Key Takeaways
- Enhanced Candidate Matching: Semantic search leverages AI and NLP to understand the context of job descriptions and resumes, resulting in more accurate and relevant candidate matches.
- Improved Efficiency: By automating the initial screening process, semantic search saves recruiters time and resources, allowing them to focus on engaging with the most qualified candidates.
- Future Trends: Advancements in AI and machine learning will continue to evolve semantic search, offering deeper contextual understanding, multilingual capabilities, and seamless integration with HR technologies.
In the fast-paced world of recruitment, where efficiency and precision are paramount, staying ahead of technological advancements can be the key to securing top talent.
Traditional recruitment methods, heavily reliant on keyword searches and manual screening, are increasingly being supplemented, and in many cases replaced, by more sophisticated technologies.
One such groundbreaking innovation is semantic search.
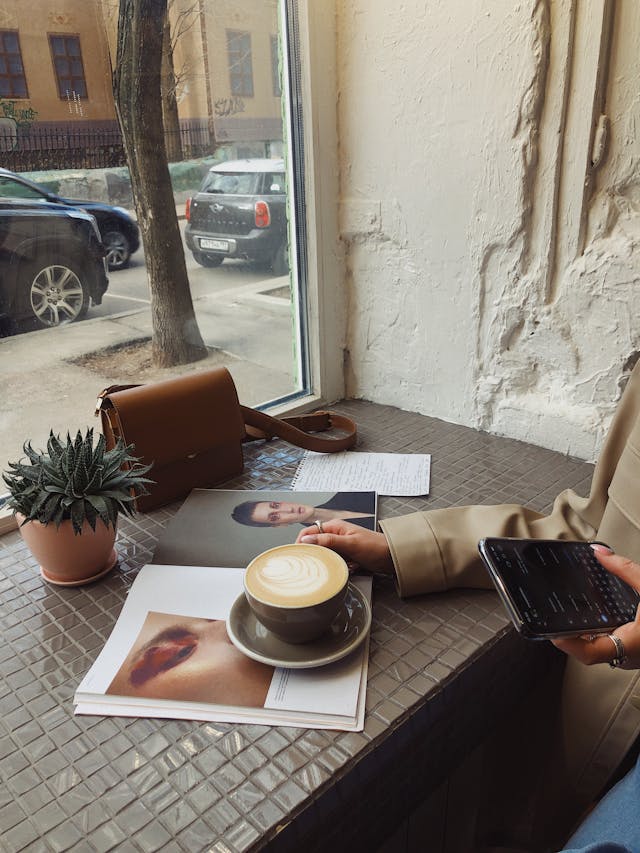
But what exactly is semantic search, and how does it revolutionize the recruitment landscape?
Semantic search represents a significant leap forward from conventional keyword-based search techniques.
While keyword searches look for exact matches to the terms entered, semantic search delves deeper, understanding the intent and contextual meaning behind the words.
This shift allows for more nuanced and accurate matches between job descriptions and candidate profiles, drastically improving the recruitment process’s effectiveness.
The roots of semantic search lie in the broader field of artificial intelligence (AI), encompassing technologies like natural language processing (NLP) and machine learning (ML).
These technologies enable systems to comprehend language in a way that mimics human understanding, recognizing synonyms, related terms, and the broader context in which words are used.
For recruiters, this means no longer being limited by the precise wording of a resume or job post, but instead being able to identify the best candidates based on a deeper understanding of skills, experience, and potential.
The adoption of semantic search in recruitment is more than just a technological upgrade; it’s a paradigm shift that brings numerous benefits to both recruiters and job seekers.
For recruiters, it means enhanced accuracy in candidate screening, saving valuable time and resources. No longer do recruiters need to sift through countless resumes that only superficially match the job requirements.
Instead, semantic search algorithms can highlight the most relevant candidates, even those who might have been overlooked by traditional methods due to unconventional wording or varied terminology.
Job seekers, on the other hand, gain from this technology through more relevant job recommendations and improved chances of being discovered for roles that truly match their skills and experiences.
This democratization of opportunity ensures that talent is recognized based on merit and relevance rather than merely on keyword matching.
To understand the full impact of semantic search, it’s essential to explore how it works, the components involved, and the practical implications for the recruitment industry.
This blog will delve into the intricacies of semantic search, elucidating its mechanisms, the benefits it offers, and the best practices for its implementation.
We’ll also look ahead to the future, examining emerging trends and potential challenges that may shape the next phase of recruitment technology.
In a competitive job market where every decision counts, embracing semantic search can be a game-changer.
By leveraging advanced AI technologies, recruiters can make more informed decisions, enhance the candidate experience, and ultimately build stronger, more effective teams.
Join us as we explore the transformative power of semantic search in recruitment and uncover how this technology works to bridge the gap between job seekers and employers, creating a more efficient and equitable hiring process.
Before we venture further into this article, we like to share who we are and what we do.
About 9cv9
9cv9 is a business tech startup based in Singapore and Asia with a strong presence all over the world.
With over eight years of startup and business experience, and being highly involved in connecting with thousands of companies and startups, the 9cv9 team has listed some important learning points in this overview of What is Semantic Search in Recruitment and How It Works.
