Key Takeaways
- Automate IT Operations with AI: AIOps tools leverage artificial intelligence and machine learning to automate routine IT tasks, enhancing efficiency and reducing manual intervention.
- Proactive Issue Resolution: These tools predict and prevent potential IT issues before they impact operations, ensuring continuous performance and minimizing downtime.
- Advanced Analytics and Insights: AIOps tools provide real-time data analytics and actionable insights, empowering IT teams to make informed decisions and optimize IT infrastructure management.
In today’s fast-paced digital landscape, the role of IT operations has become increasingly critical as businesses strive to maintain seamless and efficient services.
The complexity of modern IT environments, characterized by a diverse array of technologies, platforms, and infrastructures, presents significant challenges for IT teams.
Traditional methods of managing and monitoring IT operations are no longer sufficient to keep up with the growing demands of real-time data processing, anomaly detection, and system optimization.
This is where AIOps tools come into play—a revolutionary approach that leverages the power of artificial intelligence (AI) and machine learning to enhance IT operations.
AIOps, or Artificial Intelligence for IT Operations, represents a paradigm shift in how organizations manage their IT environments.
These tools are designed to analyze vast amounts of data generated by various IT systems and networks, identify patterns and anomalies, and automate routine tasks to improve overall efficiency.
AIOps tools are not just about automating mundane tasks; they are about providing intelligent insights and proactive solutions that enable IT teams to prevent issues before they arise and quickly resolve incidents when they do occur.
The adoption of AIOps tools is driven by the need to overcome the limitations of traditional IT operations management (ITOM) methods.
As IT infrastructures continue to evolve, becoming more distributed and hybrid in nature, the volume of data generated has skyrocketed.
Manual monitoring and analysis of this data are not only time-consuming but also prone to errors.
AIOps tools address this challenge by leveraging advanced algorithms and machine learning techniques to process and analyze data at scale, enabling organizations to achieve higher levels of automation, accuracy, and efficiency.
Moreover, AIOps tools play a crucial role in bridging the gap between IT operations and other business functions. By providing real-time insights into the health and performance of IT systems, these tools empower businesses to make data-driven decisions that align with their strategic goals.
For example, in the retail industry, AIOps tools can be used to optimize inventory management and enhance customer experiences by ensuring that IT systems are running smoothly and efficiently. In the financial sector, AIOps tools can help detect fraudulent activities and ensure the security of transactions.
One of the most significant advantages of AIOps tools is their ability to continuously learn and adapt to changing environments.
As these tools process more data and encounter different scenarios, they become more intelligent and capable of making more accurate predictions and decisions.
This continuous learning process enables AIOps tools to stay ahead of potential issues, making them an indispensable asset for organizations that want to maintain high levels of service availability and performance.
As we delve deeper into the world of AIOps tools, this blog will explore the fundamental concepts behind these innovative solutions, how they work, and the various benefits they offer to businesses.
We will also examine the challenges and considerations associated with implementing AIOps tools, as well as the future trends that are likely to shape their evolution.
Whether you are an IT professional looking to enhance your organization’s operational efficiency or a business leader seeking to stay ahead in a competitive market, understanding AIOps tools and their capabilities is essential for success in today’s digital age.
Before we venture further into this article, we would like to share who we are and what we do.
About 9cv9
9cv9 is a business tech startup based in Singapore and Asia, with a strong presence all over the world.
With over eight years of startup and business experience, and being highly involved in connecting with thousands of companies and startups, the 9cv9 team has listed some important learning points in this overview of What are AIOps Tools and How Do They Work.
If your company needs recruitment and headhunting services to hire top-quality employees, you can use 9cv9 headhunting and recruitment services to hire top talents and candidates. Find out more here, or send over an email to [email protected].
Or just post 1 free job posting here at 9cv9 Hiring Portal in under 10 minutes.
What are AIOps Tools and How Do They Work
- What are AIOps Tools?
- How Do AIOps Tools Work?
- Benefits of Using AIOps Tools
- Challenges and Considerations in Implementing AIOps Tools
- Use Cases of AIOps Tools in Different Industries
- Future Trends in AIOps Tools
1. What are AIOps Tools?
AIOps (Artificial Intelligence for IT Operations) tools represent a transformative leap in the way organizations manage their IT environments.
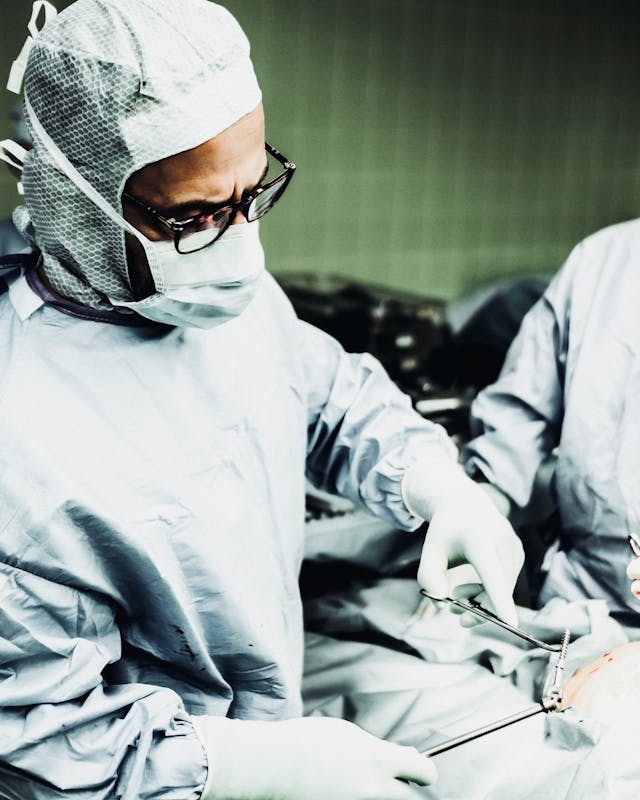
These tools combine the power of artificial intelligence (AI), machine learning (ML), and big data analytics to automate and enhance IT operations.
By analyzing large volumes of data from various sources, AIOps tools enable proactive monitoring, faster issue resolution, and improved operational efficiency.
Below, we break down the key components, features, and examples of AIOps tools.
Definition and Core Concept
- AIOps Tools Defined:
- AIOps tools are software solutions that use AI and ML to automate and optimize IT operations.
- They analyze data from diverse IT environments to detect patterns, predict issues, and automate responses.
- The primary goal is to improve the efficiency, reliability, and performance of IT operations.
- Core Components:
- Machine Learning Algorithms:
- Enable the identification of patterns and anomalies in IT data.
- Continuously learn and adapt to changing IT environments.
- Big Data Analytics:
- Process and analyze vast amounts of data from different sources.
- Provide real-time insights and actionable intelligence.
- Automation Capabilities:
- Automate routine IT tasks such as monitoring, alerting, and incident management.
- Reduce the need for manual intervention, minimizing human error.
- Machine Learning Algorithms:
Key Features of AIOps Tools
- Data Aggregation and Correlation:
- Unified Data Collection:
- Gather data from various sources, including logs, events, metrics, and traces.
- Integrate data from multiple IT environments, such as on-premises, cloud, and hybrid systems.
- Correlation of Data:
- Correlate related events and metrics to identify root causes of issues.
- Reduce alert noise by grouping related alerts into a single incident.
- Unified Data Collection:
- Real-Time Monitoring and Analysis:
- Continuous Monitoring:
- Monitor IT systems in real-time for anomalies and performance issues.
- Enable early detection of potential problems before they impact users.
- Anomaly Detection:
- Use ML algorithms to detect unusual patterns in data that may indicate issues.
- Automatically generate alerts for detected anomalies, allowing for quick action.
- Continuous Monitoring:
- Automated Incident Response:
- Proactive Remediation:
- Automatically trigger remediation actions based on predefined rules and patterns.
