Key Takeaways
- The top recommendation engines in 2025 leverage advanced AI algorithms to deliver highly personalized experiences and boost engagement.
- Key features like real-time recommendations, seamless integrations, and robust A/B testing drive significant performance improvements across industries.
- Businesses using these top engines report higher conversion rates, increased average order value (AOV), and enhanced customer satisfaction.
In today’s highly competitive digital landscape, delivering personalized experiences has become a critical differentiator for businesses seeking to capture and retain user attention. From e-commerce platforms suggesting the perfect product to streaming services curating tailor-made content playlists, recommendation engines have emerged as the driving force behind some of the most engaging user experiences online. As we move into 2025, the capabilities of recommendation systems have evolved dramatically, fueled by advancements in artificial intelligence, machine learning, and big data analytics.
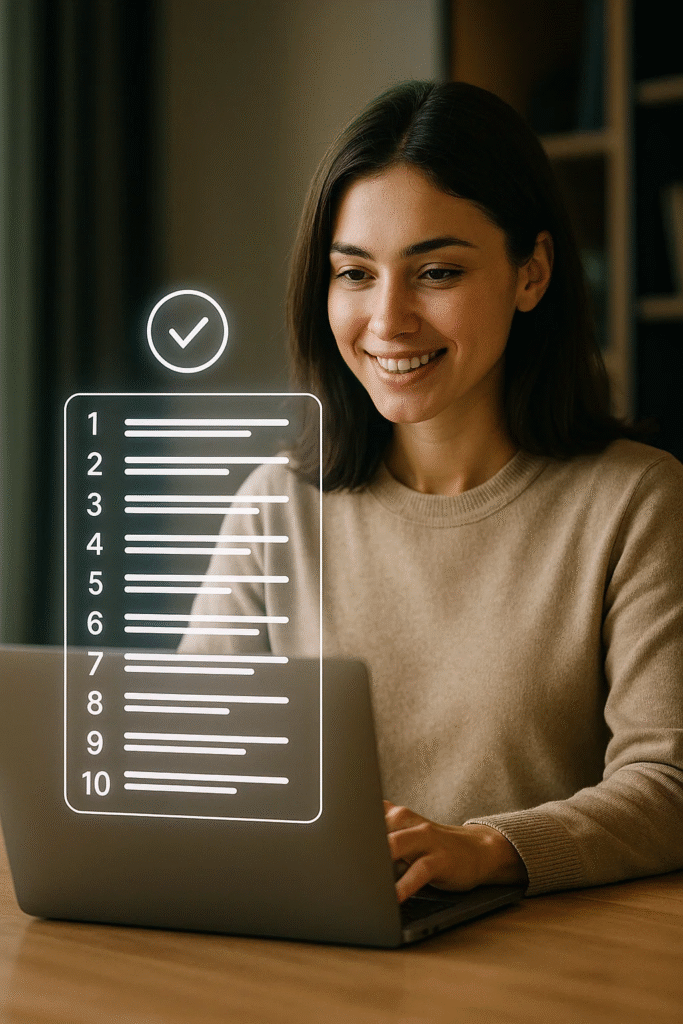
A recommendation engine, at its core, is a sophisticated software application designed to predict and present content, products, or services that align with a user’s preferences or past behavior. These systems play a vital role in various industries, including retail, entertainment, finance, healthcare, education, and travel, by helping users discover relevant items more efficiently. Whether it’s Amazon recommending products, Netflix suggesting TV shows, or Spotify generating music playlists, recommendation algorithms have fundamentally reshaped the way consumers interact with digital platforms.
What makes 2025 an especially exciting year for recommendation technologies is the exponential growth of data and the maturing of artificial intelligence tools capable of processing this information in real time. Today’s top recommendation engines are no longer confined to basic collaborative or content-based filtering methods. Instead, they leverage a fusion of deep learning, natural language processing, real-time user modeling, and hybrid recommendation strategies to deliver hyper-personalized outputs that adapt continuously to user behavior and contextual signals.
Moreover, with increasing concerns around user privacy and data transparency, the latest recommendation systems are also placing a strong emphasis on ethical AI practices, fairness, explainability, and compliance with data regulations such as GDPR and CCPA. This dual focus on precision and privacy is shaping the next generation of recommendation engines to be not only intelligent but also trustworthy and user-centric.
For businesses, integrating a top-tier recommendation engine has transitioned from a competitive advantage to an operational necessity. Enhanced customer engagement, increased conversion rates, improved retention, and higher lifetime value are just a few of the tangible benefits that organizations can achieve by leveraging best-in-class recommendation technology. As digital consumers continue to demand more intuitive, relevant, and seamless experiences, the pressure is on for companies to adopt the most effective tools available in the market.
This blog explores the Top 10 Best Recommendation Engines in 2025, analyzing their standout features, technological underpinnings, use cases, and the industries they serve. Whether you’re a developer, product manager, marketer, or business executive, this comprehensive guide will provide valuable insights into which platforms are leading the way in recommendation intelligence and how they can be harnessed to drive business growth and customer satisfaction in the coming year.
Before we venture further into this article, we would like to share who we are and what we do.
About 9cv9
9cv9 is a business tech startup based in Singapore and Asia, with a strong presence all over the world.
With over nine years of startup and business experience, and being highly involved in connecting with thousands of companies and startups, the 9cv9 team has listed some important learning points in this overview of the Top 10 Best Recommendation Engines in 2025.
If your company needs recruitment and headhunting services to hire top-quality employees, you can use 9cv9 headhunting and recruitment services to hire top talents and candidates. Find out more here, or send over an email to [email protected].
