Key Takeaways
- AI detection software is essential for identifying and mitigating AI-generated content, such as deepfakes and synthetic text, to maintain digital integrity and security.
- Understanding the workings of AI detection software—leveraging machine learning, pattern recognition, and real-time analysis—is crucial for organizations combating AI-driven threats.
- Despite its effectiveness, AI detection software faces challenges like evolving AI techniques, ethical concerns, and computational demands, necessitating ongoing innovation and global collaboration.
In the rapidly evolving landscape of artificial intelligence, the line between what is real and what is artificially generated has become increasingly blurred.
AI technologies have made remarkable strides, revolutionizing industries from healthcare to finance, entertainment to cybersecurity.
However, with these advancements comes a new set of challenges, chief among them the ability to discern human-created content from AI-generated output.
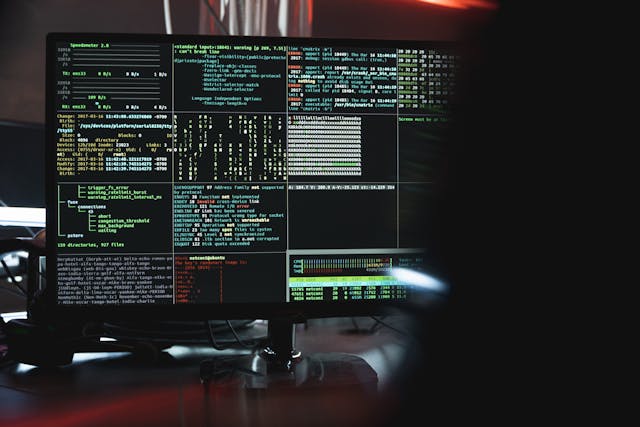
This is where AI detection software steps in, playing a pivotal role in maintaining the integrity, security, and trustworthiness of digital content and transactions.
As AI continues to integrate into various aspects of daily life, its potential to create highly convincing content—be it text, images, videos, or even entire conversations—has raised significant concerns.
From deepfakes that can manipulate video footage to generate realistic yet entirely fabricated scenarios, to AI-generated text that can mimic human writing with uncanny accuracy, the potential for misuse is vast.
These capabilities, while showcasing the power of AI, also present risks that can undermine trust in digital information, lead to widespread misinformation, and even pose threats to personal and national security.
AI detection software emerges as a critical tool in addressing these concerns.
It is specifically designed to identify, analyze, and flag content or activities that are artificially generated by AI, ensuring that individuals, businesses, and governments can distinguish between genuine human-created content and that which has been crafted by machines.
This detection capability is not just about identifying potential threats; it’s also about preserving the authenticity of content, protecting intellectual property, and maintaining ethical standards in the digital realm.
Understanding what AI detection software is and how it operates is essential for anyone navigating today’s increasingly AI-driven world.
This technology is complex, relying on advanced algorithms, machine learning, and vast datasets to detect subtle patterns and anomalies that indicate AI involvement.
As AI systems become more sophisticated, so too must the methods we use to detect and mitigate their influence.
In this article, we will delve deep into the world of AI detection software, exploring its definition, the technologies that power it, and the critical role it plays in various industries.
We will also examine the challenges it faces in keeping pace with the rapid evolution of AI technologies and consider what the future holds for this indispensable tool.
Whether you are a tech enthusiast, a business leader, or simply someone curious about the impact of AI on our world, this comprehensive guide will provide you with the insights needed to understand and appreciate the importance of AI detection software in today’s digital age.
Before we venture further into this article, we would like to share who we are and what we do.
About 9cv9
9cv9 is a business tech startup based in Singapore and Asia, with a strong presence all over the world.
With over eight years of startup and business experience, and being highly involved in connecting with thousands of companies and startups, the 9cv9 team has listed some important learning points in this overview of What is AI Detection Software and How It Works.
If your company needs recruitment and headhunting services to hire top-quality employees, you can use 9cv9 headhunting and recruitment services to hire top talents and candidates. Find out more here, or send over an email to [email protected].
Or just post 1 free job posting here at 9cv9 Hiring Portal in under 10 minutes.
What is AI Detection Software and How It Works
- The Growing Need for AI Detection Software
- What is AI Detection Software?
- How AI Detection Software Works
- Key Applications of AI Detection Software
- Challenges and Limitations
- The Future of AI Detection Software
1. The Growing Need for AI Detection Software
The rapid advancement of artificial intelligence has brought about transformative changes across numerous industries.
However, alongside these innovations, there has been a surge in the potential misuse of AI technologies. As AI-generated content becomes more sophisticated, the demand for AI detection software has grown significantly. This section explores the various factors driving the need for such software, supported by relevant examples.
1. Proliferation of AI-Generated Content
- Increase in AI-Generated Media:
- AI technologies like GPT models, DALL-E, and others have enabled the creation of highly realistic text, images, and videos.
- Example: AI-generated articles or reports that mimic human writing styles can be challenging to distinguish from those authored by humans, leading to potential issues with plagiarism or misinformation.
- Deepfakes and Synthetic Media:
- The rise of deepfake technology has made it possible to create highly realistic videos where individuals appear to say or do things they never did.
- Example: Deepfake videos of public figures used to spread misinformation or discredit individuals have become a significant concern, especially in the context of elections or sensitive geopolitical events.
- Automated Content Creation:
- AI tools that generate content for social media, advertising, and marketing can flood the digital space with synthetic content.
- Example: Automated social media accounts (bots) can produce and distribute large volumes of AI-generated posts, potentially skewing public opinion or spreading false information.
2. Security Threats and Cybersecurity Concerns
- AI-Driven Cyberattacks:
- AI is being used to develop more sophisticated cyberattacks, including phishing, malware distribution, and network intrusion.
- Example: AI-generated phishing emails that closely mimic legitimate communications can trick even the most vigilant recipients into compromising their security.
- AI in Fraudulent Activities:
- Cybercriminals are leveraging AI to create fraudulent content, such as fake identities or synthetic accounts, to bypass security measures.
- Example: AI-generated fake identities are used to commit financial fraud, apply for loans, or launder money, posing significant challenges to financial institutions.
- Detection of Anomalies:
- AI detection software is essential in identifying anomalies in network traffic, which may indicate the presence of AI-driven attacks.
- Example: AI-based anomaly detection can identify unusual patterns of behavior within a network, flagging potential security breaches before they cause significant harm.
3. Misinformation and Fake News
- Spread of AI-Generated Misinformation:
- AI can be used to generate and spread fake news at an unprecedented scale, making it difficult for users to distinguish between factual and fabricated information.
- Example: During major global events, AI-generated fake news articles can go viral, misleading the public and influencing opinions based on false narratives.
- Impact on Public Trust:
- The proliferation of AI-generated misinformation erodes public trust in media and institutions.
- Example: AI-generated deepfake videos of political leaders or public figures can create confusion, distrust, and societal division, especially during critical moments such as elections.
- Challenges in Content Verification:
- Traditional content verification methods struggle to keep up with the speed and sophistication of AI-generated content.
- Example: Fact-checking organizations require advanced AI detection tools to quickly and accurately verify the authenticity of viral content on social media platforms.
4. Ethical and Legal Implications
- Intellectual Property Concerns:
- AI-generated content raises questions about intellectual property rights and ownership.
- Example: Artists and content creators may find their work replicated by AI without consent, leading to legal disputes over copyright infringement.
- Privacy and Surveillance Issues:
- AI’s ability to generate realistic content can be used to infringe on privacy or conduct unauthorized surveillance.
- Example: AI-generated deepfakes can be used to create compromising or defamatory content without the subject’s knowledge or consent, leading to severe privacy violations.
- Regulatory and Compliance Challenges:
- Governments and organizations face challenges in regulating AI-generated content and ensuring compliance with existing laws.
- Example: The lack of clear regulations around AI-generated content has led to a gray area where malicious actors can exploit the technology without facing legal consequences.
5. The Role of AI Detection Software in Addressing These Challenges
- Enhancing Security Measures:
- AI detection software helps strengthen cybersecurity defenses by identifying and mitigating AI-driven threats.