- Examples include restarting a failed service, reallocating resources, or applying a configuration change.
- Incident Management:
- Integrate with IT service management (ITSM) tools for seamless incident tracking and resolution.
- Provide detailed incident reports with root cause analysis and remediation actions.
- Proactive Remediation:
- Predictive Analytics and Forecasting:
- Predictive Maintenance:
- Predict potential failures and performance degradation before they occur.
- Schedule maintenance activities proactively to avoid disruptions.
- Capacity Planning:
- Analyze usage trends to forecast future resource needs.
- Optimize resource allocation to meet growing demand.
- Predictive Maintenance:
Examples of AIOps Tools in Action
- Dynatrace:
- Overview:
- A popular AIOps platform that offers full-stack monitoring and AI-powered analytics.
- Capable of monitoring applications, infrastructure, and user experience in real-time.
- Key Features:
- Automatically detects and diagnoses performance issues across the entire IT stack.
- Provides precise root cause analysis and automated remediation.
- Use Case:
- Retail Industry:
- Used by e-commerce companies to ensure website performance during peak shopping seasons.
- Monitors user transactions in real-time, detecting and resolving issues that could lead to cart abandonment.
- Retail Industry:
- Overview:
- Splunk IT Service Intelligence (ITSI):
- Overview:
- A comprehensive AIOps solution that leverages machine learning for IT monitoring and incident management.
- Provides end-to-end visibility into IT services and their performance.
- Key Features:
- Analyzes machine data to provide insights into system health and performance.
- Automates incident response and reduces alert fatigue through event correlation.
- Use Case:
- Financial Services:
- Used by banks to monitor transaction processing systems.
- Detects anomalies in transaction volumes or processing times, ensuring smooth operations.
- Financial Services:
- Overview:
- Moogsoft:
- Overview:
- A leading AIOps platform focused on reducing IT noise and accelerating incident resolution.
- Uses AI to correlate events and reduce the volume of alerts IT teams need to address.
- Key Features:
- Automatically identifies root causes of incidents through event correlation.
- Provides a unified view of incidents across the IT environment.
- Use Case:
- Telecommunications:
- Used by telecom companies to monitor network performance.
- Identifies and resolves issues that could lead to service disruptions, ensuring high availability.
- Telecommunications:
- Overview:
The Evolution and Importance of AIOps Tools
- Evolution from Traditional ITOM:
- Traditional IT Operations Management (ITOM):
- Relied heavily on manual monitoring and reactive incident management.
- Often struggled with scalability and efficiency in large, complex environments.
- Transition to AIOps:
- Emerged as a response to the growing complexity and scale of modern IT environments.
- Combines automation, AI, and data analytics to overcome the limitations of traditional ITOM.
- Traditional IT Operations Management (ITOM):
- Importance in Modern IT Environments:
- Scalability:
- AIOps tools are essential for managing the scale and complexity of modern IT infrastructures.
- Capable of handling the vast amount of data generated by distributed and hybrid environments.
- Proactive Operations:
- Shift IT operations from a reactive to a proactive approach.
- Reduce downtime and improve service availability by addressing issues before they impact users.
- Enhanced Decision-Making:
- Provide IT teams with data-driven insights to make informed decisions.
- Support strategic initiatives such as digital transformation and cloud migration.
- Scalability:
AIOps tools are rapidly becoming an indispensable part of modern IT operations.
By leveraging AI, machine learning, and big data analytics, these tools empower organizations to manage their IT environments more effectively, ensuring higher levels of service reliability, performance, and scalability.
As we continue to witness the digital transformation of industries across the globe, the role of AIOps tools will only become more critical in driving operational excellence and business success.
2. How Do AIOps Tools Work?
AIOps tools operate by integrating artificial intelligence (AI), machine learning (ML), and big data analytics to transform the way IT operations are managed.
These tools streamline complex processes, automate routine tasks, and provide predictive insights that allow IT teams to stay ahead of potential issues.
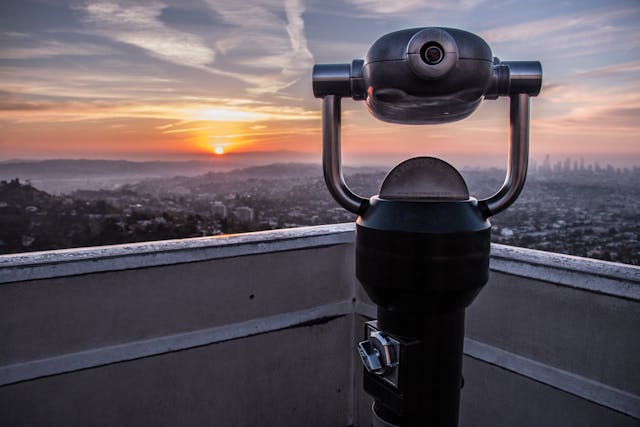
Understanding the mechanisms behind AIOps tools is crucial for appreciating how they revolutionize IT operations.
This section delves into the core functionalities of AIOps tools, breaking down their processes into manageable sub-sections.
Data Collection and Ingestion
- Unified Data Aggregation:
- Multi-Source Data Collection:
- AIOps tools collect data from a wide range of sources, including logs, events, metrics, traces, and alerts.
- These sources can span across various IT environments such as on-premises data centers, cloud infrastructures, and hybrid setups.
- Real-Time Data Ingestion:
- Data is ingested in real-time, ensuring that the AIOps tools have up-to-date information to analyze.
- Continuous data ingestion allows for timely detection of anomalies and potential issues.
- Example:
- Splunk ITSI: Aggregates data from different systems, providing a holistic view of the IT environment. This enables real-time monitoring and swift identification of anomalies.
- Multi-Source Data Collection:
Data Normalization and Correlation
- Data Normalization:
- Standardizing Data Formats:
- AIOps tools normalize the collected data, converting it into a consistent format that can be easily analyzed.
- Normalization is crucial for accurate correlation and analysis across different data types and sources.
- Contextual Enrichment:
- Enriching data with contextual information, such as the source, timestamp, and relevant metadata.
- Helps in creating a comprehensive picture of the IT environment for deeper analysis.
- Standardizing Data Formats:
- Event Correlation:
- Cross-Source Correlation:
- Correlate events across multiple data sources to identify relationships and patterns.
- Reduces alert noise by grouping related events into a single incident, making it easier for IT teams to focus on critical issues.
- Root Cause Identification:
- AIOps tools use advanced correlation techniques to pinpoint the root cause of incidents.
- Identifying the root cause allows for faster resolution and minimizes the impact on operations.
- Example:
- Moogsoft: Employs AI-driven event correlation to reduce alert fatigue by filtering out redundant alerts and focusing on the most significant incidents. This improves the efficiency of incident management.
- Cross-Source Correlation:
Machine Learning and Anomaly Detection
- Machine Learning Models:
- Pattern Recognition:
- AIOps tools use machine learning algorithms to recognize patterns in the normalized data.
- These patterns help in understanding normal operational behavior and identifying deviations that may indicate problems.
- Self-Learning Algorithms:
- Machine learning models continuously learn and adapt based on new data, improving their accuracy over time.
- The self-learning capability allows AIOps tools to evolve with the IT environment, enhancing their predictive accuracy.
- Example:
- Dynatrace: Uses AI-driven pattern recognition to automatically detect anomalies in application performance. The tool learns from historical data to predict future issues, allowing IT teams to take preemptive actions.
- Pattern Recognition:
- Anomaly Detection:
- Real-Time Anomaly Identification:
- AIOps tools monitor data streams in real-time to identify anomalies that deviate from established patterns.
- Anomalies can include unexpected spikes in CPU usage, network latency, or application errors.
- Alert Generation:
- Upon detecting an anomaly, AIOps tools generate alerts and notifications to inform IT teams of potential issues.