If your company needs recruitment and headhunting services to hire top SEO employees, you can use 9cv9 headhunting and recruitment services to hire top talents and candidates. Find out more here, or send over an email to hello@9cv9.com.
Or just post 1 free job posting here at 9cv9 Hiring Portal in under 10 minutes.
What is Semantic Search in Recruitment and How It Works
- Understanding Semantic Search
- The Mechanics of Semantic Search
- Semantic Search in Recruitment
- Implementing Semantic Search in Recruitment
- Future of Semantic Search in Recruitment
1. Understanding Semantic Search
Semantic search represents a revolutionary shift in how search technology operates, particularly in the field of recruitment.
Unlike traditional keyword-based searches, semantic search understands the intent and contextual meaning behind queries, leading to more accurate and relevant results.
To fully grasp its potential, let’s dive into its definition, history, and core components.
Definition of Semantic Search
- Semantic Search:
- Goes beyond matching keywords to understanding the context, intent, and meaning of search queries.
- Utilizes Natural Language Processing (NLP) to interpret the language nuances.
- Employs Machine Learning (ML) algorithms to improve search accuracy over time.
- Traditional Keyword-Based Search:
- Matches exact words or phrases in the search query with documents.
- Limited by the exactness of the keywords; variations or synonyms might be missed.
- Example: Searching “software engineer” only returns results containing the exact phrase “software engineer,” potentially missing relevant candidates listed as “developer” or “programmer.”
History and Development
- Early Search Technologies:
- Initial search engines relied heavily on keyword matching.
- Limited by inability to understand user intent or context.
- Evolution to Semantic Search:
- Mid-2000s: Introduction of basic NLP techniques.
- 2010s: Integration of ML and AI to enhance contextual understanding.
- Example: Google’s Hummingbird update (2013) focused on understanding searcher intent and the meaning behind queries.
How Semantic Search Works
Semantic search leverages advanced technologies to provide deeper insights and more accurate results. Here’s a look at the key mechanisms behind it:
- Natural Language Processing (NLP):
- Analyzes and understands human language.
- Breaks down text into structured data to comprehend syntax and semantics.
- Example: Recognizes that “Java” in a job listing likely refers to the programming language, not the island.
- Machine Learning (ML) Algorithms:
- Continuously learn from data to improve search relevance.
- Adapt to new language patterns and terminology over time.
- Example: Identifies trends in job titles and skills, such as understanding that “full-stack developer” includes both front-end and back-end skills.
- Ontologies and Knowledge Graphs:
- Define relationships between different concepts and entities.
- Help in understanding context and connections between terms.
- Example: A knowledge graph might link “data scientist” with related skills like “Python,” “machine learning,” and “data analysis.”
Components of Semantic Search
Understanding the core components of semantic search helps in appreciating its sophistication and effectiveness:
- Context Understanding:
- Interprets the broader context in which a term is used.
- Differentiates between homonyms based on context.
- Example: Differentiates between “lead” as a metal and “lead” as a verb meaning to guide.
- Intent Recognition:
- Identifies the purpose behind a search query.
- Ensures results align with what the user intends to find.
- Example: Recognizes that searching “jobs in NYC” implies looking for employment opportunities, not information about the city.
- Synonym and Related Term Identification:
- Identifies synonyms and related terms to broaden search results.
- Reduces the chances of missing relevant results due to varied terminology.
- Example: Understands that “customer service representative” can also be referred to as “client support specialist.”
- Entity Recognition:
- Identifies and categorizes key entities within text.
- Enhances the precision of search results by recognizing named entities.
- Example: Recognizes “Google” as a company and “San Francisco” as a location within a job description.
By comprehending these components, recruiters can better leverage semantic search to match candidates with job opportunities more effectively.
The ability to understand and process the nuances of human language and context enables semantic search to offer a transformative approach in recruitment, ensuring that both job seekers and employers find the best matches based on a deeper understanding of skills and requirements.
2. The Mechanics of Semantic Search
Understanding the mechanics of semantic search involves delving into the underlying technologies and processes that enable it to interpret and deliver highly relevant search results.
This section will explore how semantic search works, the critical technologies involved, and provide relevant examples to illustrate these concepts.
How Semantic Search Works
Semantic search operates through a combination of sophisticated technologies and algorithms designed to comprehend and interpret human language more effectively. Here’s a breakdown of the primary mechanisms:
- Natural Language Processing (NLP):
- Text Analysis:
- Breaks down sentences into their grammatical components.
- Identifies nouns, verbs, adjectives, and other parts of speech.
- Example: In the sentence “The software engineer designed a robust application,” NLP identifies “software engineer” as the subject and “designed” as the action.
- Syntax and Semantics:
- Analyzes the structure of sentences (syntax) and the meaning of words and phrases (semantics).
- Understands context and relationships between words.
- Example: Recognizes that “Java developer” and “developer proficient in Java” mean the same.
- Text Analysis:
- Machine Learning (ML) Algorithms:
- Pattern Recognition:
- Detects patterns and trends in large datasets.
- Learns from past searches and user interactions to improve future results.
- Example: Over time, ML algorithms can learn that users searching for “project manager” often also look for skills like “agile methodology” and “team leadership.”
