Key Takeaways
- Personalized recommendations drive significant business growth, with companies like Amazon and Netflix seeing major revenue boosts from their recommendation engines.
- Advanced technologies like AI, machine learning, and deep learning are transforming recommendation systems, delivering more accurate and relevant suggestions.
- Ethical considerations and data privacy will play a crucial role in the future development of recommendation engines, ensuring user trust and transparency.
In the era of digital personalization, recommendation engines have become the silent architects behind the most engaging user experiences across e-commerce, entertainment, social media, and online services.
Whether suggesting a product on Amazon, a movie on Netflix, or a song on Spotify, these sophisticated systems are designed to anticipate user preferences and deliver tailored content with impressive accuracy.
As personalization becomes the new norm, the role of recommendation engines continues to evolve rapidly—fueled by advancements in artificial intelligence (AI), machine learning (ML), and big data analytics.
Understanding the latest statistics, data points, and emerging trends in this field is essential for businesses, developers, marketers, and decision-makers aiming to enhance customer engagement and drive conversions through intelligent automation.
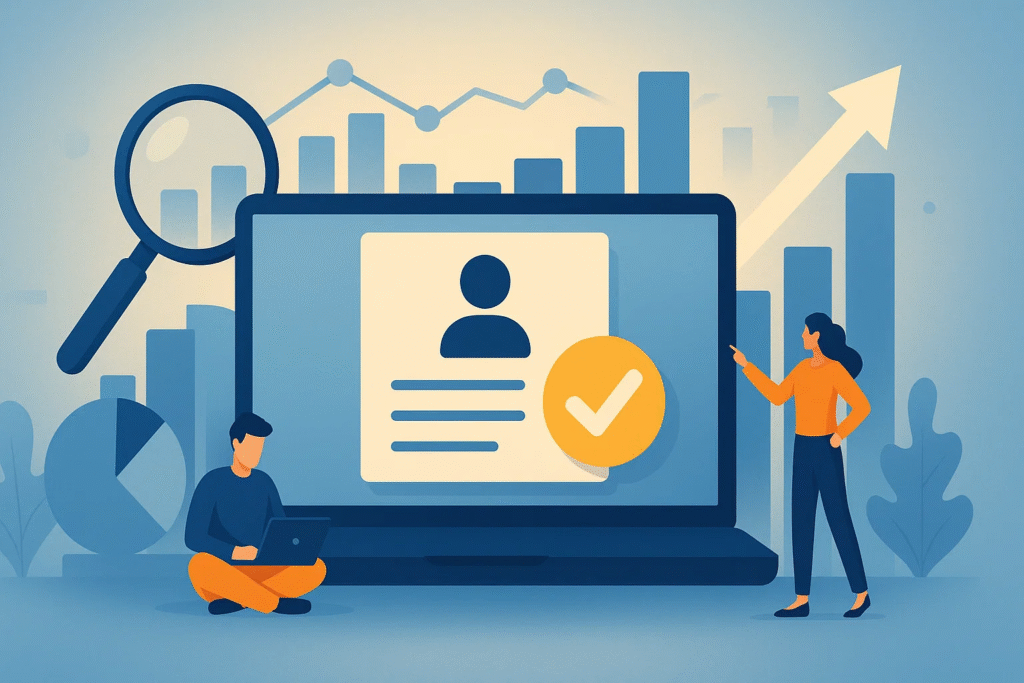
The importance of recommendation systems is underscored by their impact on consumer behavior and business outcomes. According to recent industry data, companies that effectively implement recommendation algorithms experience significant boosts in sales, user retention, and customer satisfaction.
For instance, over 35% of Amazon’s revenue is reportedly driven by its recommendation engine, while Netflix attributes more than 80% of its watched content to personalized suggestions.
These staggering figures illustrate the critical role that data-driven personalization plays in shaping digital experiences and influencing purchasing decisions.
As consumers increasingly expect real-time, relevant recommendations across every touchpoint, organizations are investing more heavily in recommendation engine technology to remain competitive in their respective industries.