- Alerts are prioritized based on severity and impact, ensuring that critical issues are addressed promptly.
- Example:
- AppDynamics: Detects anomalies in application performance by analyzing transaction data and comparing it to normal behavior. This proactive approach helps in preventing downtime and improving user experience.
- Real-Time Anomaly Identification:
Automation and Remediation
- Automated Incident Response:
- Proactive Remediation:
- AIOps tools can automatically trigger remediation actions based on predefined rules and patterns.
- Common automated responses include restarting services, reallocating resources, or applying configuration changes.
- Integration with ITSM Tools:
- Integrate with IT Service Management (ITSM) tools to create, update, and close incident tickets automatically.
- This seamless integration streamlines the incident management process, reducing manual intervention.
- Example:
- ServiceNow with AIOps Integration: Automatically creates incident tickets based on detected anomalies and triggers remediation workflows, significantly reducing the time to resolution.
- Proactive Remediation:
- Automation of Routine Tasks:
- Automated Monitoring:
- Continuous monitoring of IT systems and applications to ensure optimal performance.
- Automatically adjusts monitoring parameters based on changing conditions in the IT environment.
- Predictive Maintenance:
- Predicts potential failures before they occur, allowing for scheduled maintenance that minimizes downtime.
- Automates maintenance tasks such as patch management and system updates.
- Example:
- PagerDuty: Automates incident response by integrating with various monitoring tools and triggering automated workflows. This reduces the need for manual intervention and speeds up incident resolution.
- Automated Monitoring:
Predictive Analytics and Decision-Making
- Predictive Insights:
- Trend Analysis:
- Analyze historical data to identify trends that could impact future operations.
- Predictive insights help IT teams anticipate issues and take proactive measures to prevent them.
- Capacity Planning:
- Use predictive analytics to forecast resource needs and optimize capacity planning.
- Ensure that IT infrastructure can scale to meet future demands without compromising performance.
- Example:
- IBM Watson AIOps: Leverages predictive analytics to forecast potential system failures and recommend actions to prevent them. This proactive approach enhances system reliability and reduces unplanned downtime.
- Trend Analysis:
- Data-Driven Decision-Making:
- Real-Time Dashboards:
- AIOps tools provide real-time dashboards that visualize key performance indicators (KPIs) and system health metrics.
- These dashboards offer actionable insights, enabling IT teams to make informed decisions quickly.
- Strategic Planning:
- Use insights from AIOps tools to inform strategic planning, such as cloud migration or digital transformation initiatives.
- Data-driven decision-making ensures that IT strategies align with business objectives.
- Example:
- New Relic: Provides real-time dashboards that offer deep insights into application and infrastructure performance. These insights support data-driven decision-making, helping businesses optimize their IT operations.
- Real-Time Dashboards:
Continuous Learning and Improvement
- Feedback Loops:
- Self-Improving Models:
- AIOps tools incorporate feedback loops where outcomes of automated actions are analyzed and fed back into the system.
- This continuous learning process allows AIOps tools to refine their models and improve their accuracy over time.
- Adapting to Changes:
- As IT environments evolve, AIOps tools adapt to new conditions by learning from new data and experiences.
- This adaptability ensures that AIOps tools remain effective even in dynamic and complex IT environments.
- Example:
- Elastic APM: Continuously learns from application performance data and user feedback to improve anomaly detection and reduce false positives. This ensures that the tool remains relevant and effective in diverse IT environments.
- Self-Improving Models:
AIOps tools work by integrating AI, machine learning, and big data analytics to automate and optimize IT operations. From data collection and normalization to predictive analytics and continuous learning, these tools enable IT teams to manage complex environments more effectively. By leveraging AIOps tools, organizations can achieve higher levels of efficiency, reliability, and performance, ensuring that their IT operations are aligned with business goals and capable of supporting future growth.
3. Benefits of Using AIOps Tools
The adoption of AIOps (Artificial Intelligence for IT Operations) tools is rapidly increasing across industries due to the numerous benefits they offer.
By leveraging AI and machine learning, AIOps tools provide a competitive edge in managing complex IT environments.
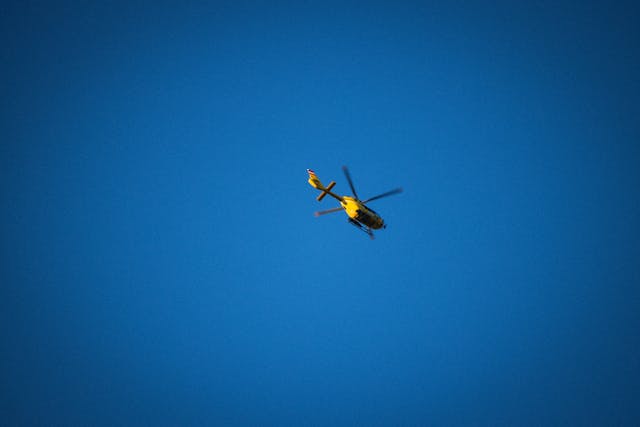
This section explores the key benefits of using AIOps tools, highlighting how they enhance efficiency, reduce costs, and improve overall IT operations.
Enhanced Efficiency and Productivity
- Automation of Repetitive Tasks:
- AIOps tools automate routine IT tasks such as monitoring, incident detection, and response, freeing up IT staff to focus on more strategic activities.
- Automated processes reduce human errors and ensure consistent execution of tasks.
- Example:
- Splunk ITSI automates the correlation of events and alerts, enabling IT teams to quickly identify and address issues without manual intervention.
- Faster Incident Resolution:
- AIOps tools use machine learning algorithms to quickly identify the root cause of incidents, significantly reducing the time required for troubleshooting.
- Automated remediation actions can be triggered instantly, minimizing downtime and service disruptions.
- Example:
- Moogsoft uses AI-driven event correlation and noise reduction to streamline incident management, allowing IT teams to resolve issues faster and more efficiently.
- Improved Resource Allocation:
- By analyzing data patterns and trends, AIOps tools help organizations optimize resource allocation, ensuring that IT resources are used efficiently.
- Proactive management of resources reduces the risk of over-provisioning or under-provisioning, leading to cost savings.
- Example:
- Dynatrace provides insights into application performance and resource usage, enabling IT teams to allocate resources more effectively and avoid bottlenecks.
Proactive Issue Prevention
- Predictive Analytics for Early Detection:
- AIOps tools use predictive analytics to detect potential issues before they escalate into major incidents.
- Early detection allows IT teams to take preventive measures, reducing the likelihood of service disruptions.
- Example:
- IBM Watson AIOps leverages predictive analytics to forecast potential system failures and recommends preemptive actions to mitigate risks.
- Anomaly Detection and Prevention:
- AIOps tools continuously monitor IT environments for anomalies that deviate from normal behavior.
- Detecting and addressing anomalies early prevents them from developing into critical issues.
- Example:
- AppDynamics uses AI to detect performance anomalies in real-time, enabling IT teams to prevent issues that could impact user experience.
- Reduced Alert Fatigue:
- AIOps tools filter out redundant alerts and focus on the most critical events, reducing the number of alerts that IT teams need to manage.
- By prioritizing alerts based on severity, AIOps tools help prevent alert fatigue, ensuring that IT staff can respond effectively to important incidents.
- Example:
- Moogsoft employs AI-driven noise reduction techniques to minimize alert fatigue, allowing IT teams to concentrate on high-priority incidents.
Cost Savings
- Optimized IT Operations:
- Automation and predictive analytics reduce the need for manual intervention, leading to significant cost savings in IT operations.
- AIOps tools help organizations achieve more with fewer resources, optimizing operational efficiency and reducing labor costs.
- Example:
- PagerDuty automates incident response workflows, reducing the need for extensive manual monitoring and intervention, which translates into lower operational costs.
