’s Federated Learning system trains models on user devices, such as smartphones, to improve services like Gboard without accessing personal data.
- Intel and the University of Pennsylvania have collaborated on a federated learning project to improve brain tumor detection.
- Explanation:
- Implications for AI & Data Teams:
- Enhanced Privacy:
- Federated learning ensures data privacy by keeping raw data on local devices, aligning with privacy regulations.
- Collaboration Opportunities:
- Teams can collaborate on model training across different organizations and geographies without sharing sensitive data.
- Enhanced Privacy:
Enhanced Human-AI Collaboration
- Trend: Human-Centered AI
- Explanation:
- The focus on human-centered AI emphasizes the collaboration between humans and AI systems to augment human capabilities.
- This approach aims to make AI more intuitive, accessible, and effective in supporting human decision-making.
- Statistics:
- Examples:
- IBM’s Watson Assistant is designed to augment customer service agents by providing AI-driven insights and support.
- Microsoft’s AI-powered tools in Office 365 help users with tasks such as writing, data analysis, and scheduling.
- Explanation:
- Implications for AI & Data Teams:
- User Experience:
- Teams must focus on developing AI systems that are user-friendly and enhance the user experience.
- Training and Support:
- Providing training and support to users to effectively collaborate with AI systems is essential.
- User Experience:
Adoption of AI Governance Frameworks
- Trend: Structured AI Governance
- Explanation:
- AI governance frameworks provide guidelines for the ethical development, deployment, and use of AI systems.
- These frameworks address issues such as accountability, transparency, and compliance.
- Examples:
- The European Union’s AI Act proposes a comprehensive regulatory framework to ensure trustworthy AI.
- The IEEE Global Initiative on Ethics of Autonomous and Intelligent Systems offers guidelines for the ethical development of AI.
- Explanation:
- Implications for AI & Data Teams:
- Regulatory Compliance:
- Adopting AI governance frameworks ensures compliance with emerging regulations and standards.
- Risk Management:
- Teams can better manage risks associated with AI development and deployment by following established guidelines.
- Regulatory Compliance:
Summary
The future of AI & data teams is shaped by a dynamic landscape of emerging trends and technologies.
By staying informed about these trends, such as the rise of AutoML, the focus on explainable AI, the growth of edge AI, and the integration of AI with IoT, businesses can strategically position themselves for success.
Additionally, addressing ethical considerations, exploring quantum computing, adopting federated learning, enhancing human-AI collaboration, and implementing AI governance frameworks are crucial for navigating the evolving AI ecosystem.
As these trends continue to unfold, AI & data teams must remain agile, continuously adapt, and embrace innovation to drive meaningful outcomes and maintain a competitive edge in the digital age.
Conclusion
In the rapidly evolving digital landscape, building a robust AI and data team is no longer just an option but a strategic necessity.
As businesses strive to leverage the transformative power of artificial intelligence and data analytics, understanding the intricacies involved in assembling and managing a high-performing team becomes paramount.
This conclusion summarizes key points and offers actionable insights to help your business succeed in the AI-driven era of 2024.
The Imperative of AI & Data Teams
- Transformational Impact:
- AI and data analytics are reshaping industries, driving innovation, enhancing operational efficiency, and unlocking new revenue streams.
- Statistics: Businesses leveraging big data see a profit increase of 8-10% and a 10% reduction in overall costs .
- Competitive Advantage:
- Companies that successfully integrate AI and data analytics into their operations are more likely to outperform their peers.
- Statistics: According to McKinsey, AI adoption can increase a company’s cash flow by 20% .
Steps to Building a Strong AI & Data Team
- Define Clear Objectives:
- Establish well-defined goals that align with your business strategy and identify specific AI applications that can deliver measurable value.
- Example: A retail company might focus on AI-driven customer personalization and inventory optimization.
- Recruit Top Talent:
- Attract and retain skilled professionals with expertise in machine learning, data engineering, data science, and related fields.
- Statistics: The demand for AI specialists has grown by 74% annually over the past four years .
- Foster Continuous Learning:
- Encourage ongoing education and professional development to keep the team updated with the latest AI technologies and methodologies.
- Example: Google encourages its AI engineers to participate in industry conferences and advanced training programs.
Key Roles in an AI & Data Team
- Data Scientists:
- Focus on extracting insights from data and developing predictive models.