Or just post 1 free job posting here at 9cv9 Hiring Portal in under 10 minutes.
Top 10 Best Recommendation Engines in 2025
- Adobe Target
- Amazon Personalize
- Google Cloud Recommendation AI (Vertex AI Search)
- Microsoft Azure Personalizer (Retiring in 2026)
- SAP Emarsys
- Dynamic Yield (by Mastercard)
- Constructor.io
- Bloomreach
- Salesforce Einstein Recommendations
- Recombee
1. Adobe Target

Adobe Target has firmly established itself as one of the most sophisticated and high-performing recommendation engines in 2025, earning top recognition across professional software review platforms such as PeerSpot, where it consistently ranks as the number one personalization solution. Designed for enterprises seeking to deliver seamless and data-driven customer experiences, Adobe Target goes far beyond traditional recommendation algorithms by integrating advanced AI-powered testing, optimization, and personalization tools into a unified platform.
At the core of Adobe Target’s value proposition lies its ability to orchestrate hyper-personalized customer journeys through actionable insights derived from continuous experimentation and real-time analytics. The platform empowers organizations to implement and refine recommendation strategies using A/B testing, multivariate testing, and auto-allocation features, ensuring that every digital interaction is informed by robust performance data. These capabilities enable businesses to adapt dynamically to user behavior, market shifts, and customer preferences, ensuring that recommendations remain contextually relevant and conversion-focused.
One of the defining strengths of Adobe Target is its rigorous optimization engine, which allows companies to evaluate multiple variables—from product placement and content sequence to layout and messaging—across a range of audience segments. The platform’s recommendation logic is fueled by Adobe Sensei, Adobe’s proprietary AI and machine learning framework, which intelligently personalizes content and product suggestions in real time based on behavioral, contextual, and historical data inputs.
Quantifiable business impact is a central reason Adobe Target is considered one of the top recommendation engines of the year. Numerous case studies highlight significant uplifts in key performance indicators. For example, organizations leveraging Adobe Target have reported conversion rate improvements of up to 41% (HanesBrands), a 25% boost for Esri, and a 30% increase for a leading online fashion retailer. Additional measurable benefits include heightened click-through rates, increased revenue per visit, higher average order values, and enhanced overall user engagement.
In terms of scalability and customization, Adobe Target offers a flexible pricing model tailored to enterprise needs. Factors influencing pricing include the range of product features selected, data volume, and the extent of omnichannel deployment. While annual costs typically begin around $36,000, enterprise implementations may exceed $200,000 depending on the complexity and breadth of use.
From a strategic standpoint, Adobe Target is particularly well-suited for large-scale businesses operating across multiple digital touchpoints that require continuous optimization and personalization at scale. Its ability to unify marketing, product, and experience teams through a centralized testing and recommendation hub makes it an essential tool for organizations aiming to maximize digital ROI and customer lifetime value in 2025.
2. Amazon Personalize

Amazon Personalize has emerged as one of the most powerful and accessible recommendation engines in 2025, providing organizations with scalable, real-time personalization capabilities backed by Amazon Web Services’ robust AI infrastructure. Positioned as a fully managed machine learning service, Amazon Personalize enables businesses to deploy tailored recommendation systems with remarkable speed and minimal operational overhead—making enterprise-grade personalization more attainable than ever before.
What sets Amazon Personalize apart is its ability to ingest and analyze billions of user interactions across millions of items to deliver precise, context-aware suggestions in real time. Leveraging the same technology that powers Amazon’s own retail platform, this solution harnesses a diverse set of machine learning algorithms, including collaborative filtering, content-based filtering, and advanced sequence modeling techniques. The platform also offers domain-optimized recommendation strategies specifically designed for high-impact industries such as e-commerce, digital media, entertainment, travel, and retail, allowing businesses to offer customized experiences across websites, mobile apps, and customer support channels.
Amazon Personalize distinguishes itself with its exceptionally low latency, enabling recommendations to be delivered almost instantaneously—an essential factor in driving user engagement and conversions during critical decision-making moments. The engine’s flexibility is further enhanced by its ability to build individualized models tailored to unique datasets and business goals, allowing for personalized ranking, user segmentation, and predictive product matching. Users benefit from a highly responsive and adaptive system that evolves continuously as more behavioral data is collected.
Industry adoption of Amazon Personalize continues to expand rapidly. As of March 2025, it holds a 13.8% market share within the personalization engine space and serves a global customer base exceeding 1,600 organizations. The platform’s effectiveness is consistently validated through empirical performance metrics, including significant improvements in click-through rates, user retention, and conversion rates. Notably, companies such as Razer have reported a 10-fold improvement in CTR compared to industry benchmarks, while brands like Dress the Population and Ticketek have achieved conversion increases of 28% and 250%, respectively. Other measurable benefits include extended watch times, increased revenue per visit, and higher purchase frequency.
One of the key advantages of Amazon Personalize lies in its accessible pricing model. It operates on a pay-as-you-go basis, allowing organizations to scale their usage according to need without upfront commitments. Charges are incurred based on data ingestion, training hours, and real-time inference calls, with a free tier available for the first two months—making it particularly appealing for startups and mid-sized enterprises exploring AI-driven personalization for the first time.
In summary, Amazon Personalize exemplifies the convergence of enterprise-grade AI capabilities and operational simplicity. Its ability to generate real-time, high-impact recommendations across diverse industry verticals, combined with flexible pricing and rapid deployment, makes it one of the top recommendation engines of 2025 for businesses aiming to deliver meaningful and dynamic user experiences at scale.