From collaborative filtering and content-based filtering to hybrid and deep learning models, the underlying technologies powering recommendation engines are becoming more complex and accurate.
Innovations such as neural networks, reinforcement learning, and contextual bandits are pushing the boundaries of what’s possible, enabling systems to learn dynamically from user behavior and contextual signals.
At the same time, the proliferation of data generated by users—through clicks, views, ratings, and purchases—is providing richer input for refining these models.
With the integration of AI and real-time analytics, recommendation engines are now capable of delivering hyper-personalized experiences at scale, across various platforms and industries.
This blog delves deep into the top 100 most compelling statistics, insightful data points, and cutting-edge trends that define the current state and future direction of recommendation engine technology.
It explores key insights from major industries such as retail, media, travel, finance, and healthcare—where personalized recommendations are playing a pivotal role in enhancing customer experiences, optimizing decision-making, and boosting operational efficiency.
Furthermore, it highlights how the use of explainable AI, ethical considerations in personalization, and the importance of data privacy are reshaping the landscape of recommendation engines in 2025 and beyond.
Whether you’re a data scientist building recommendation models, a product manager exploring personalization strategies, or a marketer seeking to increase user engagement, the data compiled in this blog provides a comprehensive overview of where the industry stands today and where it’s headed.
By analyzing the top 100 statistics, you’ll gain a clearer understanding of the technologies driving personalization, the measurable business impacts of intelligent recommendations, and the strategic advantages of leveraging data to anticipate user needs.
In a digital world inundated with choices, the ability to recommend the right content, product, or service at the right time has never been more crucial.
Are you ready to explore the most up-to-date and impactful statistics that define the world of recommendation engines? Let’s dive into the numbers and trends that are shaping the future of personalized digital experiences.
Before we venture further into this article, we would like to share who we are and what we do.
About 9cv9
9cv9 is a business tech startup based in Singapore and Asia, with a strong presence all over the world.
With over nine years of startup and business experience, and being highly involved in connecting with thousands of companies and startups, the 9cv9 team has listed some important learning points in this overview of the Top 100 Recommendation Engines Statistics, Data & Trends.
If your company needs recruitment and headhunting services to hire top-quality employees, you can use 9cv9 headhunting and recruitment services to hire top talents and candidates. Find out more here, or send over an email to [email protected].
Or just post 1 free job posting here at 9cv9 Hiring Portal in under 10 minutes.
Top 100 Recommendation Engines Statistics, Data & Trends
Market Size and Growth
- The global content recommendation engine market, which plays a crucial role in personalizing user experiences across various digital platforms, is projected to grow significantly from an estimated valuation of $7.93 billion in 2024 to approximately $10.67 billion in 2025, reflecting strong demand and rapid adoption.
- Analysts have forecasted that the content recommendation engine market will experience a compound annual growth rate (CAGR) of 34.5% between 2024 and 2025, indicating a robust expansion fueled by advancements in AI and machine learning technologies.
- Looking further ahead, the content recommendation engine market is expected to reach a substantial valuation of $39.4 billion by the year 2029, growing at an impressive CAGR of 38.6% from 2025 to 2029, as more industries integrate personalized recommendation systems.
- The product recommendation engine market, which is a subset of the broader recommendation engine sector and is particularly vital for e-commerce businesses, is projected to increase from $7.42 billion in 2024 to $10.13 billion in 2025, growing at a CAGR of 36.5% as online retailers seek to enhance customer engagement.
- By 2029, the product recommendation engine market is expected to expand further to reach $34.77 billion, maintaining a strong CAGR of 36.1%, driven by the increasing reliance on AI-powered personalization in retail and other sectors.
- The overall recommendation engine market, encompassing content, product, and other specialized recommendation systems, was valued at $6.3 billion in 2024 and is forecasted to grow rapidly to $72.6 billion by 2033, with a CAGR of 29.62% during the period from 2025 to 2033, reflecting the growing importance of personalized digital experiences.
- Market estimates suggest that the recommendation engine market size will reach approximately $9.15 billion in 2025 and is projected to surge to $38.18 billion by 2030, growing at a CAGR of 33.06%, as businesses increasingly adopt AI-driven personalization technologies.