- Reduced Downtime and Service Disruptions:
- By preventing incidents and resolving them quickly when they occur, AIOps tools minimize downtime, reducing the financial impact of service disruptions.
- Lower downtime also improves customer satisfaction and protects revenue streams.
- Example:
- New Relic helps organizations monitor their IT infrastructure in real-time, ensuring rapid incident detection and minimizing downtime, which leads to cost savings.
- Efficient Resource Utilization:
- AIOps tools optimize the use of IT resources, preventing over-provisioning, and reducing unnecessary expenditures.
- Efficient resource management helps organizations save on infrastructure costs and avoid wastage.
- Example:
- Dynatrace provides detailed insights into resource usage, helping organizations optimize their infrastructure and reduce costs associated with over-provisioning.
Improved Decision-Making
- Data-Driven Insights:
- AIOps tools analyze vast amounts of data and provide actionable insights that inform decision-making processes.
- Real-time dashboards and reports enable IT leaders to make informed decisions based on current and historical data.
- Example:
- Elastic APM offers real-time monitoring and analytics, providing IT teams with insights that drive data-driven decision-making and strategic planning.
- Strategic Planning and Forecasting:
- Predictive analytics and trend analysis provided by AIOps tools support strategic planning initiatives, such as capacity planning, cloud migration, and digital transformation.
- Data-driven forecasting ensures that IT strategies align with business goals and are prepared for future challenges.
- Example:
- IBM Watson AIOps helps organizations forecast future IT needs, supporting strategic initiatives like cloud migration by providing data-driven insights.
- Enhanced Collaboration and Communication:
- AIOps tools facilitate collaboration between IT teams by providing a centralized platform for monitoring, incident management, and reporting.
- Enhanced communication and collaboration lead to more effective incident resolution and better overall IT performance.
- Example:
- ServiceNow with AIOps Integration: Streamlines communication between IT teams by integrating incident management with AIOps tools, improving collaboration and speeding up issue resolution.
Scalability and Flexibility
- Adaptability to Complex Environments:
- AIOps tools are designed to scale with the growing needs of an organization, making them suitable for complex and dynamic IT environments.
- They can handle increasing amounts of data and integrate with a wide range of systems, ensuring that they remain effective as organizations grow.
- Example:
- Splunk ITSI scales with enterprise needs, capable of handling large volumes of data from diverse sources while maintaining high levels of performance.
- Support for Hybrid and Multi-Cloud Environments:
- AIOps tools are flexible enough to manage IT operations across on-premises, cloud, and hybrid environments, providing consistent monitoring and management.
- This flexibility is essential for organizations adopting multi-cloud strategies, ensuring seamless IT operations across different platforms.
- Example:
- Dynatrace offers full-stack monitoring and AIOps capabilities for hybrid and multi-cloud environments, ensuring consistent performance across diverse infrastructures.
- Continuous Improvement and Learning:
- AIOps tools incorporate continuous learning mechanisms, adapting to changing environments and improving their accuracy and effectiveness over time.
- This adaptability ensures that AIOps tools remain relevant and provide value as IT environments evolve.
- Example:
- Elastic APM continuously learns from application performance data and user feedback, improving its anomaly detection capabilities and reducing false positives.
The benefits of using AIOps tools are numerous and significant, offering organizations a powerful way to enhance IT operations, reduce costs, and improve decision-making.
By automating routine tasks, preventing issues before they arise, and providing data-driven insights, AIOps tools help organizations stay competitive in a rapidly evolving digital landscape.
Whether managing complex hybrid environments or optimizing resource allocation, AIOps tools are essential for modern IT operations.
4. Challenges and Considerations in Implementing AIOps Tools
While AIOps tools offer numerous benefits, their implementation comes with its own set of challenges and considerations.
Organizations must carefully plan and execute their AIOps strategies to ensure successful adoption and maximize the value of these tools.
This section explores the key challenges in implementing AIOps tools and provides insights into the considerations that can help mitigate these challenges.
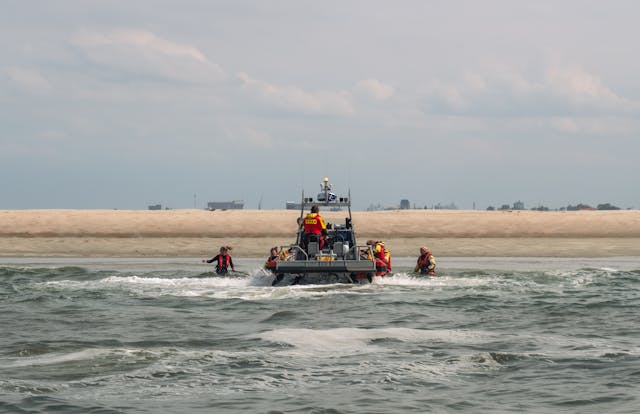
1. Data Management and Integration Challenges
- Data Silos:
- AIOps tools require access to large volumes of data from various sources across the IT environment. However, data often exists in silos, making it difficult to integrate and analyze.
- Breaking down these silos and ensuring seamless data flow is crucial for the success of AIOps implementations.
- Example:
- A large financial institution might face challenges in integrating data from legacy systems, cloud platforms, and third-party applications, hindering the effectiveness of their AIOps tool.
- Data Quality and Consistency:
- The effectiveness of AIOps tools heavily relies on the quality and consistency of the data they process. Inconsistent, incomplete, or outdated data can lead to inaccurate insights and decisions.
- Organizations need to establish robust data governance practices to ensure that data fed into AIOps tools is accurate and reliable.
- Example:
- An e-commerce company might struggle with inconsistent data across different customer interaction channels, leading to inaccurate predictions and recommendations from their AIOps tool.
- Complexity in Data Integration:
- Integrating data from diverse sources, such as on-premises systems, cloud environments, and IoT devices, can be complex and time-consuming.
- AIOps tools must be capable of handling heterogeneous data formats and integrating seamlessly with existing IT infrastructure.
- Example:
- A manufacturing company implementing AIOps tools might face difficulties in integrating data from factory floor sensors, enterprise resource planning (ERP) systems, and cloud-based analytics platforms.
2. High Implementation Costs
- Initial Investment:
- Implementing AIOps tools requires a significant initial investment in software licenses, hardware, and infrastructure upgrades.
- Organizations need to carefully evaluate the cost-benefit ratio and ensure that the expected ROI justifies the expenditure.
- Example:
- A mid-sized enterprise may hesitate to adopt AIOps tools due to the high upfront costs associated with acquiring and deploying the necessary technology stack.
- Ongoing Operational Costs:
- Beyond the initial investment, organizations must consider the ongoing operational costs, including maintenance, support, and scaling the AIOps environment as data volumes grow.
- These costs can accumulate over time, impacting the overall budget and financial planning.
- Example:
- A retail company might find that the ongoing costs of maintaining and scaling their AIOps solution exceed their initial budget estimates, leading to financial strain.
- Resource Allocation:
- Implementing AIOps tools requires allocating resources such as skilled personnel, time, and capital. Organizations must balance these demands with other ongoing IT projects and initiatives.
- Effective resource management is crucial to avoid overstretching the IT team and budget.
- Example:
- An IT service provider might struggle with allocating sufficient resources to implement AIOps tools while maintaining day-to-day operations and other critical projects.
3. Skills and Expertise Gaps
- Need for Specialized Skills:
- AIOps tools require specialized skills in AI, machine learning, data science, and IT operations. Many organizations lack the in-house expertise to effectively implement and manage these tools.
- Investing in training and hiring skilled personnel is essential for successful AIOps adoption.
- Example:
- A healthcare organization might face difficulties in finding and hiring data scientists and AI experts to implement and manage their AIOps solution, delaying the project.
- Complexity in Tool Configuration and Management:
- Configuring and managing AIOps tools can be complex, especially for organizations new to AI and machine learning technologies.