- Example: Financial institutions use AI detection tools to monitor transactions for signs of fraud, ensuring the security of customer assets.
- Preserving Content Authenticity:
- AI detection software plays a crucial role in maintaining the authenticity of digital content, especially in journalism and media.
- Example: News organizations use AI detection tools to verify the authenticity of images and videos before publication, reducing the risk of spreading misinformation.
- Supporting Legal and Ethical Standards:
- AI detection software aids in enforcing legal and ethical standards by identifying and preventing the misuse of AI-generated content.
- Example: Legal firms utilize AI detection tools to gather evidence in cases involving deepfake videos or AI-generated fraud, supporting the pursuit of justice.
The need for AI detection software is more critical than ever as AI technologies continue to evolve and integrate into various aspects of life.
From combating cybersecurity threats to preserving the integrity of information and upholding ethical standards, AI detection software is indispensable in navigating the challenges posed by AI’s rapid advancement.
2. What is AI Detection Software?
AI detection software is an increasingly vital tool in the digital age, where artificial intelligence is pervasive across industries.
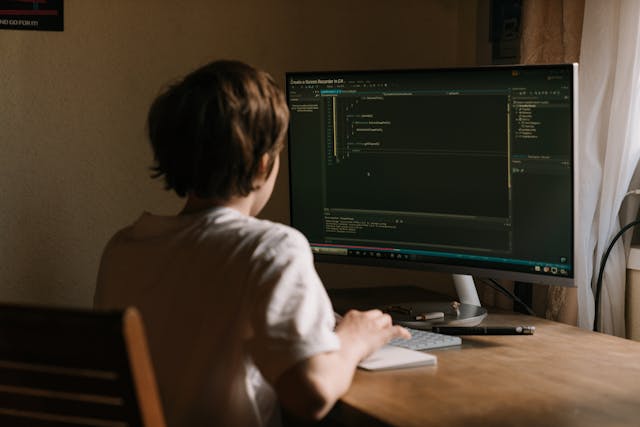
This software is designed to identify, analyze, and flag content or activities generated by AI, providing a safeguard against the potential misuse of AI technologies. Understanding the nuances of AI detection software is essential for grasping its role in maintaining the integrity, security, and authenticity of digital content. This section explores what AI detection software is, how it works, and its various applications.
1. Defining AI Detection Software
- Comprehensive Definition:
- AI detection software is a suite of tools and technologies specifically developed to detect content, activities, or data that has been created or manipulated by artificial intelligence.
- It employs advanced algorithms and machine learning models to analyze digital content and identify characteristics that suggest AI involvement.
- Core Objectives:
- The primary goal of AI detection software is to differentiate between human-generated and AI-generated content.
- It also aims to identify AI-driven anomalies in digital activities, such as fraud, misinformation, and unauthorized content creation.
- Importance in the Digital Age:
- As AI becomes more sophisticated, the distinction between real and AI-generated content blurs, making detection software crucial for maintaining trust and authenticity.
- Example: In journalism, AI detection software is used to verify the authenticity of news articles and images, ensuring that they have not been manipulated by AI.
2. How AI Detection Software Works
AI detection software operates through a series of complex processes that involve data analysis, pattern recognition, and machine learning. These processes enable the software to identify AI-generated content or activities with high accuracy.
- Underlying Technologies:
- Machine Learning (ML):
- AI detection software relies on machine learning algorithms to learn from vast datasets and improve its detection capabilities over time.
- Example: A machine learning model trained on a large corpus of human and AI-generated text can identify subtle differences in writing style, flagging content that appears to be AI-generated.
- Natural Language Processing (NLP):
- NLP techniques are used to analyze and understand the language patterns in text-based content, making it possible to detect AI-generated text.
- Example: AI detection software can identify AI-generated text by analyzing sentence structure, word usage, and coherence, which may differ from human writing.
- Computer Vision:
- For detecting AI-generated images or videos, computer vision technology is employed to analyze visual content.
- Example: AI detection software can spot inconsistencies in deepfake videos by analyzing facial movements and lighting patterns that may not align with real-life scenarios.
- Machine Learning (ML):
- Data Collection and Analysis:
- Gathering Data:
- The software collects data from various sources, including text, images, videos, and network activities, to build a comprehensive dataset for analysis.
- Example: AI detection tools used in cybersecurity gather data from network traffic to identify patterns indicative of AI-driven attacks.
- Analyzing Patterns:
- The software analyzes the collected data to identify patterns or anomalies that suggest AI involvement.
- Example: In financial services, AI detection software analyzes transaction patterns to detect fraudulent activities that may have been facilitated by AI.
- Gathering Data:
- Pattern Recognition and Anomaly Detection:
- Identifying AI Signatures:
- AI detection software looks for specific patterns, known as AI signatures, that are characteristic of AI-generated content.
- Example: AI-generated images often exhibit certain imperfections, such as unnatural lighting or inconsistent details, which can be detected by the software.
- Detecting Anomalies:
- The software flags anomalies in content or behavior that deviate from the norm, which may indicate AI manipulation.
- Example: In social media, AI detection tools can identify bot-generated posts by analyzing posting frequency, language patterns, and user interactions.
- Identifying AI Signatures:
- Model Training and Continuous Learning:
- Training Models:
- The effectiveness of AI detection software depends on the quality of the models it uses, which are trained on large datasets of both AI-generated and human-generated content.
- Example: An AI detection model trained on a diverse set of deepfake videos can improve its accuracy in identifying new deepfakes.
- Continuous Learning:
- AI detection software continuously learns and adapts to new AI techniques, ensuring it remains effective as AI technology evolves.
- Example: As AI-generated content becomes more sophisticated, the detection software updates its models to recognize new patterns and anomalies.
- Training Models:
3. Types of AI Detection Software
AI detection software comes in various forms, each tailored to detect specific types of AI-generated content or activities. Below are some common types of AI detection software, along with relevant examples.
- Text Detection Software:
- Purpose:
- Detects AI-generated text in articles, emails, social media posts, and other written content.
- Example:
- A content verification tool used by journalists to ensure that news articles are free from AI-generated text, preserving the integrity of the information.
- Purpose:
- Image and Video Detection Software:
- Purpose:
- Identifies AI-generated images and videos, such as deepfakes or synthetic media.
- Example:
- AI detection software used by social media platforms to automatically flag deepfake videos before they are uploaded and shared with users.
- Purpose:
- Fraud Detection Software:
- Purpose:
- Detects fraudulent activities facilitated by AI, such as fake accounts, synthetic identities, or AI-driven financial scams.
- Example:
- Financial institutions use AI detection software to monitor transactions for signs of fraud, ensuring that all activities are legitimate and compliant with regulations.
- Purpose:
- Network Anomaly Detection Software:
- Purpose:
- Identifies anomalies in network traffic that may indicate the presence of AI-driven cyberattacks or unauthorized activities.
- Example:
- AI detection tools in cybersecurity monitor network traffic for unusual patterns, such as sudden spikes in data transfer or unauthorized access attempts.
- Purpose:
4. Applications of AI Detection Software
AI detection software has broad applications across various industries, helping to protect against the risks associated with AI-generated content and activities. Below are some key application areas.
- Cybersecurity:
- Use Case:
- Enhances security by detecting AI-driven cyberattacks, such as phishing, malware, and network intrusions.
- Example:
- An AI detection system that identifies and blocks phishing emails generated by AI, preventing potential data breaches.
- Use Case:
- Content Verification and Media:
- Use Case:
- Verifies the authenticity of digital content, including images, videos, and text, to combat misinformation and deepfakes.
- Example:
- News organizations use AI detection software to verify the authenticity of images before publishing them, ensuring that they have not been manipulated.
- Use Case:
- Financial Services:
- Use Case:
- Detects AI-driven fraud, such as synthetic identities and fraudulent transactions, protecting financial institutions from losses.
- Example:
- A bank uses AI detection software to monitor transactions for patterns indicative of AI-generated fraud, such as unusual spending behavior or account anomalies.