3. Google Cloud Recommendation AI (Vertex AI Search)

Google Cloud Recommendation AI, now integrated into the broader Vertex AI Search framework, stands as a premier solution in the recommendation engine landscape of 2025. Powered by Google’s cutting-edge artificial intelligence and deep learning technologies, this platform delivers real-time, personalized product and content recommendations with remarkable precision and scalability. Designed for enterprises that prioritize data-driven engagement strategies, it combines automation, adaptability, and performance optimization to create highly customized digital experiences across multiple touchpoints.
One of the most compelling aspects of Google Cloud Recommendation AI is its seamless integration with the Google Cloud ecosystem. Businesses can quickly onboard and activate the engine using their existing datasets—whether derived from purchase history, cart activity, viewing behavior, or frequently co-purchased items. The platform’s architecture is designed to handle complex data inputs with minimal manual configuration, empowering teams to move from ingestion to actionable insights in a fraction of the time typically required by traditional personalization systems.
At the heart of its personalization engine are transformer-based deep learning models, which are among the most sophisticated in modern AI. These models analyze not only individual user behavior but also contextual and sequential interactions to infer user intent and deliver recommendations that are timely, relevant, and optimized for engagement. These advanced models support multiple recommendation scenarios, including similar-item suggestions, “frequently bought together” bundles, next-best-action predictions, and contextual personalization tailored to individual user journeys.
Google Cloud Recommendation AI has found widespread adoption across key verticals such as retail, e-commerce, and media & entertainment, where real-time engagement and precision targeting are essential. Its deployment enables organizations to fine-tune their personalization strategy using business rules that align with specific commercial goals—whether it’s maximizing conversions, boosting revenue, or enhancing content discovery. These customizations help businesses maintain control over their recommendation logic while benefiting from the full power of machine learning automation.
Performance metrics further validate the platform’s strategic value. Recommendation effectiveness is measured through industry-standard KPIs, including engagement rate, conversion lift, revenue impact, recall, precision, and normalized discounted cumulative gain (NDCG). Case studies highlight measurable improvements in user interaction and monetization. For instance, media companies using the platform have reported notable gains in viewership and ad revenue, while retailers have seen significant uplifts in sales volume and average order value due to more intelligently curated product suggestions.
The pricing structure for Vertex AI Search is also designed with flexibility in mind. It follows a pay-as-you-go model, allowing businesses to scale based on actual usage while benefiting from cost-efficiency through automated savingsand a free usage tier for smaller workloads or pilot projects. This structure makes it an appealing option for both large enterprises and mid-market players seeking to test and scale AI-driven personalization with predictable costs.
In conclusion, Google Cloud Recommendation AI, under the Vertex AI umbrella, represents a highly advanced, adaptable, and results-driven recommendation engine in 2025. Its deep learning foundations, customizable rule-based logic, and seamless integration into the Google Cloud infrastructure position it as a top-tier choice for organizations aiming to deliver intelligent, real-time personalization that drives engagement and business growth.
4. Microsoft Azure Personalizer (Retiring in 2026)

Microsoft Azure Personalizer, a component of the broader Azure AI Services suite, has long served as a sophisticated recommendation engine that employs reinforcement learning to deliver real-time decision-making capabilities. Despite its planned retirement on October 1, 2026, Azure Personalizer remains a noteworthy contender among the top recommendation engines in 2025 due to its distinct approach to personalization and adaptive intelligence. It exemplifies how machine learning can be applied not only to predict user preferences but also to dynamically optimize experiences based on evolving behavioral patterns.
At its core, Azure Personalizer distinguishes itself by leveraging contextual bandit algorithms, a type of reinforcement learning model specifically engineered to make immediate decisions within dynamic, uncertain environments. Unlike traditional collaborative or content-based filtering models that rely heavily on historical data, contextual bandits continuously explore and exploit the most relevant actions based on user interactions and contextual signals. This empowers applications to serve content, products, or offers that are most likely to resonate with users in real-time—without requiring extensive pre-labeled datasets.
One of the platform’s most valuable attributes is its ease of integration. Designed with developer accessibility in mind, Azure Personalizer requires minimal coding and can be embedded into existing applications with streamlined APIs. This approach significantly reduces the implementation barrier for companies seeking to harness reinforcement learning without building infrastructure from the ground up. It is particularly useful in scenarios demanding rapid inference and intelligent adaptation, such as online product recommendations, targeted content delivery, ad optimization, and personalized user journeys.
Although Azure Personalizer commands a smaller share of the personalization engine market compared to larger players like Amazon and Google, its underlying technology has made it a reliable solution in a range of enterprise applications. Industries including retail, digital publishing, gaming, and online services have utilized it to enhance click-through rates, conversion metrics, and overall user engagement. Even Microsoft’s internal use of the service—for instance, optimizing AI-powered lead qualification—has led to measurable improvements in sales conversion outcomes.
From a cost perspective, the engine operates on a pay-as-you-go pricing structure, which enables businesses to scale usage according to their operational needs. For organizations looking to evaluate its capabilities, Microsoft offers a generous free tier, providing up to 50,000 transactions per month for the first 12 months. This accessible entry point has allowed businesses of varying sizes to test real-time personalization strategies with minimal upfront investment.
Despite its scheduled deprecation in late 2026, Microsoft Azure Personalizer remains highly relevant in 2025 due to its innovative application of reinforcement learning, its real-world performance benefits, and its proven capacity to deliver personalized experiences with minimal technical overhead. For organizations exploring AI-powered personalization strategies within the Microsoft ecosystem, it continues to represent a robust and forward-thinking solution—especially for those seeking adaptive systems that learn and evolve in real time.
