Key Takeaways
- Harness the Power of Data: Predictive analytics in HR empowers organizations to leverage historical data and statistical algorithms for informed decision-making in talent management.
- Strategic Implementation: Understand the core concepts and methodologies of predictive analytics to effectively implement HR strategies, from recruitment optimization to performance management.
- Future-proof Your HR Practices: Embrace emerging trends and technologies in predictive analytics to stay ahead in the ever-evolving landscape of HR management and drive organizational success.
In today’s dynamic and competitive business landscape, Human Resource (HR) professionals are increasingly turning to data-driven insights to navigate complex workforce challenges and drive organizational success.
At the forefront of this analytical revolution lies predictive analytics, a powerful tool reshaping the way HR departments operate.
But what exactly is predictive analytics in HR, and how can organizations harness its potential to optimize talent management strategies?
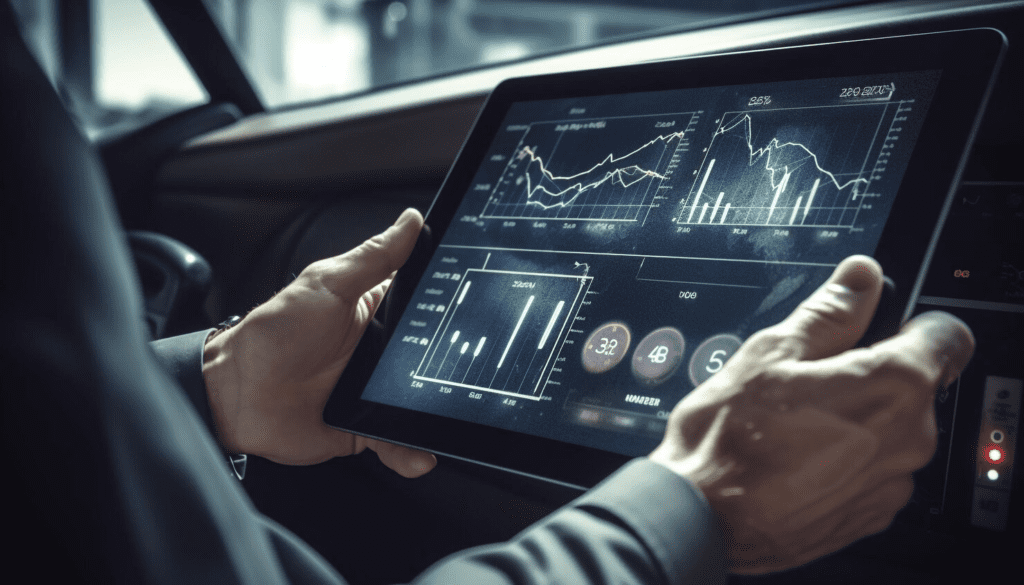
In this comprehensive guide, we delve deep into the realm of predictive analytics within the HR domain, demystifying its concepts, exploring its applications, and providing actionable insights on how to leverage it effectively.
Whether you’re a seasoned HR professional looking to enhance your analytical capabilities or a business leader seeking innovative solutions to talent-related issues, this resource serves as your roadmap to unlocking the transformative power of predictive analytics.
So, what precisely is predictive analytics in HR?
At its core, predictive analytics involves leveraging historical data, statistical algorithms, and machine learning techniques to forecast future outcomes and trends related to the workforce.
Unlike traditional descriptive analytics, which focuses on summarizing past events, predictive analytics enables HR teams to anticipate future scenarios, identify potential risks, and proactively devise strategies to mitigate them.
The applications of predictive analytics in HR are far-reaching and impactful.
From optimizing recruitment processes and enhancing employee retention to predicting performance outcomes and driving strategic workforce planning, the possibilities are virtually endless.
By harnessing the predictive power of data, organizations can gain valuable insights into their workforce dynamics, enabling them to make informed decisions that drive efficiency, productivity, and overall business performance.
But embarking on a journey into predictive analytics in HR requires more than just access to data and analytics tools.
It demands a strategic approach, a solid understanding of analytical techniques, and a commitment to fostering a data-driven culture within the organization.
Throughout this guide, we’ll explore the essential steps involved in implementing predictive analytics projects in HR, from data collection and model development to interpretation of results and iterative refinement.
Moreover, we’ll address common challenges and pitfalls encountered along the way, providing actionable strategies for overcoming them and maximizing the success of your predictive analytics initiatives.
From ensuring data quality and addressing privacy concerns to garnering stakeholder buy-in and navigating technological constraints, we’ll equip you with the knowledge and tools needed to navigate the complexities of predictive analytics in HR with confidence.
In addition to practical insights and implementation guidance, we’ll also delve into the ethical considerations surrounding predictive analytics in HR.
As organizations increasingly rely on data to drive decision-making, it’s essential to uphold ethical standards and ensure fairness, transparency, and accountability in the use of predictive models.