- In 2024, the recommendation engine market was valued at $7.48 billion, and it is forecasted to expand to a remarkable $114.08 billion by 2031, growing at a CAGR of 40.58% during the period from 2024 to 2031, driven by widespread digital transformation initiatives.
- Starting from a market size of $5.52 billion in 2024, the recommendation engine market is expected to grow to $6.61 billion in 2025, and then accelerate at a CAGR of 24.7% to reach $97.32 billion by 2037, reflecting long-term growth prospects.
- The global recommendation engine market was valued at $5.34 billion in 2024 and is anticipated to grow from $7.23 billion in 2025 to an impressive $118.46 billion by 2034, highlighting the transformative impact of AI and big data analytics in personalized recommendations.
Market Segmentation and Trends
- Hybrid recommendation systems, which combine multiple recommendation techniques such as collaborative filtering and content-based filtering, currently hold approximately 35% of the market share in 2024 and are expected to grow at a CAGR of 34% from 2024 to 2029, due to their superior accuracy and adaptability.
- Collaborative filtering remains the second-largest segment within the recommendation engine market, as it leverages user behavior data to generate personalized suggestions without requiring explicit item feature information.
- The collaborative filtering segment is anticipated to witness the fastest growth between 2025 and 2034, as improvements in algorithms and data availability continue to enhance its effectiveness in various applications.
- Cloud deployment models dominate the recommendation engine market as of 2024, offering scalability, cost efficiency, and ease of integration with existing digital infrastructure for businesses of all sizes.
- North America currently leads the global recommendation engine market, driven by high technology adoption rates, advanced digital infrastructure, and the presence of major technology companies investing heavily in AI and personalization.
- Europe holds the position as the second-largest market for recommendation engines, fueled by ongoing digital transformation efforts and stringent data privacy regulations such as the GDPR that influence technology adoption.
- E-commerce platforms are estimated to generate approximately 31% of their total revenue directly from product recommendations, underscoring the critical role these engines play in driving sales and customer engagement.
- Companies that implement omnichannel engagement strategies, which integrate recommendation engines across multiple customer touchpoints, report an average year-over-year growth rate of 10% and a corresponding 10% increase in average order value.
- Small and medium-sized businesses that have adopted personalized recommendation systems have observed up to a 20% increase in customer retention rates, demonstrating the effectiveness of these technologies beyond large enterprises.
- According to the U.S. Census Bureau, e-commerce sales accounted for 14.8% of total retail sales in the fourth quarter of 2023, up from 13.6% in the same quarter of 2022, reflecting the growing importance of digital shopping and recommendation technologies.
Technology Adoption and Usage
- Approximately 70% of companies worldwide have either implemented or are actively developing digital transformation strategies that incorporate recommendation engines to enhance customer experience and operational efficiency.
- In March 2024, Google Cloud launched a suite of AI-powered tools designed to help businesses rapidly develop and deploy recommendation engines, highlighting the growing accessibility of these technologies.
- The demand for data scientists, who are essential for building and optimizing recommendation systems, is projected to grow by 31% from 2022 to 2032, reflecting the increasing importance of big data analytics in this domain.
- Netflix employs hybrid recommendation systems that combine collaborative filtering with content-based filtering techniques to deliver highly personalized content suggestions to its users, improving engagement and retention.
- Amazon was among the pioneers in deploying collaborative filtering algorithms for complex product recommendations, enabling it to become a leader in personalized e-commerce experiences.
- Recommendation engines are widely used across multiple sectors, including B2C e-commerce, entertainment streaming, mobile applications, and educational platforms, each benefiting from tailored content delivery.
- The rapid advancements in artificial intelligence and machine learning technologies are key drivers behind the ongoing evolution and increased adoption of recommendation engines across industries.
- Emerging trends in recommendation engines include cross-platform integration and the incorporation of voice and conversational interfaces, which are expected to become mainstream by 2029.
- Enhanced privacy measures, including compliance with data protection regulations and improvements in AI explainability, are forecasted to become critical features in recommendation engine development over the next five years.