- Continuous Learning:
- Adapts to new information and evolving language use.
- Enhances the search engine’s ability to stay current with industry terminology.
- Example: As new job titles like “DevOps engineer” emerge, ML algorithms learn to associate relevant skills and responsibilities with these titles.
- Pattern Recognition:
- Ontologies and Knowledge Graphs:
- Defining Relationships:
- Establishes connections between different entities and concepts.
- Provides a structured framework for understanding complex relationships.
- Example: A knowledge graph might link “software engineering” with related fields like “computer science,” “coding,” and “software development.”
- Contextual Information:
- Enriches search results with additional context.
- Helps in disambiguating terms with multiple meanings.
- Example: If a recruiter searches for “Java,” the knowledge graph helps the system understand whether the context is programming, coffee, or the Indonesian island.
- Defining Relationships:
Key Components of Semantic Search
Several critical components contribute to the effectiveness of semantic search.
These components work together to ensure that the search engine understands and processes information accurately.
- Context Understanding:
- Contextual Analysis:
- Assesses the broader context of a query to deliver relevant results.
- Example: Understands that a search for “Python developer in San Francisco” should prioritize job listings in the specified location.
- Disambiguation:
- Differentiates between multiple meanings of a word based on context.
- Example: Recognizes that “Apple” can refer to the fruit or the tech company depending on the query context.
- Contextual Analysis:
- Intent Recognition:
- User Intent Analysis:
- Identifies the underlying purpose of a search query.
- Example: A search for “remote jobs” indicates a preference for positions that can be performed from home.
- Query Refinement:
- Adjusts search parameters to align with user intent.
- Example: If a user searches for “entry-level marketing jobs,” the system prioritizes listings that specify “entry-level” positions in marketing.
- User Intent Analysis:
- Synonym and Related Term Identification:
- Synonym Mapping:
- Recognizes and maps synonyms to ensure comprehensive search results.
- Example: Understands that “customer support” and “client service” are interchangeable terms.
- Related Terms and Concepts:
- Identifies related terms to broaden the scope of search results.
- Example: A search for “data analyst” might also return results for “business intelligence analyst” or “data scientist” roles.
- Synonym Mapping:
- Entity Recognition:
- Named Entity Recognition (NER):
- Identifies and classifies entities such as names, dates, and organizations within the text.
- Example: In a resume, NER can extract entities like “Google” (company) and “Harvard University” (institution).
- Entity Linking:
- Connects entities with additional information to provide richer search results.
- Example: Linking “Google” to its industry, location, and relevant job openings.
- Named Entity Recognition (NER):
Practical Examples in Recruitment
To illustrate how these components come together in real-world recruitment scenarios, consider the following examples:
- Improved Candidate Matching:
- A recruiter searching for a “machine learning engineer with experience in healthcare” receives results that prioritize candidates with relevant experience in both machine learning and the healthcare industry, thanks to NLP and ML algorithms understanding the context and intent.
- Enhanced Job Descriptions:
- Job descriptions enriched with semantic search technology can better capture the required skills and responsibilities. For example, a job post for a “product manager” might automatically include related terms like “project management,” “agile methodology,” and “cross-functional team leadership.”
- Diverse Candidate Pools:
- By recognizing synonyms and related terms, semantic search ensures that candidates with varied backgrounds but relevant skills are not overlooked. For example, candidates who list “content creation” rather than “content writing” are still matched to writing-focused roles.
Understanding these mechanics provides insight into how semantic search transforms recruitment, making the process more efficient, accurate, and inclusive.
By leveraging NLP, ML, ontologies, and knowledge graphs, semantic search goes beyond mere keyword matching to truly understand and meet the needs of both recruiters and job seekers.
3. Semantic Search in Recruitment
Semantic search has revolutionized the recruitment industry by enhancing the precision and efficiency of matching candidates with job opportunities.
By understanding the intent and context behind search queries, semantic search technology can identify the best candidates and job roles more effectively than traditional keyword-based searches.
This section explores the various facets of semantic search in recruitment, its benefits for both recruiters and job seekers, and provides relevant examples to illustrate its impact.
Role of Semantic Search in Recruitment
Semantic search plays a pivotal role in improving the recruitment process by leveraging advanced technologies to understand and match candidates with job openings more accurately.
- Improving Candidate Matching:
- Contextual Understanding:
- Analyzes the full context of candidate profiles and job descriptions.
- Matches candidates based on skills, experiences, and qualifications that align with job requirements.
- Example: A candidate with “data science” experience is matched to a “machine learning” role, recognizing the overlap in skill sets.
- Intent Recognition:
- Identifies the intent behind job searches and candidate applications.
- Delivers more relevant job recommendations to candidates.
- Example: A search for “remote software engineering jobs” returns roles specifically designed for remote work.
- Contextual Understanding:
- Enhancing Job Description Accuracy:
- Synonym Recognition:
- Recognizes synonyms and related terms in job descriptions and resumes.
- Ensures comprehensive matching by considering various terminologies.
- Example: A job description for a “customer service representative” also includes candidates who list “client support specialist” as their job title.
- Skill Mapping:
- Maps out related skills and competencies required for job roles.