- Use Case:
- Legal and Compliance:
- Use Case:
- Supports legal cases by identifying AI-generated evidence, such as deepfake videos or AI-manipulated documents.
- Example:
- Legal firms employ AI detection tools to analyze video evidence in cases where deepfakes are suspected, ensuring that justice is based on accurate and authentic information.
- Use Case:
- Education and Academia:
- Use Case:
- Detects AI-generated academic work, such as essays or research papers, to prevent plagiarism and uphold academic integrity.
- Example:
- Educational institutions use AI detection software to scan student submissions for AI-generated content, ensuring that all work is original.
- Use Case:
AI detection software is a powerful tool that plays a critical role in the modern digital landscape.
By understanding its functions, underlying technologies, and applications, organizations and individuals can better navigate the challenges posed by the increasing sophistication of AI-generated content and activities.
Whether it’s preserving the authenticity of information, enhancing cybersecurity, or supporting legal and ethical standards, AI detection software is indispensable in maintaining trust and integrity in the age of AI.
3. How AI Detection Software Works
AI detection software is a sophisticated tool that relies on a combination of advanced technologies and methodologies to identify, analyze, and flag AI-generated content or activities.
Understanding how this software operates requires a deep dive into the underlying processes, including data collection, pattern recognition, machine learning, and real-time detection.
This section provides a comprehensive overview of how AI detection software works, detailing each step and highlighting relevant examples to illustrate its functionality.
1. Data Collection and Preprocessing
Data collection is the first critical step in the operation of AI detection software. The software gathers vast amounts of data from various sources, which it then preprocesses to prepare for analysis.
- Sources of Data:
- Textual Data:
- Includes content from articles, social media posts, emails, and other written communications.
- Example: AI detection software collects a large corpus of news articles to distinguish between human-written and AI-generated content.
- Visual Data:
- Comprises images and videos, including deepfakes and AI-generated media.
- Example: The software scans social media platforms for images and videos that may have been manipulated by AI, such as deepfake videos of public figures.
- Behavioral Data:
- Involves patterns of user behavior, network traffic, and transactional activities.
- Example: AI detection software monitors network traffic in a corporate environment to identify unusual patterns that may indicate AI-driven cyberattacks.
- Textual Data:
- Preprocessing of Data:
- Cleaning Data:
- Removes noise, irrelevant information, and duplicates to ensure data quality.
- Example: Before analyzing textual content, the software filters out non-informative words, such as common stopwords, to focus on meaningful patterns.
- Data Normalization:
- Standardizes data to a consistent format, making it easier to analyze.
- Example: Images are resized to a uniform dimension and standardized in color space to facilitate accurate analysis.
- Feature Extraction:
- Identifies key features from the data that will be used in the detection process.
- Example: In text analysis, the software extracts linguistic features such as syntax, grammar, and word frequency to identify AI-generated content.
- Cleaning Data:
2. Pattern Recognition and Analysis
Once the data is collected and preprocessed, AI detection software uses pattern recognition techniques to analyze the data and identify signs of AI involvement.
- AI Signatures:
- Identifying Unique Patterns:
- The software searches for specific patterns, known as AI signatures, that are characteristic of AI-generated content.
- Example: AI-generated text may exhibit a repetitive structure or lack the subtle nuances of human writing, which the software can detect as AI signatures.
- Deep Learning Models:
- Employs deep learning algorithms to recognize complex patterns that may not be immediately apparent.
- Example: A deep learning model trained on deepfake videos can identify subtle inconsistencies in facial movements or lighting that indicate manipulation.
- Identifying Unique Patterns:
- Anomaly Detection:
- Identifying Deviations:
- The software compares the analyzed content against a baseline of normal behavior or content to detect anomalies.
- Example: In network security, AI detection software identifies anomalies in data traffic patterns, such as unexpected spikes in activity, which may signal an AI-driven attack.
- Behavioral Analysis:
- Monitors user or system behavior for irregularities that may indicate AI-generated activity.
- Example: In fraud detection, the software analyzes transaction behaviors that deviate from the norm, such as sudden large withdrawals, potentially flagging AI-driven financial fraud.
- Identifying Deviations:
- Contextual Analysis:
- Understanding Context:
- The software evaluates the context in which content or behavior occurs to improve detection accuracy.
- Example: AI detection software analyzing a news article will consider the context of the writing, such as the publication date and topic relevance, to determine if the content is AI-generated.
- Understanding Context:
3. Machine Learning and Model Training
Machine learning is at the heart of AI detection software, enabling it to learn from data, improve over time, and adapt to new AI techniques.
- Supervised Learning:
- Training with Labeled Data:
- Involves training models using labeled datasets, where the content is pre-identified as either AI-generated or human-created.
- Example: A model is trained on a dataset of known AI-generated and human-written essays, allowing it to learn the distinguishing features of each.
- Model Validation:
- The trained model is validated using a separate dataset to assess its accuracy and reliability.
- Example: After training, the AI detection model is tested on new, unlabeled text to see how accurately it can classify content as AI-generated or human-written.
- Training with Labeled Data:
- Unsupervised Learning:
- Identifying Patterns without Labels:
- The software analyzes data without pre-existing labels, discovering hidden patterns or anomalies.
- Example: An unsupervised learning model might be used to detect new types of AI-generated images by clustering similar images together and identifying outliers that may indicate AI manipulation.
- Clustering and Grouping:
- The software groups similar data points together, which can help in identifying patterns characteristic of AI-generated content.
- Example: The software may cluster text samples by writing style, identifying groups that share common AI-like features.
- Identifying Patterns without Labels:
- Reinforcement Learning:
- Continuous Improvement:
- The model learns through trial and error, receiving feedback on its performance and adjusting accordingly.
- Example: AI detection software used in cybersecurity might be continuously trained with new data on emerging threats, allowing it to improve its detection capabilities over time.
- Adapting to New Threats:
- The software can adapt to new AI techniques and methods as they emerge, ensuring it remains effective.
- Example: As AI-generated content evolves, the software updates its models to detect new types of manipulations, such as advanced deepfake techniques.
- Continuous Improvement:
4. Real-Time vs. Post-Process Detection
AI detection software can operate in different modes depending on the use case, either providing real-time detection or analyzing content after it has been created.
- Real-Time Detection:
- Instant Analysis:
- The software analyzes content or behavior as it is being generated, providing immediate detection and response.
- Example: Social media platforms use real-time AI detection to automatically flag and remove deepfake videos before they are shared widely.
- Applications:
- Commonly used in cybersecurity, social media moderation, and live content verification.
- Example: In live streaming, AI detection software can instantly detect and block AI-generated content that violates platform policies.
- Instant Analysis:
- Post-Process Detection:
- Analysis After Creation:
- The software examines content after it has been created or posted, identifying AI-generated elements during a subsequent review.
- Example: News organizations use post-process AI detection to verify the authenticity of images and videos submitted by journalists before publication.
- Applications:
- Often used in forensic analysis, legal investigations, and content verification.
- Example: Legal firms employ AI detection software to analyze video evidence after the fact, determining if it has been manipulated by AI to mislead the court.
- Analysis After Creation:
5. Examples of AI Detection Software in Action
The functionality of AI detection software is best illustrated through real-world examples that showcase its capabilities across different domains.
- Deepfake Detection:
- Example:
- AI detection software is employed by video-sharing platforms to scan uploaded content for deepfakes. The software analyzes facial movements, voice patterns, and other biometric data to determine if a video has been manipulated by AI.
- Impact:
- This helps prevent the spread of misleading or harmful deepfake content, protecting public figures and maintaining the integrity of online media.
- Example:
- AI-Generated Text Detection:
- Example:
- News agencies use AI detection tools to scan articles for signs of AI generation, such as unnatural phrasing or repetition. This ensures that the content published is authentic and not misleading.
- Impact:
- By filtering out AI-generated content, these agencies maintain credibility and avoid the pitfalls of spreading misinformation.
- Example:
- Fraud Detection in Financial Services:
- Example:
- Financial institutions deploy AI detection software to monitor transactions for signs of AI-driven fraud, such as the creation of synthetic identities or unusual spending patterns.