5. SAP Emarsys

SAP Emarsys has firmly established itself as one of the premier recommendation engines in 2025, owing to its robust AI-driven personalization capabilities and its ability to deliver tailored, data-informed experiences across multiple customer touchpoints. As part of SAP’s broader customer engagement and commerce ecosystem, Emarsys is purpose-built to empower brands in sectors such as retail, consumer goods, and travel with sophisticated tools for personalized marketing automation and omnichannel engagement.
Distinguished by its AI-powered predictive intelligence, SAP Emarsys enables businesses to create dynamic and highly contextual customer journeys. Through advanced machine learning algorithms, the platform analyzes vast volumes of behavioral, transactional, and engagement data to generate individualized product or content recommendations that evolve with user behavior. One of its key differentiators lies in its predictive segmentation engine, which allows marketers to anticipate future behaviors—such as likelihood to purchase or churn—and to act proactively with relevant messaging and offers.
Another standout feature is the platform’s integration of generative AI tools, designed to streamline content creation processes at scale. This capability equips marketing teams with the ability to rapidly produce on-brand, personalized copy and visuals for emails, product pages, and promotional campaigns—an invaluable asset for brands managing high-frequency communication across multiple channels. Combined with industry-specific templates and best-practice playbooks, Emarsys enables faster time-to-value while maintaining strategic alignment with sector-specific customer expectations.
SAP Emarsys’s position as a Leader in the 2025 Gartner Magic Quadrant for Personalization Engines further reinforces its status as a top-tier solution. Its growing adoption across the Americas and other global markets demonstrates its scalability and proven effectiveness in enterprise settings. The engine is particularly effective in email personalization, where its AI-driven widgets and dynamic content blocks have shown measurable increases in click-through rates, conversion metrics, and average order values.
The platform supports a range of recommendation logics, from collaborative filtering to user-based affinities, and is designed to function seamlessly across various digital interfaces, including websites, mobile apps, and email campaigns. Performance metrics cited in case studies reveal substantial business impact, including a 67% increase in lead-to-first-time buyer conversion rates and a fivefold increase in revenue generation from active customers. These outcomes reflect the engine’s ability to drive both engagement and bottom-line growth through intelligent personalization.
In terms of pricing, SAP Emarsys operates within an enterprise-grade cost structure, offering customized plans tailored to the size, scope, and complexity of a business’s digital marketing and personalization needs. While this positions it as a premium solution, the return on investment is justified by its ability to deliver tangible and sustainable business improvements at scale.
Overall, SAP Emarsys is more than just a personalization engine—it is a comprehensive customer engagement platform that combines deep AI capabilities, real-time decision-making, and intuitive marketing tools. For organizations seeking a future-ready solution to optimize customer lifecycle management and drive omnichannel personalization at scale, Emarsys remains a clear choice among the top recommendation engines of 2025.
6. Dynamic Yield (by Mastercard)

Dynamic Yield, a Mastercard-acquired technology, has emerged as one of the top-performing recommendation engines in 2025, thanks to its innovative approach to experience optimization and real-time personalization. Positioned as a next-generation AI-powered Experience Optimization Platform, it equips businesses with the tools to craft hyper-personalized digital experiences across a diverse array of customer touchpoints, including websites, mobile apps, email campaigns, and kiosks.
At the core of Dynamic Yield’s competitive advantage is its advanced algorithmic architecture, which seamlessly blends deep learning, collaborative filtering, predictive modeling, and contextual data processing. Its proprietary Algorithm Studio further enhances flexibility by allowing users to design and fine-tune bespoke algorithms aligned with unique business goals and user behavior patterns. This high level of customization makes it particularly attractive for enterprises that require nuanced control over how recommendations are presented and optimized.
Dynamic Yield is widely adopted across industries such as e-commerce, retail, financial services, travel, and quick-service restaurants, where real-time decision-making and adaptive personalization are critical for driving engagement and conversion. The platform’s contextual targeting engine factors in behavioral signals, geo-location, device type, and other real-time data points to ensure that content, products, or offers are not only relevant but delivered in the right moment and format.
The company’s sustained innovation and client satisfaction have earned it recognition as a Leader in the 2025 Gartner Magic Quadrant for Personalization Engines, underscoring its strong market presence and technical maturity. Dynamic Yield is particularly praised for its cross-channel consistency, allowing businesses to maintain cohesive messaging and recommendations across all digital interfaces, which is crucial for maintaining brand trust and optimizing user experience.
In terms of performance, Dynamic Yield delivers measurable business outcomes through rigorous A/B and multivariate testing, enabling marketers to validate the impact of their personalization strategies in real time. Key performance indicators (KPIs) often improved through the platform include average order value (AOV), click-through rate (CTR), conversion rate, and average revenue per user (ARPU). Numerous case studies have documented transformative results, such as significant lifts in engagement metrics and double-digit increases in revenue per visitor.
The platform’s pricing is positioned at the enterprise level, with customized cost structures based on usage volume, channel coverage, and strategic requirements. However, it also includes robust onboarding support, technical assistance, and dedicated customer success management—making it a high-value solution for businesses that prioritize long-term personalization growth.
Ultimately, Dynamic Yield distinguishes itself through its blend of AI sophistication, operational flexibility, and business impact. With its ability to unify data, predict customer needs, and deliver tailored recommendations at scale, the platform remains one of the most powerful personalization engines available in 2025. For enterprises seeking to unlock deeper customer insights and drive meaningful engagement across every stage of the customer journey, Dynamic Yield stands as a trusted and forward-thinking solution.