- Helps in creating more detailed and accurate job descriptions.
- Example: For a “marketing manager” role, the system identifies and includes skills like “SEO,” “content marketing,” and “social media management.”
- Synonym Recognition:
Benefits for Recruiters
Semantic search technology offers numerous advantages for recruiters, enabling them to streamline the hiring process and find the best candidates more efficiently.
- Time-Saving Through Better Candidate Screening:
- Automated Screening:
- Automatically screens and ranks candidates based on relevance to the job description.
- Reduces the time spent on manual resume reviews.
- Example: A recruiter looking for a “project manager” can quickly narrow down candidates with specific experience in “agile project management.”
- Prioritizing Top Matches:
- Highlights candidates who most closely match the job requirements.
- Allows recruiters to focus on the most promising candidates first.
- Example: Candidates with certifications or specialized training relevant to the job are given higher priority in search results.
- Automated Screening:
- Increased Relevancy in Candidate Pools:
- Enhanced Search Accuracy:
- Delivers more accurate and relevant candidate matches by understanding the nuances of job descriptions.
- Example: For a “data analyst” position, the system identifies candidates with relevant analytical tools and techniques, such as “SQL,” “Python,” and “data visualization.”
- Broadening Search Parameters:
- Considers a wider range of relevant candidates by recognizing related terms and skills.
- Example: A search for “software developer” includes candidates with titles like “programmer” and “coder,” broadening the candidate pool.
- Enhanced Search Accuracy:
Benefits for Job Seekers
Job seekers also gain significantly from semantic search technology, as it helps them discover more relevant job opportunities and improves their visibility to recruiters.
- More Accurate Job Recommendations:
- Personalized Job Matches:
- Provides job seekers with recommendations that closely match their skills and experiences.
- Example: A job seeker with a background in “digital marketing” receives recommendations for roles in “social media management” and “SEO specialist.”
- Understanding Career Aspirations:
- Recognizes career goals and preferences to deliver tailored job suggestions.
- Example: A candidate looking for career growth in “project management” is shown opportunities for “senior project manager” or “program manager” roles.
- Personalized Job Matches:
- Enhanced Visibility for Diverse Skill Sets:
- Highlighting Transferable Skills:
- Identifies and highlights transferable skills that may be relevant to different job roles.
- Example: A teacher transitioning to a corporate role is matched with training and development positions due to their instructional skills.
- Equal Opportunity Matching:
- Ensures that candidates with diverse backgrounds and varied terminologies are not overlooked.
- Example: A candidate using non-standard terminology in their resume is still matched to relevant job openings based on skill equivalence.
- Highlighting Transferable Skills:
Implementing Semantic Search in Recruitment
To leverage the full potential of semantic search, recruiters and organizations need to adopt the right tools and follow best practices for implementation.
- Tools and Technologies:
- Popular Semantic Search Tools:
- Utilize leading semantic search platforms and tools designed for recruitment.
- Example: Tools like LinkedIn Talent Insights and Hiretual offer advanced semantic search capabilities for recruiters.
- Case Studies of Successful Implementations:
- Highlight real-world examples of organizations that have successfully implemented semantic search.
- Example: A multinational corporation improved its hiring process by integrating a semantic search engine, reducing time-to-hire by 30%.
- Popular Semantic Search Tools:
- Best Practices:
- Maximizing Benefits:
- Provide comprehensive training for recruiters on using semantic search tools.
- Continuously update job descriptions and candidate profiles to reflect current terminology and skills.
- Example: Regularly reviewing and updating job postings to include emerging skills and industry trends.
- Avoiding Common Pitfalls:
- Ensure data privacy and compliance with regulations when using AI and ML technologies.
- Avoid over-reliance on automated systems by incorporating human judgment in the final decision-making process.
- Example: Balancing automated candidate screening with human interviews to ensure cultural fit and personal evaluation.
- Maximizing Benefits:
Future of Semantic Search in Recruitment
The future of semantic search in recruitment is shaped by ongoing technological advancements and emerging trends that promise to further enhance the hiring process.
- Emerging Trends:
- AI Advancements:
- Continuous improvements in AI and ML will lead to even more accurate and intuitive semantic search capabilities.
- Example: The integration of AI-powered chatbots that use semantic search to interact with candidates and provide real-time job recommendations.
- Integration with Other HR Technologies:
- Seamless integration with other HR technologies such as applicant tracking systems (ATS) and HR information systems (HRIS).
- Example: A unified platform where semantic search capabilities are integrated into the ATS, providing a streamlined end-to-end recruitment process.
- AI Advancements:
- Potential Challenges:
- Data Privacy Concerns:
- Addressing data privacy and security issues associated with handling large volumes of personal data.
- Example: Ensuring compliance with regulations like GDPR and CCPA when implementing semantic search solutions.
- Keeping Up with Technological Advancements:
- Staying updated with rapid technological changes and continuously upgrading systems.
- Example: Regularly updating AI models and algorithms to reflect the latest industry standards and best practices.
- Data Privacy Concerns:
By understanding and effectively implementing semantic search, recruiters can significantly enhance their ability to find the best candidates quickly and accurately.
This technology not only streamlines the hiring process but also ensures a better match between job seekers and employers, ultimately leading to more successful and satisfying employment outcomes.