- Impact:
- This proactive approach helps banks prevent financial losses and protect customer assets from fraudulent activities.
- Example:
- Cybersecurity Applications:
- Example:
- AI detection tools are used in corporate networks to monitor for AI-driven attacks, such as AI-generated phishing emails. The software identifies suspicious behavior and blocks potential threats before they cause damage.
- Impact:
- Enhances the overall security posture of organizations, reducing the risk of data breaches and unauthorized access.
- Example:
AI detection software works through a complex interplay of data collection, pattern recognition, machine learning, and real-time analysis.
By leveraging these technologies, the software is able to detect AI-generated content and activities with high accuracy, providing crucial protection across various domains.
Whether in cybersecurity, content verification, financial services, or other fields, AI detection software is an essential tool for maintaining the integrity and security of digital content in the age of artificial intelligence.
4. Key Applications of AI Detection Software
AI detection software has become an indispensable tool across various industries, providing critical safeguards in areas ranging from cybersecurity to content verification.
Its ability to detect AI-generated content and activities has far-reaching implications, ensuring the integrity, security, and authenticity of digital environments.
This section explores the key applications of AI detection software, highlighting how it is used in different sectors and providing relevant examples to illustrate its impact.
1. Cybersecurity and Fraud Prevention
AI detection software plays a crucial role in cybersecurity, where it is employed to detect and prevent AI-driven threats such as phishing attacks, network intrusions, and fraudulent activities.
- AI-Driven Cyber Threat Detection:
- Detecting AI-Generated Phishing Emails:
- AI detection software scans emails for patterns indicative of AI-generated phishing attempts, such as unnatural language or inconsistent formatting.
- Example: A financial institution uses AI detection software to identify phishing emails that attempt to steal customer credentials, reducing the risk of data breaches.
- Preventing Network Intrusions:
- The software monitors network traffic for anomalies that suggest an AI-driven intrusion, such as unusual data transfer spikes or unauthorized access attempts.
- Example: A corporate IT department employs AI detection tools to safeguard against AI-generated malware designed to infiltrate their network.
- Detecting AI-Generated Phishing Emails:
- Fraud Detection in Financial Services:
- Identifying Synthetic Identities:
- AI detection software is used to detect synthetic identities created by AI to commit fraud, by analyzing patterns in account creation and usage.
- Example: Banks utilize AI detection software to flag suspicious accounts that exhibit unusual behavior, such as rapid transfers or inconsistent personal information.
- Monitoring Transactional Behavior:
- The software analyzes transactional data for signs of AI-generated fraud, such as atypical spending patterns or rapid, repeated transactions.
- Example: A credit card company uses AI detection software to monitor transactions for anomalies that could indicate AI-driven fraud, helping to prevent unauthorized charges.
- Identifying Synthetic Identities:
2. Content Verification and Media Integrity
In the age of misinformation and deepfakes, AI detection software is essential for verifying the authenticity of digital content, ensuring that it has not been manipulated by AI.
- Deepfake Detection:
- Identifying AI-Generated Videos:
- AI detection software analyzes video content for signs of deepfake manipulation, such as inconsistent facial movements or unnatural lighting.
- Example: A news outlet uses AI detection tools to verify the authenticity of videos submitted by citizen journalists, preventing the spread of manipulated footage.
- Combating Misinformation:
- The software flags deepfake videos and AI-generated images that could be used to spread misinformation, ensuring that only authentic content is shared.
- Example: Social media platforms deploy AI detection software to scan for deepfakes before they go viral, protecting users from misleading content.
- Identifying AI-Generated Videos:
- Text Content Verification:
- Detecting AI-Generated Articles:
- AI detection software examines text for patterns that suggest AI generation, such as repetitive phrasing or unnatural sentence structure.
- Example: A content verification platform uses AI detection tools to scan news articles for signs of AI generation, ensuring that the information is credible and trustworthy.
- Preserving Editorial Integrity:
- The software helps publishers and media organizations maintain editorial standards by filtering out AI-generated content that may not meet quality criteria.
- Example: An online magazine employs AI detection software to ensure that all published articles are original and free from AI-generated segments.
- Detecting AI-Generated Articles:
3. Legal and Compliance
AI detection software is increasingly used in the legal and compliance sectors to verify the authenticity of digital evidence, ensure regulatory compliance, and support legal investigations.
- Digital Evidence Verification:
- Analyzing AI-Manipulated Evidence:
- The software examines digital evidence, such as videos and documents, for signs of AI manipulation that could affect the outcome of legal cases.
- Example: A legal firm uses AI detection tools to analyze video evidence in a criminal case, ensuring that it has not been tampered with by deepfake technology.
- Supporting Forensic Investigations:
- AI detection software assists forensic teams in identifying and analyzing AI-generated or manipulated content, providing crucial insights in investigations.
- Example: Law enforcement agencies employ AI detection software to uncover doctored images or videos that could mislead investigations.
- Analyzing AI-Manipulated Evidence:
- Regulatory Compliance:
- Ensuring Compliance with Data Protection Laws:
- AI detection software helps organizations comply with data protection regulations by detecting and preventing the unauthorized use of AI-generated content.
- Example: A multinational corporation uses AI detection tools to monitor employee communications, ensuring compliance with GDPR and other data privacy regulations.
- Monitoring for AI-Driven Misconduct:
- The software tracks AI-generated activities that could violate regulatory standards, such as automated trading in financial markets.
- Example: Financial regulators use AI detection software to monitor trading activities for signs of AI-driven market manipulation, ensuring fair and transparent market practices.
- Ensuring Compliance with Data Protection Laws:
4. Academic Integrity and Education
In educational institutions, AI detection software is vital for maintaining academic integrity by identifying AI-generated work, such as essays or research papers, and preventing plagiarism.
- Detecting AI-Generated Academic Work:
- Essay and Paper Analysis:
- AI detection software scans student submissions for signs of AI generation, such as inconsistent writing styles or unusual linguistic patterns.
- Example: A university employs AI detection tools to check for AI-generated essays, ensuring that all student work is original and meets academic standards.
- Preventing Academic Dishonesty:
- The software helps educators identify and address instances of academic dishonesty involving AI-generated content.
- Example: An online education platform uses AI detection software to verify the authenticity of student submissions, preventing the use of AI tools to cheat on assignments.
- Essay and Paper Analysis:
- Upholding Research Standards:
- Verifying Research Integrity:
- AI detection software is used to ensure that research papers and publications are free from AI-generated content, maintaining the integrity of academic research.
- Example: Academic journals employ AI detection tools to screen submissions for AI-generated text, ensuring that all published research is original and credible.
- Promoting Ethical Use of AI:
- The software encourages ethical use of AI in academic work by providing tools to detect and discourage unauthorized AI-generated content.
- Example: Educational institutions promote AI detection software as part of their academic integrity policies, educating students on the importance of original work.
- Verifying Research Integrity:
5. Business and Corporate Security
In the corporate world, AI detection software is utilized to protect sensitive information, secure intellectual property, and monitor employee communications for AI-driven misconduct.
- Protecting Intellectual Property:
- Monitoring for Unauthorized AI Use:
- AI detection software tracks and prevents unauthorized AI-generated content that could infringe on a company’s intellectual property.
- Example: A technology firm uses AI detection tools to monitor for AI-generated content that mimics its proprietary designs or software, protecting its IP rights.
- Securing Corporate Communications:
- The software analyzes corporate communications to detect AI-generated emails or documents that could pose a security risk.
- Example: A multinational corporation employs AI detection software to secure internal communications, preventing AI-driven phishing attacks or data leaks.
- Monitoring for Unauthorized AI Use:
- Ensuring Corporate Compliance:
- Monitoring for AI-Driven Misconduct:
- AI detection software helps companies detect and address instances of AI-driven misconduct, such as automated insider trading or unauthorized content creation.
- Example: A financial services firm uses AI detection tools to monitor employee activities for signs of AI-driven trading violations, ensuring compliance with regulatory standards.