- Misconfigurations can lead to suboptimal performance, inaccurate insights, and missed opportunities for automation.
- Example:
- A telecommunications company might struggle with configuring their AIOps tool to effectively monitor and manage their complex network infrastructure, resulting in missed alerts and delayed incident response.
- Cultural Resistance and Change Management:
- The introduction of AIOps tools often requires a cultural shift within the IT organization. Resistance to change can hinder the adoption and effective use of these tools.
- Organizations must invest in change management strategies to foster a culture of innovation and acceptance of AI-driven solutions.
- Example:
- An established financial services firm might face resistance from IT staff who are accustomed to traditional tools and processes, slowing down the adoption of AIOps solutions.
4. Security and Compliance Concerns
- Data Privacy and Security:
- AIOps tools process large volumes of sensitive data, raising concerns about data privacy and security. Ensuring that these tools comply with data protection regulations is critical.
- Organizations need to implement robust security measures, such as encryption, access controls, and monitoring, to protect data.
- Example:
- A healthcare provider implementing AIOps tools must ensure compliance with HIPAA regulations, safeguarding patient data while leveraging AI for IT operations.
- Regulatory Compliance:
- Different industries are subject to various regulatory requirements, which AIOps tools must adhere to. Non-compliance can result in legal and financial penalties.
- Organizations need to ensure that their AIOps tools are configured to meet industry-specific regulatory standards.
- Example:
- A financial institution implementing AIOps tools must comply with regulations such as GDPR and PCI-DSS, ensuring that customer data is processed securely and in accordance with legal requirements.
- Risk of Data Breaches:
- The centralization of data in AIOps tools can increase the risk of data breaches. A single point of failure can lead to widespread exposure of sensitive information.
- Implementing robust security frameworks and conducting regular audits are necessary to mitigate these risks.
- Example:
- A multinational corporation might face heightened risks of data breaches as their AIOps tool integrates data from various global operations, requiring stringent security measures to protect their IT environment.
5. Complexity of Change Management
- Organizational Resistance:
- The shift from traditional IT operations to AI-driven processes can be met with resistance from employees accustomed to legacy systems.
- Overcoming this resistance requires effective communication, training, and demonstration of the tangible benefits of AIOps tools.
- Example:
- A government agency might encounter resistance from its IT staff, who may be reluctant to adopt AIOps tools due to concerns about job displacement or the complexity of new systems.
- Need for Clear Strategy and Roadmap:
- Implementing AIOps tools without a clear strategy and roadmap can lead to confusion, misalignment of goals, and failure to achieve desired outcomes.
- Organizations need to define clear objectives, milestones, and success metrics to guide the implementation process.
- Example:
- A manufacturing company might struggle with AIOps implementation due to a lack of a well-defined strategy, leading to delays and unmet expectations.
- Continuous Monitoring and Adaptation:
- AIOps tools are not a one-time implementation; they require continuous monitoring, adaptation, and optimization to remain effective.
- Organizations must be prepared to regularly update their AIOps tools and processes to keep pace with changing IT environments.
- Example:
- An online retail platform must continuously monitor and adjust their AIOps tool to adapt to changing customer behavior, new technology integrations, and evolving market demands.
Implementing AIOps tools presents a variety of challenges that organizations must carefully navigate to realize their full potential.
From managing data integration and addressing security concerns to overcoming cultural resistance and skill gaps, these considerations are critical to ensuring a successful AIOps deployment.
By proactively addressing these challenges and planning with a clear strategy, organizations can unlock the transformative benefits of AIOps tools, driving efficiency, improving decision-making, and maintaining a competitive edge in an increasingly complex digital landscape.
5. Use Cases of AIOps Tools in Different Industries
AIOps (Artificial Intelligence for IT Operations) tools have emerged as powerful solutions for managing and optimizing IT operations across various industries.
By leveraging AI and machine learning, AIOps tools automate complex processes, predict potential issues, and enhance overall operational efficiency.
In this section, we will explore the diverse use cases of AIOps tools in different industries, providing relevant examples to illustrate their impact.
1. Financial Services
- Fraud Detection and Prevention
- AIOps tools can analyze vast amounts of transaction data in real time, identifying patterns indicative of fraudulent activity.
- By automating the detection process, these tools help financial institutions prevent fraud before it causes significant damage.
- Example:
- A large bank uses an AIOps tool to monitor millions of daily transactions. The tool detects anomalies that suggest credit card fraud, enabling the bank to take immediate action and minimize losses.
- Predictive Maintenance for ATMs
- Financial institutions rely on AIOps tools to predict and prevent ATM failures, ensuring continuous service availability.
- These tools analyze historical data to identify patterns that precede failures, allowing proactive maintenance.
- Example:
- A global financial institution implements AIOps to monitor its network of ATMs. The tool predicts hardware malfunctions, scheduling maintenance before issues occur, reducing downtime and improving customer satisfaction.
- Regulatory Compliance
- AIOps tools help financial institutions maintain compliance with regulatory requirements by automating the monitoring and reporting of data.
- These tools ensure that all transactions, communications, and operations adhere to industry regulations.
- Example:
- A multinational bank uses AIOps to automatically monitor compliance with GDPR. The tool flags any potential data breaches or non-compliant activities, allowing the bank to address issues promptly.
2. Healthcare
- Patient Data Management
- AIOps tools streamline the management of patient data, ensuring that healthcare providers have access to accurate and up-to-date information.
- These tools can analyze data from multiple sources, such as electronic health records (EHRs), wearable devices, and lab results.
- Example:
- A hospital network employs AIOps to integrate and analyze patient data from various departments. The tool ensures that doctors have real-time access to comprehensive patient records, improving diagnosis and treatment.
- Predictive Analytics for Patient Care
- AIOps tools use predictive analytics to anticipate patient needs, identify high-risk patients, and suggest proactive care measures.
- These insights help healthcare providers improve patient outcomes and reduce hospital readmissions.
- Example:
- A healthcare provider uses AIOps to analyze patient data and predict which patients are at risk of readmission. The tool recommends personalized care plans, leading to better patient outcomes and lower readmission rates.
- Optimizing IT Infrastructure
- In healthcare, AIOps tools optimize IT infrastructure, ensuring that critical systems, such as EHRs and medical imaging platforms, are always available.
- These tools detect potential issues and trigger automated responses to prevent downtime.
- Example:
- A large hospital system uses AIOps to monitor its IT infrastructure. The tool predicts server overloads and automatically adjusts resource allocation, preventing system crashes during peak times.
3. Retail
- Inventory Management
- Retailers use AIOps tools to optimize inventory management by predicting demand, managing stock levels, and reducing waste.
- These tools analyze sales trends, seasonal factors, and customer behavior to make accurate inventory predictions.
- Example:
- A major retailer implements AIOps to forecast product demand during holiday seasons. The tool helps the retailer maintain optimal inventory levels, reducing overstock and stockouts.
- Personalized Customer Experiences
- AIOps tools enable retailers to deliver personalized shopping experiences by analyzing customer data, such as purchase history and browsing behavior.
- These insights allow retailers to tailor promotions, product recommendations, and marketing campaigns to individual customers.
- Example:
- An e-commerce platform uses AIOps to analyze customer behavior in real time. The tool recommends products based on browsing history and previous purchases, increasing conversion rates and customer satisfaction.
- Supply Chain Optimization
- AIOps tools optimize the supply chain by predicting disruptions, managing logistics, and ensuring timely delivery of products.
- These tools help retailers minimize delays and reduce costs associated with supply chain inefficiencies.
- Example:
- A global retail chain employs AIOps to monitor its supply chain. The tool predicts potential delays due to weather conditions and adjusts shipping routes, ensuring on-time delivery.
