Key Takeaways
- Understand Key Roles: Learn the essential roles within a data science team—from data scientists to machine learning engineers—and how each contributes to transforming data into actionable insights.
- Build Collaborative Teams: Effective data science teams require a balance of technical and analytical skills, alongside strong communication to align with business goals.
- Overcome Management Challenges: Addressing challenges like role alignment, project prioritization, and continuous upskilling is essential for maximizing a data science team’s impact.
In today’s data-driven landscape, businesses that effectively harness the power of data gain a strategic edge, enabling them to make informed decisions, streamline processes, and innovate faster. From improving customer experiences to forecasting market trends, data science has become the backbone of modern enterprises. But as data-driven demands increase, so does the complexity of building a successful data science team. A well-rounded team isn’t just a group of data experts; it’s a diverse assembly of professionals, each bringing unique skills and expertise to address different facets of data science projects. Understanding the essential roles within a data science team is crucial for organizations aiming to stay competitive in an evolving market.
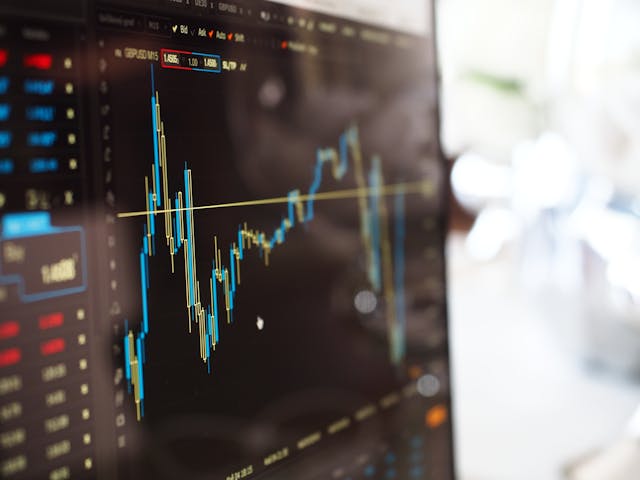
A data science team combines technical acumen, analytical prowess, and business insight to turn raw data into actionable insights. However, effective data science teams consist of more than just data scientists. Successful teams include data engineers who ensure data reliability, data analysts who bring clarity to data insights, and machine learning engineers who operationalize models into production environments. Additionally, there are architects, statisticians, and product managers, each playing a vital part in designing, analyzing, and implementing data solutions. This blend of roles ensures that data pipelines are optimized, insights are meaningful, and predictive models are effectively implemented.
Each role in a data science team carries specific responsibilities, skill sets, and collaborative needs. For example, data scientists focus on complex analyses and model development, while data engineers build the infrastructure that makes such analyses possible. Business intelligence analysts transform technical findings into strategies that align with business goals, creating a bridge between raw data and actionable business decisions. This intricate ecosystem of roles highlights the need for collaboration and clarity in responsibilities to produce reliable, impactful results.
This guide will take a deep dive into the essential roles within a data science team, exploring the unique contributions each position makes. By understanding these roles and how they work together, organizations can better structure their data teams to achieve maximum efficiency, accuracy, and innovation. Whether you’re a business leader aiming to strengthen your company’s data capabilities or an aspiring data professional seeking insight into career paths, this comprehensive guide will provide a roadmap to building and maintaining a top-tier data science team.
Before we venture further into this article, we would like to share who we are and what we do.
About 9cv9
9cv9 is a business tech startup based in Singapore and Asia, with a strong presence all over the world.
With over eight years of startup and business experience, and being highly involved in connecting with thousands of companies and startups, the 9cv9 team has listed some important learning points in this overview of Essential Roles in a Data Science Team.
If your company needs recruitment and headhunting services to hire top-quality employees, you can use 9cv9 headhunting and recruitment services to hire top talents and candidates. Find out more here, or send over an email to [email protected].
Or just post 1 free job posting here at 9cv9 Hiring Portal in under 10 minutes.
Essential Roles in a Data Science Team: A Comprehensive Guide
- Why Every Data-Driven Company Needs a Data Science Team
- Key Roles in a Data Science Team: Overview
- Data Scientist
- Data Engineer
- Data Analyst
- Machine Learning Engineer
- Data Architect
- Business Intelligence (BI) Analyst
- Statistician
- Data Product Manager
- Data Analyst vs. Data Scientist: Understanding the Differences
- Key Skills for Success in a Data Science Team
- Building a Successful Data Science Team: Tips and Best Practices
- Challenges in Managing a Data Science Team
1. Why Every Data-Driven Company Needs a Data Science Team
In a world where data is at the heart of every successful business decision, companies that fail to leverage data effectively risk falling behind. A dedicated data science team is the engine that enables organizations to turn raw data into actionable insights, ensuring competitive advantage and sustainable growth. The following sections outline why data science teams are essential for data-driven companies, highlighting specific examples and applications where their contributions lead to tangible results.
1. Transforming Data into Strategic Insights
- Bridging Data and Decision-Making: Data science teams enable companies to make evidence-based decisions by:
- Analyzing vast datasets to reveal trends and correlations that aren’t immediately visible
- Providing insights that guide business strategies, such as identifying new market opportunities or optimizing existing processes
- Example: A retail company uses its data science team to analyze customer purchase histories and seasonality, allowing it to forecast demand and adjust inventory proactively.
- Improving Forecasting Accuracy:
- Data scientists use advanced algorithms to improve the accuracy of predictions, enabling better planning and resource allocation.
- This capability is critical in sectors like finance, where precise forecasting can minimize risk.
- Example: Financial firms leverage data science teams to predict stock trends and guide investment strategies based on historical data and market analysis.
- Enhanced Competitiveness Through Innovation:
- By identifying emerging trends and consumer behaviors, data science teams position companies to innovate and adapt swiftly.
- This innovation can take the form of new product development, enhanced customer experiences, or optimized supply chains.
- Example: Netflix’s data science team helps personalize recommendations based on viewer history, resulting in higher engagement and retention rates.
2. Enhancing Customer Experiences with Data-Driven Personalization
- Creating Tailored Customer Journeys:
- Data scientists analyze customer behavior to create personalized experiences, which drive satisfaction and loyalty.
- They use techniques like clustering and segmentation to categorize customers by preferences, location, or purchase history.
- Example: Amazon’s recommendation system, powered by its data science team, customizes product suggestions for each user, which increases cross-selling opportunities and sales.
- Real-Time Customer Feedback and Insights:
- Real-time data collection allows companies to monitor customer sentiment and respond to issues promptly, improving service quality.
- Sentiment analysis tools help data science teams gauge customer satisfaction from social media and other online reviews.
- Example: Airlines often use data science to monitor social media for feedback on delays or service issues, allowing them to address complaints proactively.
- Predictive Models for Customer Retention:
- Data science teams can build predictive models that identify customers likely to churn, enabling preemptive action to retain them.
- These models often incorporate factors such as purchase frequency, product usage, and customer feedback.
- Example: Telecom companies analyze customer usage patterns to offer targeted incentives or personalized plans to reduce churn rates.
3. Optimizing Operational Efficiency
- Streamlining Supply Chains:
- Data science teams analyze supply chain data to identify inefficiencies, reduce bottlenecks, and optimize logistics.
- By forecasting demand more accurately, they help reduce stock shortages and overstocking issues.
- Example: Walmart’s data science team uses predictive analytics to forecast demand and adjust its inventory and supply chain processes accordingly, cutting down on excess stock and improving turnover.
- Automation of Repetitive Tasks:
- Data scientists use machine learning algorithms to automate routine tasks, reducing the workload on employees and improving overall efficiency.
- Common applications include automating customer support through chatbots or using predictive maintenance in manufacturing.
- Example: Manufacturing companies use predictive maintenance algorithms developed by data scientists to monitor equipment, reducing unexpected breakdowns and saving repair costs.
- Enhanced Decision-Making in Resource Allocation:
- Data science teams use optimization models to determine the best allocation of resources based on data-driven insights.
- This includes everything from workforce planning to budgeting decisions.
- Example: Healthcare providers leverage data to optimize staffing levels, ensuring the right number of healthcare professionals are available based on predicted patient inflow.
4. Strengthening Risk Management and Fraud Detection
- Identifying Potential Risks Proactively:
- Data science teams develop predictive models that help companies identify and mitigate risks before they impact the business.
- They analyze past data to predict trends in various risk factors, from operational to market-related risks.
- Example: Insurance companies rely on data scientists to assess the risk of policyholders based on historical claims data, enabling accurate pricing and premium adjustments.
- Fraud Detection through Machine Learning:
- By analyzing transaction data, data scientists can detect unusual patterns indicative of fraud.
- Fraud detection models use machine learning algorithms to identify potential fraud in real-time, reducing financial losses.
- Example: Banks use fraud detection algorithms to flag suspicious credit card transactions, preventing fraudulent activities and improving customer security.
- Developing Data Security Protocols:
- Data science teams also contribute to cybersecurity by analyzing network patterns and identifying security vulnerabilities.
- Using anomaly detection techniques, they can spot irregular activities and reinforce defenses.
- Example: Large corporations implement data security protocols advised by data scientists, reducing the risk of data breaches and safeguarding customer information.
5. Facilitating Continuous Business Innovation
- Creating Data-Driven Products:
- Many data-driven companies develop new products and services based on data insights, such as recommendation systems, personalized experiences, and intelligent automation tools.
- These products not only meet current customer needs but also adapt to future demands.
- Example: Spotify’s data science team developed its “Discover Weekly” playlist, an innovative product that uses algorithms to recommend songs based on listening habits, enhancing user engagement.
- Experimentation and Rapid Prototyping:
- Data science teams allow companies to experiment with new ideas through rapid prototyping and A/B testing, measuring success based on data before full-scale implementation.
- This data-driven experimentation enables companies to test ideas with minimal risk.
- Example: E-commerce platforms like Etsy run A/B tests through their data science teams to determine which product recommendations or page layouts drive the most sales.
- Predicting Market Trends and Consumer Demand:
- Data science teams are instrumental in anticipating future market shifts and consumer preferences, helping companies stay ahead of competitors.
- Predictive analytics enables businesses to refine their offerings, ensuring they meet emerging trends and customer needs.
- Example: Fashion retailers analyze customer purchase data to identify upcoming style trends, allowing them to tailor inventory for the next season.
6. Supporting Strategic Business Decisions
- Data-Driven Business Strategy:
- Data science teams provide strategic insights that guide business direction, from expanding into new markets to scaling existing operations.
- Their insights often shape long-term strategies by predicting potential revenue streams and profitability.
- Example: Global companies rely on data science insights to decide which markets offer the highest growth potential, tailoring marketing and investment strategies accordingly.
- Enhanced Product Development:
- By analyzing user feedback and behavior data, data science teams help companies refine existing products or develop new offerings that better meet customer needs.
- This data-centric approach ensures that products are continuously improving in alignment with market demands.
- Example: Mobile app companies utilize user data analysis to identify popular features, which are then enhanced or expanded in future updates.
- Cross-Departmental Insights for Cohesion:
- Data science teams provide insights that connect various departments, ensuring that all parts of the organization are aligned with overall goals.
- Marketing, sales, product, and customer service teams can all benefit from data-informed guidance, creating a cohesive business strategy.
- Example: By working with both the marketing and product teams, a data science team might identify a feature driving customer engagement, leading to more targeted marketing campaigns and product updates.
In today’s rapidly changing digital landscape, the need for a data science team within any data-driven company is more apparent than ever. These teams enable businesses to stay agile, innovate continuously, and make strategic decisions rooted in data. From providing insights that transform customer experiences to ensuring operational efficiency, data science teams drive value across the organization, empowering businesses to not only meet current demands but to anticipate and adapt to future ones. Investing in a skilled data science team is no longer optional—it is essential for companies aiming to lead in a data-first world.
2. Key Roles in a Data Science Team: Overview
Building a successful data science team involves more than just hiring data scientists; it requires a diverse group of professionals with complementary skills to handle different aspects of data collection, analysis, and implementation. Each role is specialized, contributing unique expertise that together enables the team to generate valuable insights, streamline processes, and develop data-driven solutions. In this section, we’ll explore the essential roles in a data science team, explaining their responsibilities, skills, and contributions, with examples to illustrate their impact.
1. Data Scientist
- Primary Responsibilities:
- Designs and develops machine learning models to extract actionable insights from data.
- Analyzes structured and unstructured data to discover patterns and trends.
- Collaborates with stakeholders to translate business goals into data-driven solutions.
- Key Skills:
- Proficiency in programming languages like Python and R, with expertise in machine learning frameworks (e.g., TensorFlow, PyTorch).
- Strong foundation in statistics, data visualization, and data mining techniques.
- Example:
- In a healthcare setting, a data scientist might develop a model to predict patient readmission rates, helping hospitals allocate resources effectively and improve patient care.
2. Data Engineer
- Primary Responsibilities:
- Builds and maintains data pipelines and infrastructure for data storage, processing, and retrieval.
- Ensures data integrity and reliability across different sources and formats.
- Collaborates with data scientists to ensure data availability and scalability for machine learning models.
- Key Skills:
- Expertise in ETL (Extract, Transform, Load) processes, data warehousing, and databases (e.g., SQL, NoSQL).
- Proficiency in big data technologies like Hadoop, Spark, and cloud platforms (AWS, Google Cloud, Azure).
- Example:
- In a retail company, data engineers set up systems to capture, clean, and store transactional data, enabling data scientists to analyze purchase behaviors and optimize product recommendations.
3. Data Analyst
- Primary Responsibilities:
- Performs data cleaning, processing, and analysis to generate insights that support decision-making.
- Creates reports, dashboards, and visualizations to make data insights accessible to non-technical stakeholders.
- Conducts exploratory data analysis (EDA) to understand trends, patterns, and correlations.
- Key Skills:
- Proficiency in data visualization tools (e.g., Tableau, Power BI) and analytical software (e.g., SQL, Excel).
- Strong ability to interpret data and communicate findings effectively to different departments.
- Example:
- A data analyst in a financial institution may create dashboards to track real-time metrics, such as daily transactions and customer engagement, enabling quick adjustments to marketing strategies.
4. Machine Learning Engineer
- Primary Responsibilities:
- Develops, tests, and deploys machine learning models into production environments.
- Optimizes models for scalability and performance, ensuring they can handle real-time data processing.
- Works closely with software engineers to integrate machine learning models into applications.
- Key Skills:
- Knowledge of ML lifecycle management, model versioning, and tools like Docker for model deployment.
- Experience with machine learning frameworks (e.g., Scikit-Learn, Keras) and software engineering skills.
- Example:
- In e-commerce, machine learning engineers may develop recommendation engines that personalize product suggestions, integrating the model directly into the website for real-time user interaction.
5. Data Architect
- Primary Responsibilities:
- Designs the overall data architecture and data strategy for the organization.
- Determines the appropriate technology stack and data management systems for efficient data flow.
- Ensures compliance with data governance standards, including data privacy and security.
- Key Skills:
- Expertise in data modeling, database design, and enterprise architecture frameworks.
- Familiarity with big data tools and cloud infrastructure to support large-scale data systems.
- Example:
- A data architect at a multinational company may design a global data framework that allows for seamless data sharing and integration across regions while adhering to local data regulations.