- The growing demand for real-time recommendations, which provide instant personalized suggestions based on current user behavior, is significantly boosting the growth of product recommendation engines.
User Engagement and Business Impact
- Businesses that implement personalized recommendation strategies have reported an average revenue increase of 15% compared to those that rely on non-personalized approaches, highlighting the financial benefits of tailored user experiences.
- Companies utilizing recommendation engines consistently observe higher levels of customer engagement and improved retention rates, as personalized suggestions encourage repeat visits and purchases.
- The major benefits of recommendation engines cited by businesses include enhanced customer retention and an increase in average order value, both of which contribute to improved profitability.
- Recommendation engines have been shown to improve conversion rates by delivering relevant product or content suggestions, thereby fostering greater customer loyalty and satisfaction.
- Organizations with robust recommendation systems report a 25% increase in close rates for sales and marketing efforts, demonstrating the effectiveness of personalized engagement.
- Studies indicate that digitalization, supported by technologies such as recommendation engines, reduces labor productivity losses by approximately 20% in sectors with higher digital adoption rates.
- As of 2023, over 5.4 billion people worldwide, representing about 67% of the global population, used the Internet, thereby expanding the potential user base for recommendation engines across various digital platforms.
- The North American recommendation engine market is projected to reach a valuation of $31.05 billion by 2037, driven by continued innovation and strong investment in AI technologies.
- The Asia Pacific region is experiencing rapid growth in recommendation engine adoption, fueled by increasing digital penetration and the expansion of e-commerce platforms across emerging markets.
- Streaming services such as Netflix leverage recommendation engines extensively to boost user satisfaction and engagement by providing highly personalized content discovery experiences.
Industry-Specific Data
- Key end-user industries for recommendation engines include retail, media and entertainment, healthcare, banking, financial services and insurance (BFSI), and information technology and telecommunications (IT & telecom), each applying personalized recommendations to improve customer experience.
- E-commerce platforms attribute approximately 31% of their total revenue to product recommendations, underscoring the critical financial impact of these systems in online retail.
- Retailers employing omnichannel strategies that integrate recommendation engines report a 10% increase in average order value, demonstrating the value of personalized experiences across multiple channels.
- The healthcare sector is increasingly adopting recommendation engines to deliver personalized patient engagement and tailored health information, improving care outcomes and patient satisfaction.
- Media and entertainment companies use recommendation engines to enhance content discovery, resulting in higher user engagement and longer session durations on their platforms.
- The BFSI industry utilizes recommendation engines to offer personalized financial product suggestions, improving customer satisfaction and cross-selling opportunities.
- The rapid growth of mobile commerce is driving increased demand for product recommendation engines optimized for mobile platforms, enhancing user experience on smartphones and tablets.
- Subscription-based services benefit from recommendation engines by improving customer retention rates and reducing churn through personalized content and product suggestions.
- Cloud-based recommendation engines are favored by media platforms for their scalability and ability to process large volumes of data in real time, enabling dynamic content personalization.
- AI-powered recommendation engines significantly improve customer experience in digital marketing campaigns by delivering highly targeted and relevant offers.
Regional Market Data
- North America leads the global recommendation engine market due to early adoption of AI technologies, robust digital infrastructure, and significant investments by leading technology companies.
- Europe’s recommendation engine market growth is influenced by stringent data privacy laws such as GDPR, which encourage the development of transparent and privacy-compliant recommendation systems.
- Asia Pacific is an emerging and rapidly expanding market for recommendation engines, driven by increasing internet penetration, mobile device usage, and the growth of e-commerce.
- Latin America and the Middle East & Africa regions represent smaller but fast-growing markets for recommendation engines, as digital transformation initiatives gain momentum.
- Germany, the United Kingdom, and France are among the leading European countries in the adoption and deployment of recommendation engines across various industries.
- North America’s recommendation engine market benefits from the presence of major technology companies and startups that continuously innovate and invest in AI-driven personalization technologies.