We’ll explore best practices for mitigating bias, protecting employee privacy, and promoting responsible data governance in HR analytics.
Furthermore, we’ll provide a glimpse into the future of predictive analytics in HR, examining emerging trends, technologies, and opportunities shaping the landscape of workforce analytics.
From advancements in artificial intelligence (AI) and machine learning to the growing focus on personalization and employee experience, we’ll explore how organizations can stay ahead of the curve and leverage predictive analytics to gain a competitive edge in talent management.
Intrigued to learn more?
Join us on this illuminating journey into the world of predictive analytics in HR, where data-driven insights pave the way for smarter, more strategic human resource management.
Whether you’re a data enthusiast eager to explore the latest trends or an HR professional seeking actionable strategies for success, this guide is your ultimate companion to unlocking the full potential of predictive analytics in HR.
Let’s embark on this transformative journey together.
Before we venture further into this article, we like to share who we are and what we do.
About 9cv9
9cv9 is a business tech startup based in Singapore and Asia with a strong presence all over the world.
With over eight years of startup and business experience, and being highly involved in connecting with thousands of companies and startups, the 9cv9 team has listed some important learning points in this overview of What is Predictive Analytics in HR and How to Use It.
If your company needs recruitment and headhunting services to hire top employees, you can use 9cv9 headhunting and recruitment services to hire top talents and candidates. Find out more here, or send over an email to [email protected].
Or just post 1 free job posting here at 9cv9 Hiring Portal in under 10 minutes.
What is Predictive Analytics in HR and How to Use It
- Understanding Predictive Analytics in HR
- Getting Started with Predictive Analytics in HR
- Implementing Predictive Analytics Projects in HR
- Overcoming Challenges and Pitfalls
- Ethical Considerations in Predictive Analytics
- Future Trends and Opportunities
1. Understanding Predictive Analytics in HR
In today’s data-driven world, Human Resource (HR) departments are increasingly turning to predictive analytics to gain deeper insights into their workforce and drive strategic decision-making.
Predictive analytics in HR involves leveraging historical data, statistical algorithms, and machine learning techniques to forecast future outcomes and trends related to talent management.
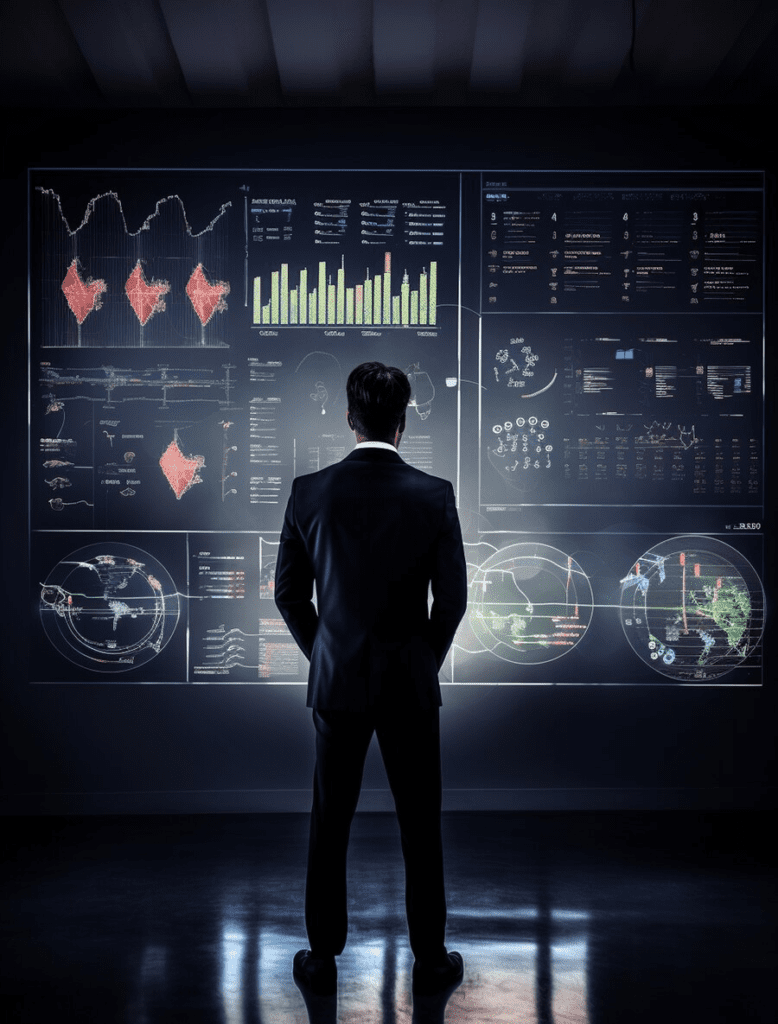
Let’s delve deeper into this transformative approach and uncover its key components and methodologies:
Core Concepts and Methodologies
- Predictive Modeling: At the heart of predictive analytics in HR lies predictive modeling, which involves using historical data to build mathematical models that predict future events or behaviors.