7. Constructor.io

Constructor.io has rapidly established itself as one of the most impactful and sophisticated recommendation engines in 2025, particularly within the enterprise e-commerce sector. Designed to power intelligent, hyper-personalized product discovery, the platform is distinguished by its ability to tailor search and recommendation experiences that are aligned with both user intent and business performance goals.
At the heart of Constructor.io’s innovation is its machine learning-driven relevance engine, which leverages real-time behavioral signals—such as clickstream data, purchase intent, and browsing patterns—to optimize product rankings based not only on relevance, but also on product attractiveness and potential revenue impact. Rather than simply returning items that match a search query, Constructor’s AI evaluates which products are most likely to convert and contribute to metrics like revenue per visitor (RPV), average order value (AOV), and conversion rate.
One of the key differentiators of Constructor.io is its merchandiser-centric design philosophy. The platform includes intuitive tools and business rule interfaces that empower merchandisers to customize recommendation strategies without heavy reliance on engineering teams. Whether optimizing for profitability, inventory velocity, or seasonal promotions, retail teams have granular control over how recommendations are shaped and deployed across digital storefronts.
Constructor.io supports a diverse array of recommendation logics, including complementary items, frequently bought together bundles, alternative products, trending bestsellers, and personalized suggestions. These recommendation types are dynamically optimized through continuous learning models that adapt in real time to shifting user behavior and market trends.
Its growing adoption across prominent e-commerce brands is a testament to the platform’s ability to drive measurable outcomes. Case studies from global retailers like White Stuff and Princess Auto have reported impressive uplifts in key metrics—such as substantial increases in conversion rates, CTR, and overall sales performance—after deploying Constructor.io’s solutions.
The platform’s performance is further validated by its robust A/B testing framework, which enables data-driven optimization and iterative refinement of recommendation logic. Retailers can test different approaches across audience segments and measure precise business impact, enhancing the ROI of their personalization efforts.
Positioned as a premium offering, Constructor.io follows a custom pricing model tailored to enterprise-level needs, factoring in catalog size, traffic volume, and desired feature set. This ensures that pricing aligns with expected value delivery and scalability requirements for large-scale digital commerce operations.
With its focus on combining cutting-edge AI with user-friendly merchandising controls, Constructor.io has become a preferred choice for e-commerce companies seeking to convert intent into revenue while delivering a personalized, engaging shopping journey. Its ability to unify relevance, personalization, and business strategy makes it a standout solution among the top recommendation engines of 2025.
8. Bloomreach

In 2025, Bloomreach has solidified its reputation as one of the most advanced and forward-looking recommendation engines in the market, offering a dynamic, AI-driven personalization ecosystem tailored for the demands of modern digital commerce. At the core of its offering lies the agentic personalization platform, an innovation that empowers businesses to automate customer experiences across the entire journey—from search and product discovery to marketing and conversion—through the power of Loomi AI, Bloomreach’s proprietary artificial intelligence engine.
This platform is purpose-built to deliver autonomous search, conversational commerce, and self-optimizing marketing campaigns, making it particularly effective for enterprises seeking scalable, real-time personalization across digital channels. Bloomreach’s intelligent system continuously interprets user behavior, preferences, and context to adapt content, product suggestions, and engagement strategies on the fly—thereby enhancing both user satisfaction and business outcomes.
One of Bloomreach’s defining strengths is its unified data architecture, which seamlessly integrates customer profiles, behavioral insights, and product data into a single operational layer. This foundation enables businesses to deploy a range of personalization models, from traditional rule-based recommendations to advanced AI-powered algorithms, including collaborative filtering, natural language processing, and machine learning-enhanced personalization tactics.
The versatility of Bloomreach makes it highly effective across a wide range of industries, including retail, financial services, travel, and hospitality. Its ability to tailor recommendations based on unique industry needs has contributed to its growing adoption among global brands and earned it recognition as a market leader in personalization platforms.
Performance measurement in Bloomreach is rigorously data-driven, with metrics such as revenue per visitor (RPV), average order value (AOV), conversion rate, click-through rate (CTR), and customer engagement playing central roles. The platform also includes robust A/B and multivariate testing capabilities, allowing marketing and e-commerce teams to iterate and refine personalization strategies with statistical confidence.
Bloomreach follows a customized pricing model, which scales based on factors like the number of active users, catalog size, and event volume. This enterprise-tier approach ensures flexibility and value alignment for businesses of all sizes, particularly those with complex digital infrastructure and personalization needs.
Real-world results underscore the platform’s impact. Notable case studies include a 27% lift in conversion rates for Terno, a Central European grocery retailer, and an 8.4% increase in RPV for Jumbo Supermarkten, a leading supermarket chain in the Netherlands. These examples highlight Bloomreach’s proven ability to turn advanced AI capabilities into measurable revenue gains.
With its cutting-edge automation, data-centric personalization, and strategic emphasis on omnichannel customer journeys, Bloomreach has positioned itself as an indispensable tool for digital-first enterprises. Its inclusion in the top 10 recommendation engines of 2025 is a reflection of both its technological innovation and its demonstrable impact on business performance.
9. Salesforce Einstein Recommendations

Salesforce Einstein Recommendations stands out in 2025 as one of the most robust and intelligent recommendation engines, seamlessly embedded within the broader Salesforce ecosystem. Developed to elevate digital engagement strategies, this AI-powered solution harnesses the depth of Salesforce’s data architecture to deliver highly personalized and contextually relevant recommendations across channels.