- Europe places a strong emphasis on ethical data practices and transparency in recommendation systems, which shapes market dynamics and technology development.
- The Asia Pacific region’s rapid growth in internet penetration and digital commerce is a key factor fueling the expansion of the recommendation engine market.
- Cloud-based recommendation engine adoption in North America is the highest globally, driven by the need for scalable, flexible, and cost-effective personalization solutions.
- The United States leads the world in integrating artificial intelligence within recommendation engines, supported by a strong ecosystem of technology companies and research institutions.
Algorithm and System Performance
- Hybrid recommendation systems, which combine collaborative filtering and content-based filtering techniques, have been shown to significantly improve recommendation accuracy compared to systems relying on a single method.
- Collaborative filtering algorithms recommend items based on the preferences and behaviors of similar users, enabling personalized suggestions without requiring detailed knowledge of item attributes.
- Content-based filtering algorithms generate recommendations by analyzing item features and matching them with a user’s past behavior and preferences, providing a personalized experience.
- Hybrid systems integrate collaborative filtering and content-based filtering to leverage the strengths of both approaches, resulting in more relevant and diverse recommendations.
- Advances in artificial intelligence and machine learning have enhanced the relevance and personalization capabilities of recommendation engines, leading to improved user satisfaction.
- Real-time analytics capabilities enable recommendation engines to respond instantly to user interactions, providing up-to-date and contextually relevant suggestions.
- The adoption of cloud computing platforms allows recommendation engines to perform faster updates, handle large data volumes efficiently, and reduce maintenance costs for businesses.
- The integration of voice and conversational interfaces into recommendation engines is expected to increase significantly by 2029, enabling more natural and interactive user experiences.
- Context-aware recommendation systems, which consider factors such as location, time, and user mood, are becoming increasingly popular as they provide more personalized and relevant suggestions.
- AI explainability and transparency are gaining importance in recommendation engine design, as users demand to understand how and why certain recommendations are made to build trust.
User Behavior and Adoption Statistics
- Approximately 31% of total revenue generated by e-commerce platforms is attributed directly to product recommendations, demonstrating their critical role in driving online sales.
- As of 2023, 67% of the global population, or over 5.4 billion people, were internet users, greatly expanding the potential audience for recommendation engines across digital platforms.
- Businesses that implement personalized recommendation strategies report an average increase in revenue of 15% compared to those that do not utilize personalization technologies.
- Small businesses that have adopted personalized recommendation systems have observed a 20% increase in customer retention rates, highlighting the effectiveness of these tools in competitive markets.
- Companies that employ omnichannel engagement strategies supported by recommendation engines experience a 25% higher close rate in sales and marketing efforts.
- Approximately 70% of companies worldwide have developed or are in the process of developing digital transformation strategies that include the deployment of recommendation engines to enhance customer experience.
- Recommendation engines are extensively used in streaming services, e-commerce platforms, and digital marketing campaigns to deliver personalized content and offers.
- Retailers utilizing recommendation engines report an average increase of 10% in order value, driven by personalized product suggestions across multiple channels.
- Cloud-based recommendation engines dominate the market due to their scalability, flexibility, and cost-effectiveness, making them the preferred choice for businesses of all sizes.
- Artificial intelligence-driven recommendation engines have demonstrated improved accuracy and higher user engagement compared to traditional recommendation methods.
Forecast and Future Trends
- The recommendation engine market is projected to reach an estimated value of $118.46 billion by 2034, reflecting rapid adoption and technological advancements in AI and big data analytics.
- The compound annual growth rate (CAGR) of the recommendation engine market is expected to exceed 30% through 2030, driven by increasing demand for personalized digital experiences.
- Hybrid recommendation systems are forecasted to continue growing at the fastest pace, with a CAGR of approximately 34% through 2029, due to their enhanced accuracy and flexibility.
- By 2029, real-time and context-aware recommendation capabilities are expected to become standard features in most recommendation engines, improving relevance and user satisfaction.
- Privacy and data protection concerns will increasingly shape the development and deployment of recommendation engines, leading to innovations in data handling and user consent mechanisms.