- Statistical Techniques and Algorithms: Various statistical techniques and algorithms are employed in predictive analytics, including regression analysis, decision trees, neural networks, and ensemble methods such as random forests and gradient boosting.
- Differentiation from Descriptive Analytics: Unlike descriptive analytics, which focuses on summarizing past data to describe what has happened, predictive analytics aims to anticipate future outcomes based on historical patterns and trends.
Key Components of Predictive Analytics
- Data Collection and Preprocessing: HR departments gather data from various sources, including HRIS (Human Resource Information Systems), performance reviews, recruitment platforms, and employee surveys. Data preprocessing involves cleaning, transforming, and preparing the data for analysis.
- Model Development and Validation: HR professionals use statistical modeling techniques to develop predictive models based on historical data. These models are then validated using techniques such as cross-validation to assess their accuracy and reliability.
Example: Recruitment Predictive Analytics
Imagine a company looking to optimize its recruitment process.
By analyzing historical data on successful hires, including candidate qualifications, previous job experience, and performance evaluations, HR can develop predictive models to identify the characteristics and traits that are most predictive of success in specific roles.
This enables the company to prioritize candidates who are likely to excel in the position, leading to more effective hiring decisions and reduced turnover rates.
Example: Employee Turnover Prediction
Another example of predictive analytics in HR is employee turnover prediction.
By analyzing factors such as job satisfaction, engagement levels, and career advancement opportunities, HR can build models to predict which employees are at risk of leaving the organization.
Armed with this insight, HR can proactively implement retention strategies, such as career development programs or improved work-life balance initiatives, to retain top talent and reduce turnover rates.
Understanding predictive analytics in HR is essential for HR professionals looking to leverage data-driven insights to optimize talent management strategies and drive organizational success.
By grasping the core concepts, methodologies, and key components of predictive analytics, HR departments can unlock the power of data to make informed decisions, enhance employee engagement, and gain a competitive edge in today’s rapidly evolving business landscape.
2. Getting Started with Predictive Analytics in HR
Embarking on a journey into predictive analytics in HR can be daunting, but with the right approach and tools, organizations can unlock valuable insights into their workforce dynamics.
Let’s explore the essential steps to getting started with predictive analytics in HR:
Building the Foundation: Data Collection and Management
- Identifying Relevant HR Data Sources: HR departments should identify and collect data from various sources, including HRIS (Human Resource Information Systems), recruitment platforms, performance management systems, employee surveys, and external sources like social media and industry reports.
- Ensuring Data Quality and Integrity: It’s crucial to ensure that the data collected is accurate, complete, and consistent. This involves cleaning, deduplicating, and validating the data to remove errors and inconsistencies.
- Data Privacy and Compliance Considerations: Organizations must adhere to data privacy regulations such as GDPR and CCPA and implement appropriate measures to protect employee data and ensure compliance.
Selecting the Right Tools and Technologies
- Overview of HR Analytics Platforms and Software: There are numerous HR analytics platforms and software available in the market, ranging from standalone predictive analytics tools to integrated HRIS systems with built-in analytics capabilities.
- Criteria for Evaluating and Choosing Predictive Analytics Tools: When selecting a predictive analytics tool, organizations should consider factors such as ease of use, scalability, integration capabilities, predictive modeling techniques supported, and vendor reputation.
Cultivating a Data-Driven HR Culture
- Training and Upskilling HR Professionals: HR teams should undergo training to familiarize themselves with data analytics concepts, tools, and techniques. This may involve attending workshops, webinars, or online courses on topics such as data analysis, statistics, and machine learning.
- Integrating Analytics into HR Decision-Making Processes: Organizations should encourage a culture of data-driven decision-making, where HR professionals use data and analytics to inform their strategic initiatives, such as recruitment, talent management, and workforce planning.
- Overcoming Resistance and Fostering Buy-In: Some HR professionals may be resistant to change or skeptical about the benefits of predictive analytics. It’s essential to communicate the value proposition of predictive analytics, demonstrate tangible benefits through pilot projects or case studies, and involve key stakeholders in the process to gain buy-in and support.
Example: Predictive Recruitment Analytics
Consider a scenario where a company is experiencing high turnover rates among sales representatives.
By leveraging predictive analytics, the HR team analyzes historical data on successful and unsuccessful hires, including factors such as previous sales experience, educational background, and performance metrics.
Based on these insights, HR develops predictive models to identify the characteristics and traits that are most predictive of success in sales roles.
Armed with this information, the company can refine its recruitment criteria, target candidates who are more likely to succeed in the role, and ultimately reduce turnover rates and improve sales performance.