4. Manufacturing
- Predictive Maintenance
- In manufacturing, AIOps tools are used to predict equipment failures and schedule maintenance before breakdowns occur.
- These tools analyze sensor data from machines to identify early signs of wear and tear, preventing costly downtime.
- Example:
- A manufacturing plant uses AIOps to monitor machinery performance. The tool predicts when a critical machine is likely to fail and schedules maintenance, reducing unplanned downtime and maintenance costs.
- Quality Control
- AIOps tools enhance quality control by analyzing production data in real time, identifying defects, and ensuring products meet standards.
- These tools help manufacturers reduce waste and improve product quality.
- Example:
- An automotive manufacturer implements AIOps to monitor the production line. The tool detects anomalies in real time, flagging potential defects before they impact the final product.
- Supply Chain Resilience
- Manufacturers use AIOps tools to strengthen supply chain resilience by predicting disruptions, managing supplier relationships, and optimizing logistics.
- These tools ensure that production schedules are maintained, even in the face of external challenges.
- Example:
- A global electronics manufacturer employs AIOps to monitor its supply chain. The tool predicts delays due to supplier issues and suggests alternative sourcing options, ensuring uninterrupted production.
5. Telecommunications
- Network Performance Monitoring
- AIOps tools are used in telecommunications to monitor network performance, detect anomalies, and optimize bandwidth usage.
- These tools ensure that networks operate efficiently, reducing downtime and improving customer experience.
- Example:
- A telecom provider uses AIOps to monitor its 5G network. The tool detects potential network congestion and automatically reallocates resources to maintain optimal performance.
- Customer Service Automation
- Telecommunications companies use AIOps tools to automate customer service processes, such as troubleshooting and ticket resolution.
- These tools analyze customer interactions to identify common issues and suggest automated responses.
- Example:
- A telecom company implements AIOps to enhance its customer service chatbot. The tool analyzes past interactions to improve the chatbot’s accuracy in resolving common customer issues.
- Predictive Maintenance of Infrastructure
- AIOps tools predict maintenance needs for telecom infrastructure, such as cell towers and data centers, ensuring continuous service availability.
- These tools analyze data from infrastructure components to identify potential failures.
- Example:
- A telecom operator uses AIOps to monitor its data centers. The tool predicts when cooling systems are likely to fail and schedules maintenance, preventing costly outages.
6. Energy and Utilities
- Smart Grid Management
- AIOps tools play a crucial role in managing smart grids by optimizing energy distribution, predicting outages, and improving grid resilience.
- These tools analyze data from sensors across the grid to ensure efficient energy management.
- Example:
- A utility company uses AIOps to monitor its smart grid. The tool predicts potential power outages due to equipment failures and adjusts energy distribution to minimize impact on customers.
- Predictive Maintenance of Infrastructure
- Energy companies use AIOps tools to predict maintenance needs for critical infrastructure, such as power plants and transmission lines.
- These tools prevent unexpected failures and reduce maintenance costs.
- Example:
- An energy provider implements AIOps to monitor its power plant equipment. The tool predicts when turbines are likely to require maintenance, allowing the company to plan and minimize downtime.
- Energy Consumption Optimization
- AIOps tools help utilities optimize energy consumption by analyzing usage patterns, predicting demand, and suggesting energy-saving measures.
- These tools support sustainability initiatives and reduce operational costs.
- Example:
- A utility company uses AIOps to analyze customer energy usage patterns. The tool recommends energy-saving measures to customers, helping them reduce their bills and supporting the company’s sustainability goals.
AIOps tools are revolutionizing industries by automating and optimizing IT operations, improving efficiency, and enabling proactive decision-making.
From financial services to manufacturing, telecommunications to energy, the use cases of AIOps tools are diverse and impactful.
As industries continue to embrace digital transformation, the role of AIOps will only grow, driving innovation and competitive advantage across the global economy.
6. Future Trends in AIOps Tools
As technology advances, AIOps (Artificial Intelligence for IT Operations) tools are poised to undergo significant transformations.
These tools, which blend AI and machine learning to enhance IT operations, will continue to evolve, offering even greater capabilities and integration across various industries.
This section explores the future trends in AIOps tools, highlighting the directions in which this technology is heading and providing relevant examples to illustrate these trends.
1. Enhanced Predictive Capabilities
- More Accurate Predictive Analytics
- Future AIOps tools will offer even more accurate predictive analytics by leveraging larger datasets and advanced machine learning algorithms.
- These tools will be able to predict issues with greater precision, enabling IT teams to address potential problems before they impact operations.
- Example:
- A healthcare provider may use an advanced AIOps tool that predicts equipment failures with 99% accuracy, allowing for timely maintenance and minimizing downtime.
- Predictive Incident Management
- AIOps tools will increasingly focus on predicting incidents across IT environments, allowing teams to prevent disruptions before they occur.
- This trend will see AIOps tools integrating with incident management systems to automate the resolution process.
- Example:
- A financial institution could deploy an AIOps tool that predicts network outages during peak transaction periods, triggering automatic rerouting of traffic to prevent service disruptions.
2. Integration with Cloud and Edge Computing
- Seamless Cloud Integration
- Future AIOps tools will offer deeper integration with cloud platforms, enabling seamless monitoring and management of cloud-native applications.
- These tools will help organizations optimize their cloud resources, reduce costs, and ensure continuous availability.
- Example:
- A retail company using AIOps could achieve real-time monitoring and optimization of its cloud-based e-commerce platform, ensuring high performance during flash sales.
- Edge Computing and AIOps
- The rise of edge computing will drive the development of AIOps tools that can operate at the edge, providing real-time insights and automation closer to data sources.
- These tools will be crucial for industries that rely on edge devices, such as IoT and autonomous systems.
- Example:
- An energy company might use an AIOps tool at the edge to monitor and optimize the performance of smart meters and grid components in real-time.
3. Autonomous IT Operations
- Self-Healing IT Systems
- AIOps tools will advance toward fully autonomous IT operations, where systems can automatically detect, diagnose, and resolve issues without human intervention.
- This trend will lead to the development of self-healing IT systems that continuously monitor and optimize themselves.
- Example:
- A global logistics company could deploy an AIOps tool that automatically detects and fixes server configuration errors, ensuring uninterrupted service for its tracking systems.
- AI-Driven Decision Making
- Future AIOps tools will increasingly make autonomous decisions based on real-time data, reducing the need for manual intervention.
- These tools will analyze complex scenarios and recommend or implement actions to maintain optimal operations.
- Example:
- In a manufacturing setting, an AIOps tool might autonomously decide to shut down a production line temporarily to avoid overheating, based on real-time sensor data.
4. Greater Focus on Security
- AI-Driven Cybersecurity
- As cyber threats become more sophisticated, AIOps tools will integrate advanced security features, leveraging AI to detect and respond to threats in real-time.
- These tools will help organizations protect their IT environments from increasingly complex cyber attacks.
- Example:
- A government agency could use an AIOps tool to monitor its network for unusual activity, automatically triggering a security protocol when a potential breach is detected.
- Proactive Threat Mitigation
- Future AIOps tools will not only detect threats but will also predict and prevent them by analyzing patterns and identifying vulnerabilities before they are exploited.
- This proactive approach will significantly reduce the risk of cyber incidents.
- Example:
- A financial services firm could deploy an AIOps tool that predicts potential phishing attacks by analyzing communication patterns, allowing the company to warn employees before the attacks occur.
5. Advanced Data Analytics and Visualization
- Enhanced Data Correlation
- AIOps tools will improve their ability to correlate data across multiple sources, providing a more comprehensive view of IT operations.
- These tools will enable organizations to uncover hidden insights and trends, leading to better decision-making.
- Example:
- A telecommunications company could use an AIOps tool to correlate data from customer service logs and network performance metrics, identifying issues that impact customer satisfaction.