6. Business Intelligence (BI) Analyst
- Primary Responsibilities:
- Converts complex data insights into understandable business intelligence that guides decision-making.
- Builds and maintains dashboards and reporting tools for executive and departmental use.
- Analyzes key performance indicators (KPIs) to assess the effectiveness of strategies and initiatives.
- Key Skills:
- Proficiency in BI tools like Power BI, Looker, or Tableau, and solid SQL skills.
- Strong analytical abilities to interpret data trends and translate them into business insights.
- Example:
- A BI analyst in a telecommunications company may analyze customer churn rates and usage data to recommend strategies that improve customer retention.
7. Statistician
- Primary Responsibilities:
- Conducts statistical analyses to validate hypotheses, ensuring data-driven conclusions.
- Assesses data quality and helps design experiments to collect data relevant to business objectives.
- Collaborates with data scientists on model validation and accuracy testing.
- Key Skills:
- Expertise in statistical software (e.g., SAS, SPSS) and advanced statistical methodologies.
- Strong analytical skills to interpret data distributions, variability, and patterns.
- Example:
- In pharmaceuticals, statisticians work on clinical trial data, ensuring that study results are statistically significant and guiding data collection protocols.
8. Product Manager
- Primary Responsibilities:
- Aligns data science projects with business goals, ensuring that data solutions address core business needs.
- Acts as a liaison between data science, engineering, and business teams, translating technical capabilities into actionable business plans.
- Defines project requirements, timelines, and metrics for success.
- Key Skills:
- Strong understanding of business strategy, data analytics, and project management.
- Ability to communicate technical details in a way that resonates with stakeholders.
- Example:
- A product manager in a tech startup might oversee the development of a data-driven app feature, coordinating with data scientists to ensure the feature enhances user experience and aligns with business goals.
9. Data Privacy and Security Specialist
- Primary Responsibilities:
- Ensures data compliance with regulatory requirements (e.g., GDPR, CCPA) to protect customer and organizational data.
- Monitors data access, implementing encryption, anonymization, and other data security protocols.
- Works with IT to detect and respond to data breaches and security vulnerabilities.
- Key Skills:
- Knowledge of data security practices, encryption techniques, and familiarity with legal requirements for data privacy.
- Proficiency with security tools and data governance frameworks.
- Example:
- A data privacy specialist at a healthcare provider ensures that patient data is stored securely and access is limited, minimizing the risk of breaches while maintaining regulatory compliance.
10. Data Governance Lead
- Primary Responsibilities:
- Develops and enforces data policies and standards to ensure data consistency and quality across the organization.
- Oversees data management processes, including data lineage and metadata management.
- Works with data stewards and stakeholders to address data quality issues and implement best practices.
- Key Skills:
- Deep understanding of data management, data quality, and compliance standards.
- Strong project management skills to coordinate data governance initiatives across departments.
- Example:
- In a financial services firm, the data governance lead ensures that customer and transaction data meet regulatory standards, enhancing data reliability and reducing risk exposure.
Together, these roles form the foundation of a high-performing data science team. By integrating these varied skill sets, companies can ensure that they are not only collecting data but transforming it into valuable insights that drive decision-making, foster innovation, and improve customer experience. Each position brings a unique perspective and set of skills, collectively enabling the data science team to tackle complex challenges and deliver business value.
3. Data Scientist
The role of a data scientist has gained tremendous prominence in recent years as organizations increasingly rely on data-driven strategies to remain competitive. A data scientist is a multifaceted professional who combines expertise in statistics, computer science, and domain knowledge to extract meaningful insights from vast amounts of data. This section delves deep into the responsibilities, essential skills, tools, and real-world applications of data scientists, illustrating why they are critical assets in today’s data-centric business environment.
Also, read our top guide on How to Hire a Data Scientist.
1. Primary Responsibilities of a Data Scientist
Data scientists perform a wide range of tasks that are essential for harnessing the power of data. Their responsibilities can be grouped into several key areas:
- Data Collection and Preparation:
- Gather data from various sources, including databases, APIs, and external datasets.
- Clean, preprocess, and transform raw data to ensure its quality and relevance for analysis.
- Exploratory Data Analysis (EDA):
- Analyze datasets to identify trends, patterns, and anomalies.
- Use statistical techniques to summarize the main characteristics of the data, often with visual aids like graphs and charts.
- Model Development:
- Design, develop, and validate predictive models using machine learning algorithms.
- Select appropriate modeling techniques based on the problem at hand (e.g., regression, classification, clustering).
- Deployment and Monitoring:
- Implement machine learning models into production systems for real-time data processing.
- Monitor model performance over time and make necessary adjustments to improve accuracy and efficiency.
- Communication and Collaboration:
- Present findings and insights to stakeholders in an understandable format.
- Collaborate with cross-functional teams, including data engineers, analysts, and product managers, to align data projects with business objectives.
2. Essential Skills for Data Scientists
Data scientists require a diverse skill set to effectively perform their roles. Key skills include:
- Statistical Analysis:
- Proficiency in statistical concepts such as hypothesis testing, probability distributions, and regression analysis.
- Ability to interpret statistical results and derive actionable insights.
- Programming Skills:
- Strong programming knowledge in languages such as Python, R, or SQL to manipulate data and implement algorithms.
- Familiarity with data manipulation libraries (e.g., Pandas, NumPy) and visualization tools (e.g., Matplotlib, Seaborn).
- Machine Learning:
- Understanding of various machine learning algorithms, including supervised and unsupervised learning techniques.
- Experience with frameworks like Scikit-Learn, TensorFlow, or PyTorch for model development.
- Data Visualization:
- Skills in creating visual representations of data to communicate complex findings effectively.
- Proficiency in visualization tools like Tableau, Power BI, or D3.js.
- Domain Knowledge:
- Familiarity with the specific industry or domain in which the data scientist operates (e.g., finance, healthcare, retail).
- Ability to contextualize data insights within the framework of business objectives.
3. Tools and Technologies Used by Data Scientists
Data scientists leverage various tools and technologies to perform their analyses and build models. Some commonly used tools include:
- Programming Languages:
- Python: Widely used for data analysis, machine learning, and visualization due to its simplicity and rich libraries.
- R: Preferred for statistical analysis and data visualization, especially in academic settings.
- Data Manipulation and Analysis Libraries:
- Pandas: A powerful library for data manipulation and analysis in Python.
- NumPy: Used for numerical computing and handling large multidimensional arrays and matrices.
- Machine Learning Frameworks:
- Scikit-Learn: A comprehensive library for implementing standard machine learning algorithms.
- TensorFlow: A flexible framework for building deep learning models.
- PyTorch: An open-source machine learning library that excels in tensor computations and deep learning.
- Data Visualization Tools:
- Tableau: A popular BI tool that allows for interactive data visualization and dashboard creation.
- Power BI: A Microsoft tool for business analytics, providing visualizations and sharing insights across organizations.
- Big Data Technologies:
- Hadoop: A framework for distributed storage and processing of large datasets.
- Spark: An open-source unified analytics engine for big data processing, known for its speed and ease of use.
4. Real-World Applications of Data Science
Data scientists apply their skills and knowledge across various industries, delivering solutions that drive efficiency, innovation, and profitability. Some notable examples include:
- Healthcare:
- Predictive Analytics: Data scientists develop models to predict patient outcomes, readmission rates, and disease outbreaks, aiding hospitals in resource allocation.
- Personalized Medicine: By analyzing genetic data, data scientists can assist in tailoring treatments to individual patients, improving health outcomes.
- Finance:
- Fraud Detection: Machine learning algorithms are employed to identify unusual transaction patterns that may indicate fraudulent activity, enabling financial institutions to act swiftly.
- Risk Assessment: Data scientists analyze market trends and consumer behavior to create models that evaluate credit risk, informing lending decisions.
- Retail:
- Customer Segmentation: Data scientists analyze purchasing behavior to segment customers into distinct groups, allowing for targeted marketing strategies.
- Inventory Optimization: By forecasting demand patterns, data scientists help retailers manage inventory levels efficiently, reducing waste and improving sales.
- Telecommunications:
- Churn Prediction: Data scientists analyze customer data to identify factors contributing to churn, allowing companies to implement retention strategies proactively.
- Network Optimization: Machine learning models help optimize network performance by predicting peak usage times and identifying potential service issues.
5. The Impact of Data Scientists on Business Outcomes
Data scientists play a pivotal role in transforming data into actionable insights that significantly impact business outcomes:
- Improved Decision-Making:
- By providing data-driven insights, data scientists enable organizations to make informed decisions that enhance efficiency and effectiveness.
- Enhanced Customer Experience:
- Through analysis of customer data, businesses can tailor their offerings and interactions, leading to improved customer satisfaction and loyalty.
- Increased Competitive Advantage:
- Organizations leveraging data science can identify emerging trends and opportunities, allowing them to stay ahead of competitors.
- Operational Efficiency:
- Data scientists streamline processes through automation and predictive modeling, reducing costs and improving productivity.
6. The Future of Data Science
As the field of data science continues to evolve, several trends are shaping its future:
- Automation of Data Processes:
- The rise of automated machine learning (AutoML) tools is making data science more accessible and efficient, enabling non-experts to harness the power of data.
- Focus on Ethics and Privacy:
- With increasing concerns over data privacy and ethical considerations in AI, data scientists will need to ensure their practices adhere to regulatory standards while maintaining transparency.
- Integration of Artificial Intelligence:
- Data scientists will increasingly work with AI technologies to enhance predictive analytics and decision-making processes, leading to more sophisticated solutions.
- Emphasis on Interdisciplinary Collaboration:
- The future of data science will involve closer collaboration between data scientists, domain experts, and business leaders to align data initiatives with organizational goals.
In conclusion, data scientists are essential players in the modern data landscape, driving innovation and transformation across various sectors. Their unique blend of technical skills, analytical thinking, and domain expertise enables organizations to leverage data as a strategic asset. By understanding the responsibilities, skills, tools, and applications associated with data scientists, businesses can appreciate the critical role these professionals play in shaping data-driven decision-making and fostering growth.
4. Data Engineer
Data engineers are crucial contributors to the data science ecosystem, focusing on the architecture, design, and maintenance of data systems and pipelines. Their primary role involves the preparation of data so that data scientists and analysts can perform their analyses efficiently. This section provides an in-depth overview of the responsibilities, essential skills, tools, and real-world applications of data engineers, illustrating their indispensable value in managing and optimizing data workflows.
Also, read our top guide on how to hire a top data engineer.
1. Primary Responsibilities of a Data Engineer
Data engineers handle various tasks that are fundamental to the effective flow and storage of data within an organization. Their responsibilities typically include the following key areas:
- Data Pipeline Development:
- Design, construct, and maintain robust data pipelines that facilitate the extraction, transformation, and loading (ETL) of data from various sources.
- Ensure data pipelines are efficient, reliable, and scalable to accommodate growing data volumes.
- Database Management:
- Develop and manage databases, both relational (SQL) and non-relational (NoSQL), to store and retrieve data efficiently.
- Optimize database performance and implement best practices for data storage and indexing.
- Data Modeling:
- Create data models that define the structure and organization of data within databases and data warehouses.
- Work closely with stakeholders to understand data requirements and translate them into scalable data models.
- Data Quality Assurance:
- Implement data quality checks to ensure the accuracy, completeness, and consistency of data throughout the pipeline.
- Monitor data flows and troubleshoot any issues that arise, maintaining high standards of data integrity.
- Collaboration with Data Teams:
- Collaborate with data scientists, analysts, and business stakeholders to understand their data needs and provide the necessary infrastructure.
- Participate in cross-functional teams to align data engineering efforts with business objectives.
2. Essential Skills for Data Engineers
Data engineers must possess a diverse skill set that enables them to design and manage complex data systems effectively. Key skills include:
- Programming Skills:
- Proficiency in programming languages such as Python, Java, or Scala for developing data processing applications.
- Experience with scripting languages (e.g., Bash) for automating data workflows.
- Database Technologies:
- Strong knowledge of relational databases (e.g., MySQL, PostgreSQL) and NoSQL databases (e.g., MongoDB, Cassandra) for data storage solutions.
- Familiarity with data warehousing solutions (e.g., Amazon Redshift, Google BigQuery) for analytical processing.
- ETL Tools and Frameworks:
- Experience with ETL tools (e.g., Apache NiFi, Talend) for data integration and processing.
- Understanding of frameworks like Apache Airflow for orchestrating complex data workflows.
- Big Data Technologies:
- Familiarity with big data processing frameworks such as Apache Hadoop and Apache Spark to handle large datasets.
- Knowledge of distributed computing concepts and cloud-based data storage solutions (e.g., AWS S3, Google Cloud Storage).
- Data Architecture and Modeling:
- Skills in designing data architectures that support data lakes, data warehouses, and real-time data processing.
- Ability to create efficient data models that optimize storage and retrieval.
3. Tools and Technologies Used by Data Engineers
Data engineers utilize a variety of tools and technologies to build and maintain data infrastructure. Some commonly used tools include:
- Programming Languages:
- Python: Widely used for data manipulation and ETL processes due to its rich libraries (e.g., Pandas, NumPy).
- Java/Scala: Often used in big data processing and distributed computing environments.
- Database Management Systems:
- MySQL/PostgreSQL: Popular relational database systems for structured data storage.
- MongoDB/Cassandra: Leading NoSQL databases designed for handling unstructured or semi-structured data.
- ETL and Data Integration Tools:
- Apache NiFi: A data flow automation tool that simplifies the movement of data between systems.
- Talend: An open-source ETL tool that provides a suite of data integration capabilities.
- Big Data Technologies:
- Apache Spark: A powerful engine for large-scale data processing that supports batch and streaming data.
- Hadoop: A framework that allows for the distributed storage and processing of large datasets across clusters.
- Cloud Platforms:
- AWS: Amazon Web Services offers a range of tools for data storage (S3), processing (EMR), and analytics (Redshift).
- Google Cloud Platform: Provides solutions like BigQuery for data warehousing and Dataflow for stream and batch data processing.
4. Real-World Applications of Data Engineering
Data engineers play a vital role across various industries, ensuring that organizations can effectively manage and leverage their data assets. Notable examples include:
- Healthcare:
- Patient Data Management: Data engineers develop systems to aggregate patient data from various sources, enabling healthcare providers to gain insights into patient care and outcomes.
- Real-Time Monitoring: Building pipelines that facilitate real-time data processing from wearable devices, allowing for timely interventions in patient health.
- Finance:
- Transaction Processing: Data engineers design systems that process large volumes of financial transactions in real time, ensuring data accuracy and compliance.
- Risk Management: Developing data architectures that enable financial institutions to assess risk by analyzing historical transaction data and market trends.
- E-Commerce:
- Recommendation Systems: Data engineers build data pipelines that collect and analyze customer behavior, enabling personalized product recommendations.
- Inventory Management: Creating systems that integrate sales data with inventory levels to optimize stock replenishment and reduce overstock.
- Telecommunications:
- Network Performance Analysis: Data engineers develop solutions to collect and analyze data from network devices, helping companies optimize performance and troubleshoot issues.
- Customer Churn Prediction: Building data models that analyze customer usage patterns, enabling proactive measures to retain subscribers.