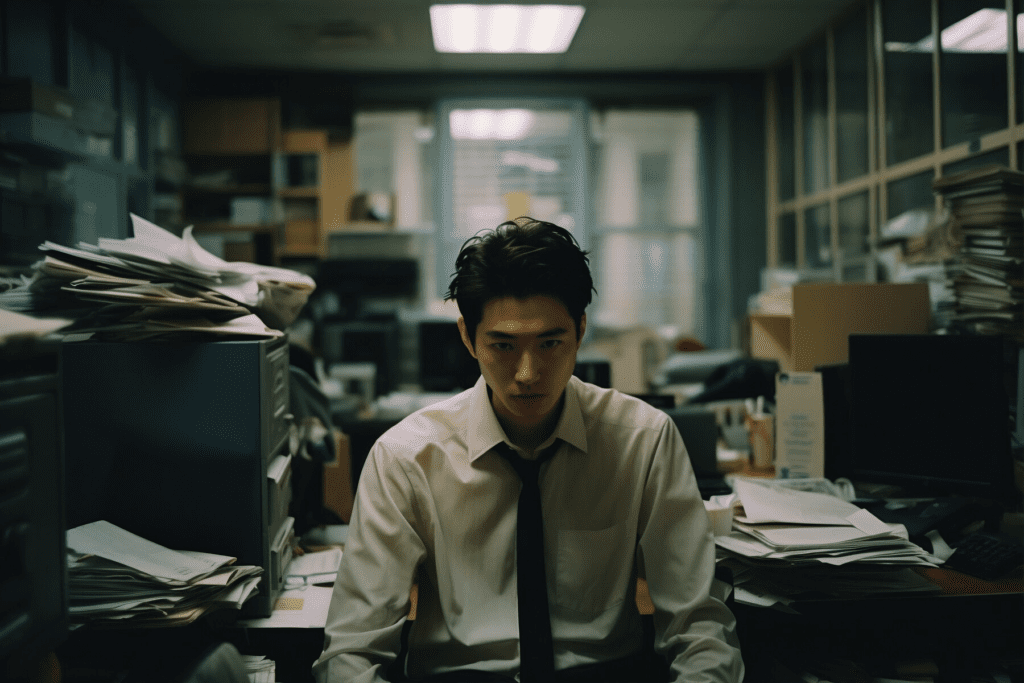
Example: Employee Performance Prediction
Another example of predictive analytics in HR is employee performance prediction.
By analyzing data on employee demographics, job roles, skills, and past performance evaluations, HR can develop predictive models to forecast future performance outcomes.
These models can help HR identify high-potential employees, allocate resources more effectively, and design targeted development plans to enhance employee performance and career growth.
Getting started with predictive analytics in HR requires a strategic approach, including building a solid foundation of data collection and management, selecting the right tools and technologies, and fostering a data-driven culture within the organization.
By following these essential steps and leveraging relevant examples, organizations can harness the power of predictive analytics to optimize their talent management strategies, drive organizational performance, and gain a competitive edge in today’s fast-paced business environment.
3. Implementing Predictive Analytics Projects in HR
Implementing predictive analytics projects in HR requires careful planning, execution, and ongoing refinement to ensure success.
Let’s explore the essential steps and best practices for effectively implementing predictive analytics projects in HR:
Defining Clear Objectives and KPIs
- Aligning with Organizational Goals: Before embarking on a predictive analytics project, HR teams should clearly define the objectives and ensure alignment with the organization’s strategic goals. Whether the goal is to improve recruitment efficiency, reduce employee turnover, or enhance performance management, it’s essential to articulate the desired outcomes.
- Establishing Measurable Outcomes and Success Criteria: HR teams should define key performance indicators (KPIs) and metrics to measure the success of the predictive analytics project. These metrics may include metrics such as time-to-fill for recruitment, turnover rates, employee engagement scores, or performance ratings.
Data Analysis and Model Development
- Exploratory Data Analysis (EDA): Before building predictive models, HR teams should conduct exploratory data analysis to understand the underlying patterns, relationships, and trends in the data. This involves visualizing data, identifying correlations, and uncovering insights that may inform model development.
- Building Predictive Models: HR professionals can leverage various predictive modeling techniques, such as regression analysis, decision trees, random forests, or neural networks, depending on the nature of the HR problem and the available data. It’s essential to select the most appropriate modeling approach and fine-tune the model parameters to optimize performance.
Interpretation and Validation of Results
- Interpreting Model Outputs and Insights: Once predictive models are developed, HR teams should interpret the results and extract actionable insights. This involves understanding the model predictions, identifying influential variables or features, and translating the findings into meaningful HR strategies or interventions.
- Validating Model Performance and Accuracy: HR teams should validate the predictive models using appropriate validation techniques, such as cross-validation or holdout validation, to assess their performance and accuracy. Validation helps ensure that the models generalize well to unseen data and provide reliable predictions in real-world scenarios.