At its core, Einstein Recommendations leverages machine learning and predictive analytics to analyze customer behavior, historical interactions, preferences, and real-time signals. What distinguishes this platform is its tight integration with Salesforce CRM, Marketing Cloud, Commerce Cloud, and Service Cloud, enabling businesses to deploy consistent, personalized experiences across the entire customer journey—from email and web to mobile apps and customer service interactions.
One of the defining capabilities of Einstein Recommendations is its flexibility in what can be recommended. Whether it’s products, services, content, or offers, the system can dynamically suggest the most relevant option to any individual user based on their unique data profile. This empowers enterprises to go beyond traditional commerce personalization and extend AI-driven decision-making across touchpoints such as onboarding flows, upsell/cross-sell programs, support interactions, and digital campaigns.
Serving a wide array of industries—including retail, e-commerce, financial services, telecommunications, and healthcare—Salesforce Einstein Recommendations benefits from Salesforce’s dominant position in the global CRM market. The engine is particularly well-suited for enterprises that already operate within the Salesforce environment, offering native integration, streamlined data flow, and centralized customer insights without the need for complex third-party implementation.
Performance and ROI are measured through comprehensive business impact metrics, including conversion rates, average order value (AOV), customer lifetime value (CLV), and overall customer satisfaction scores (CSAT). Additionally, the platform enhances operational efficiency by automating decision-making processes that would otherwise require manual analysis and intervention.
Salesforce offers this AI recommendation capability through various pricing tiers—Enterprise, Performance, and Unlimited Editions—which are designed to meet the needs of organizations of varying sizes and digital maturity levels. These editions allow customers to scale personalization initiatives in alignment with their broader Salesforce investments.
Numerous case studies have demonstrated the tangible impact of Einstein Recommendations. Companies using the platform report significant uplifts in conversion rates, user engagement, and customer retention, alongside marked improvements in personalization-driven sales and marketing effectiveness. These outcomes validate Salesforce Einstein’s position as a trusted and scalable personalization engine for enterprise-grade applications.
As businesses increasingly prioritize intelligent automation and omnichannel personalization, Salesforce Einstein Recommendations continues to be a go-to solution for organizations seeking AI-powered insights, operational agility, and customer-centric innovation. Its comprehensive integration capabilities and measurable business value make it a deserving inclusion in the list of the Top 10 Best Recommendation Engines in 2025.
10. Recombee

Recombee has solidified its place in the competitive landscape of recommendation engines in 2025 as a highly versatile SaaS platform offering real-time content and product recommendations through an intuitive API. Designed to be flexible and customizable, Recombee serves a wide range of industries, offering a tailored approach to personalization and user engagement. While its market share may be smaller compared to larger industry leaders, Recombee’s robust features and adaptability make it a standout option for businesses seeking a scalable recommendation solution.
A defining strength of Recombee lies in its ability to deliver real-time recommendations. By leveraging AI and machine learning algorithms, the platform is capable of processing user actions in real-time and offering personalized suggestions that are both relevant and timely. This ensures that businesses can respond to user needs with precision, optimizing the customer experience at every touchpoint. Whether for e-commerce, content delivery, or media, Recombee’s engine adapts to diverse business requirements, making it a highly flexible tool for companies in search of tailored recommendation solutions.
The platform supports various recommendation strategies, such as collaborative filtering, content-based recommendations, and hybrid approaches, allowing businesses to select the method that best fits their objectives. Whether users are looking for complementary products, popular items, or newly released content, Recombee can generate personalized recommendations that maximize engagement and drive conversions. Its customizable engineempowers businesses to tweak and adjust recommendations based on specific KPIs and goals, offering a highly dynamic and user-centric solution.
Recombee has successfully positioned itself as a versatile tool for companies across diverse sectors, ranging from e-commerce to entertainment, and even education and finance. The solution’s wide applicability, combined with its focus on real-time data processing, makes it suitable for businesses of varying sizes that require a highly adaptable recommendation system. Despite its smaller presence compared to industry giants, Recombee has earned a reputation for delivering consistent performance and outstanding customer support, setting it apart in the market.
The engine’s effectiveness is evaluated through key performance indicators (KPIs) such as click-through rates (CTR)and conversion rates, both of which showcase the platform’s ability to drive tangible business results. Additionally, its SaaS-based pricing model offers scalability, allowing businesses to access and integrate Recombee’s powerful recommendation system without the burden of complex installations or infrastructure. The platform’s pricing is structured to reflect its customizable nature, catering to businesses that require a recommendation engine that can be fine-tuned to meet specific demands.
Numerous case studies highlight Recombee’s success in delivering increased engagement, higher conversion rates, and improved customer satisfaction. The platform’s combination of real-time recommendations, powerful machine learning capabilities, and an intuitive API make it a top contender in the recommendation engine market for 2025. Its focus on flexibility, exceptional customer support, and a high degree of customization ensure that it remains a valuable tool for businesses seeking to enhance their personalization efforts.
In conclusion, Recombee’s position as one of the Top 10 Best Recommendation Engines in 2025 is reinforced by its ability to deliver tailored, real-time suggestions across multiple domains, offering businesses a scalable, flexible, and efficient solution for enhancing user engagement and driving conversion rates. Its growing reputation in the industry, combined with its powerful AI-driven engine and customer-first approach, makes Recombee a significant player in the field of personalization technology.