5. The Impact of Data Engineers on Business Outcomes
Data engineers significantly influence business outcomes by ensuring that data flows seamlessly through an organization’s ecosystem:
- Efficiency in Data Processing:
- By designing efficient data pipelines, data engineers minimize the time it takes to prepare data for analysis, enabling quicker insights.
- Informed Decision-Making:
- Well-structured data environments allow organizations to make data-driven decisions, enhancing overall strategic planning and execution.
- Scalability:
- Data engineers create scalable data architectures that can grow with the organization, accommodating increasing data volumes without compromising performance.
- Cost Reduction:
- Efficient data management reduces operational costs associated with data storage and processing, providing a direct impact on the bottom line.
6. The Future of Data Engineering
As the field of data engineering continues to evolve, several trends are shaping its future:
- Increased Automation:
- Automation of data pipeline development and management will become more prevalent, allowing data engineers to focus on higher-level tasks such as architecture design and strategy.
- Integration with AI and Machine Learning:
- Data engineers will increasingly collaborate with data scientists to build systems that support advanced analytics and machine learning workflows.
- Focus on Data Governance:
- With rising concerns about data privacy and security, data engineers will play a critical role in implementing data governance frameworks to ensure compliance with regulations.
- Cloud-Native Architectures:
- The shift towards cloud-native data solutions will continue, with data engineers leveraging cloud platforms for scalability, flexibility, and cost-effectiveness.
In conclusion, data engineers are indispensable to any data-driven organization, responsible for the design and maintenance of the data infrastructure that underpins analytics and decision-making. By understanding the essential roles, skills, tools, and applications associated with data engineers, businesses can better appreciate the critical role these professionals play in shaping the future of data management and utilization.
5. Data Analyst
Data analysts play a pivotal role in transforming raw data into actionable insights, empowering organizations to make informed decisions. By utilizing statistical tools and analytical techniques, data analysts examine data trends and patterns, providing the necessary information for strategic planning and operational efficiency. This section delves into the key responsibilities, essential skills, tools, and real-world applications of data analysts, highlighting their significance in the data-driven landscape.
Also, read our top guide on how to hire a data analyst
1. Primary Responsibilities of a Data Analyst
Data analysts are tasked with a variety of responsibilities that enable them to derive meaningful insights from complex datasets. Their core duties typically include:
- Data Collection and Cleaning:
- Gather data from various sources, including databases, spreadsheets, and external datasets.
- Clean and preprocess data to eliminate errors, inconsistencies, and missing values, ensuring high data quality for analysis.
- Data Exploration and Visualization:
- Utilize exploratory data analysis (EDA) techniques to identify patterns, trends, and outliers in the data.
- Create visualizations using tools like Tableau, Power BI, or matplotlib to communicate findings effectively to stakeholders.
- Statistical Analysis:
- Apply statistical methods to analyze data, including hypothesis testing, regression analysis, and correlation studies.
- Interpret statistical results to identify significant relationships and insights relevant to business objectives.
- Reporting and Documentation:
- Generate comprehensive reports that summarize findings, highlight key insights, and provide recommendations for action.
- Document methodologies, data sources, and analytical processes to ensure reproducibility and transparency.
- Collaboration with Cross-Functional Teams:
- Work closely with data scientists, business analysts, and decision-makers to understand business needs and translate them into analytical requirements.
- Participate in cross-departmental projects to align data analysis efforts with organizational goals.
2. Essential Skills for Data Analysts
Data analysts require a diverse set of skills to navigate complex datasets and extract valuable insights effectively. Key skills include:
- Statistical Knowledge:
- Understanding of statistical concepts and methodologies, including descriptive and inferential statistics.
- Ability to perform A/B testing and assess the significance of findings.
- Programming and Data Manipulation:
- Proficiency in programming languages such as Python or R for data analysis and manipulation.
- Familiarity with SQL for querying databases and retrieving relevant data.
- Data Visualization:
- Experience with data visualization tools (e.g., Tableau, Power BI, or D3.js) to create compelling visual representations of data.
- Ability to present data insights in a clear and understandable manner, tailored to various audiences.
- Critical Thinking and Problem-Solving:
- Strong analytical skills to identify trends, make data-driven decisions, and solve complex business problems.
- Ability to approach problems creatively and develop actionable insights from data.
- Communication Skills:
- Effective verbal and written communication skills to convey complex data findings to non-technical stakeholders.
- Proficiency in creating reports and presentations that highlight key insights and recommendations.
3. Tools and Technologies Used by Data Analysts
Data analysts leverage a variety of tools and technologies to perform their analyses and communicate insights effectively. Some commonly used tools include:
- Programming Languages:
- Python: A versatile programming language with libraries like Pandas, NumPy, and SciPy, ideal for data manipulation and analysis.
- R: A language designed for statistical analysis and visualization, widely used among data analysts.
- Data Visualization Tools:
- Tableau: A leading data visualization tool that enables analysts to create interactive and shareable dashboards.
- Power BI: A Microsoft tool for business analytics that allows for data visualization and sharing insights across organizations.
- Database Management Systems:
- SQL: Structured Query Language is essential for querying databases and managing large datasets.
- Excel: A widely used spreadsheet application for data analysis, manipulation, and visualization, suitable for smaller datasets.
- Statistical Analysis Software:
- SAS: A software suite used for advanced analytics, business intelligence, and predictive analytics.
- SPSS: A statistical software package used for data analysis and visualization, particularly in social sciences.
- Business Intelligence Platforms:
- Looker: A platform that provides data analytics and business intelligence capabilities to organizations.
- QlikView: A business intelligence tool that allows users to create visualizations and dashboards for data analysis.
4. Real-World Applications of Data Analysts
Data analysts apply their skills across various industries, enabling organizations to leverage data for decision-making and strategic planning. Notable examples include:
- Retail:
- Customer Behavior Analysis: Data analysts examine purchasing patterns and customer demographics to enhance marketing strategies and improve customer experience.
- Inventory Optimization: By analyzing sales data, analysts help retailers forecast demand and optimize inventory levels, reducing stockouts and excess inventory.
- Finance:
- Risk Assessment: Analysts evaluate historical financial data to identify risks and trends, informing investment strategies and compliance efforts.
- Market Analysis: Data analysts conduct market research to assess economic trends, helping financial institutions make informed decisions regarding investments.
- Healthcare:
- Patient Outcomes Analysis: Data analysts examine healthcare data to assess treatment efficacy, optimize resource allocation, and improve patient care.
- Operational Efficiency: By analyzing operational data, healthcare organizations can identify areas for improvement, leading to cost savings and enhanced service delivery.
- Manufacturing:
- Quality Control: Analysts monitor production data to identify defects and improve quality control processes, enhancing product reliability.
- Supply Chain Management: By analyzing supply chain data, analysts help manufacturers optimize logistics, reduce costs, and improve delivery times.
- Marketing:
- Campaign Effectiveness: Data analysts measure the success of marketing campaigns through data analysis, allowing businesses to allocate budgets effectively.
- Segmentation Analysis: By analyzing customer data, analysts help organizations segment their audience for targeted marketing efforts, improving conversion rates.
5. The Impact of Data Analysts on Business Outcomes
Data analysts significantly influence business outcomes by providing insights that drive strategic decision-making and operational improvements. Their contributions can lead to:
- Enhanced Decision-Making:
- Data-driven insights enable organizations to make informed decisions, reducing reliance on intuition and guesswork.
- Clear reporting and visualization facilitate understanding and alignment among stakeholders.
- Improved Operational Efficiency:
- By identifying inefficiencies and bottlenecks through data analysis, organizations can streamline operations and reduce costs.
- Insights from data analysis lead to better resource allocation and process optimization.
- Increased Revenue and Profitability:
- Data analysts identify market trends and customer preferences, enabling businesses to capitalize on new opportunities and maximize revenue.
- Targeted marketing and sales strategies informed by data lead to higher conversion rates and customer retention.
- Competitive Advantage:
- Organizations that leverage data analysis effectively gain a competitive edge by responding more quickly to market changes and consumer demands.
- Insights from data analysis can help identify emerging trends and drive innovation.
6. The Future of Data Analysis
As the field of data analysis evolves, several trends are shaping its future:
- Automation and AI:
- Increasing use of automated data analysis tools and AI algorithms to streamline repetitive tasks and enhance analytical capabilities.
- AI-driven analytics will enable analysts to focus on higher-level strategic insights rather than manual data processing.
- Integration of Advanced Analytics:
- Greater integration of predictive and prescriptive analytics into data analysis processes to provide forward-looking insights and recommendations.
- Adoption of machine learning techniques to uncover deeper insights and improve decision-making.
- Focus on Data Governance and Ethics:
- Growing emphasis on data governance and ethical considerations in data analysis, ensuring compliance with regulations and protecting consumer privacy.
- Data analysts will play a critical role in implementing data governance frameworks to uphold data integrity and security.
- Collaboration with Data Science:
- Increased collaboration between data analysts and data scientists to leverage each other’s expertise and enhance analytical outcomes.
- Analysts will contribute to the development of machine learning models by providing domain knowledge and insights from data analysis.
In summary, data analysts are essential players in the data-driven landscape, tasked with extracting valuable insights from data to guide strategic decision-making. By understanding the key responsibilities, essential skills, tools, and applications associated with data analysts, organizations can appreciate their critical role in optimizing operations and driving growth in an increasingly competitive environment.
6. Machine Learning Engineer
Machine learning engineers are at the forefront of developing algorithms and models that enable machines to learn from data and make predictions or decisions without explicit programming. They blend expertise in software engineering, data science, and artificial intelligence to create robust machine learning solutions. This section explores the core responsibilities, essential skills, tools, real-world applications, and future trends surrounding machine learning engineers, emphasizing their significance in the rapidly evolving tech landscape.
Also, read our top guide on how to hire Machine Learning Engineer here.
1. Core Responsibilities of a Machine Learning Engineer
Machine learning engineers have a diverse set of responsibilities that focus on building and deploying machine learning models. Their key duties typically include:
- Model Development and Training:
- Design and implement machine learning models tailored to specific problems, including supervised and unsupervised learning approaches.
- Train models using large datasets, tuning hyperparameters to optimize performance.
- Data Preparation and Processing:
- Collect, clean, and preprocess data to ensure high-quality inputs for model training.
- Perform feature engineering to enhance model accuracy, selecting relevant features and transforming data as necessary.
- Model Evaluation and Optimization:
- Assess model performance using various metrics such as accuracy, precision, recall, and F1 score.
- Utilize techniques like cross-validation and grid search to fine-tune models and improve their predictive capabilities.
- Deployment and Maintenance:
- Deploy machine learning models into production environments, ensuring they integrate seamlessly with existing systems.
- Monitor model performance over time, implementing updates or retraining as needed to maintain accuracy and relevance.
- Collaboration and Communication:
- Work closely with data scientists, software engineers, and business stakeholders to understand requirements and deliver effective solutions.
- Present findings and model performance metrics to technical and non-technical audiences, facilitating data-driven decision-making.
2. Essential Skills for Machine Learning Engineers
To excel in their roles, machine learning engineers need a combination of technical skills and domain expertise. Key skills include:
- Programming Proficiency:
- Strong knowledge of programming languages such as Python and R, which are commonly used for developing machine learning algorithms.
- Familiarity with languages like Java and C++ for implementing machine learning solutions in production systems.
- Mathematics and Statistics:
- Solid understanding of mathematical concepts, including linear algebra, calculus, and probability, which form the foundation of machine learning algorithms.
- Knowledge of statistical analysis techniques for data interpretation and model evaluation.
- Machine Learning Algorithms:
- In-depth understanding of various machine learning algorithms, including regression, decision trees, neural networks, and clustering techniques.
- Ability to select appropriate algorithms based on the problem context and data characteristics.
- Data Manipulation and Analysis:
- Proficiency in data manipulation libraries such as Pandas and NumPy for data analysis and preprocessing.
- Experience with data visualization tools (e.g., Matplotlib, Seaborn) to communicate insights effectively.
- Deep Learning Frameworks:
- Familiarity with deep learning frameworks like TensorFlow and PyTorch for building and training complex neural networks.
- Understanding of convolutional neural networks (CNNs), recurrent neural networks (RNNs), and other deep learning architectures.
- Cloud Computing and Deployment:
- Knowledge of cloud platforms (e.g., AWS, Google Cloud, Microsoft Azure) for deploying machine learning models at scale.
- Understanding of containerization tools like Docker and orchestration platforms like Kubernetes for managing model deployments.
3. Tools and Technologies Used by Machine Learning Engineers
Machine learning engineers utilize a range of tools and technologies to develop, evaluate, and deploy models. Some commonly used tools include:
- Programming Languages:
- Python: A versatile language with extensive libraries (e.g., scikit-learn, Keras) specifically designed for machine learning tasks.
- R: Often used in academia and research, R provides robust statistical analysis and visualization capabilities.
- Machine Learning Frameworks:
- TensorFlow: An open-source library developed by Google, widely used for building and deploying machine learning models, particularly deep learning.
- PyTorch: A popular deep learning framework known for its dynamic computation graph, favored by researchers and developers alike.
- Data Processing Libraries:
- Pandas: A powerful data manipulation library in Python, essential for data cleaning and preprocessing.
- NumPy: A library for numerical computations in Python, facilitating array manipulations and mathematical operations.
- Visualization Tools:
- Matplotlib: A plotting library for Python that provides a flexible framework for creating static, animated, and interactive visualizations.
- Seaborn: Built on top of Matplotlib, Seaborn offers a higher-level interface for drawing attractive statistical graphics.
- Cloud Platforms:
- AWS SageMaker: A fully managed service that allows developers to build, train, and deploy machine learning models at scale.
- Google Cloud AI Platform: Provides tools and services for developing and deploying machine learning applications on the Google Cloud infrastructure.
4. Real-World Applications of Machine Learning Engineers
Machine learning engineers apply their skills across various industries, creating solutions that address real-world challenges. Notable examples include:
- Healthcare:
- Predictive Analytics: Developing models to predict patient outcomes, such as the likelihood of readmission or disease progression, improving patient care and resource allocation.
- Medical Imaging: Using deep learning techniques to analyze medical images, assisting radiologists in diagnosing conditions such as tumors or fractures.
- Finance:
- Fraud Detection: Implementing machine learning algorithms to analyze transaction patterns and identify fraudulent activities in real time, enhancing security measures for financial institutions.
- Credit Scoring: Building models to assess credit risk based on historical data, enabling lenders to make informed decisions about loan approvals.
- Retail:
- Recommendation Systems: Developing personalized recommendation engines that analyze customer behavior and preferences to suggest relevant products, increasing sales and customer satisfaction.
- Demand Forecasting: Using historical sales data to predict future demand for products, helping retailers optimize inventory and reduce stockouts.
- Automotive:
- Autonomous Vehicles: Working on algorithms that enable self-driving cars to process data from sensors and make real-time decisions for navigation and safety.
- Predictive Maintenance: Analyzing vehicle performance data to predict maintenance needs, reducing downtime and enhancing vehicle reliability.
- Telecommunications:
- Churn Prediction: Building models to identify customers at risk of leaving a service provider, allowing companies to implement retention strategies proactively.
- Network Optimization: Analyzing network traffic data to optimize performance, identify bottlenecks, and enhance user experience.