- Real-Time Visualization Dashboards
- Future AIOps tools will feature more advanced visualization capabilities, offering real-time dashboards that provide actionable insights at a glance.
- These dashboards will be customizable, allowing IT teams to monitor the metrics that matter most to their operations.
- Example:
- An airline might use an AIOps tool with a real-time dashboard to monitor its booking system, displaying key performance indicators such as transaction speed and error rates.
6. Personalized and Context-Aware AIOps
- Contextual AI Insights
- AIOps tools will evolve to provide context-aware insights, tailoring their recommendations based on the specific needs and conditions of the organization.
- These tools will consider factors such as business goals, industry regulations, and operational constraints when making recommendations.
- Example:
- A pharmaceutical company might use an AIOps tool that considers regulatory requirements when suggesting optimizations for its R&D data management systems.
- Personalized User Experiences
- Future AIOps tools will offer personalized experiences for different user roles within an organization, ensuring that each user receives the most relevant insights and recommendations.
- These tools will adapt their interfaces and outputs based on the user’s responsibilities and expertise.
- Example:
- In a large enterprise, an IT manager might see high-level trends and alerts in the AIOps dashboard, while a network engineer receives detailed technical insights relevant to their role.
7. Collaboration and Communication Features
- Integrated Collaboration Tools
- AIOps tools will increasingly integrate with collaboration platforms, enabling IT teams to communicate and coordinate more effectively within the same environment.
- These integrations will streamline incident management and enhance team collaboration.
- Example:
- An IT department might use an AIOps tool that integrates with Slack or Microsoft Teams, allowing team members to discuss alerts and share insights directly within their communication channels.
- Automated Incident Reporting
- Future AIOps tools will automate the reporting process, generating detailed incident reports that can be shared with stakeholders and used for post-incident analysis.
- These reports will include actionable insights and recommendations for preventing similar issues in the future.
- Example:
- A technology company could use an AIOps tool to automatically generate a report after a system outage, providing a summary of the incident, the root cause, and recommended preventive measures.
8. Increased Adoption of Open-Source AIOps
- Growth of Open-Source AIOps Solutions
- The open-source movement will play a significant role in the future of AIOps, with more organizations adopting open-source AIOps tools for their flexibility and cost-effectiveness.
- These tools will benefit from community-driven innovation, leading to rapid advancements in features and capabilities.
- Example:
- A startup might adopt an open-source AIOps tool that allows for customization to fit its unique IT environment, benefiting from continuous updates and improvements contributed by the community.
- Interoperability and Integration
- Open-source AIOps tools will offer greater interoperability with other IT systems and tools, enabling seamless integration across diverse IT environments.
- This trend will encourage organizations to mix and match tools that best meet their needs, rather than relying on a single vendor.
- Example:
- An enterprise might use an open-source AIOps tool that integrates with its existing IT service management (ITSM) platform, providing a unified view of operations and incidents.
The future of AIOps tools is marked by innovation and transformation, driven by advances in AI, machine learning, and IT infrastructure.
As these tools evolve, they will offer enhanced predictive capabilities, deeper integration with cloud and edge computing, and more autonomous operations.
The focus on security, advanced analytics, and personalization will further solidify AIOps as a critical component of modern IT operations.
Organizations across various industries will continue to leverage these tools to stay ahead of the curve, ensuring efficiency, resilience, and competitive advantage in an increasingly complex digital landscape.
Conclusion
AIOps (Artificial Intelligence for IT Operations) tools represent a transformative shift in how organizations manage and optimize their IT infrastructure.
By integrating artificial intelligence, machine learning, and big data analytics, AIOps tools empower businesses to automate and enhance their IT operations, leading to more efficient, reliable, and proactive management of complex IT environments.
1. The Evolution and Importance of AIOps
- Addressing the Complexity of Modern IT Environments
- The modern IT landscape is increasingly complex, with diverse technologies, distributed systems, and vast amounts of data. Traditional IT operations tools often fall short in managing this complexity, leading to inefficiencies, downtime, and increased operational costs.
- AIOps tools have emerged as a solution to these challenges by automating routine tasks, providing real-time insights, and enabling predictive analytics. This evolution is not just a trend but a necessity for organizations aiming to maintain a competitive edge in the digital age.
- Significance in Today’s Digital Transformation
- As organizations continue to undergo digital transformation, the role of AIOps tools becomes even more critical. These tools facilitate the seamless integration of new technologies, ensuring that IT operations can keep pace with the rapid changes in the business environment.
- AIOps tools support continuous improvement by identifying patterns, predicting issues, and recommending solutions, which are vital for maintaining the agility and responsiveness needed in today’s fast-paced markets.
2. Key Benefits of AIOps Tools
- Enhanced Efficiency and Automation
- AIOps tools drastically reduce the manual effort required in IT operations by automating repetitive tasks such as monitoring, incident detection, and root cause analysis. This leads to significant time savings and allows IT teams to focus on strategic initiatives.
- The automation capabilities of AIOps tools also contribute to reducing human error, which is a common cause of downtime and performance issues. By ensuring more accurate and consistent IT operations, organizations can improve service quality and customer satisfaction.
- Proactive Issue Resolution
- One of the most significant advantages of AIOps tools is their ability to predict and prevent issues before they impact business operations. By analyzing historical data and identifying patterns, AIOps tools can foresee potential problems and trigger preventive measures.
- This proactive approach not only minimizes downtime but also helps in maintaining optimal performance levels, thereby supporting business continuity and reducing costs associated with unplanned outages.
- Improved Decision-Making and Insights
- AIOps tools provide IT teams with actionable insights derived from vast amounts of data collected across the IT environment. These insights enable better decision-making by highlighting areas for improvement and optimizing resource allocation.
- The advanced analytics capabilities of AIOps tools allow organizations to understand the underlying causes of recurring issues, streamline processes, and implement best practices that enhance overall operational efficiency.
3. Challenges and Considerations in Implementing AIOps Tools
- Complexity of Integration
- While AIOps tools offer numerous benefits, their implementation can be challenging, particularly in complex IT environments. Organizations must carefully plan the integration of AIOps tools with existing systems to avoid disruptions and ensure seamless operation.
- Consideration must also be given to the training and upskilling of IT staff to effectively use these tools. The shift to AIOps requires a change in mindset, as IT teams need to transition from reactive management to proactive, AI-driven operations.
- Data Quality and Management
- The effectiveness of AIOps tools is heavily dependent on the quality and availability of data. Organizations need to ensure that their data is accurate, complete, and accessible to maximize the benefits of AIOps.
- Managing large volumes of data, ensuring data privacy, and overcoming data silos are critical challenges that must be addressed to fully leverage the potential of AIOps tools.
4. Future Prospects of AIOps
- Advancements in AI and Machine Learning
- The future of AIOps is closely tied to advancements in AI and machine learning. As these technologies continue to evolve, AIOps tools will become more sophisticated, offering even greater predictive accuracy, automation capabilities, and integration with emerging technologies such as cloud and edge computing.
- Organizations can expect AIOps tools to become increasingly autonomous, capable of self-learning and self-optimizing, which will further reduce the need for manual intervention in IT operations.
- Broader Industry Adoption
- As the benefits of AIOps tools become more apparent, their adoption is expected to grow across various industries. From healthcare to finance, retail to manufacturing, AIOps tools will be instrumental in helping organizations manage their IT operations in a more efficient, reliable, and scalable manner.
- The rise of open-source AIOps solutions will also contribute to broader adoption, providing organizations with flexible, cost-effective options that can be tailored to their specific needs.
Final Thoughts
In conclusion, AIOps tools are not just a technological advancement; they are a fundamental shift in how IT operations are managed.
By harnessing the power of AI and machine learning, AIOps tools offer organizations the ability to automate processes, predict and prevent issues, and make data-driven decisions that enhance overall IT performance.