Conclusion
In 2025, the landscape of recommendation engines has become more dynamic and essential than ever. As businesses continue to prioritize customer-centric experiences, the role of AI-powered recommendation systems in driving personalization and engagement has never been more critical. The Top 10 Best Recommendation Engines in 2025showcase a diverse range of platforms that cater to different industry needs, each with its own unique set of features, capabilities, and performance metrics. These engines are not only enhancing user experiences but also contributing significantly to business growth through improved conversion rates, increased customer satisfaction, and optimized revenue per visitor.
From Amazon Personalize to Recombee, these engines are leveraging the latest in artificial intelligence and machine learning technologies to process vast amounts of data in real-time, ensuring that businesses can offer hyper-personalized experiences at scale. Whether it’s delivering product recommendations based on user behavior, content preferences, or previous purchases, these systems utilize advanced algorithms such as collaborative filtering, content-based filtering, and deep learning to ensure that every suggestion is relevant, timely, and impactful.
One of the key trends we see across the top recommendation engines is the integration of real-time decision-making. This allows businesses to respond instantly to user actions, delivering personalized experiences in the moment, which is especially crucial in industries such as e-commerce, media, and entertainment. Engines like Google Cloud Recommendation AI and Microsoft Azure Personalizer excel in offering scalable, low-latency solutions that enable businesses to process data and make recommendations in real time, ensuring a seamless user journey and improved engagement rates.
Another standout feature is the customizability offered by many of these engines. Platforms such as Dynamic Yieldand Recombee provide businesses with the flexibility to tailor recommendation models to suit their specific needs. This level of customization allows companies to create unique customer experiences that reflect their brand identity and business goals. Whether it’s selecting specific recommendation strategies, adjusting algorithms to prioritize certain KPIs, or integrating recommendation engines with existing systems, the ability to customize recommendations ensures that businesses can fine-tune their approach for optimal results.
The ability to integrate seamlessly with existing systems is another essential feature of the best recommendation engines in 2025. Engines like Salesforce Einstein Recommendations and SAP Emarsys provide easy-to-use APIs and integration capabilities with widely used enterprise platforms. This ensures that businesses can implement recommendation engines without significant disruption to their operations. Moreover, these engines are designed to scale with business growth, making them ideal solutions for both small enterprises and large corporations.
One of the key performance indicators for evaluating the effectiveness of these systems is conversion rate. As seen with case studies from companies such as Razer and Dress the Population, the best recommendation engines can significantly boost conversion rates by presenting customers with products or content they are most likely to engage with. In some cases, this has led to impressive results such as a 250% uplift in sales and increased revenue per visit, proving that well-implemented recommendation systems are not just a tool for enhancing the user experience but also a powerful driver of revenue growth.
Furthermore, the data-driven insights provided by these engines are invaluable for businesses looking to optimize their marketing and sales strategies. By analyzing customer behavior and interactions in-depth, companies can uncover trends, preferences, and pain points that can inform their broader strategy. The predictive capabilities of recommendation engines help businesses stay ahead of the curve, enabling them to anticipate user needs and provide relevant offers before the customer even asks.
Looking towards the future, the role of AI and machine learning in recommendation engines will continue to evolve. Innovations in natural language processing, deep learning, and context-aware recommendations will further enhance the personalization capabilities of these systems, leading to even more tailored and intuitive experiences. As businesses continue to collect vast amounts of data, the potential for hyper-personalized recommendations will only grow, offering more opportunities to drive customer loyalty and satisfaction.
In conclusion, the Top 10 Best Recommendation Engines in 2025 represent the pinnacle of personalization technology, each offering a unique set of features designed to meet the needs of diverse industries. Whether it’s enhancing the user experience with intelligent recommendations, optimizing conversion rates, or providing valuable insights for business strategy, these platforms are instrumental in shaping the future of personalization. As AI continues to advance, these engines will only become more sophisticated, helping businesses provide more relevant, timely, and engaging experiences for their customers. For businesses looking to stay competitive and drive growth in 2025 and beyond, investing in a cutting-edge recommendation engine is no longer optional—it’s a necessity.
If you find this article useful, why not share it with your hiring manager and C-level suite friends and also leave a nice comment below?
We, at the 9cv9 Research Team, strive to bring the latest and most meaningful data, guides, and statistics to your doorstep.
To get access to top-quality guides, click over to 9cv9 Blog.
People Also Ask
What are recommendation engines?
Recommendation engines use algorithms to suggest products, services, or content based on user preferences, behaviors, or historical data, improving personalization and driving engagement.
Why are recommendation engines important for businesses in 2025?
Recommendation engines help businesses increase user engagement, boost conversion rates, and enhance customer satisfaction by delivering tailored suggestions, making them essential in 2025 for staying competitive.
What is the role of AI in recommendation engines?
AI in recommendation engines allows for real-time personalization, improved accuracy, and better predictions of user preferences by analyzing vast amounts of data through machine learning algorithms.
How do recommendation engines improve customer experience?
By analyzing customer behaviors and preferences, recommendation engines provide personalized suggestions that improve satisfaction, making the shopping or content discovery process easier and more enjoyable for users.
What industries benefit from recommendation engines in 2025?
Recommendation engines benefit a wide range of industries, including e-commerce, entertainment, retail, healthcare, and finance, by delivering tailored experiences and increasing customer engagement across multiple touchpoints.
How do recommendation engines increase conversion rates?
By suggesting relevant products or content based on customer preferences, recommendation engines drive more targeted interactions, leading to higher chances of conversion, increased average order value, and repeat business.
What are the key features of top recommendation engines in 2025?
Top recommendation engines offer real-time suggestions, seamless integration, AI-driven algorithms, A/B testing capabilities, and robust performance metrics to improve personalization and enhance user engagement.