- AI explainability, which provides transparency into how recommendations are generated, will become a key feature of recommendation engines by 2029 to build user trust.
- Cross-platform integration will increase substantially, enabling seamless recommendation experiences across devices and channels.
- Voice and conversational interfaces will be widely integrated into recommendation engines by 2029, allowing users to interact with personalized systems through natural language.
- Advances in big data analytics will continue to drive the sophistication and effectiveness of recommendation engines, enabling deeper insights and more accurate personalization.
- The adoption of cloud computing for recommendation engines will steadily increase through 2034, providing businesses with scalable and cost-efficient personalization solutions.
Business and Economic Impact
- Digitalization efforts supported by recommendation engines have been shown to reduce labor productivity losses by approximately 20%, enhancing overall operational efficiency in various sectors.
- Recommendation engines contribute significantly to business revenue growth, particularly in e-commerce, by enabling personalized product discovery and targeted marketing.
- Companies that deploy recommendation engines gain a competitive advantage through enhanced personalization, which improves customer satisfaction and loyalty.
- Recommendation engines play a critical role in improving customer lifetime value by fostering loyalty and encouraging repeat purchases through tailored suggestions.
- Investments in artificial intelligence and machine learning technologies for recommendation engines are increasing rapidly as businesses seek to capitalize on personalization benefits.
- The retail sector’s shift toward omnichannel strategies has boosted demand for recommendation engines that can deliver consistent personalized experiences across multiple platforms.
- Subscription-based services utilize recommendation engines to reduce customer churn and increase retention by providing personalized content and product recommendations.
- Recommendation engines help businesses optimize their marketing and sales strategies by delivering targeted offers and improving customer segmentation.
- The exponential growth in digital content volume across media, e-commerce, and social platforms is driving the expansion of the recommendation engine market.
- Recommendation engines have become critical tools for customer engagement in digital transformation initiatives, enabling businesses to deliver personalized experiences at scale.
Conclusion
As we’ve explored throughout this blog, recommendation engines are no longer just a supplementary feature but a cornerstone of modern digital ecosystems. From enhancing the consumer experience to driving significant business growth, the impact of these systems is undeniable. The statistics and trends presented here illustrate just how central personalized recommendations have become in shaping consumer behavior and influencing purchasing decisions. Whether it’s the staggering revenue figures generated by Amazon’s recommendation engine or the 80% of Netflix content watched based on personalized suggestions, the evidence is clear—recommendation engines are revolutionizing the way businesses engage with users.
The evolution of recommendation engine technologies, from simple collaborative filtering models to sophisticated deep learning algorithms, highlights the industry’s constant drive for innovation. The rise of AI, machine learning, and big data analytics has made it possible to create hyper-personalized experiences that cater to individual preferences in real-time. With advancements in neural networks, reinforcement learning, and contextual bandits, the accuracy and relevance of recommendations are improving, offering businesses an unparalleled opportunity to enhance customer satisfaction and retention.
Looking ahead, it’s clear that the future of recommendation engines will be marked by even greater personalization and precision. As data continues to grow in volume and complexity, the challenge of managing and interpreting that data will be met by more advanced models and tools. In particular, the growing importance of explainable AI will ensure that consumers and businesses alike understand how recommendations are generated, fostering trust and improving the transparency of these systems. Furthermore, data privacy and ethical considerations will remain crucial as companies strive to balance personalization with user rights and protection.
The businesses that continue to embrace and innovate with recommendation engine technology will be the ones that lead in an increasingly competitive digital landscape. Those that leverage the latest insights, technologies, and strategies will unlock new opportunities to deepen customer relationships, boost conversions, and drive long-term loyalty. As we move further into 2025 and beyond, companies will need to stay ahead of emerging trends—such as the integration of augmented reality (AR) for personalized shopping experiences or the rise of conversational AI-driven recommendations—if they hope to remain relevant in an increasingly digital and dynamic marketplace.