5. The Impact of Machine Learning Engineers on Business Outcomes
Machine learning engineers significantly influence business outcomes by developing innovative solutions that enhance operational efficiency and drive revenue growth. Their contributions can lead to:
- Enhanced Decision-Making:
- Data-driven insights provided by machine learning models enable organizations to make informed decisions, reducing reliance on intuition.
- Real-time predictions allow businesses to respond swiftly to changing market conditions.
- Increased Efficiency:
- Automation of routine tasks through machine learning frees up human resources for more strategic initiatives.
- Optimized processes result in cost savings and improved productivity across various functions.
- Improved Customer Experience:
- Personalized recommendations and services based on machine learning enhance customer satisfaction and loyalty.
- Predictive models help organizations anticipate customer needs and tailor offerings accordingly.
- Innovation and Competitive Advantage:
- Organizations leveraging machine learning solutions can differentiate themselves in the marketplace by offering unique products and services.
- Continuous improvement of models leads to ongoing innovation, keeping businesses ahead of the competition.
6. The Future of Machine Learning Engineering
The field of machine learning engineering is rapidly evolving, with several trends shaping its future landscape:
- Integration of AI and ML:
- Growing collaboration between machine learning and artificial intelligence disciplines, leading to more advanced and intelligent systems.
- Development of AI-driven tools that simplify the machine learning workflow, making it accessible to non-experts.
- Ethical AI and Fairness:
- Increased focus on ethical considerations in machine learning, including fairness, transparency, and accountability in algorithms.
- Machine learning engineers will play a crucial role in developing models that mitigate bias and uphold ethical standards.
- Automated Machine Learning (AutoML):
- Rising popularity of AutoML tools that automate the model selection, hyperparameter tuning, and feature engineering processes.
- These tools will enable a broader range of users to leverage machine learning capabilities without extensive technical knowledge.
- Edge Computing and IoT:
- Expansion of machine learning applications in edge computing and the Internet of Things (IoT), enabling real-time data processing and decision-making.
- Machine learning engineers will work on deploying models to edge devices, improving efficiency and responsiveness in various applications.
In summary, machine learning engineers are vital contributors to the development and deployment of intelligent systems that harness the power of data. Their expertise in model development, data processing, and collaboration with stakeholders positions them as key players in driving innovation and transforming industries. By understanding the core responsibilities, essential skills, tools, applications, and future trends associated with machine learning engineers, organizations can better appreciate their critical role in the evolving data landscape.
7. Data Architect
A data architect is a pivotal figure in the landscape of data management and utilization within an organization. Responsible for creating, managing, and optimizing the data framework, data architects ensure that data is accessible, reliable, and aligned with the organization’s business objectives. This section delves into the core responsibilities, essential skills, tools, real-world applications, and the strategic importance of data architects in driving data-driven decision-making across various industries.
1. Core Responsibilities of a Data Architect
Data architects have a multifaceted role that encompasses the design and maintenance of data systems and architecture. Their primary responsibilities include:
- Data Modeling and Design:
- Develop conceptual, logical, and physical data models to structure and organize data effectively.
- Use entity-relationship diagrams (ERDs) and other modeling tools to represent data relationships and flows.
- Database Management:
- Select appropriate database technologies (e.g., relational, NoSQL) based on organizational needs and data types.
- Design and implement database schemas, ensuring data integrity, security, and optimal performance.
- Data Integration:
- Oversee the integration of data from multiple sources, including databases, APIs, and third-party services.
- Develop ETL (Extract, Transform, Load) processes to ensure that data is consistently updated and transformed for analysis.
- Data Governance and Security:
- Establish data governance frameworks to ensure compliance with regulations (e.g., GDPR, HIPAA) and best practices.
- Implement security measures to protect sensitive data from unauthorized access and breaches.
- Collaboration and Stakeholder Engagement:
- Work closely with data engineers, data scientists, and business stakeholders to understand data requirements and translate them into architectural solutions.
- Communicate technical concepts to non-technical stakeholders, facilitating alignment on data strategies.
2. Essential Skills for Data Architects
To succeed as data architects, individuals must possess a unique combination of technical skills, analytical abilities, and business acumen. Key skills include:
- Technical Proficiency:
- Database Technologies: Strong knowledge of various database management systems, including SQL databases (e.g., MySQL, PostgreSQL) and NoSQL databases (e.g., MongoDB, Cassandra).
- Data Warehousing: Familiarity with data warehousing solutions (e.g., Amazon Redshift, Google BigQuery) for organizing and analyzing large datasets.
- Data Modeling and Design:
- Expertise in data modeling techniques and tools such as ER/Studio or Lucidchart, enabling the creation of accurate and efficient data structures.
- Understanding of normalization and denormalization concepts to optimize database performance.
- ETL Tools and Processes:
- Proficiency in ETL tools (e.g., Apache Nifi, Talend, Informatica) to manage data integration and transformation tasks effectively.
- Ability to design data pipelines that automate data extraction, transformation, and loading processes.
- Programming Skills:
- Familiarity with programming languages such as Python or R for data manipulation and scripting tasks.
- Knowledge of query languages like SQL for interacting with databases and retrieving data efficiently.
- Cloud Computing:
- Experience with cloud platforms (e.g., AWS, Azure, Google Cloud) that offer data storage and processing solutions, including cloud-based databases and data lakes.
- Understanding of cloud architecture principles to design scalable and cost-effective data solutions.
- Data Governance and Compliance:
- Knowledge of data governance frameworks and best practices, including data stewardship, data lineage, and metadata management.
- Awareness of regulatory requirements and standards related to data privacy and protection.
3. Tools and Technologies Used by Data Architects
Data architects leverage various tools and technologies to design, implement, and manage data architecture effectively. Some commonly used tools include:
- Database Management Systems:
- MySQL: An open-source relational database management system widely used for web applications and data-driven systems.
- MongoDB: A NoSQL database designed for flexibility and scalability, suitable for handling unstructured data.
- Data Modeling Tools:
- ER/Studio: A comprehensive data modeling tool that allows data architects to create and manage data models visually.
- Lucidchart: An online diagramming tool used to create data flow diagrams, ER diagrams, and other visual representations of data architecture.
- ETL and Data Integration Tools:
- Apache Nifi: A powerful data integration tool that automates data flows between systems, enabling seamless data ingestion and transformation.
- Informatica PowerCenter: A widely used ETL tool that provides a comprehensive suite for data integration and management.
- Cloud Data Solutions:
- Amazon Redshift: A fully managed data warehouse service that enables organizations to analyze large datasets using SQL.
- Google BigQuery: A cloud-based data warehouse that allows for fast SQL queries on large datasets, optimized for analytics.
- Data Governance Platforms:
- Collibra: A data governance platform that helps organizations manage data privacy, quality, and compliance through effective data stewardship.
- Alation: A data catalog tool that facilitates data discovery, collaboration, and governance within organizations.
4. Real-World Applications of Data Architects
Data architects apply their skills and expertise across various industries, helping organizations harness the power of data for strategic decision-making. Notable examples include:
- Healthcare:
- Patient Data Management: Designing systems to integrate electronic health records (EHR) from various providers, ensuring that healthcare professionals have access to comprehensive patient information.
- Predictive Analytics for Patient Outcomes: Implementing data architectures that facilitate the analysis of patient data to predict outcomes, improve treatment plans, and enhance overall patient care.
- Finance:
- Risk Management: Developing data models to assess credit risk and prevent fraud, integrating data from multiple sources to provide a holistic view of customer profiles.
- Regulatory Compliance: Designing data architectures that ensure compliance with financial regulations, such as anti-money laundering (AML) and know your customer (KYC) policies.
- Retail:
- Inventory Management: Implementing data architectures that integrate sales, inventory, and supplier data, enabling real-time visibility and optimization of stock levels.
- Customer Insights: Creating systems to analyze customer behavior and preferences, facilitating personalized marketing strategies and improving customer engagement.
- Telecommunications:
- Network Performance Optimization: Designing architectures that analyze network traffic data to identify performance issues and optimize resource allocation.
- Churn Prediction: Building models that predict customer churn based on usage patterns, enabling proactive retention strategies.
- Manufacturing:
- Supply Chain Optimization: Developing data systems that integrate supply chain data to enhance visibility and decision-making, reducing lead times and costs.
- Predictive Maintenance: Implementing data architectures that collect and analyze machine performance data, allowing for predictive maintenance and reducing downtime.
5. The Impact of Data Architects on Business Outcomes
Data architects play a crucial role in shaping how organizations leverage data to drive business success. Their contributions can lead to:
- Improved Data Accessibility:
- Streamlined data architectures facilitate easy access to relevant data for decision-makers, enhancing data-driven decision-making.
- Centralized data repositories reduce data silos, promoting collaboration across departments.
- Enhanced Data Quality:
- Robust data governance practices implemented by data architects ensure high data quality, reliability, and accuracy.
- Improved data quality directly impacts business outcomes, leading to more informed and effective decisions.
- Increased Operational Efficiency:
- Automated data integration and processing workflows reduce manual effort, saving time and resources.
- Efficient data management practices streamline operations, allowing organizations to focus on strategic initiatives.
- Strategic Insights and Innovation:
- Data architects enable organizations to harness advanced analytics and machine learning techniques, unlocking new insights and innovation opportunities.
- Data-driven insights lead to the development of new products, services, and business models, driving competitive advantage.
6. The Future of Data Architecture
The field of data architecture is evolving rapidly, influenced by advancements in technology and changing business needs. Key trends shaping the future of data architecture include:
- Cloud-Native Architectures:
- Increasing adoption of cloud-native data architectures that leverage cloud resources for scalability, flexibility, and cost-effectiveness.
- Data architects will focus on designing cloud-based data solutions that can easily integrate with existing systems.
- Data Mesh and Decentralization:
- The emergence of data mesh concepts, promoting decentralized data ownership and architecture, will reshape how organizations manage data.
- Data architects will need to embrace collaborative approaches, empowering teams to manage and leverage their data domains.
- AI and Machine Learning Integration:
- Growing demand for data architectures that support AI and machine learning initiatives, enabling real-time analytics and decision-making.
- Data architects will play a critical role in designing infrastructures that facilitate the integration of AI-driven applications.
- Emphasis on Data Ethics and Governance:
- Increased focus on ethical data practices and governance frameworks will shape data architecture strategies, ensuring compliance and responsible data use.
- Data architects will need to stay informed about regulatory changes and best practices in data governance.
In summary, data architects are integral to the successful management and utilization of data within organizations. Their expertise in designing data systems, ensuring data quality, and enabling strategic insights empowers businesses to thrive in a data-driven world. By understanding the core responsibilities, essential skills, tools, applications, and future trends associated with data architects, organizations can better appreciate their vital role in driving innovation and achieving business objectives.
8. Business Intelligence (BI) Analyst
A Business Intelligence (BI) Analyst plays a crucial role in helping organizations make data-driven decisions. By transforming raw data into actionable insights, BI Analysts enable businesses to understand trends, identify opportunities, and optimize performance. This section provides a comprehensive overview of the key responsibilities, essential skills, tools, real-world applications, and the strategic importance of BI Analysts in various industries.
1. Core Responsibilities of a BI Analyst
BI Analysts are responsible for a wide range of tasks focused on data analysis and visualization to support business decision-making. Their primary responsibilities include:
- Data Collection and Integration:
- Gather data from various sources, including databases, spreadsheets, and external data feeds.
- Integrate data into a centralized data warehouse or BI platform to ensure consistency and accessibility.
- Data Analysis and Interpretation:
- Analyze complex datasets to identify trends, patterns, and anomalies that can influence business decisions.
- Conduct exploratory data analysis (EDA) to gain insights into customer behavior, market trends, and operational efficiencies.
- Reporting and Visualization:
- Create comprehensive reports and dashboards that communicate insights clearly and effectively.
- Utilize visualization tools to represent data graphically, making it easier for stakeholders to understand and act upon findings.
- Collaboration with Stakeholders:
- Work closely with business units, management, and IT teams to understand data needs and business objectives.
- Provide support in decision-making processes by translating data insights into actionable recommendations.
- Performance Monitoring and Optimization:
- Monitor key performance indicators (KPIs) and metrics to assess organizational performance.
- Recommend strategies for performance improvement based on data insights and analysis.
2. Essential Skills for BI Analysts
To excel in their roles, BI Analysts must possess a unique blend of technical, analytical, and communication skills. Key skills include:
- Data Analysis and Statistical Skills:
- Proficiency in statistical analysis methods and techniques to interpret complex data sets effectively.
- Strong analytical skills to identify trends, correlations, and insights from data.
- Technical Proficiency:
- Database Management: Knowledge of SQL and relational database management systems (RDBMS) to extract, manipulate, and analyze data.
- Data Visualization Tools: Familiarity with BI tools such as Tableau, Power BI, and Looker for creating interactive dashboards and reports.
- Business Acumen:
- Understanding of business operations, industry trends, and key performance indicators relevant to the organization.
- Ability to align data analysis with business goals and objectives, providing actionable insights to drive strategic initiatives.
- Problem-Solving Skills:
- Strong problem-solving capabilities to identify data-related challenges and develop effective solutions.
- Creativity in analyzing data from multiple angles to uncover new insights.
- Communication Skills:
- Excellent verbal and written communication skills to convey complex data findings to non-technical stakeholders.
- Ability to create clear and concise reports and presentations that highlight key insights and recommendations.
3. Tools and Technologies Used by BI Analysts
BI Analysts utilize a variety of tools and technologies to perform data analysis, visualization, and reporting effectively. Common tools include:
- Data Visualization and BI Tools:
- Tableau: A leading data visualization tool that enables analysts to create interactive and shareable dashboards.
- Power BI: A Microsoft tool that allows users to connect to various data sources, create reports, and visualize data insights.
- Database Management Systems:
- SQL Server: A relational database management system used for data storage, retrieval, and manipulation through SQL.
- MySQL: An open-source RDBMS widely used for web applications and data analysis.
- Data Preparation Tools:
- Alteryx: A data preparation tool that allows analysts to cleanse, blend, and analyze data from multiple sources without coding.
- Talend: An open-source data integration platform that provides tools for ETL (Extract, Transform, Load) processes.
- Statistical Analysis Software:
- R: A programming language and software environment for statistical computing and graphics, widely used for data analysis.
- Python: A versatile programming language with libraries (e.g., Pandas, NumPy) that facilitate data manipulation and analysis.
- Collaboration Tools:
- Slack: A communication platform that enables teams to collaborate effectively and share insights in real-time.
- Microsoft Teams: A collaboration tool that integrates with Office 365, allowing teams to work together on data projects.
4. Real-World Applications of BI Analysts
BI Analysts are employed across various industries, using data insights to enhance operations, improve customer experiences, and drive growth. Notable examples include:
- Healthcare:
- Patient Care Optimization: Analyzing patient data to identify trends in treatment effectiveness and improve care plans, leading to better patient outcomes.
- Cost Management: Monitoring operational metrics to identify areas for cost reduction and resource optimization in healthcare facilities.
- Retail:
- Sales Performance Analysis: Examining sales data to identify top-performing products, seasonal trends, and customer preferences, helping retailers optimize inventory and marketing strategies.
- Customer Segmentation: Utilizing data analytics to segment customers based on behavior and preferences, allowing for personalized marketing and improved customer engagement.