- Supporting Corporate Investigations:
- The software aids in internal investigations by identifying and analyzing AI-generated content that could be linked to corporate misconduct.
- Example: A corporate legal team uses AI detection software to analyze internal documents for signs of AI-generated fraud or misrepresentation.
- Monitoring for AI-Driven Misconduct:
6. Healthcare and Medical Research
AI detection software is also making significant inroads in the healthcare sector, where it is used to ensure the integrity of medical research, protect patient data, and detect AI-generated medical content.
- Verifying Medical Research Integrity:
- Detecting AI-Generated Research Papers:
- AI detection software scans medical research papers for AI-generated content, ensuring the accuracy and reliability of published findings.
- Example: A medical journal employs AI detection tools to screen submissions for AI-generated text, maintaining the credibility of its published research.
- Preventing AI-Driven Data Fabrication:
- The software helps prevent the fabrication of medical data by identifying inconsistencies or anomalies that may indicate AI manipulation.
- Example: A research institution uses AI detection software to verify the authenticity of clinical trial data, ensuring that results are not manipulated by AI.
- Detecting AI-Generated Research Papers:
- Protecting Patient Data:
- Monitoring for AI-Generated Data Breaches:
- AI detection software tracks and prevents AI-driven attempts to breach patient data, safeguarding sensitive information.
- Example: A healthcare provider employs AI detection tools to monitor its networks for signs of AI-generated cyberattacks aimed at accessing patient records.
- Ensuring Compliance with Health Regulations:
- The software helps healthcare organizations comply with regulations like HIPAA by detecting unauthorized AI-generated activities that could compromise patient privacy.
- Example: A hospital uses AI detection software to ensure that all communications involving patient data are secure and compliant with health regulations.
- Monitoring for AI-Generated Data Breaches:
AI detection software is a versatile tool with wide-ranging applications across various industries. Its ability to detect and prevent AI-generated content and activities has become essential in maintaining security, integrity, and compliance in today’s increasingly AI-driven world.
Whether in cybersecurity, content verification, legal compliance, education, business, or healthcare, AI detection software is helping organizations navigate the challenges and opportunities presented by artificial intelligence.
5. Challenges and Limitations
While AI detection software offers significant advantages in identifying and managing AI-generated content, it is not without its challenges and limitations.
The rapidly evolving landscape of artificial intelligence presents numerous hurdles that make the detection process complex and sometimes imperfect.
This section delves into the key challenges and limitations faced by AI detection software, providing examples where applicable to illustrate the impact of these issues on various applications.
1. Evolving Nature of AI
The rapid advancement of AI technologies is a major challenge for detection software, which must constantly adapt to keep pace with new developments.
- AI’s Ability to Mimic Human Behavior:
- Increasing Sophistication of AI-Generated Content:
- As AI models improve, they become better at mimicking human behavior, making it more difficult for detection software to distinguish between human-created and AI-generated content.
- Example: AI-generated text from advanced language models like GPT-4 can closely mimic human writing styles, leading to false negatives where AI detection software fails to identify AI involvement.
- Continuous Learning and Improvement:
- AI models continuously learn from new data, evolving their capabilities to bypass detection methods that once worked effectively.
- Example: Deepfake technologies have advanced to the point where subtle cues that once indicated AI manipulation, such as unnatural eye movements, are now much harder to detect.
- Increasing Sophistication of AI-Generated Content:
- Emergence of New AI Techniques:
- AI’s Capacity for Innovation:
- New AI techniques and methodologies are constantly being developed, some of which may be specifically designed to evade detection.
- Example: The emergence of generative adversarial networks (GANs) has introduced more realistic AI-generated images and videos, challenging detection software to keep up with these innovations.
- Lag in Detection Capabilities:
- Detection software often lags behind the latest AI developments, creating a window of vulnerability where new AI-generated content may go undetected.
- Example: Shortly after new AI-generated content techniques are released, it can take months for detection software to be updated to identify these new patterns.
- AI’s Capacity for Innovation:
2. High False Positives and False Negatives
AI detection software is not infallible, and its operation is often plagued by high rates of false positives and false negatives, which can undermine its effectiveness.
- False Positives:
- Misidentification of Human-Created Content:
- The software may incorrectly flag human-created content as AI-generated, leading to false positives that can have serious consequences.
- Example: In academic settings, a student’s original essay might be wrongly flagged as AI-generated, potentially leading to unfair penalties or accusations of plagiarism.
- Impact on Trust and Credibility:
- High rates of false positives can erode trust in AI detection software, leading users to question its reliability.
- Example: A news outlet may hesitate to use AI detection tools if they consistently flag legitimate content, fearing it could damage the outlet’s credibility.
- Misidentification of Human-Created Content:
- False Negatives:
- Failure to Detect AI-Generated Content:
- The software may fail to identify AI-generated content, leading to false negatives that allow harmful or misleading content to go undetected.
- Example: AI-generated deepfake videos that slip through detection filters could be used to spread misinformation, causing significant harm before they are identified.
- Challenges in Complex Scenarios:
- False negatives are particularly problematic in complex scenarios where the consequences of missed detection are severe.
- Example: In cybersecurity, failure to detect AI-driven malware could result in a significant data breach, compromising sensitive information and causing financial losses.
- Failure to Detect AI-Generated Content:
3. Computational Complexity and Resource Intensity
The sophisticated nature of AI detection software requires significant computational resources, which can limit its accessibility and scalability.
- High Computational Requirements:
- Resource-Intensive Processes:
- AI detection involves complex algorithms and large datasets, requiring substantial computational power to operate effectively.
- Example: Running deep learning models to detect AI-generated content in real-time can be resource-intensive, necessitating powerful servers and significant energy consumption.
- Limitations for Small Organizations:
- Smaller organizations with limited resources may struggle to implement AI detection software due to the high costs associated with the necessary hardware and software.
- Example: A small business might find it challenging to afford the infrastructure needed to run AI detection tools, limiting their ability to protect against AI-driven threats.
- Resource-Intensive Processes:
- Scalability Issues:
- Challenges in Large-Scale Deployments:
- Scaling AI detection software across large networks or platforms can be challenging due to the increased computational load and data processing requirements.
- Example: A global social media platform may encounter difficulties in scaling AI detection tools to monitor billions of posts daily, leading to gaps in coverage and potential undetected content.
- Balancing Speed and Accuracy:
- There is often a trade-off between the speed of detection and the accuracy of the results, with faster detection potentially leading to more errors.
- Example: In real-time content moderation, AI detection software may prioritize speed over accuracy, resulting in a higher rate of false positives or negatives.
- Challenges in Large-Scale Deployments:
4. Adversarial Attacks and AI Evasion Techniques
Adversarial attacks, where AI-generated content is specifically designed to evade detection, pose a significant threat to the effectiveness of AI detection software.
- Adversarial Examples:
- Crafting Content to Evade Detection:
- Attackers can create adversarial examples—AI-generated content that is intentionally designed to bypass detection algorithms.
- Example: In cybersecurity, adversaries might craft malware that exploits specific weaknesses in AI detection algorithms, allowing it to remain undetected by traditional security measures.
- Challenges in Robust Detection:
- Adversarial attacks highlight the difficulty of developing robust detection models that can adapt to evolving threats.
- Example: AI detection software used in deepfake detection might struggle to identify videos where adversarial techniques have been employed to obscure telltale signs of manipulation.
- Crafting Content to Evade Detection:
- AI Evasion Techniques:
- Continuous Evolution of Evasion Methods:
- As AI detection methods improve, so too do the techniques used by adversaries to evade them, creating a constant arms race between detection and evasion.
- Example: Hackers may use techniques such as subtle pixel manipulation in images to fool AI detection tools, bypassing security filters without raising alarms.
- Limitations in Defensive Capabilities:
- Detection software often has limited capabilities to defend against sophisticated evasion techniques, making it an ongoing challenge to stay ahead of attackers.
- Example: AI detection tools that rely on pattern recognition may be less effective against AI-generated content that uses randomized patterns to evade detection.