As these tools continue to evolve, they will play an increasingly vital role in helping organizations navigate the complexities of modern IT environments and achieve their digital transformation goals.
For organizations looking to stay ahead in a rapidly changing digital landscape, investing in AIOps tools is not just an option but a strategic imperative.
By embracing AIOps, businesses can ensure that their IT operations are not only efficient and reliable but also future-ready, capable of adapting to the challenges and opportunities of tomorrow.
If your company needs HR, hiring, or corporate services, you can use 9cv9 hiring and recruitment services. Book a consultation slot here, or send over an email to [email protected].
If you find this article useful, why not share it with your hiring manager and C-level suite friends and also leave a nice comment below?
We, at the 9cv9 Research Team, strive to bring the latest and most meaningful data, guides, and statistics to your doorstep.
To get access to top-quality guides, click over to 9cv9 Blog.
People Also Ask
What are AIOps tools?
AIOps tools are AI-driven solutions that enhance IT operations by automating tasks, analyzing data, and providing insights to manage complex IT environments more efficiently.
How do AIOps tools work?
AIOps tools use AI and machine learning to collect and analyze large volumes of IT data, automate incident management, predict issues, and provide actionable insights to improve IT operations.
What are the benefits of using AIOps tools?
AIOps tools offer benefits such as increased operational efficiency, proactive issue resolution, improved decision-making through advanced analytics, and reduced manual effort in IT management.
What industries can benefit from AIOps tools?
Industries like healthcare, finance, retail, and manufacturing can benefit from AIOps tools by optimizing IT operations, reducing downtime, and enhancing overall system performance.
How do AIOps tools improve IT efficiency?
AIOps tools improve IT efficiency by automating routine tasks, reducing manual intervention, providing real-time monitoring, and enabling proactive management of IT infrastructure.
Can AIOps tools predict IT issues?
Yes, AIOps tools can predict IT issues by analyzing historical data and identifying patterns that signal potential problems before they impact operations.
What is the role of machine learning in AIOps tools?
Machine learning in AIOps tools helps analyze large datasets, detect anomalies, automate tasks, and improve predictive accuracy for managing IT environments.
How do AIOps tools integrate with cloud services?
AIOps tools integrate with cloud services by providing real-time monitoring, optimizing resource usage, and ensuring seamless management of cloud-native applications and infrastructure.
Are AIOps tools suitable for small businesses?
Yes, AIOps tools are suitable for small businesses as they offer scalable solutions that improve IT efficiency and reduce the complexity of managing IT operations.
What are the challenges of implementing AIOps tools?
Challenges include integrating with existing systems, ensuring data quality, managing large volumes of data, and training staff to use the new tools effectively.
How do AIOps tools contribute to cybersecurity?
AIOps tools enhance cybersecurity by detecting unusual activity, predicting potential threats, and automating responses to mitigate risks and protect IT environments.
What features should you look for in AIOps tools?
Key features include real-time monitoring, automated incident management, predictive analytics, data correlation, and integration capabilities with existing IT systems.
How can AIOps tools help in incident management?
AIOps tools streamline incident management by automating detection, diagnosis, and resolution processes, reducing response times and improving overall efficiency.
What is the future of AIOps tools?
The future of AIOps tools involves advancements in AI and machine learning, greater integration with emerging technologies, and increased automation and predictive capabilities.
How do AIOps tools enhance decision-making?
AIOps tools enhance decision-making by providing real-time analytics, actionable insights, and data-driven recommendations that help IT teams make informed decisions.
Can AIOps tools be customized for specific needs?
Yes, many AIOps tools offer customization options to fit specific organizational needs, including integration with existing systems and tailoring features to match unique requirements.
How do AIOps tools handle data management?
AIOps tools manage data by collecting, analyzing, and correlating information from various sources, ensuring data accuracy and providing actionable insights for IT operations.
What are the key trends in AIOps tools?
Key trends include enhanced predictive capabilities, integration with cloud and edge computing, autonomous IT operations, and increased focus on security and advanced analytics.
Do AIOps tools require special training?
Yes, using AIOps tools effectively often requires training to ensure IT staff can leverage the full range of features and capabilities and adapt to the new workflows.
How do AIOps tools compare to traditional IT management tools?
AIOps tools offer more advanced automation, predictive analytics, and real-time insights compared to traditional IT management tools, leading to improved efficiency and reduced manual effort.
What are the advantages of cloud-based AIOps tools?
Cloud-based AIOps tools offer scalability, flexibility, cost-effectiveness, and ease of integration with cloud services, enabling efficient management of cloud-native applications.
How do AIOps tools support digital transformation?
AIOps tools support digital transformation by automating IT operations, providing real-time insights, and enabling organizations to adapt quickly to technological changes and business needs.
What role do AIOps tools play in proactive IT management?
AIOps tools play a crucial role in proactive IT management by predicting potential issues, automating resolutions, and ensuring continuous monitoring to prevent disruptions.
Can AIOps tools integrate with existing ITSM platforms?
Yes, many AIOps tools offer integration capabilities with existing IT Service Management (ITSM) platforms, providing a unified view of IT operations and incident management.
What is the impact of AIOps tools on IT costs?
AIOps tools can reduce IT costs by automating tasks, minimizing downtime, improving resource utilization, and decreasing the need for extensive manual intervention.
How do AIOps tools handle scalability?
AIOps tools handle scalability by providing flexible, scalable solutions that can adapt to growing data volumes and increasing IT complexity without compromising performance.
What is the significance of real-time monitoring in AIOps tools?
Real-time monitoring in AIOps tools is significant as it provides immediate visibility into IT operations, allowing for prompt detection and resolution of issues to maintain system performance.
How do AIOps tools improve operational resilience?
AIOps tools improve operational resilience by predicting and preventing issues, automating responses, and ensuring continuous monitoring, which helps maintain consistent IT performance.
Can AIOps tools be used for IT infrastructure optimization?
Yes, AIOps tools can optimize IT infrastructure by analyzing performance data, identifying inefficiencies, and providing recommendations for resource allocation and system improvements.
What types of data do AIOps tools analyze?
AIOps tools analyze various types of data, including system logs, performance metrics, network traffic, and application data, to provide comprehensive insights into IT operations.
How do AIOps tools support multi-cloud environments?
AIOps tools support multi-cloud environments by providing unified monitoring and management across different cloud platforms, ensuring consistent performance and operational efficiency.
What are the key challenges in adopting AIOps tools?
Key challenges include integrating with legacy systems, managing data quality, ensuring effective deployment, and addressing the learning curve for IT staff.
How do AIOps tools contribute to better IT service delivery?
AIOps tools contribute to better IT service delivery by automating incident response, providing actionable insights, and optimizing IT operations, leading to improved service quality and customer satisfaction.
What are the benefits of using AI in IT operations?
Using AI in IT operations offers benefits such as enhanced automation, predictive analytics, real-time insights, and improved decision-making, leading to more efficient and effective IT management.
How can AIOps tools enhance security operations?
AIOps tools enhance security operations by detecting anomalies, predicting potential threats, and automating responses to security incidents, improving overall protection of IT assets.
What is the role of data correlation in AIOps tools?
Data correlation in AIOps tools involves analyzing and linking data from various sources to identify patterns, root causes, and insights that improve IT operations and problem resolution.
How do AIOps tools handle incident resolution?
AIOps tools handle incident resolution by automating the detection, diagnosis, and remediation of issues, reducing response times and improving overall IT service continuity.
Can AIOps tools help with compliance management?
Yes, AIOps tools can assist with compliance management by providing visibility into IT operations, automating reporting, and ensuring adherence to regulatory requirements through continuous monitoring.
What are the emerging trends in AIOps technology?
Emerging trends in AIOps technology include advanced machine learning algorithms, increased integration with edge computing, enhanced security features, and more autonomous operations.