- Finance:
- Risk Assessment: Analyzing financial data to assess credit risk and identify potential fraud, enabling more informed lending decisions.
- Investment Analysis: Monitoring market trends and performance metrics to provide insights for investment strategies and portfolio management.
- Telecommunications:
- Churn Prediction: Analyzing customer usage patterns to predict churn and develop retention strategies to improve customer loyalty.
- Network Performance Monitoring: Utilizing data insights to optimize network operations, reduce downtime, and enhance customer satisfaction.
- Manufacturing:
- Supply Chain Optimization: Analyzing production data to identify inefficiencies and optimize supply chain processes, reducing costs and improving delivery times.
- Quality Control: Monitoring product quality metrics to identify defects and improve manufacturing processes.
5. The Impact of BI Analysts on Business Outcomes
BI Analysts significantly impact organizational performance and decision-making by providing valuable insights derived from data. Their contributions can lead to:
- Informed Decision-Making:
- BI Analysts equip decision-makers with the data and insights needed to make strategic choices, leading to more effective business outcomes.
- Data-driven decisions reduce reliance on gut feelings and assumptions, fostering a culture of evidence-based practices.
- Enhanced Operational Efficiency:
- By analyzing operational data, BI Analysts identify bottlenecks and inefficiencies, leading to streamlined processes and cost savings.
- Continuous monitoring of KPIs helps organizations adjust strategies and tactics promptly to improve performance.
- Increased Revenue and Profitability:
- Insights from sales data enable organizations to identify growth opportunities and optimize pricing strategies, driving revenue growth.
- Improved customer insights lead to more targeted marketing campaigns, increasing conversion rates and customer loyalty.
- Competitive Advantage:
- Organizations that effectively leverage BI Analysts’ insights gain a competitive edge by responding swiftly to market changes and customer needs.
- Data-driven innovation fosters the development of new products and services, enhancing market positioning.
6. The Future of Business Intelligence Analysts
As organizations increasingly rely on data for decision-making, the role of BI Analysts is evolving. Key trends shaping the future of BI Analysts include:
- Integration of AI and Machine Learning:
- BI Analysts will increasingly leverage AI and machine learning algorithms to analyze complex datasets, uncover patterns, and generate predictive insights.
- The integration of AI tools will enable more advanced analytics, enhancing the ability to forecast trends and make proactive decisions.
- Self-Service BI:
- The rise of self-service BI tools empowers business users to access and analyze data independently, reducing the reliance on IT and BI teams.
- BI Analysts will focus on training and supporting users in leveraging these tools effectively, ensuring data governance and integrity.
- Real-Time Analytics:
- The demand for real-time insights will continue to grow, prompting BI Analysts to implement solutions that provide up-to-the-minute data for decision-making.
- Organizations will invest in technologies that support real-time data processing and analysis, enhancing responsiveness to changing business conditions.
- Data Democratization:
- The trend towards data democratization aims to make data accessible to all employees, fostering a data-driven culture across organizations.
- BI Analysts will play a crucial role in promoting data literacy and empowering teams to make data-informed decisions.
In summary, Business Intelligence Analysts are instrumental in transforming raw data into meaningful insights that drive organizational success. Their expertise in data analysis, visualization, and collaboration with stakeholders allows businesses to make informed decisions, optimize operations, and enhance overall performance. By understanding the core responsibilities, essential skills, tools, applications, and future trends associated with BI Analysts, organizations can better appreciate their vital role in navigating the complexities of today’s data-driven landscape.
9. Statistician
Statisticians play a pivotal role in interpreting and analyzing data across various fields, providing insights that drive decision-making processes. Their expertise in statistical methods and their ability to translate complex data into meaningful information make them essential in a data-driven world. This section explores the core responsibilities, essential skills, tools, real-world applications, and the strategic importance of statisticians in different industries.
1. Core Responsibilities of a Statistician
Statisticians engage in a variety of tasks focused on data analysis, model building, and interpretation. Their primary responsibilities include:
- Data Collection:
- Design experiments or surveys to gather relevant data.
- Determine appropriate sampling methods to ensure representative data collection.
- Collect data systematically to minimize biases and errors.
- Data Analysis:
- Apply statistical techniques to analyze and interpret complex data sets.
- Utilize descriptive statistics (mean, median, mode) to summarize data.
- Conduct inferential statistics to draw conclusions about populations based on sample data.
- Model Development:
- Develop predictive models to forecast future trends and outcomes.
- Employ regression analysis, time series analysis, and other advanced statistical methods to model relationships between variables.
- Validate and refine models to ensure accuracy and reliability.
- Report Generation:
- Create detailed reports summarizing findings, methodologies, and implications of the analysis.
- Visualize data using charts, graphs, and tables to communicate insights effectively.
- Present results to stakeholders, translating statistical findings into actionable recommendations.
- Consultation and Collaboration:
- Work with interdisciplinary teams to address specific research questions or business challenges.
- Provide statistical expertise in various domains, such as healthcare, finance, or social sciences.
- Collaborate with data scientists and analysts to enhance data-driven strategies.
2. Essential Skills for Statisticians
To excel in their roles, statisticians must possess a diverse set of skills that combine technical expertise with analytical thinking. Key skills include:
- Mathematical Proficiency:
- Strong foundation in mathematics, particularly in calculus, linear algebra, and probability theory.
- Ability to apply mathematical concepts to solve real-world problems.
- Statistical Knowledge:
- Expertise in a wide range of statistical methods, including hypothesis testing, ANOVA, regression analysis, and Bayesian statistics.
- Familiarity with sampling techniques and survey design.
- Analytical Skills:
- Strong analytical capabilities to interpret complex data and identify trends and patterns.
- Critical thinking skills to evaluate results and make informed decisions based on statistical analysis.
- Technical Proficiency:
- Proficiency in statistical software and programming languages, such as:
- R: Widely used for statistical analysis and data visualization.
- Python: Popular for data analysis, particularly with libraries like NumPy and Pandas.
- SAS: A software suite used for advanced analytics, business intelligence, and data management.
- Proficiency in statistical software and programming languages, such as:
- Communication Skills:
- Excellent verbal and written communication skills to explain complex statistical concepts to non-technical stakeholders.
- Ability to create clear and concise reports and visualizations that effectively convey findings.
3. Tools and Technologies Used by Statisticians
Statisticians utilize various tools and technologies to facilitate data analysis and model development. Common tools include:
- Statistical Software:
- R: An open-source programming language used for statistical computing and graphics, ideal for data manipulation and visualization.
- SAS: A powerful analytics software suite used for advanced statistical analysis and business intelligence.
- SPSS: A statistical software package widely used in social science research for data analysis.
- Data Visualization Tools:
- Tableau: A leading data visualization tool that enables users to create interactive and shareable dashboards.
- Microsoft Power BI: A business analytics tool that provides interactive visualizations and business intelligence capabilities.
- Programming Languages:
- Python: A versatile language used for data analysis, with libraries such as SciPy and StatsModels specifically designed for statistical tasks.
- MATLAB: A programming environment used for numerical computing and data visualization, popular in academia and engineering.
- Data Management Systems:
- SQL: A standard language for managing and querying relational databases, allowing statisticians to extract and manipulate data efficiently.
- NoSQL Databases: Used for handling unstructured data, providing flexibility in data storage and retrieval.
4. Real-World Applications of Statisticians
Statisticians are employed across various sectors, utilizing their expertise to derive insights that inform strategies and improve outcomes. Notable examples include:
- Healthcare:
- Clinical Trials: Statisticians design and analyze clinical trials to evaluate the effectiveness of new drugs and treatments.
- Example: A statistician may develop a randomized controlled trial to assess a new medication’s efficacy, ensuring rigorous analysis of patient outcomes.
- Epidemiology: Analyzing health data to identify patterns and trends in disease prevalence and risk factors.
- Example: Statisticians study the spread of infectious diseases, using statistical models to predict outbreaks and inform public health responses.
- Clinical Trials: Statisticians design and analyze clinical trials to evaluate the effectiveness of new drugs and treatments.
- Finance:
- Risk Assessment: Statisticians develop models to assess financial risks and uncertainties in investments and lending.
- Example: A statistician might use predictive modeling to analyze historical market data, helping banks make informed decisions on loan approvals.
- Portfolio Optimization: Analyzing investment portfolios to maximize returns while minimizing risk.
- Example: Using statistical techniques to determine the optimal asset allocation in a diversified investment portfolio.
- Risk Assessment: Statisticians develop models to assess financial risks and uncertainties in investments and lending.
- Marketing:
- Market Research: Conducting surveys and analyzing consumer data to understand market trends and consumer behavior.
- Example: Statisticians may analyze survey data to identify target demographics for a new product launch.
- A/B Testing: Implementing experimental designs to test marketing strategies and optimize campaign effectiveness.
- Example: Analyzing conversion rates from different advertising approaches to determine the most effective marketing strategy.
- Market Research: Conducting surveys and analyzing consumer data to understand market trends and consumer behavior.
- Manufacturing:
- Quality Control: Applying statistical process control (SPC) to monitor and improve production processes, ensuring product quality.
- Example: Statisticians analyze defect rates in production lines, identifying factors that contribute to quality issues.
- Supply Chain Optimization: Using statistical models to forecast demand and optimize inventory levels.
- Example: A statistician might analyze historical sales data to predict future demand, enabling better inventory management.
- Quality Control: Applying statistical process control (SPC) to monitor and improve production processes, ensuring product quality.
- Education:
- Educational Assessment: Analyzing test scores and educational data to evaluate teaching effectiveness and student performance.
- Example: Statisticians may analyze standardized test scores to identify areas for curriculum improvement.
- Surveys and Polling: Conducting surveys to gather data on student experiences and perceptions.
- Example: Designing and analyzing student satisfaction surveys to inform institutional improvements.
- Educational Assessment: Analyzing test scores and educational data to evaluate teaching effectiveness and student performance.
5. The Impact of Statisticians on Business Outcomes
Statisticians significantly influence organizational success by providing data-driven insights that enhance decision-making processes. Their contributions lead to:
- Informed Decision-Making:
- Statisticians provide critical data analysis that informs strategic decisions, reducing reliance on assumptions and intuition.
- Evidence-based decision-making enhances the likelihood of successful outcomes and minimizes risks.
- Improved Operational Efficiency:
- By analyzing operational data, statisticians identify inefficiencies and recommend process improvements.
- Continuous monitoring of key metrics enables organizations to adapt and optimize their operations.
- Enhanced Product Development:
- Statistical analysis supports product testing and validation, ensuring that new products meet consumer needs and quality standards.
- Data-driven insights from market research inform product development strategies.
- Increased Revenue and Market Share:
- Statisticians help businesses understand market trends, enabling targeted marketing and sales strategies that drive revenue growth.
- Improved customer insights lead to enhanced customer experiences, fostering loyalty and repeat business.
- Competitive Advantage:
- Organizations that leverage statistical analysis gain insights into industry trends and customer behavior, allowing them to stay ahead of competitors.
- Data-driven innovation supports the development of new products and services that meet market demands.
6. The Future of Statisticians
As the demand for data analysis continues to grow, the role of statisticians is evolving. Key trends shaping the future of statisticians include:
- Integration of Advanced Analytics:
- The rise of big data and machine learning technologies will enable statisticians to employ more sophisticated analytical methods.
- Statisticians will increasingly work alongside data scientists to leverage AI and predictive analytics in their analyses.
- Data Democratization:
- The trend toward data democratization will empower more individuals within organizations to engage in data analysis.
- Statisticians will play a vital role in training and supporting non-technical stakeholders in understanding and using data effectively.
- Real-Time Data Analysis:
- The growing need for real-time insights will prompt statisticians to develop systems for immediate data processing and analysis.
- Organizations will invest in technologies that facilitate real-time decision-making based on live data streams.
- Ethics in Data Analysis:
- As data usage increases, statisticians will need to navigate ethical considerations related to data privacy and bias.
- Emphasizing transparency and accountability in data analysis will become paramount in maintaining public trust.
In summary, statisticians are essential in translating complex data into actionable insights that drive organizational success. Their expertise in data collection, analysis, modeling, and reporting allows businesses to make informed decisions, optimize operations, and enhance overall performance. By understanding the core responsibilities, essential skills, tools, applications, and future trends associated with statisticians, organizations can better appreciate their vital role in navigating the complexities of today’s data-driven landscape.
10. Data Product Manager
A Data Product Manager (DPM) plays a vital role in any data science team, bridging the gap between business objectives and technical capabilities to ensure that data products are valuable, usable, and aligned with company goals. As organizations increasingly rely on data-driven strategies, the DPM’s ability to oversee the end-to-end development of data products, from ideation to execution, has become crucial for success. This section will detail the core responsibilities, essential skills, tools, and examples of how Data Product Managers impact organizations.
1. Core Responsibilities of a Data Product Manager
Data Product Managers oversee the entire lifecycle of a data product, aligning it with the organization’s strategic goals and ensuring it meets user needs.
- Defining Product Vision and Strategy:
- Collaborate with stakeholders to define a product vision that aligns with organizational goals.
- Translate business requirements into data-focused product goals that provide actionable insights and drive decision-making.
- Product Roadmap Development:
- Create and manage the product roadmap to outline key milestones, deliverables, and timelines.
- Prioritize features based on business impact, technical feasibility, and user needs, ensuring alignment with overall strategy.
- Stakeholder Communication:
- Act as the main point of contact for various teams (e.g., engineering, marketing, data science) to facilitate clear and consistent communication.
- Regularly update stakeholders on product progress, timelines, and any adjustments needed due to emerging data insights or technical constraints.
- User and Market Research:
- Conduct market research and analyze user feedback to understand the needs of the target audience.
- Integrate data usage patterns and behavioral insights into product development to enhance user experience and maximize engagement.
- Data Governance and Compliance:
- Ensure data products meet industry standards for data security, privacy, and compliance.
- Work closely with legal and compliance teams to align product features with data governance policies, especially in regulated industries like healthcare and finance.
- Performance Tracking and Optimization:
- Define key performance indicators (KPIs) to track the success of the data product.
- Use A/B testing, user feedback, and performance analytics to continuously optimize product features and improve usability.
2. Essential Skills for a Data Product Manager
A successful Data Product Manager combines technical, analytical, and business acumen to manage the lifecycle of data products effectively.
- Technical Proficiency:
- Knowledge of data science fundamentals, including machine learning, data modeling, and analytics, to make informed decisions during product development.
- Familiarity with data architecture and cloud platforms such as AWS, Google Cloud, or Azure, which are commonly used in data product infrastructures.
- Business and Strategic Thinking:
- Ability to align product development with business objectives, ensuring each feature provides measurable value to the organization.
- Strong understanding of industry trends and the competitive landscape to develop products that meet market demand.
- Analytical Skills:
- Proficiency in data analysis to interpret user behavior, product usage metrics, and market trends, often using tools like SQL, Python, or Tableau.
- Ability to analyze large datasets, derive insights, and use data to justify product decisions or pivots.
- Project Management Skills:
- Expertise in project management methodologies, such as Agile or Scrum, to manage timelines, resources, and deliverables.
- Ability to coordinate with cross-functional teams, setting clear objectives, timelines, and dependencies.