- Statistics: Data scientist roles have increased by 37% in recent years .
- Machine Learning Engineers:
- Design and implement machine learning algorithms and ensure they are scalable and efficient.
- Example: Netflix employs machine learning engineers to develop recommendation algorithms that enhance user experience.
- Data Engineers:
- Build and maintain data infrastructure, ensuring reliable data flow and storage.
- Example: Airbnb uses data engineers to manage vast amounts of data generated by users and listings.
Skills and Qualifications to Look For
- Technical Proficiency:
- Proficiency in programming languages such as Python, R, and Java.
- Understanding of machine learning frameworks like TensorFlow and PyTorch.
- Analytical Thinking:
- Strong problem-solving skills and the ability to analyze complex data sets to derive actionable insights.
- Domain Expertise:
- Knowledge of industry-specific challenges and opportunities that AI can address.
- Example: In healthcare, understanding medical terminologies and patient data management is crucial.
Tools and Technologies to Equip Your Team
- Data Management Platforms:
- Tools like Apache Hadoop and Google BigQuery for efficient data storage and processing.
- AI Development Platforms:
- Platforms such as Microsoft Azure AI and Amazon SageMaker for building, training, and deploying machine learning models.
- Collaboration Tools:
- Tools like Slack and GitHub to facilitate seamless communication and project management within the team.
Best Practices for Managing an AI & Data Team
- Agile Methodologies:
- Implement agile practices to enhance flexibility and responsiveness to changing project requirements.
- Example: Spotify uses agile frameworks to streamline its AI and data projects.
- Cross-Functional Collaboration:
- Foster collaboration between AI & data teams and other departments to ensure alignment with business objectives.
- Example: IBM encourages cross-functional teams to collaborate on AI projects, blending expertise from various domains.
- Performance Metrics:
- Establish key performance indicators (KPIs) to measure the success and impact of AI initiatives.
- Example: KPIs might include model accuracy, time-to-market, and ROI on AI investments.
Future Trends to Watch
- AutoML and Democratization of AI:
- Increased adoption of AutoML tools making AI accessible to non-experts.
- Statistics: The AutoML market is expected to reach $14.5 billion by 2024, growing at a CAGR of 44.5% .
- Explainable AI (XAI):
- Focus on transparency and interpretability of AI models to build trust and meet regulatory requirements.
- Statistics: 65% of executives believe that improving AI explainability is critical to gaining user trust .
- Edge AI:
- Deployment of AI at the edge to enable real-time processing and enhanced data privacy.
- Statistics: The edge AI market is projected to grow from $1.15 billion in 2020 to $12.2 billion by 2026 .
Overcoming Challenges
- Talent Acquisition:
- Address the shortage of skilled AI professionals through targeted recruitment and training programs.
- Example: Amazon’s AWS Training and Certification program helps develop AI talent.
- Data Quality and Security:
- Implement robust data governance frameworks to ensure data integrity and compliance with regulations.
- Statistics: Businesses lose an average of $15 million annually due to poor data quality .
- Managing Project Timelines:
- Use project management tools and methodologies to keep projects on track and within budget.
- Example: Jira by Atlassian is widely used to manage AI and data projects efficiently.
Conclusion: The Path Forward
Building a strong AI & data team in 2024 requires a strategic approach, focusing on acquiring the right talent, fostering a culture of continuous learning, and equipping the team with the best tools and technologies.
By staying abreast of emerging trends and implementing best practices, businesses can harness the full potential of AI and data analytics, driving innovation and maintaining a competitive edge.
As AI continues to transform industries, the ability to build and manage a proficient AI & data team will be a key differentiator for forward-thinking organizations.
If your company needs HR, hiring, or corporate services, you can use 9cv9 hiring and recruitment services. Book a consultation slot here, or send over an email to [email protected].
If you find this article useful, why not share it with your hiring manager and C-level suite friends and also leave a nice comment below?
We, at the 9cv9 Research Team, strive to bring the latest and most meaningful data, guides, and statistics to your doorstep.
To get access to top-quality guides, click over to 9cv9 Blog.
People Also Ask
What are the key roles in an AI and data team?
Key roles include data scientists, machine learning engineers, data engineers, data analysts, and AI ethicists.
What skills are essential for AI and data team members?