Iterative Improvement and Refinement
- Continuous Monitoring and Recalibration: Predictive analytics projects in HR are not one-time endeavors but ongoing initiatives that require continuous monitoring and recalibration. HR teams should monitor model performance over time, track changes in data patterns or distributions, and recalibrate the models as needed to maintain accuracy and relevance.
- Feedback Loops for Ongoing Improvement: Incorporating feedback loops into predictive analytics projects enables HR teams to continuously learn and improve. By collecting feedback from stakeholders, evaluating the impact of predictive insights on HR outcomes, and incorporating learnings into future iterations, organizations can drive continuous improvement and innovation in their HR practices.
Example: Predictive Performance Management
Imagine a company aiming to improve its performance management process using predictive analytics. HR collects data on various employee attributes, such as skills, experience, and performance metrics, and develops predictive models to forecast future performance outcomes.
By leveraging these models, HR can identify high-potential employees, design targeted development plans, and allocate resources more effectively to drive performance improvement initiatives.
Example: Predictive Workforce Planning
Another example of predictive analytics in HR is workforce planning.
By analyzing historical workforce data, including demographics, turnover rates, and business demand forecasts, HR can develop predictive models to forecast future workforce needs.
These models enable HR to anticipate talent gaps, identify critical skill shortages, and develop proactive recruitment and training strategies to ensure that the organization has the right talent in place to meet its business objectives.
Implementing predictive analytics projects in HR requires a systematic approach, including defining clear objectives and KPIs, conducting data analysis and model development, interpreting and validating results, and iteratively improving and refining predictive models over time.
By following these essential steps and leveraging relevant examples, organizations can harness the power of predictive analytics to optimize their HR practices, drive better business outcomes, and gain a competitive advantage in today’s dynamic business environment.
4. Overcoming Challenges and Pitfalls
Implementing predictive analytics projects in HR comes with its own set of challenges and pitfalls.
However, with careful planning and mitigation strategies, organizations can overcome these obstacles and maximize the success of their analytics initiatives.
Let’s explore some common challenges and effective strategies for overcoming them:
Common Obstacles in Predictive Analytics Projects
- Data Quality Issues and Biases: Poor data quality, incomplete datasets, and biases in data collection can significantly impact the accuracy and reliability of predictive models. Biases may arise from factors such as sample selection bias, where certain demographic groups are underrepresented in the data, or measurement bias, where subjective criteria are used to assess performance.
- Lack of Stakeholder Alignment and Support: Resistance from stakeholders, including HR professionals, managers, and employees, can impede the adoption and implementation of predictive analytics projects. Resistance may stem from skepticism about the value of predictive analytics, concerns about job security or privacy, or a lack of understanding of the technology and its implications.
- Technological Constraints and Limitations: Limited access to advanced analytics tools and technologies, legacy systems, and IT infrastructure constraints can pose challenges to implementing predictive analytics projects in HR. Organizations may lack the necessary resources, expertise, or budget to invest in cutting-edge analytics solutions, hindering their ability to leverage predictive analytics effectively.
Strategies for Mitigating Risks and Maximizing Success
- Proactive Data Governance and Management Practices: Implementing robust data governance practices, including data quality assessment, data lineage tracking, and data stewardship programs, can help organizations ensure the accuracy, integrity, and reliability of their HR data. By establishing data standards, policies, and procedures, organizations can minimize the risk of data quality issues and biases.
- Communication and Collaboration Across Departments: Building cross-functional partnerships and fostering collaboration between HR, IT, and other relevant departments is essential for the success of predictive analytics projects. By involving key stakeholders from the outset, organizations can ensure alignment with business objectives, secure buy-in and support, and leverage diverse perspectives and expertise to address challenges effectively.
- Leveraging External Expertise and Resources: Organizations can supplement internal capabilities by leveraging external expertise and resources, such as consulting firms, data analytics vendors, or academic institutions. External partners can provide specialized skills, industry knowledge, and best practices, accelerating the implementation process and helping organizations navigate complex technical and strategic challenges.
Example: Addressing Data Quality Issues
Consider a scenario where a company experiences data quality issues in its predictive analytics project due to inconsistent data entry practices and outdated HR systems.
To address this challenge, the HR team implements data cleansing and normalization techniques to standardize the data and remove duplicates or errors.
Additionally, the organization invests in data governance tools and processes to monitor data quality continuously and enforce data standards across the organization, ensuring the accuracy and reliability of predictive models.