4. Implementing Semantic Search in Recruitment
Implementing semantic search in recruitment requires careful planning and strategic use of technology to maximize its benefits.
This section outlines the tools and technologies involved, provides best practices for implementation, and discusses common pitfalls to avoid.
By following these guidelines, recruiters can leverage semantic search to enhance their hiring processes significantly.
Tools and Technologies
A variety of tools and technologies are available to support the implementation of semantic search in recruitment.
These tools harness advanced algorithms and data processing capabilities to deliver more accurate and relevant search results.
- Popular Semantic Search Tools:
- LinkedIn Talent Insights:
- Provides deep insights into talent pools and market trends using semantic search algorithms.
- Example: Recruiters can use LinkedIn Talent Insights to find candidates with specific skills or experiences, such as “data science” or “machine learning,” even if they use different terminology.
- Hiretual:
- An AI-powered recruitment tool that utilizes semantic search to source and engage candidates.
- Example: Hiretual allows recruiters to search for “software engineers” with specific expertise in “cloud computing” and “DevOps,” presenting candidates whose profiles might not explicitly mention these terms.
- Textkernel:
- Offers semantic search and matching technology to improve candidate-job matching.
- Example: Textkernel’s semantic search can identify candidates with “project management” experience, including those who list relevant certifications like “PMP” or methodologies like “Agile.”
- LinkedIn Talent Insights:
- Case Studies of Successful Implementations:
- Multinational Corporation:
- A large multinational corporation integrated a semantic search engine into their recruitment process, reducing time-to-hire by 30%.
- Example: The corporation used semantic search to identify and prioritize candidates with emerging skills in “AI” and “blockchain,” which were crucial for their innovation projects.
- Tech Startup:
- A tech startup adopted semantic search to streamline their hiring for niche technical roles.
- Example: By implementing semantic search, the startup efficiently matched candidates with skills in “full-stack development” and “microservices architecture,” significantly improving their recruitment outcomes.
- Multinational Corporation:
Best Practices
Implementing semantic search in recruitment involves following best practices to ensure the technology is used effectively and efficiently.
- Maximizing Benefits:
- Comprehensive Training:
- Provide training for recruiters on how to use semantic search tools effectively.
- Example: Conduct workshops and webinars to help recruiters understand the capabilities and limitations of semantic search, enabling them to refine their search queries for better results.
- Regular Updates:
- Continuously update job descriptions and candidate profiles to reflect current terminology and skills.
- Example: Review and revise job postings regularly to include new industry-specific terms and emerging skills, ensuring the semantic search engine can match the most relevant candidates.
- Utilizing Feedback:
- Collect and analyze feedback from recruiters and candidates to improve the search process.
- Example: Implement a system to gather feedback on the relevance of search results, allowing the AI algorithms to learn and adapt for better future matches.
- Comprehensive Training:
- Avoiding Common Pitfalls:
- Data Privacy and Compliance:
- Ensure data privacy and compliance with regulations when using AI and ML technologies.
- Example: Implement robust data security measures and ensure compliance with GDPR, CCPA, and other relevant regulations to protect candidate information.
- Balancing Automation with Human Judgment:
- Avoid over-reliance on automated systems by incorporating human judgment in the final decision-making process.
- Example: Use semantic search to shortlist candidates, but conduct human interviews to assess cultural fit and personal qualities.
- Managing Expectations:
- Set realistic expectations about the capabilities and limitations of semantic search.
- Example: Educate stakeholders that while semantic search can significantly improve matching accuracy, it is not a replacement for comprehensive recruitment strategies.
- Data Privacy and Compliance:
Steps for Implementation
Implementing semantic search involves several key steps to ensure a smooth transition and effective utilization of the technology.
- Assessment and Planning:
- Needs Analysis:
- Conduct a thorough needs analysis to determine the specific requirements of the recruitment process.
- Example: Identify the types of roles that frequently require advanced search capabilities, such as highly specialized technical positions or roles with niche skill sets.
- Vendor Selection:
- Evaluate and select the right semantic search vendors based on your organization’s needs.
- Example: Compare features, pricing, and customer reviews of different semantic search tools to find the best fit for your recruitment process.
- Needs Analysis:
- Integration and Testing:
- System Integration:
- Integrate the semantic search tool with existing recruitment systems, such as Applicant Tracking Systems (ATS) and Human Resource Information Systems (HRIS).
- Example: Ensure seamless data flow between the semantic search tool and the ATS to streamline candidate sourcing and management.
- Pilot Testing:
- Conduct pilot tests to evaluate the effectiveness of the semantic search tool.
- Example: Run a pilot project with a specific department or type of role to gather initial insights and make necessary adjustments before full-scale implementation.
- System Integration:
- Training and Adoption:
- Recruiter Training:
- Train recruiters on how to use the new semantic search tool effectively.
- Example: Provide hands-on training sessions, user manuals, and support resources to help recruiters get comfortable with the new technology.
- Stakeholder Buy-In:
- Secure buy-in from all stakeholders, including HR managers and senior leadership.
- Example: Present the benefits and potential ROI of semantic search to stakeholders to gain their support for the initiative.