- Communication and Collaboration:
- Strong communication skills to present complex data concepts in an understandable way for non-technical stakeholders.
- Proven experience in working with cross-functional teams, balancing differing viewpoints, and finding consensus to move projects forward.
3. Tools and Technologies Used by Data Product Managers
Data Product Managers rely on a variety of tools to streamline product management, data analysis, and collaboration.
- Product Management and Collaboration Tools:
- Jira and Trello: Used to manage tasks, track product development progress, and organize sprints within the Agile framework.
- Asana: A task management tool that helps coordinate cross-team work, set priorities, and track project timelines.
- Data Visualization and Analytics Tools:
- Tableau: Used to visualize data insights, analyze usage patterns, and create reports that can guide product decisions.
- Looker: A BI platform that enables exploration and analysis of real-time data, often used to track product KPIs.
- Customer Feedback and Research Tools:
- Qualtrics: A survey tool that allows Data Product Managers to gather user feedback, ensuring product development aligns with user needs.
- UsabilityHub: A platform for testing user experiences and gathering data on user interactions with prototypes or feature ideas.
- Programming Languages and Data Analysis Tools:
- SQL: A core tool for querying databases, allowing Data Product Managers to analyze product usage and user behavior.
- Python or R: Often used for data analysis, enabling deeper insights into user patterns and product engagement.
4. Real-World Applications of a Data Product Manager’s Work
The contributions of Data Product Managers can be seen across various industries, from enhancing customer experience to optimizing internal operations. Below are a few examples:
- E-commerce Platforms:
- Example: A Data Product Manager at an e-commerce company may oversee the development of a recommendation engine that suggests products based on past purchases and browsing history.
- They analyze customer behavior, optimize recommendation algorithms, and track KPIs such as click-through and conversion rates.
- Example: A Data Product Manager at an e-commerce company may oversee the development of a recommendation engine that suggests products based on past purchases and browsing history.
- Healthcare:
- Example: In healthcare, a DPM might manage a product that provides predictive analytics for patient health outcomes.
- By aggregating data from electronic health records, the DPM ensures the model adheres to compliance standards and optimizes treatment recommendations for clinicians.
- Example: In healthcare, a DPM might manage a product that provides predictive analytics for patient health outcomes.
- Finance:
- Example: A DPM at a bank could develop a credit scoring product that assesses creditworthiness using machine learning models.
- The DPM would collaborate with data scientists to improve model accuracy, ensuring regulatory compliance while increasing loan approval rates.
- Example: A DPM at a bank could develop a credit scoring product that assesses creditworthiness using machine learning models.
- Retail:
- Example: In retail, DPMs can develop demand forecasting products that predict inventory needs based on historical sales data and seasonality.
- They work with supply chain teams to ensure the product meets inventory demands and optimizes stock levels, reducing overstock and stockouts.
- Example: In retail, DPMs can develop demand forecasting products that predict inventory needs based on historical sales data and seasonality.
5. The Strategic Importance of Data Product Managers
Data Product Managers offer immense strategic value by connecting business goals with data solutions, ensuring products are designed and refined to meet both market demands and internal objectives.
- Driving Innovation:
- Data Product Managers ensure that data is leveraged to drive product innovation, allowing companies to capitalize on emerging market trends.
- By coordinating R&D efforts and focusing on market-relevant solutions, they help organizations stay competitive.
- Enhancing Customer Experience:
- With a focus on user-centric data products, DPMs develop tools and features that improve customer interactions and satisfaction.
- Example: At a streaming service, a DPM might enhance recommendation systems, helping users discover content that aligns with their preferences and boosting user retention.
- Supporting Data-Driven Decision Making:
- DPMs provide essential insights into data product performance, guiding executives and managers in making informed decisions.
- By focusing on measurable impact, they ensure resources are allocated to features that generate the highest return on investment.
- Optimizing Operations:
- Data Product Managers create products that streamline operations, from automation to predictive maintenance, improving efficiency and reducing costs.
- Example: A DPM in logistics may develop a data product that uses historical data to predict delivery times, helping optimize route planning and reduce shipping costs.
- Ensuring Compliance and Security:
- In industries where data security and privacy are critical, DPMs ensure products meet all regulatory and compliance requirements.
- This is particularly crucial in sectors like finance and healthcare, where breaches in data security can result in substantial fines and reputational damage.
6. Future Trends and the Evolving Role of Data Product Managers
The role of Data Product Managers is rapidly evolving as new data technologies and methodologies emerge. Key trends shaping the future of Data Product Management include:
- Increased Use of AI and Machine Learning:
- Data Product Managers will increasingly manage products that leverage machine learning for real-time data insights and predictive analytics.
- Example: DPMs may oversee AI-powered products that personalize user experiences, using algorithms that evolve based on user interaction.
- Focus on Responsible AI and Data Ethics:
- As data products grow more sophisticated, DPMs will play a key role in establishing ethical guidelines to prevent biased algorithms and ensure responsible data usage.
- There will be an emphasis on transparency and fairness, particularly in sectors such as hiring, lending, and healthcare.
- Enhanced Collaboration with Data Governance Teams:
- With heightened focus on data privacy and compliance, DPMs will increasingly collaborate with data governance and legal teams.
- They’ll ensure data products adhere to privacy regulations such as GDPR and CCPA, especially when products involve sensitive customer data.
- Real-Time Data Products and IoT:
- The rise of IoT is driving demand for real-time data products, and DPMs will oversee the integration of IoT data with analytics platforms.
- This trend is prominent in industries like manufacturing and transportation, where real-time data can enhance operational efficiency.
- Data Democratization within Organizations:
- As organizations embrace data democratization, DPMs will develop self-service data products that empower non-technical users to access and analyze data.
- This will support a data-driven culture across departments, increasing the overall impact of data on organizational growth.
In summary, the role of a Data Product Manager is essential in today’s data-driven organizations. By combining business acumen with technical expertise, DPMs drive data product success, create value for stakeholders, and ensure products align with strategic objectives.
11. Data Analyst vs. Data Scientist: Understanding the Differences
Understanding the distinctions between data analysts and data scientists is crucial for organizations aiming to leverage data effectively. While both roles work with data to derive insights, they differ in their focus, skill sets, tools, and the complexity of tasks they perform. Here’s an in-depth look into these two roles to clarify their unique contributions.
Role and Responsibilities
Data Analyst: Primarily Focused on Interpreting Data
- Objective: Data analysts aim to support decision-making by analyzing historical data and presenting actionable insights.
- Primary Responsibilities:
- Extract, clean, and interpret data from multiple sources to support business decisions.
- Identify trends and patterns within datasets to provide straightforward, descriptive analysis.
- Collaborate with business teams to create reports and dashboards that make data insights accessible.
- Examples:
- A retail data analyst might analyze seasonal purchasing patterns to inform inventory decisions.
- In a marketing context, they might assess the effectiveness of a recent campaign by examining engagement metrics.
Data Scientist: Emphasis on Predictive and Prescriptive Analytics
- Objective: Data scientists focus on creating predictive models that forecast future trends and automate decision-making processes using advanced algorithms.
- Primary Responsibilities:
- Conduct exploratory data analysis to uncover deeper insights and establish hypotheses.
- Develop machine learning models that predict outcomes or classify data based on past trends.
- Create data-driven algorithms that automate processes, often integrating models directly into applications.
- Examples:
- A financial data scientist may develop credit scoring models to predict loan defaults.
- In healthcare, they might build predictive models to forecast patient health outcomes based on historical data.
Key Skills Required
Data Analyst Skills
- Statistical Analysis: Proficiency in statistics for interpreting data and understanding correlations and trends.
- Data Visualization: Expertise in tools like Tableau, Power BI, and Excel to create charts and dashboards for data presentation.
- SQL: Strong SQL skills for querying relational databases and extracting the specific data required for analysis.
- Attention to Detail: A keen eye for spotting anomalies or inconsistencies in data.
- Business Acumen: Knowledge of industry-specific metrics and KPIs to provide relevant insights.
Data Scientist Skills
- Machine Learning and AI: Proficiency in machine learning algorithms (e.g., regression, classification, clustering) to develop predictive models.
- Programming: Expertise in Python, R, or Scala for data manipulation, analysis, and building complex algorithms.
- Big Data and Distributed Computing: Familiarity with big data tools like Hadoop and Spark to process large datasets.
- Advanced Statistical Knowledge: In-depth understanding of statistical modeling and experimental design.
- Data Engineering Fundamentals: Basic knowledge of data pipelines and data architecture for effective data preparation.
Tools Used by Data Analysts vs. Data Scientists
Data Analyst Tools
- Data Visualization Tools: Tableau, Power BI, Google Data Studio for creating interactive dashboards.
- Excel: Widely used for quick data analysis and reporting.
- SQL Databases: SQL Server, MySQL, PostgreSQL for data extraction and manipulation.
- Statistical Software: Basic statistical tools like SAS or SPSS for performing descriptive analytics.
Data Scientist Tools
- Programming Languages: Python (NumPy, Pandas, Scikit-learn), R for machine learning and statistical analysis.
- Machine Learning Libraries: TensorFlow, PyTorch, Keras for building and training complex models.
- Big Data Technologies: Hadoop, Spark for handling large volumes of data.
- Data Engineering Tools: SQL, NoSQL databases, and data warehousing solutions like AWS Redshift and Google BigQuery for data preparation.
- Visualization Libraries: Matplotlib, Seaborn, Plotly in Python for exploratory data visualization.
Educational Background
Data Analyst Education
- Typical Qualifications: A bachelor’s degree in business, statistics, or a related field is generally sufficient.
- Additional Certifications: Certifications in data visualization (Tableau, Power BI) or SQL can add value.
- Emphasis: More emphasis is placed on business analytics, statistical methods, and visualization skills.
Data Scientist Education
- Typical Qualifications: A master’s or Ph.D. in data science, computer science, mathematics, or a similar field is often required.
- Additional Certifications: Courses in machine learning, AI, or data engineering from platforms like Coursera or Udacity are highly valued.
- Emphasis: Focus on advanced statistics, machine learning, programming, and big data processing.
Examples of Real-World Applications
Data Analyst Example
- Scenario: A retail company wants to analyze customer purchase behavior during the holiday season.
- Data Analyst Role: The analyst would examine transaction data, identify top-selling products, and present findings through a dashboard, helping the marketing team tailor future promotions.
Data Scientist Example
- Scenario: A healthcare organization aims to reduce patient readmission rates.
- Data Scientist Role: The scientist develops a machine learning model to predict which patients are at high risk of readmission, helping staff allocate resources and personalize care.
Career Growth and Opportunities
Data Analyst Career Path
- Starting Role: Data analysts often start as junior analysts, focusing on reporting and visualizing data.
- Growth Opportunities:
- Move into senior analyst roles or specialized roles such as marketing analyst or financial analyst.
- Transition to data scientist roles with additional training in machine learning and programming.
- Examples: A data analyst in a tech company might advance to become a business intelligence manager, overseeing analytics across departments.
Data Scientist Career Path
- Starting Role: Data scientists often enter as junior or entry-level scientists, working under senior scientists to build models.
- Growth Opportunities:
- Move into senior scientist roles or specialization in fields like NLP (Natural Language Processing) or computer vision.
- Progress to leadership roles such as data science manager or head of data science.
- Examples: A data scientist specializing in finance may move up to lead a team developing trading algorithms for a financial institution.
Impact on Business Decision-Making
Data Analyst Impact
- Role in Business: Provides historical insights that help teams understand past performance and identify areas for improvement.
- Decision-Making Influence: By delivering straightforward, actionable insights, data analysts enable managers to make data-informed decisions without technical knowledge.
Data Scientist Impact
- Role in Business: Focuses on building predictive models and automated processes that guide future decisions and optimize operations.
- Decision-Making Influence: By implementing AI models, data scientists allow organizations to predict outcomes, personalize customer experiences, and drive innovation.
Summary of Key Differences
- Scope of Work: Data analysts focus on historical data analysis and reporting, while data scientists focus on predictive modeling and machine learning.
- Skill Requirements: Data analysts prioritize SQL, Excel, and visualization, whereas data scientists require programming, machine learning, and big data knowledge.
- Educational Background: Data analysts typically hold a bachelor’s degree; data scientists often have advanced degrees.
- Business Impact: Data analysts support tactical decision-making; data scientists drive strategic initiatives through predictive analytics.
Choosing the Right Role for Your Team
For data-driven organizations, having both data analysts and data scientists is essential to cover a broad spectrum of data needs:
- When to Hire a Data Analyst: Ideal for companies needing insights on business metrics, trend analysis, and reporting.
- When to Hire a Data Scientist: Best for organizations aiming to create predictive models, automate decisions, or handle complex, unstructured data.
This comprehensive understanding of data analysts versus data scientists enables organizations to build balanced teams capable of delivering impactful insights, supporting informed decisions, and driving long-term business success.
12. Key Skills for Success in a Data Science Team
In a data science team, the skills of individual members directly impact the team’s effectiveness and success. While technical skills are fundamental, a well-rounded data science team also requires expertise in communication, business understanding, and collaboration. This section will cover essential skills for data science team members, organized by technical, analytical, communication, and soft skills categories, providing relevant examples to illustrate their importance in real-world applications.
1. Technical Skills
Technical skills are foundational to a data science team, enabling team members to handle data processing, analysis, and model development with precision and efficiency.
- Programming Proficiency:
- Python and R are the primary languages for data analysis and machine learning due to their extensive libraries (e.g., NumPy, pandas, Scikit-Learn).
- Example: A data scientist using Python’s Scikit-Learn to train and evaluate a predictive model, or R for statistical analysis.
- SQL: Essential for querying databases, allowing data scientists and analysts to access, filter, and manipulate large datasets.
- Example: SQL helps a Data Engineer in optimizing database queries to speed up data retrieval processes in an e-commerce platform.
- Java and Scala: Important for building scalable, production-level data applications, often used in big data environments.
- Example: A Machine Learning Engineer might use Scala with Apache Spark for large-scale data processing.
- Python and R are the primary languages for data analysis and machine learning due to their extensive libraries (e.g., NumPy, pandas, Scikit-Learn).
- Data Manipulation and Wrangling:
- Proficiency in handling data cleaning and preprocessing tasks, which often require tools like Python’s pandas or specialized libraries like Dplyr in R.
- Example: A Data Analyst cleaning transactional data for analysis, ensuring accurate insights without skewed or incomplete information.
- Statistical Analysis and Mathematics:
- Strong foundation in probability, linear algebra, calculus, and statistics to support data modeling and interpretation.
- Example: A Statistician using probability theory to model customer churn predictions for a subscription-based service.
- Machine Learning and Deep Learning:
- Skills in machine learning frameworks like TensorFlow, PyTorch, and Keras for building and training models.
- Example: A Machine Learning Engineer using TensorFlow to develop a neural network that classifies customer feedback by sentiment.
- Skills in machine learning frameworks like TensorFlow, PyTorch, and Keras for building and training models.
- Big Data Technologies:
- Knowledge of distributed computing and big data frameworks like Apache Hadoop and Spark for handling massive datasets.
- Example: A Data Engineer processing terabytes of data using Spark to improve recommendation algorithms for a streaming platform.
- Data Visualization:
- Proficiency with tools like Tableau, Power BI, Matplotlib, and Seaborn to create visually compelling and interpretable data presentations.