In conclusion, the data and trends presented in this blog underscore the transformative power of recommendation engines across industries. Whether for e-commerce, entertainment, or content distribution, these systems are indispensable tools that are fundamentally changing how businesses interact with customers. As the technology continues to evolve, staying informed about the latest developments and best practices will be essential for organizations seeking to harness the full potential of recommendation engines. By doing so, they can not only meet consumer expectations but also exceed them, delivering personalized, seamless experiences that drive success in an increasingly complex and competitive market. The future of recommendation engines is bright, and those who adapt will be best positioned to lead the way in delivering exceptional customer experiences and achieving business growth.
If you find this article useful, why not share it with your hiring manager and C-level suite friends and also leave a nice comment below?
We, at the 9cv9 Research Team, strive to bring the latest and most meaningful data, guides, and statistics to your doorstep.
To get access to top-quality guides, click over to 9cv9 Blog.
People Also Ask
What are recommendation engines and how do they work?
Recommendation engines use algorithms to suggest relevant products, services, or content to users based on their preferences, past behaviors, and interactions. They leverage data to provide personalized experiences across various platforms like e-commerce and streaming.
What are the top industries using recommendation engines?
E-commerce, entertainment (Netflix, Spotify), online retail, travel (Airbnb, Expedia), and social media (Facebook, Instagram) are the primary industries benefiting from recommendation engines, driving engagement and conversions.
How do recommendation engines improve customer experience?
Recommendation engines personalize content and product suggestions based on users’ preferences, improving their browsing experience, saving time, and increasing satisfaction by offering relevant options without overwhelming them.
What are the benefits of recommendation engines for businesses?
Businesses using recommendation engines experience higher user engagement, increased conversions, better customer retention, and enhanced user satisfaction, ultimately driving revenue growth through personalized offerings.
What are collaborative filtering and content-based filtering?
Collaborative filtering recommends items based on similar user behavior, while content-based filtering suggests items similar to what a user has shown interest in, based on attributes like genre, features, or keywords.
How do machine learning algorithms improve recommendation engines?
Machine learning allows recommendation engines to analyze vast amounts of user data to refine recommendations over time, adapting to user preferences and behaviors, enhancing the accuracy of suggestions.
What are hybrid recommendation engines?
Hybrid recommendation engines combine multiple algorithms, like collaborative filtering and content-based filtering, to improve recommendation accuracy by addressing the limitations of individual methods.
What are the key statistics for recommendation engines in 2025?
Recent data shows that personalized recommendations account for over 35% of e-commerce revenue, and 80% of Netflix’s content is viewed due to personalized suggestions, highlighting the importance of recommendation engines.
How do recommendation engines impact e-commerce sales?
Recommendation engines drive higher sales by suggesting relevant products to customers, increasing average order value, and promoting upsells or cross-sells, ultimately boosting conversions and improving user experience.
What role does AI play in recommendation engines?
AI enhances recommendation engines by enabling them to learn from user data, predict preferences, and optimize recommendations in real-time, creating highly personalized and accurate suggestions.
What are some common challenges in building recommendation engines?
Challenges include data sparsity, bias in algorithms, real-time processing requirements, and ensuring user privacy while delivering accurate, personalized recommendations at scale.
How do recommendation engines use user data?
Recommendation engines analyze user behavior, such as clicks, ratings, searches, and past purchases, to identify patterns and preferences, which they use to offer personalized content or product recommendations.
What is explainable AI in recommendation engines?
Explainable AI in recommendation engines ensures transparency by providing users with clear reasons for why specific recommendations are made, improving trust and accountability.
How does recommendation engine accuracy impact businesses?
Highly accurate recommendations increase user engagement, improve conversion rates, and reduce churn, leading to stronger customer loyalty and ultimately higher revenue for businesses.
Can recommendation engines be used in healthcare?
Yes, recommendation engines are used in healthcare to suggest personalized treatments, medical content, and wellness programs based on individual patient data, improving care and outcomes.
What is the future of recommendation engines?
The future includes increased use of deep learning and AI, more adaptive models, better integration with emerging technologies like augmented reality (AR), and a stronger focus on ethical AI and data privacy.
How does data privacy affect recommendation engines?