- Recruiter Training:
Monitoring and Optimization
Once implemented, continuous monitoring and optimization are crucial to maximize the effectiveness of semantic search in recruitment.
- Performance Monitoring:
- Key Metrics:
- Track key performance metrics such as time-to-hire, quality of hire, and candidate satisfaction.
- Example: Use analytics to measure the improvement in matching accuracy and the reduction in time spent on candidate screening.
- Regular Reviews:
- Conduct regular reviews to assess the performance of the semantic search tool.
- Example: Schedule quarterly reviews to evaluate search results and make adjustments based on recruiter feedback and performance data.
- Key Metrics:
- Continuous Improvement:
- Algorithm Updates:
- Regularly update the AI algorithms to incorporate new data and improve search accuracy.
- Example: Continuously feed the system with new resumes and job descriptions to refine its understanding of emerging skills and industry trends.
- Feedback Loop:
- Establish a feedback loop with recruiters and candidates to gather insights and improve the system.
- Example: Use surveys and focus groups to collect feedback on the relevance and accuracy of search results, and use this information to fine-tune the algorithms.
- Algorithm Updates:
By following these steps and best practices, organizations can successfully implement semantic search in their recruitment processes, leading to more accurate candidate matches, improved hiring efficiency, and a better overall experience for both recruiters and job seekers.
5. Future of Semantic Search in Recruitment
Semantic search is poised to dramatically transform the recruitment landscape in the coming years.
As technology advances, semantic search will become even more sophisticated, enabling more precise candidate matching and streamlined hiring processes.
This section explores the future of semantic search in recruitment, including emerging trends, technological advancements, and potential challenges.
Emerging Trends
Several emerging trends indicate the future directions of semantic search in recruitment.
These trends are driven by continuous advancements in artificial intelligence, machine learning, and data analytics.
- AI and Machine Learning Enhancements:
- Improved Contextual Understanding:
- Future AI models will have an enhanced ability to understand context, leading to even more accurate matching of candidates to job descriptions.
- Example: Advanced AI can distinguish between industry-specific jargon and general terminology, ensuring that searches for “cloud” in tech roles focus on cloud computing rather than weather-related contexts.
- Adaptive Learning Algorithms:
- Algorithms that learn and adapt in real-time based on new data and user interactions will become standard.
- Example: A recruiter searching for “UI/UX designer” will see results evolve as the system learns from recent hiring trends and candidate behaviors.
- Improved Contextual Understanding:
- Integration with Advanced HR Technologies:
- Unified Talent Management Systems:
- Semantic search will be seamlessly integrated with other HR technologies such as Applicant Tracking Systems (ATS), Human Resource Information Systems (HRIS), and performance management tools.
- Example: An ATS integrated with semantic search will automatically parse resumes, match candidates to open positions, and track their progress through the hiring pipeline.
- AI-Powered Chatbots and Virtual Assistants:
- The use of AI chatbots and virtual assistants to engage with candidates and provide real-time job recommendations will become more prevalent.
- Example: An AI chatbot could interact with job seekers on a career site, ask about their skills and preferences, and use semantic search to suggest the most relevant job openings.
- Unified Talent Management Systems:
- Personalized Candidate Experiences:
- Tailored Job Recommendations:
- Semantic search will enable highly personalized job recommendations based on a candidate’s unique skills, experiences, and career aspirations.
- Example: A candidate with experience in “digital marketing” and an interest in “data analytics” will receive recommendations for roles that combine both fields, such as “Digital Marketing Analyst.”
- Enhanced Candidate Profiles:
- Future systems will automatically enrich candidate profiles with data from various sources, providing a more holistic view of their capabilities.
- Example: Profiles might include not only resume data but also information from social media, professional networks, and industry certifications.
- Tailored Job Recommendations:
Technological Advancements
Technological advancements will drive the evolution of semantic search, making it more powerful and versatile.
These advancements will enable recruiters to harness the full potential of semantic search in their hiring processes.
- Natural Language Processing (NLP) Innovations:
- Deeper Semantic Understanding:
- Enhanced NLP techniques will enable deeper understanding of the semantics of job descriptions and resumes.
- Example: NLP models will better understand complex sentence structures and relationships between skills, ensuring accurate parsing of job requirements and candidate qualifications.
- Multilingual Capabilities:
- Future NLP models will support multiple languages, making semantic search accessible to global organizations.
- Example: A multinational company can search for candidates across different countries and languages, ensuring consistent and accurate results regardless of the language used in resumes or job descriptions.
- Deeper Semantic Understanding:
- Knowledge Graphs and Ontologies:
- Enhanced Knowledge Graphs:
- Future knowledge graphs will be more comprehensive and detailed, capturing a wider array of relationships between skills, job roles, and industries.
- Example: A knowledge graph might include intricate connections between roles like “data engineer,” “data scientist,” and “machine learning engineer,” highlighting their unique and overlapping skill sets.
- Dynamic Ontologies:
- Ontologies that dynamically update with new industry trends and emerging technologies will become standard.
- Example: As new programming languages or tools become popular, the ontology will update to include these, ensuring they are considered in search results.