Essential skills include proficiency in programming languages (Python, R), machine learning frameworks (TensorFlow, PyTorch), data analysis, and domain-specific knowledge.
How do you recruit top AI and data talent?
Recruit through specialized job boards, AI competitions, university partnerships, and offering competitive salaries and growth opportunities.
What is the importance of continuous learning for AI teams?
Continuous learning ensures team members stay updated with the latest AI technologies and methodologies, enhancing innovation and effectiveness.
How can cross-functional collaboration benefit an AI team?
It ensures alignment with business objectives, promotes diverse perspectives, and fosters innovative solutions by integrating expertise from various domains.
What tools and technologies are essential for an AI and data team?
Essential tools include data management platforms (Hadoop, BigQuery), AI development platforms (Azure AI, SageMaker), and collaboration tools (Slack, GitHub).
How does AI improve operational efficiency?
AI automates repetitive tasks, predicts maintenance needs, optimizes supply chains, and enhances decision-making processes.
What are the best practices for managing an AI team?
Implement agile methodologies, encourage continuous learning, promote cross-functional collaboration, and set clear performance metrics.
Why is AI important for customer experience?
AI personalizes interactions, provides tailored recommendations, and offers efficient customer support, enhancing satisfaction and loyalty.
How can AI and data analytics drive business innovation?
They accelerate product development, uncover new business models, and optimize operations, leading to increased competitiveness and growth.
What challenges might you face when building an AI team?
Challenges include talent acquisition, data quality and security issues, and managing project timelines and expectations.
How can businesses overcome AI team building challenges?
Address challenges by investing in recruitment, implementing robust data governance frameworks, and using effective project management tools.
What are the future trends in AI and data teams?
Trends include AutoML, explainable AI, edge AI, AI integration with IoT, and the growing importance of AI ethics and governance.
Why is data quality important for AI projects?
High-quality data ensures accurate AI models, reliable insights, and effective decision-making, reducing risks and enhancing outcomes.
How does AI support data-driven decision-making?
AI analyzes large data sets quickly, providing actionable insights, real-time analytics, and predictive capabilities to guide strategic decisions.
What are the benefits of AI in supply chain management?
AI predicts demand, manages inventory, optimizes logistics, and reduces costs, leading to a more efficient and responsive supply chain.
How can AI enhance cybersecurity?
AI detects and responds to threats in real-time, adapts to new threats, and enhances overall security measures, protecting sensitive data and systems.
What is the role of machine learning engineers in an AI team?
They design and implement machine learning algorithms, ensuring models are scalable, efficient, and aligned with business needs.
How does AI enable personalized marketing?
AI analyzes consumer behavior and preferences, delivering targeted campaigns and personalized experiences that increase engagement and conversions.
Why is explainable AI important?
Explainable AI builds trust, ensures compliance with regulations, and helps stakeholders understand AI decisions and their implications.
What is the impact of AI on healthcare?
AI improves precision medicine, early disease detection, and operational efficiency, enhancing patient outcomes and reducing costs.
How can AI help in financial services?
AI enables automated trading, enhances fraud detection, and provides 24/7 customer support, improving service levels and operational efficiency.
What is the significance of AI governance frameworks?
AI governance ensures ethical AI deployment, compliance with regulations, and mitigates risks associated with AI development and usage.
How does AI contribute to environmental sustainability?
AI optimizes energy consumption, improves climate modeling, and supports sustainable practices, reducing carbon footprints and promoting eco-friendly initiatives.
What are the advantages of AI in predictive maintenance?
AI predicts equipment failures, schedules maintenance proactively, and reduces downtime, leading to cost savings and improved operational efficiency.
How do agile methodologies benefit AI teams?
Agile methodologies enhance flexibility, responsiveness to changes, and streamline project management, improving overall team performance.
What is the role of data engineers in an AI team?
Data engineers build and maintain data infrastructure, ensuring reliable data flow and storage for analysis and AI model development.
How does AI facilitate real-time insights?
AI processes data streams instantly, providing real-time analytics and enabling businesses to respond quickly to market changes and customer needs.
What is federated learning and its importance?
Federated learning allows AI models to learn from data across multiple locations without centralizing data, enhancing privacy and collaboration.
Why is human-AI collaboration crucial?
Human-AI collaboration combines human creativity and AI’s analytical power, leading to innovative solutions and more effective decision-making.