As recommendation engines rely on user data, ensuring data privacy is critical. Businesses must comply with regulations like GDPR and implement privacy measures to maintain user trust while providing personalized recommendations.
What is the role of big data in recommendation engines?
Big data enables recommendation engines to analyze vast amounts of user behavior, preferences, and interaction data, enhancing the accuracy and effectiveness of recommendations and personalizations.
How do recommendation engines use natural language processing (NLP)?
NLP helps recommendation engines understand and analyze textual data, such as reviews, comments, and search queries, allowing for better matching of content or products to users based on language and intent.
What are the limitations of recommendation engines?
Limitations include data privacy concerns, reliance on historical data which can lead to bias, and the challenge of personalizing recommendations for new or inactive users with limited data.
How do recommendation engines affect user engagement?
By delivering relevant and personalized suggestions, recommendation engines keep users engaged, encouraging them to spend more time on platforms, explore more content, and make purchases.
How do recommendation engines impact social media platforms?
Recommendation engines on social media suggest relevant posts, friends, groups, and advertisements, driving engagement, improving content discovery, and increasing time spent on the platform.
Can recommendation engines be integrated with mobile apps?
Yes, recommendation engines are commonly integrated with mobile apps, providing users with personalized recommendations based on their activity, preferences, and location for a seamless experience.
What is reinforcement learning in recommendation engines?
Reinforcement learning allows recommendation engines to improve over time by learning from user feedback and interactions, adjusting recommendations based on rewards (e.g., clicks or purchases) to refine suggestions.
How can businesses use recommendation engines for marketing?
Businesses can leverage recommendation engines in marketing by delivering personalized product suggestions, targeted promotions, and dynamic content that resonate with individual users, boosting conversions and customer satisfaction.
What is collaborative filtering in recommendation engines?
Collaborative filtering is a method that predicts a user’s interests by analyzing preferences and behaviors of similar users, providing personalized recommendations based on their actions.
How do recommendation engines handle new users with no data?
New users, or “cold-start” users, are typically served with popular or trending items until enough user behavior data is collected to personalize the recommendations based on their preferences.
What impact do recommendation engines have on content platforms?
Content platforms like YouTube and Spotify use recommendation engines to suggest videos and music, increasing user engagement, keeping users on the platform longer, and optimizing content discovery.
What are the ethical considerations for recommendation engines?
Ethical considerations include avoiding algorithmic bias, ensuring data privacy, providing transparency in how recommendations are made, and avoiding manipulative or harmful content recommendations.
How do recommendation engines affect customer retention?
Personalized recommendations improve customer retention by fostering loyalty, creating a sense of individualized attention, and providing users with content or products that align with their preferences.
How do recommendation engines handle diverse user preferences?
Recommendation engines handle diverse preferences by segmenting users into groups based on shared behaviors or attributes and offering tailored suggestions that match the unique interests of each segment.
What types of recommendation algorithms are used?
Common algorithms include collaborative filtering, content-based filtering, matrix factorization, and deep learning models, each offering strengths in different contexts and data types.
How do recommendation engines drive upselling and cross-selling?
Recommendation engines suggest complementary or higher-value products to users based on their purchase history and preferences, encouraging upselling and cross-selling that boosts average order value.
How can businesses track the performance of recommendation engines?
Businesses track the performance of recommendation engines through metrics like click-through rates, conversion rates, revenue per user, and customer engagement levels, enabling them to assess effectiveness and optimize models.
Sources
- Precedence Research – Recommendation Engine Market Size and Forecasts (2024–2034)
- The Business Research Company – Global Content Recommendation Engine Market Report 2025
- IndustryARC – Recommendation Engine Market Research and Industry Analysis (2020–2025)
- Research Nester – Recommendation Engine Market Size, Historic Data, and Forecasts to 2037
- Mordor Intelligence – Product Recommendation Engine Market Size & Share Analysis (2025–2030)
- The Business Research Company – Product Recommendation Engine Market Insights 2025–2034
- The Business Research Company – Product Recommendation Engine Global Market Report 2025
- Research and Markets – Product Recommendation Engine Market Report 2025