- Continuous Evolution of Evasion Methods:
5. Ethical and Privacy Concerns
The use of AI detection software raises ethical and privacy concerns that must be carefully managed to avoid unintended consequences.
- Infringement on Privacy:
- Monitoring and Data Collection:
- AI detection software often requires extensive data collection and monitoring, which can infringe on users’ privacy rights.
- Example: In corporate environments, AI detection tools that monitor employee communications for AI-generated content might also collect sensitive personal information, leading to potential privacy violations.
- Balancing Security and Privacy:
- There is a delicate balance between ensuring security and protecting privacy, with AI detection software sometimes tipping the scale towards invasive practices.
- Example: AI detection software used in social media moderation might monitor private messages for AI-generated content, raising concerns about user surveillance.
- Monitoring and Data Collection:
- Ethical Implications of AI Detection:
- Bias and Fairness Issues:
- AI detection software can exhibit biases in its detection processes, potentially leading to unfair outcomes for certain groups or individuals.
- Example: If AI detection tools are trained on biased datasets, they might disproportionately flag content from specific demographic groups as AI-generated, leading to discriminatory practices.
- Impact on Freedom of Expression:
- The widespread use of AI detection software in content moderation can impact freedom of expression, particularly if legitimate content is mistakenly flagged and removed.
- Example: Activists and journalists might find their content wrongly censored by AI detection tools that misinterpret their work as AI-generated, stifling important voices.
- Bias and Fairness Issues:
6. Dependency on Large Datasets and Training
AI detection software relies heavily on large datasets for training, which can introduce challenges related to data availability, quality, and diversity.
- Data Availability and Quality:
- Access to High-Quality Data:
- Effective AI detection requires access to large, high-quality datasets that accurately represent both AI-generated and human-created content.
- Example: A cybersecurity firm might struggle to obtain enough high-quality examples of AI-driven attacks to train its detection models effectively.
- Challenges with Data Labeling:
- Properly labeling datasets for training is time-consuming and prone to errors, which can affect the accuracy of the detection software.
- Example: If a dataset used to train AI detection software includes mislabeled content, the resulting model may struggle to correctly identify AI-generated material.
- Access to High-Quality Data:
- Diversity of Training Data:
- Need for Diverse Datasets:
- To be effective across different contexts and applications, AI detection software requires diverse training datasets that cover a wide range of content types and languages.
- Example: AI detection tools trained predominantly on English-language content may perform poorly when analyzing content in other languages, leading to gaps in detection capabilities.
- Risk of Overfitting:
- Overfitting occurs when AI detection models are too closely tailored to their training data, reducing their ability to generalize to new or unseen content.
- Example: An AI detection model trained on a specific type of deepfake video might fail to detect new deepfake techniques that were not represented in the training dataset.
- Need for Diverse Datasets:
The challenges and limitations of AI detection software highlight the complexities involved in developing and deploying these tools effectively.
While AI detection software offers significant potential in safeguarding digital environments, it must continuously evolve to overcome the hurdles posed by advancing AI technologies, computational demands, ethical considerations, and the need for high-quality training data.
Addressing these challenges is crucial to ensuring that AI detection software remains a reliable and effective tool in the fight against AI-generated threats and content.
6. The Future of AI Detection Software
The future of AI detection software is poised to evolve rapidly as both artificial intelligence and the need for detection technologies continue to grow.
With advancements in AI capabilities, the development of more sophisticated detection tools is essential to stay ahead of emerging threats and challenges.
This section explores the potential future trends, innovations, and directions for AI detection software, providing insights into how this technology may shape industries and society in the coming years.
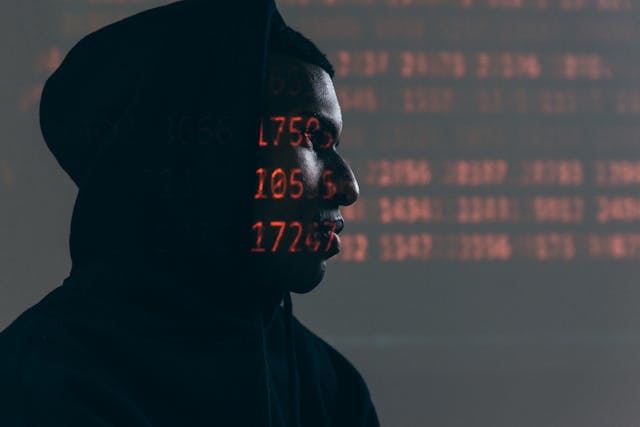
1. Advancements in Detection Algorithms
The future of AI detection software will likely see significant improvements in the underlying algorithms, enabling more accurate and efficient detection of AI-generated content.
- Enhanced Machine Learning Models:
- Use of Deep Learning Techniques:
- Future AI detection software is expected to leverage more advanced deep learning models that can better understand and analyze complex patterns in AI-generated content.
- Example: Improved convolutional neural networks (CNNs) could be used to detect deepfake images and videos with higher accuracy by recognizing subtle discrepancies in pixel arrangements and lighting.
- Incorporation of Transfer Learning:
- Transfer learning, where models pre-trained on one task are adapted to another, could be used to improve AI detection across different content types and languages.
- Example: An AI detection model trained on English text could be adapted to identify AI-generated content in other languages with minimal retraining, enhancing its versatility.
- Use of Deep Learning Techniques:
- Real-Time Detection Capabilities:
- Faster and More Efficient Algorithms:
- As computational power increases, future AI detection software will likely offer real-time detection capabilities, allowing for instantaneous identification of AI-generated content.
- Example: Real-time detection could be crucial in social media platforms where AI-generated content needs to be identified and flagged before it spreads widely.
- Improved Scalability:
- Future developments may focus on making AI detection algorithms more scalable, enabling them to handle larger volumes of data without compromising speed or accuracy.
- Example: A global e-commerce platform could deploy scalable AI detection software to monitor millions of product listings for AI-generated reviews or descriptions.
- Faster and More Efficient Algorithms:
2. Integration with Other Technologies
The future of AI detection software will likely involve greater integration with other technologies, enhancing its capabilities and expanding its applications across different sectors.
- Integration with Blockchain:
- Ensuring Content Authenticity:
- Combining AI detection with blockchain technology could create a more robust system for verifying the authenticity of digital content by storing and tracking content creation and modification history on an immutable ledger.
- Example: A media company could use blockchain-integrated AI detection software to verify that news articles have not been altered or manipulated after publication.
- Tracking AI-Generated Content:
- Blockchain could be used to track the origins of AI-generated content, ensuring that any detected AI activity is documented and traceable.
- Example: In creative industries, blockchain could be used to ensure that AI-generated art or music is properly attributed and not passed off as human-created.
- Ensuring Content Authenticity:
- Collaboration with Cybersecurity Tools:
- Enhancing Threat Detection:
- AI detection software could be integrated with existing cybersecurity tools to provide a comprehensive defense against AI-driven threats such as phishing, malware, and network intrusions.
- Example: A cybersecurity suite could incorporate AI detection capabilities to identify and neutralize AI-generated emails or messages designed to breach network security.
- Automated Response Systems:
- Future AI detection software could be linked with automated response systems that take immediate action when AI-generated threats are detected, reducing response times and minimizing damage.
- Example: An automated security system could instantly block or quarantine suspicious content flagged by AI detection software, preventing it from reaching users.
- Enhancing Threat Detection:
3. Ethical AI Detection and Bias Mitigation
As AI detection software becomes more prevalent, addressing ethical concerns and mitigating biases will be critical to ensuring its responsible use.
- Developing Fair and Unbiased Detection Models:
- Diverse and Representative Training Data:
- The future of AI detection will likely involve efforts to create more fair and unbiased models by using diverse and representative training datasets that encompass a wide range of content types and demographics.
- Example: AI detection tools used in content moderation could be trained on datasets that include content from different cultural backgrounds, reducing the risk of bias against specific groups.
- Ethical AI Practices:
- AI detection software developers may increasingly focus on incorporating ethical AI practices, ensuring that detection algorithms do not unfairly target or censor specific content or users.