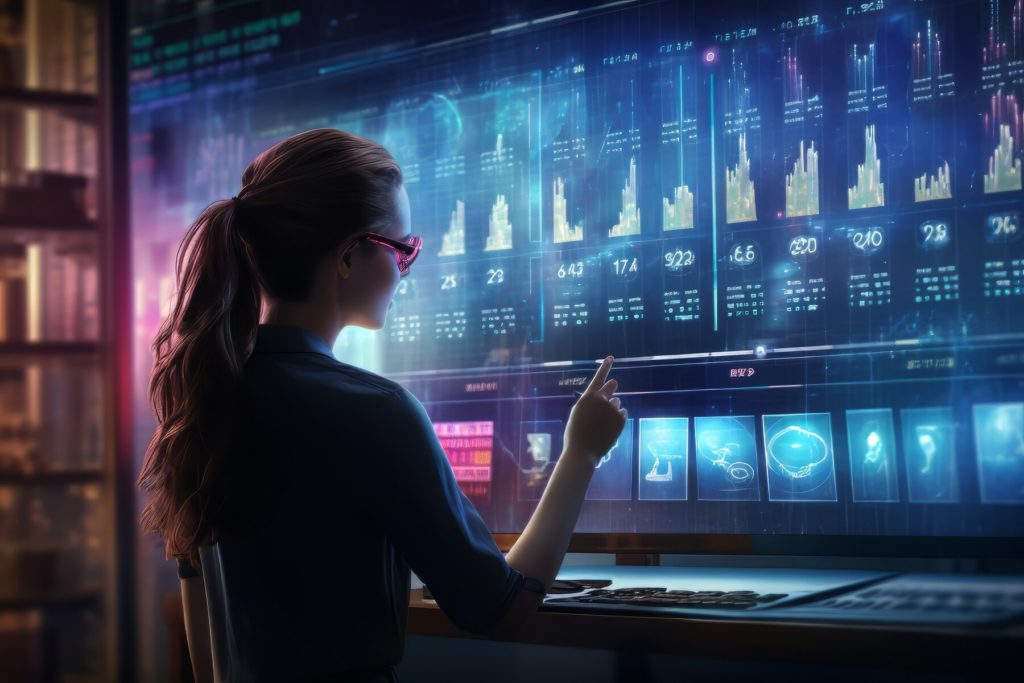
Example: Fostering Stakeholder Alignment
In another example, a company faces resistance from HR professionals and managers who are skeptical about the value of predictive analytics and concerned about job security.
To overcome this challenge, the HR team initiates a comprehensive communication and change management strategy, highlighting the potential benefits of predictive analytics for improving HR decision-making, enhancing employee engagement, and driving business results.
The organization conducts training sessions, workshops, and town hall meetings to educate stakeholders about the capabilities of predictive analytics, address their concerns, and involve them in the project planning and implementation process.
Overcoming challenges and pitfalls in predictive analytics projects requires a proactive and strategic approach, including addressing data quality issues, fostering stakeholder alignment and support, and leveraging external expertise and resources.
By implementing robust data governance practices, fostering collaboration across departments, and communicating the value of predictive analytics, organizations can mitigate risks, maximize the success of their analytics initiatives, and unlock the full potential of predictive analytics for HR.
5. Ethical Considerations in Predictive Analytics
Predictive analytics in HR holds immense potential for optimizing talent management strategies and driving organizational success.
However, it also raises important ethical considerations that organizations must address to ensure fairness, transparency, and accountability in their use of predictive models.
Let’s explore some key ethical considerations and best practices for navigating them:
Addressing Fairness and Bias Concerns
- Ensuring Fairness in Algorithmic Decision-Making: Organizations must strive to ensure that predictive models do not discriminate against individuals based on protected characteristics such as race, gender, age, or ethnicity. It’s essential to assess the fairness of algorithms using fairness metrics and techniques such as fairness-aware machine learning algorithms.
- Mitigating Bias in Data Collection and Model Development: Biases in data can lead to biased outcomes in predictive models. Organizations should carefully evaluate their data sources and preprocessing techniques to identify and mitigate potential biases. This may involve removing biased variables, augmenting underrepresented groups, or adjusting model outputs to achieve fairness.
Protecting Employee Privacy and Confidentiality
- Adhering to Data Protection Regulations: Organizations must comply with data protection regulations such as the General Data Protection Regulation (GDPR) and the California Consumer Privacy Act (CCPA) when collecting, processing, and storing employee data. This includes obtaining informed consent from employees, implementing data security measures, and providing transparency about data usage.
- Implementing Transparent and Ethical Data Practices: Organizations should adopt transparent and ethical data practices, including providing clear explanations of how predictive analytics are used in HR decision-making, allowing individuals to access and correct their personal data, and limiting data access to authorized personnel only.
Example: Addressing Bias in Recruitment Algorithms
Imagine a company uses predictive analytics to screen job applicants based on their likelihood of success in a sales role.
However, the predictive model inadvertently discriminates against candidates from underrepresented minority groups, leading to biased hiring outcomes.
To address this issue, the company conducts a bias audit of its recruitment algorithms, identifies biased variables or features, and adjusts the model to achieve fairness. Additionally, the company implements measures to diversify its talent pipeline and ensure equitable hiring practices.