- Example: A BI Analyst using Tableau to create dashboards that track key performance indicators (KPIs) for marketing teams.
2. Analytical and Problem-Solving Skills
Analytical skills enable data science team members to interpret data meaningfully and solve complex business challenges effectively.
- Critical Thinking:
- Ability to approach data with a questioning mindset, assessing data sources, quality, and relevance before analysis.
- Example: A Data Scientist identifying potential biases in a training dataset before developing a predictive model to ensure fairness.
- Pattern Recognition:
- Skilled in recognizing patterns, trends, and anomalies within datasets that could indicate significant business insights.
- Example: A Data Analyst identifying seasonal trends in retail sales data, allowing the marketing team to plan promotions accordingly.
- Statistical Modeling and Hypothesis Testing:
- Capability to create models to test hypotheses and draw actionable conclusions based on data.
- Example: A Statistician conducting A/B testing on different product layouts on a website to determine which design drives higher conversion rates.
- Problem Decomposition:
- Breaking down complex business problems into smaller, manageable components to facilitate focused data analysis and solution design.
- Example: A Data Product Manager decomposing a churn reduction initiative into components like feature extraction, customer segmentation, and predictive modeling.
3. Business Acumen and Domain Knowledge
Understanding the business context is essential for data science teams, enabling them to align their efforts with strategic goals.
- Industry-Specific Knowledge:
- Awareness of specific industry metrics, terminology, and best practices, which allows data science teams to apply data insights effectively.
- Example: A Data Scientist in healthcare knowing how to interpret patient data within HIPAA regulations or understanding clinical metrics to ensure compliance.
- Understanding Business Objectives:
- Ability to connect data solutions to business goals, ensuring all analysis and modeling efforts are aligned with company strategy.
- Example: A Data Product Manager working with the sales team to develop a predictive model that identifies high-value leads, thus boosting conversion rates.
- Financial Literacy:
- Knowledge of financial metrics and principles, enabling data science professionals to provide value-driven recommendations.
- Example: A BI Analyst tracking revenue per customer and other financial KPIs to assess the success of a new subscription model.
- Customer-Centric Mindset:
- Focusing on customer needs and behavior to develop data products that enhance user experience and engagement.
- Example: A Data Engineer developing a recommendation engine for an online retailer that tailors suggestions based on previous purchases and browsing history.
4. Communication Skills
Data science team members must effectively communicate their insights and collaborate with stakeholders across various departments.
- Data Storytelling:
- Presenting data insights in a clear, engaging, and understandable way that resonates with non-technical stakeholders.
- Example: A Data Scientist creating a narrative around customer behavior trends to inform marketing strategies.
- Report Writing:
- Writing clear, concise, and informative reports that summarize data findings and recommendations.
- Example: A Data Analyst delivering a monthly report detailing sales trends, using data to suggest tactics for increasing revenue.
- Presentation Skills:
- Ability to communicate complex technical findings in a way that’s accessible and meaningful for non-technical audiences.
- Example: A Data Architect presenting a new data pipeline architecture to the executive team, explaining how it will improve data processing efficiency.
- Cross-Functional Collaboration:
- Working effectively with different departments to ensure data solutions align with broader business needs.
- Example: A Machine Learning Engineer collaborating with product and marketing teams to develop customer segmentation models.
5. Soft Skills for Team Collaboration
In a data science team, strong interpersonal skills can enhance collaboration, foster creativity, and improve problem-solving dynamics.
- Adaptability:
- Flexibility to adjust to new challenges, tools, and methodologies as data science rapidly evolves.
- Example: A Data Scientist learning a new machine learning library to improve model accuracy and stay current with industry practices.
- Curiosity and Continuous Learning:
- Demonstrating a desire to explore new tools, trends, and techniques, often through self-study or professional development.
- Example: A Statistician experimenting with deep learning techniques to expand their analytical capabilities in predictive modeling.
- Attention to Detail:
- Precision and thoroughness in data handling, essential for preventing errors and ensuring data integrity.
- Example: A Data Analyst double-checking calculations and code to ensure that reports reflect accurate insights.
- Problem Ownership and Initiative:
- Taking responsibility for project outcomes, proactively addressing issues as they arise, and pushing projects forward.
- Example: A Data Engineer identifying a bottleneck in the data pipeline and implementing a solution without waiting for direction.
- Time Management and Prioritization:
- Ability to manage multiple projects, prioritize tasks, and deliver insights within deadlines.
- Example: A Data Product Manager juggling various stakeholder requests while maintaining focus on delivering high-priority product features.
6. Tool-Specific Skills
Proficiency in key tools ensures that data science team members can perform their duties effectively and efficiently.
- Data Manipulation Tools:
- Pandas, NumPy, and Dplyr for data cleaning and transformation.
- Example: A Data Scientist using pandas to clean and format large datasets before feeding them into a machine learning model.
- Pandas, NumPy, and Dplyr for data cleaning and transformation.
- Big Data and Cloud Platforms:
- Familiarity with AWS, Google Cloud Platform, and Microsoft Azure for handling large datasets and scalable data solutions.
- Example: A Data Engineer using AWS Redshift to store and analyze petabytes of transaction data.
- Familiarity with AWS, Google Cloud Platform, and Microsoft Azure for handling large datasets and scalable data solutions.
- Machine Learning Libraries:
- Proficiency in TensorFlow, Keras, and Scikit-Learn for building and testing machine learning models.
- Example: A Machine Learning Engineer using TensorFlow to create a deep learning model that automates image recognition.
- Proficiency in TensorFlow, Keras, and Scikit-Learn for building and testing machine learning models.
- Data Visualization Tools:
- Skilled in Tableau, Power BI, and Matplotlib for creating clear and insightful data visualizations.
- Example: A BI Analyst building a Power BI dashboard to provide real-time insights into company sales performance.
- Skilled in Tableau, Power BI, and Matplotlib for creating clear and insightful data visualizations.
- Project Management Software:
- Tools like Jira, Asana, and Trello for task management, collaboration, and keeping track of project milestones.
- Example: A Data Product Manager using Jira to coordinate team sprints and track the progress of feature development.
- Tools like Jira, Asana, and Trello for task management, collaboration, and keeping track of project milestones.
By mastering these skills, data science team members not only enhance their individual contributions but also support the overall effectiveness and success of their team. In an industry that continuously evolves, data science professionals must commit to ongoing skill development to stay competitive and responsive to business needs.
13. Building a Successful Data Science Team: Tips and Best Practices
Establishing a well-functioning data science team is essential for organizations looking to harness data-driven insights to drive growth, innovation, and strategic decision-making. A successful data science team is not only about gathering the right talent but also involves fostering a collaborative culture, providing ongoing training, and aligning the team’s objectives with business goals. Here’s a comprehensive guide on building a data science team that can achieve impactful results.
1. Define Clear Goals and Objectives
Setting clear objectives ensures that the team’s efforts align with the organization’s strategic priorities, maximizing the impact of data-driven insights.
- Identify Key Business Problems:
- Outline specific business problems or areas where data science can add value, such as customer segmentation, predictive analytics, or operational optimization.
- Example: A retail company setting a goal for the data science team to develop a customer churn model to improve retention rates.
- Set Measurable Objectives:
- Develop quantifiable targets to track progress, like increasing conversion rates, reducing operational costs, or improving customer satisfaction.
- Example: A telecommunications company aiming to use predictive analytics to reduce customer churn by 15% within a year.
- Prioritize Projects by ROI:
- Select projects with the highest potential return on investment, balancing quick wins with long-term strategic initiatives.
- Example: Focusing on improving product recommendation algorithms that can immediately boost e-commerce sales.
2. Assemble a Diverse and Skilled Team
Building a diverse team with complementary skill sets ensures that the team can tackle complex problems from multiple angles, leveraging different expertise areas.
- Hire for Core Roles:
- Essential roles include Data Scientists, Data Engineers, Machine Learning Engineers, Data Analysts, and BI Analysts.
- Example: A finance company recruiting data engineers to manage data pipelines and data scientists to focus on developing machine learning models.
- Seek a Blend of Technical and Business Skills:
- While technical skills are foundational, hiring team members with domain-specific knowledge and business acumen is crucial.
- Example: In a healthcare setting, recruiting data scientists with experience in patient data management ensures compliance with healthcare regulations and enhances relevant insights.
- Promote Diversity in Backgrounds:
- Diverse educational and professional backgrounds can bring fresh perspectives to problem-solving and innovation.
- Example: A diverse team comprising statisticians, engineers, and business strategists can approach a marketing optimization project with varied methodologies.
3. Establish a Collaborative Team Culture
Creating a collaborative culture fosters knowledge sharing, minimizes silos, and enhances team productivity and morale.
- Encourage Open Communication:
- Foster an environment where team members feel comfortable sharing ideas, asking questions, and offering feedback.
- Example: A weekly team meeting where each member discusses their current projects and any challenges they face.
- Promote Cross-Functional Collaboration:
- Collaborate with other departments such as marketing, sales, and product to ensure alignment with company goals and data-driven decision-making.
- Example: Data scientists working directly with the product team to develop features based on user behavior insights.
- Use Agile Methodologies:
- Adopting Agile practices, such as sprints and regular stand-ups, helps manage workload, fosters teamwork, and ensures projects progress efficiently.
- Example: A data science team using Scrum with two-week sprints to incrementally develop a recommendation system.
4. Invest in Ongoing Training and Development
In the rapidly evolving field of data science, continuous learning is essential. Providing opportunities for upskilling keeps the team competitive and engaged.
- Technical Training and Certification Programs:
- Offer training in popular tools, languages, and frameworks such as TensorFlow, Python, SQL, and big data technologies like Hadoop and Spark.
- Example: A machine learning engineer completing a certification in deep learning to enhance their capabilities in developing neural networks.
- Soft Skills Development:
- Encourage training in communication, presentation, and teamwork skills, which are vital for working in collaborative environments and presenting findings effectively.
- Example: A workshop on data storytelling techniques that helps data analysts communicate complex insights to non-technical stakeholders.
- Encourage Participation in Industry Conferences:
- Support team attendance at data science conferences and events to stay updated on the latest trends and network with industry experts.
- Example: Sending team members to the NeurIPS conference to learn about cutting-edge advancements in machine learning.
5. Foster a Data-Driven Decision-Making Culture
Integrating data-driven thinking across the organization enables a data science team to be more impactful and align its projects with broader business objectives.
- Involve Data Science in Strategic Planning:
- Ensure data science leaders are part of strategic discussions and decision-making to identify areas where data insights can drive growth.
- Example: Including the Head of Data Science in quarterly planning meetings to discuss how predictive models could improve inventory forecasting.
- Educate Non-Technical Teams on Data Literacy:
- Providing data literacy training for non-technical teams helps them understand data insights and collaborate effectively with data scientists.
- Example: Offering workshops for sales teams on understanding data dashboards and interpreting metrics like customer lifetime value.
- Promote Transparency with Data Access:
- Allow relevant departments access to data dashboards and visualizations, fostering transparency and enabling data-backed decision-making across teams.
- Example: Giving product managers access to customer engagement data, enabling them to make informed feature development decisions.
6. Ensure Robust Data Infrastructure and Tools
Equipping the data science team with the right tools and infrastructure ensures efficient data processing, analysis, and model deployment.
- Invest in Scalable Data Storage Solutions:
- Use scalable data storage options such as cloud databases and data lakes (e.g., AWS S3, Google BigQuery) to handle large volumes of data.
- Example: An e-commerce company using AWS Redshift to store massive volumes of transaction data for real-time analysis.
- Provide Advanced Data Processing Tools:
- Utilize distributed processing tools like Apache Spark and Hadoop for handling large datasets efficiently.
- Example: A Data Engineer using Spark to preprocess large amounts of sensor data for predictive maintenance modeling.
- Establish Model Deployment Pipelines:
- Implement CI/CD pipelines to streamline model deployment, allowing faster and more reliable deployment of machine learning models.
- Example: A Machine Learning Engineer automating model deployment to a cloud environment using tools like Kubernetes and Docker.
7. Monitor Performance and Adjust Strategies
Regularly evaluating the team’s performance ensures that data science initiatives are meeting business goals and allows for iterative improvements.
- Use Key Performance Indicators (KPIs):
- Define KPIs to measure the team’s impact, such as time-to-insight, project completion rate, and accuracy of models.
- Example: Tracking the accuracy of a fraud detection model, with KPIs on false positive and negative rates, to ensure optimal performance.
- Collect Stakeholder Feedback:
- Gather feedback from internal stakeholders on how data products are supporting their needs, allowing for refinements and enhancements.
- Example: A Data Product Manager obtaining feedback from the marketing team on the effectiveness of a customer segmentation model.
- Hold Regular Performance Reviews:
- Conduct monthly or quarterly reviews to assess project outcomes, resource allocation, and any challenges the team may face.
- Example: A quarterly review meeting that assesses the success of a recent data-driven campaign and identifies areas for improvement.
8. Emphasize Ethical Considerations and Data Privacy
Data ethics and privacy are critical considerations, particularly when handling sensitive or personal data.
- Implement Data Privacy Policies:
- Ensure the team adheres to data privacy regulations like GDPR and CCPA, protecting user data and building trust.
- Example: A data science team in the healthcare industry strictly following HIPAA guidelines for patient data privacy.
- Promote Fairness and Bias Mitigation:
- Regularly audit models for potential biases and implement fair practices to avoid discriminatory outcomes.
- Example: A data scientist testing a hiring algorithm to ensure it does not favor candidates based on race, gender, or other protected attributes.
- Foster Transparency in Model Interpretability:
- Prioritize transparency by choosing interpretable models where possible and explaining decisions made by complex models.
- Example: A credit risk model with explainable AI techniques, allowing bank customers to understand why certain applications were approved or denied.
By following these best practices, organizations can build a data science team that’s not only skilled but also aligned with business goals, adaptable to industry trends, and equipped to make data-driven decisions ethically and effectively. A well-rounded data science team can transform raw data into actionable insights that support innovation, drive revenue, and create a competitive advantage in today’s data-centric world.
14. Challenges in Managing a Data Science Team
Managing a data science team comes with unique challenges that stem from the complexity of the work, the need for interdisciplinary expertise, and the rapid evolution of technology. Understanding these challenges helps managers support their teams effectively, ensuring they stay aligned with organizational goals while also fostering an environment where data scientists can thrive. Here’s an in-depth look at some common challenges faced in managing a data science team and strategies to overcome them.
1. Bridging the Gap Between Business Goals and Technical Capabilities
Ensuring alignment between the data science team’s projects and the company’s strategic objectives can be challenging, especially given the technical nature of data science.
- Challenge of Translating Business Needs into Data Projects:
- Data scientists may struggle to fully understand business objectives or stakeholders may not grasp the technical limitations of data science.
- Example: A data science team might develop a model for customer segmentation, but without clear business goals, the segmentation criteria may not align with marketing or sales strategies.
- Lack of Business Context:
- Data scientists often lack a deep understanding of the industry context or customer perspective, which can hinder their ability to create valuable models.
- Solution: Encourage cross-functional meetings and workshops that help data scientists understand business priorities and customer pain points.
- Solution – Assigning a Data Translator:
- Consider hiring or assigning a “data translator” role that bridges the gap, communicating effectively with both business stakeholders and technical teams.