- Example: Social media platforms could adopt AI detection tools that are regularly audited for fairness and transparency, ensuring that all users are treated equally.
- Diverse and Representative Training Data:
- Transparent and Explainable AI Detection:
- Improving Transparency in Decision-Making:
- Future AI detection software may include more transparent and explainable AI features, allowing users to understand how and why certain content was flagged as AI-generated.
- Example: An AI detection tool used in academic settings could provide detailed explanations for why a student’s work was flagged, helping to avoid misunderstandings and ensuring fair evaluation.
- User Control and Customization:
- Users may be given more control over how AI detection software operates, with customizable settings that allow them to adjust detection sensitivity and preferences.
- Example: A corporate IT department could configure AI detection tools to prioritize certain types of content or threats, tailoring the software to the company’s specific needs.
- Improving Transparency in Decision-Making:
4. Expansion of AI Detection Applications
As AI continues to permeate various industries, the applications of AI detection software will expand, with new use cases emerging across different sectors.
- Healthcare and Medical Research:
- Detecting AI-Generated Medical Content:
- AI detection software could play a growing role in healthcare by identifying AI-generated content in medical research, clinical trials, and patient records, ensuring the integrity and accuracy of medical data.
- Example: A research institution could use AI detection tools to verify that clinical trial data has not been manipulated by AI, safeguarding the validity of research findings.
- Preventing AI-Driven Healthcare Fraud:
- The software could be used to detect AI-driven fraud in healthcare, such as falsified medical records or fraudulent billing practices.
- Example: Insurance companies could deploy AI detection software to identify suspicious claims generated by AI, reducing the risk of healthcare fraud.
- Detecting AI-Generated Medical Content:
- Legal and Compliance Monitoring:
- Ensuring Legal Document Integrity:
- AI detection software could be increasingly used in the legal sector to verify the authenticity of legal documents, contracts, and evidence, preventing the use of AI-generated forgeries.
- Example: Law firms could use AI detection tools to ensure that all submitted legal documents are original and free from AI-generated tampering, protecting the integrity of legal proceedings.
- Compliance with AI Regulations:
- As regulations around AI usage become more stringent, AI detection software could help organizations comply by monitoring for unauthorized or unethical AI-generated content.
- Example: A financial institution could use AI detection software to ensure that all automated trading activities comply with new AI regulations, avoiding potential fines or legal action.
- Ensuring Legal Document Integrity:
5. AI Detection in Emerging Technologies
The future of AI detection will also be shaped by its application in emerging technologies, where new challenges and opportunities will arise.
- AI Detection in Autonomous Systems:
- Monitoring AI Behavior in Autonomous Vehicles:
- AI detection software could be used to monitor the behavior of AI systems in autonomous vehicles, ensuring that decisions are not influenced by unauthorized AI-generated inputs or manipulations.
- Example: An autonomous vehicle manufacturer could use AI detection tools to verify that the AI systems controlling the vehicle are operating based on accurate and unaltered data, improving safety and reliability.
- Ensuring the Safety of AI-Powered Drones:
- The software could also be used to monitor AI-powered drones, detecting any AI-generated anomalies that could compromise their operation.
- Example: A logistics company using AI-powered drones for deliveries could deploy AI detection software to ensure that the drones are operating safely and according to predefined parameters.
- Monitoring AI Behavior in Autonomous Vehicles:
- AI Detection in Virtual and Augmented Reality:
- Identifying AI-Generated Content in VR/AR:
- AI detection software could be applied to virtual and augmented reality environments to identify and flag AI-generated content that could affect user experience or safety.
- Example: A gaming company could use AI detection tools to monitor VR environments for unauthorized AI-generated modifications, ensuring that players experience the intended game content without interference.
- Preventing AI-Driven Manipulation in Immersive Experiences:
- The software could help prevent AI-driven manipulations in immersive experiences, such as altered sensory inputs or misleading virtual environments.
- Example: In training simulations, AI detection software could ensure that the virtual environment remains accurate and consistent, preventing AI-driven changes that could compromise the effectiveness of the training.
- Identifying AI-Generated Content in VR/AR:
6. Global Collaboration and Standardization
The future of AI detection software will likely involve greater global collaboration and the development of standardized practices to address AI-generated content on a global scale.
- International Cooperation on AI Detection:
- Cross-Border Collaboration:
- As AI-generated content becomes a global issue, there may be increased collaboration between countries and organizations to develop unified AI detection standards and practices.
- Example: International bodies such as the United Nations could spearhead efforts to create global guidelines for AI detection software, promoting consistency and cooperation across borders.
- Sharing Best Practices:
- Organizations and governments may share best practices and technologies related to AI detection, fostering a collaborative approach to tackling AI-driven threats.
- Example: A consortium of tech companies could collaborate on developing open-source AI detection tools, making them available to smaller organizations that might lack the resources to develop their own.
- Cross-Border Collaboration:
- Standardization of AI Detection Technologies:
- Development of Industry Standards:
- The creation of industry-wide standards for AI detection software could help ensure that all organizations have access to effective and reliable detection tools.
- Example: The financial industry could establish standardized protocols for AI detection, ensuring that all institutions are equipped to detect and prevent AI-driven financial fraud.
- Certification and Compliance Programs:
- Future AI detection software may be subject to certification and compliance programs that verify its effectiveness and adherence to ethical standards.
- Example: AI detection tools used in government agencies could undergo regular certification to ensure they meet stringent security and privacy requirements.
- Development of Industry Standards:
The future of AI detection software is bright and full of potential, with significant advancements and innovations expected to shape the way this technology is developed and deployed.
From improved algorithms and integration with other technologies to addressing ethical concerns and expanding applications, AI detection software will continue to evolve to meet the growing challenges posed by AI-generated content.
As this technology progresses, it will play an increasingly important role in safeguarding digital environments, protecting users, and ensuring the responsible use of artificial intelligence across various sectors.
Conclusion
As we navigate an era increasingly influenced by artificial intelligence, the role of AI detection software has become crucial in safeguarding the integrity and trustworthiness of digital content.
The evolution of AI technology has brought about remarkable innovations, but it has also introduced complex challenges, particularly in the form of AI-generated content that can be difficult to discern from human-created material.
From deepfake videos and synthetic text to AI-driven cyberattacks, the potential for misuse of AI is vast, making the need for effective detection tools more pressing than ever.
AI detection software stands as a critical defense mechanism in this landscape, providing organizations, governments, and individuals with the tools necessary to identify, analyze, and mitigate the impact of AI-generated content.
By understanding how this software works, the underlying technologies it employs, and its wide-ranging applications, we can better appreciate its importance in maintaining the authenticity of digital interactions and information.
The Expanding Scope of AI Detection Software
As AI continues to permeate various sectors, the scope of AI detection software is expected to expand correspondingly.
Initially, AI detection tools were primarily focused on identifying fake news or deepfake media, but their applications have since broadened to encompass a wide array of industries and use cases.
- In Cybersecurity:
- AI detection software plays a vital role in identifying AI-driven cyber threats such as phishing schemes, malware, and unauthorized access attempts. By detecting these threats in real-time, organizations can proactively protect their networks and data from sophisticated attacks.
- In Media and Content Verification:
- As the prevalence of AI-generated content increases, the media industry has turned to AI detection tools to ensure the authenticity of news articles, videos, and images. This helps in preserving the credibility of information disseminated to the public.
- In Academic Integrity:
- Educational institutions are adopting AI detection software to safeguard academic integrity by detecting AI-generated essays and research papers. This ensures that academic work remains original and credible.
- In E-commerce and Digital Marketing:
- AI detection tools are used to monitor and verify the authenticity of online reviews, product descriptions, and advertisements, preventing the spread of misleading or fraudulent content.
These examples highlight the diverse applications of AI detection software and underscore its growing importance in maintaining trust and security across digital platforms.