Example: Protecting Employee Privacy in Performance Prediction
In another example, a company develops predictive models to forecast employee performance based on historical data.
However, employees express concerns about the privacy implications of using their personal data for predictive analytics.
To address these concerns, the company implements stringent data protection measures, including anonymizing employee data, limiting access to sensitive information, and providing transparency about how predictive analytics are used.
Additionally, the company educates employees about their rights regarding data privacy and ensures that their consent is obtained before using their data for predictive modeling.
Ethical considerations are paramount in the use of predictive analytics in HR to ensure fairness, transparency, and accountability.
By addressing fairness and bias concerns, protecting employee privacy and confidentiality, and implementing transparent and ethical data practices, organizations can leverage predictive analytics responsibly to optimize talent management strategies while upholding ethical standards and legal regulations.
As predictive analytics continues to evolve, it’s essential for organizations to prioritize ethical considerations and foster a culture of ethical data use in HR analytics.
6. Future Trends and Opportunities
As technology continues to advance, and organizations increasingly rely on data-driven insights to inform their HR strategies, predictive analytics is poised to play an even more significant role in shaping the future of talent management.
Let’s explore some emerging trends and opportunities in predictive analytics for HR:
Advancements in Artificial Intelligence (AI) and Machine Learning
- Leveraging Deep Learning for HR Insights: Deep learning techniques, such as neural networks and natural language processing (NLP), offer unprecedented capabilities for extracting insights from unstructured HR data sources, such as employee feedback, performance reviews, and social media sentiment.
- Automation of HR Processes through AI: AI-powered automation technologies, including chatbots, virtual assistants, and robotic process automation (RPA), are streamlining routine HR tasks, such as candidate screening, onboarding, and performance evaluations, freeing up HR professionals to focus on more strategic initiatives.
Predictive Workforce Analytics in the Era of Remote Work
- Adapting to Remote Work Dynamics: The shift to remote work due to the COVID-19 pandemic has necessitated a reevaluation of workforce planning and management strategies. Predictive analytics can help organizations anticipate the impact of remote work on employee productivity, engagement, and well-being, enabling them to adapt their HR policies and practices accordingly.
- Optimizing Virtual Team Dynamics: Predictive analytics can help HR teams optimize virtual team dynamics by analyzing collaboration patterns, communication styles, and team composition to identify factors that contribute to team success and productivity in remote work environments.
Personalization and Employee Experience
- Customizing HR Interventions and Policies: Predictive analytics enables HR teams to personalize HR interventions and policies based on individual employee needs, preferences, and performance profiles. By tailoring training programs, career development plans, and benefits packages to the unique needs of each employee, organizations can enhance employee satisfaction, engagement, and retention.
- Enhancing Employee Well-Being and Productivity: Predictive analytics can help organizations identify factors that impact employee well-being and productivity, such as workload, stress levels, and work-life balance. By proactively addressing these factors through targeted interventions, organizations can create a supportive work environment that fosters employee well-being and enhances overall productivity.
Example: AI-Powered Talent Acquisition
Imagine a company leveraging AI-powered chatbots to streamline its talent acquisition process.
These chatbots engage with job applicants via text or voice chat, answering questions about the company, conducting initial screenings, and scheduling interviews.
By automating routine tasks and providing real-time assistance to candidates, the chatbots enhance the candidate experience, reduce time-to-hire, and enable HR professionals to focus on higher-value activities, such as candidate engagement and relationship-building.
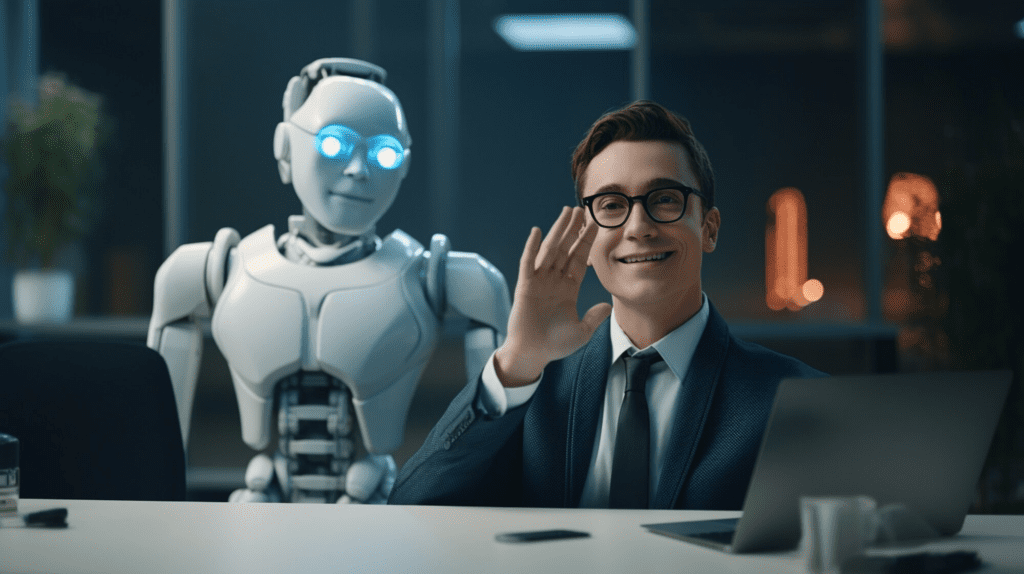
Example: Predictive Analytics for Employee Well-Being
In another example, a company utilizes predictive analytics to identify factors contributing to employee burnout and stress.