- Example: A retail company appointing a product manager with a data background to facilitate clear communication between the data science and marketing teams.
2. Hiring and Retaining Specialized Talent
Data science requires a combination of skills, and finding individuals with expertise in data engineering, statistics, machine learning, and domain-specific knowledge can be a challenge.
- High Demand for Data Science Professionals:
- The talent pool for data scientists is competitive, and organizations often struggle to attract and retain skilled individuals due to high demand.
- Example: A tech startup competing with larger companies that can offer higher salaries and extensive benefits to attract data scientists.
- Solution – Offer Career Development Opportunities:
- Providing opportunities for growth, such as certifications, conferences, and advanced training, can make your organization more attractive to data scientists.
- Example: A company offering to cover expenses for its data science team members to attend conferences like NeurIPS or KDD for skill development and networking.
- Challenge of Cross-Functional Skills:
- Data scientists with both technical expertise and domain knowledge are hard to find. For instance, healthcare companies often require data scientists with clinical knowledge to make informed models.
- Solution: Upskill data scientists with domain training or recruit domain experts and train them in data science basics.
3. Managing Data Quality and Availability
Data science teams rely heavily on data availability and quality, and any issues with data can stall projects or lead to flawed models.
- Data Quality Issues:
- Incomplete, inconsistent, or outdated data can lead to erroneous insights and impact model accuracy.
- Example: A banking data science team building a fraud detection model may face challenges if transaction data is not updated in real-time, resulting in potential missed fraud cases.
- Data Accessibility Across Systems:
- Data often resides in siloed systems, which complicates access and integration, particularly in larger organizations.
- Solution: Develop a unified data warehouse or data lake where all relevant data sources are centralized, allowing data scientists easy access.
- Need for Data Engineering Support:
- Without sufficient data engineering resources, data scientists may spend excessive time on data cleaning and preparation, reducing their focus on modeling.
- Example: Hiring data engineers to handle data pipelines so data scientists can focus on analysis, as seen in e-commerce companies where timely data updates are crucial for demand forecasting.
4. Ensuring Model Interpretability and Ethical Use of Data
With growing concerns over data privacy and the ethical implications of AI, managers face challenges ensuring that models are interpretable, ethical, and fair.
- Balancing Model Complexity with Interpretability:
- Highly complex models like deep neural networks may provide accurate predictions but lack transparency, making it hard to explain their outcomes to stakeholders.
- Solution: Use interpretable models where possible or employ techniques like SHAP (SHapley Additive exPlanations) for explaining complex models.
- Ethical Considerations and Bias Mitigation:
- Ensuring that models do not unintentionally introduce biases, particularly in fields like hiring, lending, or law enforcement.
- Example: A hiring algorithm may favor certain demographics if the training data reflects existing biases. Regular audits are essential to mitigate this risk.
- Complying with Data Privacy Regulations:
- Compliance with regulations like GDPR and CCPA requires careful data handling, which can complicate data science processes.
- Solution: Collaborate closely with legal teams and implement anonymization or de-identification techniques to safeguard personal data.
5. Handling Expectations and Communicating Results to Stakeholders
Stakeholders may have high expectations for quick results, which may not be realistic given the complexities of data science projects.
- Challenge of Managing Unrealistic Expectations:
- Data science projects often require extensive time for data processing, model training, and testing, which can conflict with stakeholders’ timelines.
- Example: Executives expecting a fully operational recommendation engine within weeks, whereas the actual timeline requires several months for data collection and model fine-tuning.
- Solution – Set Clear Timelines and Milestones:
- Break down data science projects into smaller milestones and provide stakeholders with regular updates on progress and potential delays.
- Example: A project timeline for a sentiment analysis tool broken into phases such as data collection, model selection, testing, and deployment.
- Communicating Technical Results to Non-Technical Stakeholders:
- Data science findings are often complex, making it challenging to present insights in a way that is actionable and understandable for non-technical teams.
- Solution: Train data scientists in data storytelling and visualization, using tools like Tableau or Power BI to make results more accessible.
6. Keeping Up with Rapid Technological Changes
The fast pace of technological advancements in data science means that team skills and tools can quickly become outdated.
- Challenge of Tool and Framework Obsolescence:
- Data science tools and libraries evolve rapidly, and relying on outdated software can hinder team performance.
- Solution: Regularly assess and update the tech stack, ensuring the team is using the latest tools like TensorFlow, PyTorch, or Scikit-Learn for machine learning.
- Need for Continuous Learning:
- Data science team members need to keep learning new methods, languages, and tools to stay effective, which can be time-consuming.
- Example: An organization supporting its data scientists in learning cloud-based ML platforms like AWS SageMaker or Google AI Platform to stay competitive.
- Balancing Productivity with Experimentation:
- Staying updated can sometimes mean experimenting with new methodologies, which may slow down short-term productivity.
- Solution: Designate time for experimentation and skill development, so team members stay updated without impacting project timelines.
7. Resource Allocation and Budget Constraints
Data science projects require substantial resources, from computing power to skilled personnel, which can strain budgets and resources.
- High Costs of Infrastructure and Tools:
- Running data science models, especially those with large datasets, requires powerful computing resources, which can be costly.
- Example: A media company facing high AWS costs when running data-intensive models for content recommendation, leading them to seek optimization solutions.
- Balancing Project Costs with Expected ROI:
- Determining which projects yield the highest ROI is critical, especially when resources are limited.
- Solution: Conduct feasibility studies and prioritize projects that align with business priorities and have a high likelihood of success.
- Challenge of Resource Sharing in Multi-Team Environments:
- In large organizations, multiple teams may require access to limited resources like cloud storage or GPU clusters.
- Example: Scheduling time blocks for each team or department to access computational resources to prevent bottlenecks.
8. Maintaining Team Morale and Motivation
Data science projects can be challenging and time-consuming, and without support, data scientists may face burnout.
- Challenge of Complex Problem Solving:
- Data science projects often involve complex problem-solving, requiring persistence and resilience, which can lead to frustration and stress.
- Solution: Offer mentorship and support, allowing team members to discuss challenges and brainstorm solutions collaboratively.
- Solution – Recognize and Celebrate Successes:
- Acknowledge small wins and project milestones, which can boost morale and give a sense of accomplishment.
- Example: Celebrating the successful deployment of a fraud detection model or acknowledging a data scientist for optimizing an existing model’s accuracy.
- Promote a Healthy Work-Life Balance:
- Encourage reasonable work hours and avoid setting unrealistic deadlines that can lead to burnout.
- Example: A manager limiting weekend work for data scientists working on intensive projects, providing them with downtime to recharge.
By recognizing and proactively addressing these challenges, managers can create an environment that supports the data science team’s success. With thoughtful leadership, well-aligned goals, and the right tools and strategies, organizations can empower their data science teams to drive meaningful business impact.
Conclusion
In today’s data-driven world, the role of a well-structured, skilled data science team has become central to a company’s ability to innovate, make data-backed decisions, and stay competitive. This guide has outlined the essential roles that bring unique value to a data science team—from the strategic perspective of a data product manager to the technical expertise of data engineers, data scientists, machine learning engineers, and beyond. Each role contributes vital skills and perspectives to the team, enabling businesses to fully leverage the potential of data science.
As more organizations recognize the value of data-driven insights, understanding the responsibilities and interactions between these roles becomes essential. Here are some key takeaways:
- Specialized Roles Enable Comprehensive Solutions:
- Each role within the data science team plays a unique part, whether it’s structuring data, developing machine learning models, or interpreting results for business insights.
- For example, data engineers ensure clean, accessible data pipelines, while machine learning engineers bring algorithms to life. Together, they build solutions that are technically sound and aligned with business needs.
- Collaboration and Communication Drive Success:
- Effective communication and collaboration across roles—from statisticians who understand data variability to business intelligence analysts who focus on data-driven strategy—are critical for success.
- This cross-functional teamwork ensures that data science projects align with organizational goals and deliver actionable insights that drive business value.
- Adapting to New Technologies and Trends is Key:
- Data science is an ever-evolving field with rapid advancements in tools and techniques. Teams that commit to ongoing learning and adaptation will be better positioned to capitalize on emerging opportunities, such as advancements in artificial intelligence, data processing speed, and cloud-based platforms.
- Regular training, upskilling, and resource allocation for the latest technologies are essential to stay competitive.
- Building the Right Team Requires Strategic Investment:
- Developing a high-performing data science team requires careful consideration of both technical and soft skills, including data literacy, problem-solving, and a strong understanding of the business context.
- Investing in the right mix of talent and fostering a supportive environment enables teams to work efficiently and achieve better outcomes.
- Addressing Challenges in Data Science Management:
- Managing a data science team brings unique challenges, from aligning project goals with business objectives to managing high expectations and ensuring ethical use of data.
- Organizations that anticipate these challenges and proactively implement solutions—such as assigning data translators, promoting clear communication, and creating accessible data infrastructure—will experience smoother project workflows and greater overall impact.
In conclusion, a successful data science team is not only defined by the individual skills of its members but by their collective ability to work together toward strategic business outcomes. Each role, from data analyst to data architect, contributes essential expertise that helps transform raw data into powerful insights. By understanding and investing in these roles, businesses can create a resilient data science team capable of turning data into a valuable asset that drives growth, innovation, and sustainable success.
As data science continues to evolve, organizations that prioritize structured, well-defined roles and foster a culture of collaboration and continuous learning will be better equipped to navigate challenges and seize new opportunities. This investment in a comprehensive data science team is more than a technical necessity—it is a strategic advantage that can propel companies to the forefront of their industries.
If you find this article useful, why not share it with your hiring manager and C-level suite friends and also leave a nice comment below?
We, at the 9cv9 Research Team, strive to bring the latest and most meaningful data, guides, and statistics to your doorstep.
To get access to top-quality guides, click over to 9cv9 Blog.
People Also Ask
What are the essential roles in a data science team?
The essential roles in a data science team include data scientists, data engineers, machine learning engineers, data analysts, business intelligence analysts, statisticians, data product managers, and data architects. Each role contributes unique skills to the overall success of data-driven projects.
What does a data scientist do?
A data scientist analyzes complex data sets to extract insights, develop predictive models, and inform strategic decisions. They use statistical techniques, programming languages, and machine learning algorithms to transform data into actionable insights for businesses.
What are the responsibilities of a data engineer?
Data engineers design and maintain the data architecture, ensuring data is collected, processed, and stored efficiently. They build data pipelines, integrate various data sources, and optimize database systems for data access and analysis.
How does a machine learning engineer contribute to a data science team?
Machine learning engineers develop algorithms and models that enable machines to learn from data. They focus on implementing and optimizing predictive models, ensuring they perform well in production environments while maintaining scalability and reliability.
What is the role of a data analyst?
Data analysts interpret data to provide insights that support decision-making. They use statistical tools to analyze trends, create visualizations, and generate reports, helping organizations understand their performance and identify opportunities for improvement.
What does a business intelligence (BI) analyst do?
BI analysts focus on data analysis to support business decision-making. They design and develop dashboards, perform data visualization, and conduct data mining to help organizations uncover insights and track key performance indicators (KPIs).
What skills are essential for a data scientist?
Key skills for a data scientist include proficiency in programming languages (like Python and R), strong statistical knowledge, experience with machine learning algorithms, data visualization expertise, and excellent problem-solving abilities.
Why is a data engineer important in a data science team?
Data engineers are crucial because they ensure that the data pipeline is robust, reliable, and scalable. They prepare and manage data so that data scientists and analysts can focus on extracting insights and building models without data quality issues.
What tools do machine learning engineers use?
Machine learning engineers commonly use tools and frameworks like TensorFlow, PyTorch, Scikit-learn, and Keras for building models. They also rely on programming languages such as Python and R for data manipulation and analysis.
How can data analysts add value to a company?
Data analysts provide valuable insights by identifying trends and patterns in data. Their analyses help inform business strategies, optimize operations, and improve customer experiences, ultimately driving better decision-making.
What qualifications should a business intelligence analyst have?
A BI analyst typically holds a degree in business, computer science, or a related field. Skills in data visualization tools (like Tableau or Power BI), SQL, and an understanding of data warehousing concepts are also essential for this role.
What is the role of a statistician in a data science team?
Statisticians apply statistical theories and methods to collect, analyze, and interpret data. They provide insights on data variability and help design experiments, ensuring that analyses are scientifically valid and reliable.
How does a data product manager contribute to a data science team?
Data product managers bridge the gap between technical teams and business stakeholders. They define product vision, prioritize features based on user needs, and ensure that data science projects align with business objectives.
What is a data architect’s role in a data science team?
Data architects design and manage the overall data infrastructure, ensuring it supports analytics and reporting needs. They develop data models, establish data governance policies, and ensure the security and integrity of data systems.
How does collaboration enhance a data science team’s effectiveness?
Collaboration fosters communication and knowledge sharing among team members, enabling diverse perspectives to inform data analyses. It leads to better problem-solving and helps align projects with business goals, increasing overall impact.
What are common challenges faced by data science teams?
Common challenges include data quality issues, lack of clear project goals, difficulties in collaboration, and managing evolving technology stacks. Addressing these challenges is crucial for the team’s success and efficiency.
What tools do data scientists typically use?
Data scientists use a variety of tools, including programming languages (Python, R), data visualization software (Tableau, Matplotlib), and data manipulation libraries (Pandas, NumPy) for data analysis and visualization.
What educational background is best for a data scientist?
A strong educational background in mathematics, statistics, computer science, or a related field is ideal for a data scientist. Many also pursue advanced degrees or specialized certifications in data science or analytics.
How do data engineers ensure data quality?
Data engineers implement data validation checks, maintain data pipelines, and monitor data integrity throughout the collection and processing stages. They establish protocols to catch errors early and ensure consistent data quality.
What soft skills are important for a data science team?
Soft skills such as effective communication, teamwork, critical thinking, and adaptability are essential for success in a data science team. These skills enhance collaboration and ensure clear conveyance of insights to stakeholders.
What role does data visualization play in data science?
Data visualization helps simplify complex data and makes insights more accessible. By presenting data visually, teams can communicate findings effectively, enabling stakeholders to grasp trends and make informed decisions quickly.
How can organizations build a successful data science team?
Organizations can build successful teams by defining clear roles, fostering a collaborative culture, investing in ongoing training, and aligning projects with business goals. Strategic hiring practices also ensure a diverse skill set within the team.
What impact does a data science team have on business strategy?
A data science team can significantly influence business strategy by providing actionable insights, identifying market trends, and optimizing operations. Their work supports data-driven decision-making and enhances competitive advantage.
How do companies measure the success of their data science teams?
Companies measure success through metrics such as project completion rates, accuracy of insights, business outcomes influenced by data initiatives, and feedback from stakeholders on the value delivered by the data science team.
What is the future of data science teams?
The future of data science teams is likely to include greater integration of AI and automation, increased emphasis on ethical data practices, and a growing demand for real-time analytics. Teams will need to adapt to rapidly changing technologies and methodologies.
How can continuous learning benefit data science professionals?
Continuous learning helps data science professionals stay current with emerging technologies and methodologies, improving their skill sets. It fosters innovation, enhances problem-solving abilities, and keeps teams competitive in a rapidly evolving field.