Challenges and Ongoing Developments
Despite its critical role, AI detection software faces several challenges that must be addressed to enhance its effectiveness. The rapid advancement of AI technologies means that detection tools must constantly evolve to keep pace with new threats and techniques. Some of the key challenges include:
- Evolving AI Techniques:
- As AI-generated content becomes more sophisticated, detection algorithms must be continuously updated to recognize new patterns and anomalies. The rise of more advanced AI models, such as generative adversarial networks (GANs), presents a significant challenge for detection tools.
- Data Quality and Availability:
- The effectiveness of AI detection software heavily depends on the quality and diversity of training data. Access to high-quality datasets that accurately represent various forms of AI-generated content is essential for developing reliable detection algorithms.
- Ethical Considerations:
- The deployment of AI detection software raises ethical questions, particularly regarding privacy, bias, and the potential for misuse. Developers and users must ensure that these tools are designed and implemented in a way that respects ethical standards and minimizes harm.
- Computational Demands:
- AI detection software often requires significant computational resources to operate effectively, particularly when analyzing large volumes of data in real-time. Ensuring that these tools are both efficient and scalable is a critical challenge for developers.
Addressing these challenges will require collaboration between researchers, developers, and industry stakeholders. By investing in research and development, enhancing data sharing, and establishing ethical guidelines, the AI detection field can continue to advance and meet the demands of an ever-changing digital landscape.
The Future Outlook: Staying Ahead of AI-Driven Threats
Looking ahead, the future of AI detection software is poised for significant growth and innovation. As AI technology continues to advance, so too will the methods used to detect and mitigate its potential misuse. Key areas of focus for the future include:
- Integration with Other Technologies:
- The integration of AI detection software with other emerging technologies, such as blockchain and IoT, could enhance its capabilities and provide more comprehensive solutions for detecting and managing AI-generated content.
- Improvement in Real-Time Detection:
- Real-time detection will become increasingly important, particularly in high-stakes environments like cybersecurity and social media. Future advancements in algorithms and computational power will likely lead to more efficient and immediate detection capabilities.
- Global Collaboration and Standardization:
- As the impact of AI-generated content becomes a global concern, there will be a growing need for international cooperation and standardization of AI detection practices. Establishing global guidelines and best practices will be essential for effectively combating AI-driven threats.
- Ethical AI and Bias Mitigation:
- The development of fair and unbiased AI detection models will be crucial to ensuring that these tools are used responsibly. Future advancements in ethical AI practices will help address concerns related to bias, transparency, and user privacy.
In conclusion, AI detection software is an indispensable tool in the modern digital landscape, providing critical protection against the misuse of artificial intelligence.
As the technology behind AI continues to evolve, so too must the tools designed to detect and mitigate its potential threats.
By staying ahead of these challenges and investing in the development of robust, ethical, and effective detection solutions, we can ensure that AI continues to be a force for good, while minimizing its potential for harm.
The ongoing advancement of AI detection software will play a vital role in maintaining the integrity, security, and trustworthiness of our digital world, shaping a future where AI and human creativity coexist in harmony.
If your company needs HR, hiring, or corporate services, you can use 9cv9 hiring and recruitment services. Book a consultation slot here, or send over an email to [email protected].
If you find this article useful, why not share it with your hiring manager and C-level suite friends and also leave a nice comment below?
We, at the 9cv9 Research Team, strive to bring the latest and most meaningful data, guides, and statistics to your doorstep.
To get access to top-quality guides, click over to 9cv9 Blog.
People Also Ask
What is AI detection software?
AI detection software identifies and analyzes AI-generated content, such as deepfakes, synthetic text, and manipulated media, to ensure the integrity of digital information.
How does AI detection software work?
AI detection software uses machine learning, pattern recognition, and algorithms to analyze data and detect anomalies indicative of AI-generated content.
Why is AI detection software important?
AI detection software is crucial for safeguarding digital environments by identifying and mitigating AI-generated content that could mislead, deceive, or cause harm.
What types of content can AI detection software identify?
AI detection software can detect deepfakes, synthetic text, manipulated images, AI-driven videos, and other forms of AI-generated content.
Can AI detection software identify deepfakes?
Yes, AI detection software is specifically designed to identify deepfakes by analyzing inconsistencies in facial movements, lighting, and audio that may indicate manipulation.
Is AI detection software used in cybersecurity?
Yes, AI detection software plays a critical role in cybersecurity by detecting AI-driven threats such as phishing emails, malware, and unauthorized access attempts.
How accurate is AI detection software?
The accuracy of AI detection software varies based on the complexity of the AI-generated content and the sophistication of the detection algorithms used.
Can AI detection software be fooled?
While AI detection software is highly effective, advanced AI-generated content can sometimes bypass detection, especially if the content mimics human patterns closely.
What industries benefit from AI detection software?
Industries like cybersecurity, media, education, finance, and e-commerce benefit from AI detection software by protecting against AI-driven fraud, misinformation, and manipulation.
How does AI detection software contribute to content verification?
AI detection software ensures content authenticity by identifying and flagging AI-generated or manipulated material, helping maintain trust in digital media.
Is AI detection software used in social media platforms?
Yes, social media platforms use AI detection software to monitor and remove AI-generated content, such as deepfake videos or fake news, to protect users.
What are the challenges of AI detection software?
Challenges include staying ahead of evolving AI techniques, managing computational demands, ensuring unbiased detection, and maintaining data quality.
Can AI detection software operate in real-time?
Yes, many AI detection tools are designed for real-time analysis, enabling immediate identification and mitigation of AI-generated threats.
How does AI detection software handle large volumes of data?
AI detection software uses scalable algorithms and cloud computing to process and analyze large data sets efficiently, ensuring timely detection of AI-generated content.
What role does machine learning play in AI detection software?
Machine learning is central to AI detection software, allowing it to learn from data patterns and improve its ability to detect new and sophisticated AI-generated content.
How do organizations implement AI detection software?
Organizations integrate AI detection software into their digital infrastructure to monitor, analyze, and protect against AI-generated threats in real-time.
What is the future of AI detection software?
The future of AI detection software includes advancements in real-time detection, integration with other technologies, and improved algorithms to counteract evolving AI threats.
How does AI detection software address ethical concerns?
AI detection software developers focus on creating fair and unbiased detection models, using diverse training data, and implementing ethical AI practices to minimize harm.
Can AI detection software be customized?
Yes, AI detection software can often be customized to meet the specific needs of an organization, including adjusting detection sensitivity and integrating with existing systems.
Is AI detection software essential for businesses?
For businesses operating in digital environments, AI detection software is essential for protecting against AI-generated fraud, misinformation, and security threats.
How do AI detection tools enhance cybersecurity?
AI detection tools enhance cybersecurity by identifying and mitigating AI-driven attacks, such as phishing schemes and malware, before they can cause harm.
What are the limitations of AI detection software?
Limitations include potential biases in detection, the need for continuous updates to keep pace with evolving AI, and the significant computational resources required.
How does AI detection software ensure data integrity?
AI detection software ensures data integrity by identifying and flagging AI-generated or manipulated content, preventing the spread of false information.
What is the impact of AI detection software on digital media?
AI detection software helps maintain the credibility of digital media by ensuring that published content is authentic and free from AI-generated manipulation.
How do AI detection tools combat misinformation?
AI detection tools combat misinformation by identifying AI-generated content, such as fake news articles or doctored images, and flagging or removing them from digital platforms.
Can AI detection software be integrated with other technologies?
Yes, AI detection software can be integrated with technologies like blockchain and cybersecurity tools to enhance content verification and digital security.
What are the ethical implications of AI detection software?
Ethical implications include ensuring that detection algorithms are unbiased, protecting user privacy, and preventing misuse of the software in censorship or surveillance.
How does AI detection software handle AI-generated text?
AI detection software analyzes linguistic patterns, context, and anomalies in text to identify and flag AI-generated content, such as automated essays or fake reviews.
What advancements are expected in AI detection software?
Advancements include more sophisticated algorithms, better integration with other digital tools, real-time detection capabilities, and improved handling of large-scale data.
How do AI detection tools support academic integrity?
AI detection tools support academic integrity by identifying AI-generated essays, research papers, or exam responses, ensuring that academic work remains original and credible.