Which recommendation engine is best for e-commerce?
Amazon Personalize and Dynamic Yield are among the top choices for e-commerce, as they leverage AI to provide personalized product suggestions and enhance the shopping experience, increasing conversion rates and revenue.
How does machine learning improve recommendation engines?
Machine learning helps recommendation engines learn from user interactions and continuously refine their algorithms to provide more accurate, personalized, and relevant recommendations over time.
What is the difference between collaborative and content-based filtering in recommendation engines?
Collaborative filtering recommends items based on user similarities, while content-based filtering suggests items based on the attributes of the items themselves, offering complementary approaches to personalization.
How can businesses measure the success of recommendation engines?
Success is measured through key performance indicators (KPIs) like conversion rates, click-through rates, average order value (AOV), customer retention, and overall revenue, reflecting how well the engine drives business outcomes.
What is real-time personalization in recommendation engines?
Real-time personalization tailors recommendations instantly based on the current behavior or preferences of users, ensuring that suggestions remain relevant and timely, improving user engagement and satisfaction.
How do recommendation engines work with big data?
Recommendation engines analyze vast datasets, such as user behavior, transaction history, and preferences, using advanced algorithms to generate personalized suggestions and insights at scale.
What is the future of recommendation engines in 2025?
The future of recommendation engines in 2025 will be dominated by more advanced AI techniques, deeper personalization, and seamless omnichannel integration, allowing businesses to deliver highly targeted and engaging user experiences.
What is the role of A/B testing in recommendation engines?
A/B testing allows businesses to test different recommendation strategies, compare their effectiveness, and optimize personalization by analyzing which version performs better in driving user engagement and conversions.
Are recommendation engines suitable for small businesses?
Yes, many recommendation engines offer scalable solutions suitable for small businesses, enabling them to enhance customer engagement and increase sales through personalized product or content suggestions without heavy upfront costs.
How do recommendation engines handle customer data privacy?
Top recommendation engines comply with data protection regulations such as GDPR and CCPA, ensuring that user data is handled securely and only used to improve personalization while maintaining user privacy.
Can recommendation engines improve content discovery in media and entertainment?
Yes, recommendation engines are widely used in media and entertainment platforms to suggest movies, shows, and music based on user preferences, increasing engagement and providing a personalized content experience.
How do recommendation engines drive customer loyalty?
By consistently delivering personalized experiences and relevant suggestions, recommendation engines help businesses build trust and loyalty with their customers, leading to repeat visits and sustained relationships.
What is the cost of implementing a recommendation engine?
The cost varies depending on the platform, features, and business size. Custom pricing models are often available, with enterprise-level solutions potentially costing tens of thousands annually, while smaller solutions may have lower fees.
How do recommendation engines impact SEO?
By increasing user engagement and reducing bounce rates through personalized content and product suggestions, recommendation engines can indirectly improve SEO, as more interaction signals to search engines that users find the content valuable.
What types of recommendation algorithms are used in 2025?
In 2025, recommendation engines use algorithms like collaborative filtering, content-based filtering, hybrid models, reinforcement learning, and deep learning to provide more accurate and personalized suggestions.
How do recommendation engines affect revenue growth?
By increasing conversion rates, boosting average order value (AOV), and driving repeat purchases, recommendation engines have a direct impact on revenue growth, helping businesses maximize their return on investment.
Are recommendation engines effective for B2B businesses?
Yes, recommendation engines can be effective in B2B settings by suggesting relevant products, services, or content to business clients, improving engagement and helping businesses foster stronger relationships with their partners.
What is the best recommendation engine for media and entertainment?
Google Cloud Recommendation AI and Bloomreach are highly effective for media and entertainment industries, delivering personalized content recommendations based on user behavior and preferences.
Can recommendation engines be integrated with other tools?
Yes, many recommendation engines are designed to integrate seamlessly with other marketing, CRM, and analytics tools, allowing businesses to create a cohesive, personalized experience across multiple platforms.
How do recommendation engines affect user engagement?
Recommendation engines enhance user engagement by delivering highly relevant content or product suggestions, encouraging users to spend more time on the platform and interact more frequently with the content or offerings.
How do recommendation engines improve website navigation?
By providing personalized navigation and suggestions based on user behavior, recommendation engines make it easier for customers to find relevant content or products, improving the overall user experience and increasing time spent on the site.
What is the difference between rule-based and AI-powered recommendation engines?
Rule-based recommendation engines follow predefined rules for making suggestions, while AI-powered engines use machine learning to continuously adapt and improve recommendations based on real-time user data and behaviors.
What is the impact of recommendation engines on customer satisfaction?
By offering personalized and relevant suggestions, recommendation engines increase customer satisfaction, as users feel understood and more likely to discover products or content that meets their needs.
Can recommendation engines be used for mobile apps?
Yes, many recommendation engines are optimized for mobile apps, providing personalized recommendations in real-time based on user behavior, preferences, and in-app interactions to enhance the mobile user experience.
Do recommendation engines require a lot of data to be effective?
While having more data helps, many recommendation engines are designed to work effectively even with limited data by using algorithms that can analyze smaller datasets and still provide relevant suggestions.
How do recommendation engines improve content monetization?
Recommendation engines can help improve content monetization by driving more user engagement and increasing the likelihood of users interacting with ads, subscriptions, or premium content, ultimately increasing revenue.
What are some common challenges with recommendation engines?
Challenges include data privacy concerns, the complexity of integrating recommendation systems with existing platforms, the need for high-quality data, and managing the accuracy of suggestions to avoid overwhelming users.