- Enhanced Knowledge Graphs:
- Predictive Analytics and Insights:
- Predictive Hiring Models:
- Semantic search will incorporate predictive analytics to forecast candidate success and tenure in specific roles.
- Example: Based on historical hiring data, the system might predict which candidates are likely to succeed in a “sales manager” role based on their career trajectory and performance in similar positions.
- Data-Driven Decision Making:
- Recruiters will have access to detailed insights and analytics, helping them make more informed hiring decisions.
- Example: Data dashboards will provide metrics on candidate match quality, time-to-hire, and diversity statistics, all derived from semantic search data.
- Predictive Hiring Models:
Potential Challenges
While the future of semantic search in recruitment is promising, several challenges must be addressed to fully realize its potential.
- Data Privacy and Security:
- Compliance with Regulations:
- Ensuring compliance with data privacy regulations like GDPR and CCPA will be critical as semantic search tools handle sensitive candidate information.
- Example: Implementing robust data protection measures and obtaining explicit consent from candidates for data processing will be necessary to maintain compliance.
- Secure Data Handling:
- Protecting candidate data from breaches and unauthorized access will be a priority.
- Example: Employing advanced encryption methods and secure data storage solutions to safeguard candidate information.
- Compliance with Regulations:
- Bias and Fairness:
- Addressing Algorithmic Bias:
- Ensuring that semantic search algorithms do not perpetuate biases present in historical hiring data will be essential.
- Example: Regularly auditing and refining algorithms to prevent discrimination based on gender, race, age, or other protected characteristics.
- Promoting Diversity and Inclusion:
- Semantic search should be designed to promote diversity and inclusion in hiring practices.
- Example: Developing algorithms that prioritize diverse candidate pools and actively identify underrepresented talent.
- Addressing Algorithmic Bias:
- Keeping Pace with Rapid Technological Change:
- Continuous Learning and Adaptation:
- Staying current with rapid advancements in AI and machine learning will be necessary to keep semantic search tools effective.
- Example: Continuously updating AI models and incorporating the latest research and technological developments into the system.
- Resource Allocation:
- Allocating sufficient resources for the ongoing development and maintenance of semantic search systems.
- Example: Investing in skilled personnel and infrastructure to support the continuous improvement of semantic search capabilities.
- Continuous Learning and Adaptation:
By anticipating and addressing these challenges, organizations can ensure that they fully leverage the benefits of semantic search in recruitment, leading to more efficient and effective hiring processes.
Future Applications
The future applications of semantic search in recruitment are vast, with the potential to transform various aspects of the hiring process.
- Enhanced Talent Sourcing:
- Proactive Talent Acquisition:
- Semantic search will enable recruiters to proactively identify and engage with passive candidates.
- Example: Using semantic search to find professionals with highly sought-after skills who are not actively looking for new opportunities but match the organization’s needs.
- Talent Pool Optimization:
- Building and maintaining optimized talent pools for future hiring needs.
- Example: Creating a dynamic talent pool of “cybersecurity experts” that updates with new candidates and tracks their career progress.
- Proactive Talent Acquisition:
- Improved Candidate Experience:
- Personalized Candidate Journeys:
- Delivering personalized experiences for candidates throughout the recruitment process.
- Example: Tailoring communication and job recommendations to individual candidates based on their skills, interests, and career goals.
- Real-Time Feedback and Updates:
- Providing candidates with real-time updates and feedback on their application status.
- Example: Using AI-driven chatbots to keep candidates informed and engaged throughout the hiring process.
- Personalized Candidate Journeys:
- Strategic Workforce Planning:
- Skills Gap Analysis:
- Using semantic search to identify skills gaps within the organization and plan for future workforce needs.
- Example: Analyzing current employee skills and predicting future requirements to develop targeted training and recruitment strategies.
- Talent Mobility:
- Facilitating internal talent mobility by matching current employees with new roles and development opportunities within the organization.
- Example: Using semantic search to identify employees with the potential to transition to emerging roles like “AI specialist” or “sustainability manager.”
- Skills Gap Analysis:
The future of semantic search in recruitment is bright, with continuous advancements promising to enhance the precision, efficiency, and overall effectiveness of the hiring process.
By staying ahead of emerging trends, leveraging technological innovations, and addressing potential challenges, organizations can fully harness the power of semantic search to build stronger, more diverse, and highly skilled workforces.
Conclusion
Semantic search in recruitment represents a significant leap forward in the way organizations identify, evaluate, and engage with potential candidates.
By moving beyond traditional keyword-based searches to understand the context and intent behind queries, semantic search technology offers a more nuanced and accurate approach to talent acquisition.
This transformative capability not only streamlines the recruitment process but also enhances the quality of matches, benefiting both employers and job seekers.
The Evolution and Benefits of Semantic Search
Semantic search has evolved from basic keyword matching to sophisticated systems that leverage artificial intelligence (AI), natural language processing (NLP), and machine learning (ML).
These advancements allow semantic search engines to comprehend the deeper meanings of words, recognize synonyms, and understand complex relationships between skills and job requirements.
- Enhanced Candidate Matching:
- Semantic search improves the precision of candidate-job matching by considering the full context of resumes and job descriptions.
- Example: A search for “data analyst” returns candidates with experience in “business intelligence” and “data visualization,” recognizing these relevant skills.