By analyzing data from employee surveys, performance evaluations, and health and wellness programs, HR can develop predictive models to forecast employees at risk of burnout and proactively implement interventions, such as flexible work arrangements, wellness initiatives, and stress management programs, to support employee well-being and prevent burnout.
The future of predictive analytics in HR is filled with exciting opportunities to revolutionize talent management practices and drive organizational success.
By embracing advancements in AI and machine learning, adapting to remote work dynamics, and prioritizing personalization and employee experience, organizations can harness the power of predictive analytics to optimize HR strategies, enhance employee engagement and well-being, and gain a competitive edge in today’s rapidly evolving business landscape.
As predictive analytics continues to evolve, HR professionals must stay abreast of emerging trends and technologies to remain at the forefront of HR
Conclusion
Predictive analytics in HR represents a transformative approach to talent management that leverages data-driven insights to drive strategic decision-making and optimize HR processes.
Throughout this comprehensive guide, we’ve explored the fundamentals of predictive analytics in HR, from defining its core concepts and methodologies to understanding its applications and implementation best practices.
Predictive analytics enables HR professionals to anticipate future workforce trends, identify potential risks, and proactively devise strategies to mitigate them.
By leveraging historical data, statistical algorithms, and machine learning techniques, organizations can gain valuable insights into their workforce dynamics, enabling them to make informed decisions that drive efficiency, productivity, and overall business performance.
From predicting candidate success in recruitment to forecasting employee turnover and performance outcomes, the applications of predictive analytics in HR are diverse and impactful.
By harnessing the predictive power of data, organizations can optimize their talent management strategies, enhance employee engagement and retention, and gain a competitive edge in today’s dynamic business landscape.
However, implementing predictive analytics projects in HR comes with its own set of challenges and pitfalls, including data quality issues, stakeholder resistance, and ethical considerations.
Addressing these challenges requires a strategic approach, including building a solid foundation of data collection and management, selecting the right tools and technologies, and fostering a data-driven culture within the organization.
Moreover, as technology continues to advance, and organizations increasingly rely on data-driven insights to inform their HR strategies, the future of predictive analytics in HR holds immense potential.
Emerging trends such as advancements in artificial intelligence and machine learning, the adaptation to remote work dynamics, and the prioritization of personalization and employee experience present exciting opportunities to revolutionize talent management practices and drive organizational success.
In essence, predictive analytics in HR is not just a tool; it’s a strategic imperative for organizations looking to stay ahead in today’s competitive business landscape.
By embracing predictive analytics, HR professionals can unlock the full potential of their workforce, drive better business outcomes, and create a workplace where employees thrive.
As predictive analytics continues to evolve, HR professionals must remain agile, adaptable, and forward-thinking to leverage its transformative power effectively.
If your company needs HR, hiring, or corporate services, you can use 9cv9 hiring and recruitment services. Book a consultation slot here, or send over an email to [email protected].
If you find this article useful, why not share it with your hiring manager and C-level suite friends and also leave a nice comment below?
We, at the 9cv9 Research Team, strive to bring the latest and most meaningful data, guides, and statistics to your doorstep.
To get access to top-quality guides, click over to 9cv9 Blog.
People Also Ask
How is predictive analytics used in HR?
Predictive analytics in HR is used to forecast future trends and behaviors related to workforce dynamics, such as recruitment success, employee turnover, and performance outcomes. By leveraging historical data and statistical algorithms, HR professionals can make informed decisions to optimize talent management strategies and drive organizational success.
What is a predictive approach in HR?
The predictive approach in HR involves using historical data and statistical algorithms to anticipate future workforce trends and behaviors. By analyzing patterns and correlations, HR professionals can make proactive decisions to optimize recruitment, retention, and performance management strategies, driving organizational success.
What is prescriptive analytics in HR?
Prescriptive analytics in HR goes beyond predicting future outcomes to recommend specific actions to achieve desired outcomes. By analyzing data and leveraging advanced algorithms, HR professionals can make data-driven recommendations for optimizing HR processes, such as talent acquisition, performance management, and employee development, leading to more effective decision-making and improved business results.