- Improved Efficiency:
- By automating the initial screening process, semantic search saves recruiters valuable time, allowing them to focus on the most promising candidates.
- Example: Recruiters can quickly identify top candidates for a “software developer” position by filtering out resumes that do not meet the core requirements.
- Broader Talent Pools:
- Semantic search broadens the talent pool by recognizing diverse terminologies and related skills, ensuring no qualified candidate is overlooked.
- Example: A job description seeking “customer support” professionals will also capture candidates with “client service” experience.
- Personalized Candidate Experience:
- For job seekers, semantic search provides more accurate job recommendations tailored to their skills and career aspirations.
- Example: A marketing professional looking for growth in digital marketing receives relevant job suggestions in SEO, content marketing, and social media management.
Implementing Semantic Search in Recruitment
The successful implementation of semantic search in recruitment involves leveraging the right tools, following best practices, and continuously optimizing the process.
- Choosing the Right Tools:
- Organizations must select semantic search tools that align with their specific recruitment needs.
- Example: Tools like LinkedIn Talent Insights and Hiretual offer robust features for semantic search, helping recruiters find and engage top talent.
- Training and Adoption:
- Comprehensive training for recruiters is essential to maximize the benefits of semantic search technology.
- Example: Training sessions and workshops help recruiters understand how to effectively use semantic search tools, refining their search queries for better results.
- Continuous Monitoring and Optimization:
- Regularly monitoring performance metrics and gathering feedback ensures the semantic search system remains effective.
- Example: Quarterly reviews and updates to job descriptions and search algorithms help maintain high search accuracy and relevance.
Future Prospects and Challenges
The future of semantic search in recruitment is promising, with continuous advancements in AI, ML, and NLP paving the way for even more sophisticated and intuitive search capabilities.
However, organizations must be prepared to address potential challenges to fully realize the benefits.
- Technological Advancements:
- Future developments will enhance semantic search’s ability to understand context, support multiple languages, and integrate seamlessly with other HR technologies.
- Example: AI-powered chatbots that use semantic search to interact with candidates and provide real-time job recommendations will become more common.
- Data Privacy and Security:
- Ensuring compliance with data privacy regulations and protecting candidate information will be critical.
- Example: Implementing robust data security measures and maintaining compliance with GDPR and CCPA standards.
- Bias and Fairness:
- Addressing algorithmic bias and promoting diversity and inclusion in hiring practices will be essential.
- Example: Regular audits and updates to AI algorithms to prevent discrimination based on gender, race, age, or other protected characteristics.
Final Thoughts
Semantic search in recruitment is more than just a technological innovation; it is a strategic tool that transforms how organizations find and engage with talent.
By understanding the deeper context and intent behind search queries, semantic search bridges the gap between job seekers and employers, creating a more efficient, inclusive, and effective hiring process.
For recruiters, adopting semantic search means leveraging advanced technologies to improve hiring outcomes, save time, and enhance the candidate experience.
For job seekers, it means receiving more relevant job recommendations and having a better chance of being noticed for roles that truly match their skills and career goals.
As we look to the future, the continuous evolution of semantic search promises to bring even greater precision, efficiency, and innovation to the recruitment industry.
By embracing this technology and addressing its challenges, organizations can build stronger, more diverse, and highly skilled workforces, ultimately driving their success in an increasingly competitive talent landscape.
If your company needs HR, hiring, or corporate services, you can use 9cv9 hiring and recruitment services. Book a consultation slot here, or send over an email to hello@9cv9.com.
If you find this article useful, why not share it with your hiring manager and C-level suite friends and also leave a nice comment below?
We, at the 9cv9 Research Team, strive to bring the latest and most meaningful data, guides, and statistics to your doorstep.
To get access to top-quality guides, click over to 9cv9 Blog.
People Also Ask
What is semantic search in recruitment?
Semantic search in recruitment uses AI and NLP to understand the context of job descriptions and resumes, enabling more accurate and relevant candidate matches.
How does semantic search differ from keyword search in recruitment?
Unlike keyword search, semantic search comprehends the meaning and context of words, identifying related concepts and synonyms to deliver better matching results.
Why is semantic search important in recruitment?
Semantic search improves the efficiency and accuracy of candidate matching, reduces time-to-hire, and enhances the overall recruitment experience by finding the best-fit candidates.
What technologies power semantic search in recruitment?
Semantic search relies on artificial intelligence (AI), machine learning (ML), and natural language processing (NLP) to analyze and understand textual data.
How does semantic search improve candidate matching?
Semantic search considers the context and relationships between skills, experiences, and job requirements, resulting in more precise matches between candidates and job openings.
Can semantic search identify candidates with transferable skills?
Yes, semantic search can recognize transferable skills and related experiences, even if candidates use different terminology than what’s in the job description.
How does semantic search handle synonyms and related terms?
Semantic search algorithms are designed to understand synonyms and related terms, ensuring that searches capture a broader range of relevant candidates.
What are the benefits of using semantic search in recruitment?
Benefits include enhanced candidate matching, improved hiring efficiency, broader talent pools, and a more personalized candidate experience.
How can semantic search tools be integrated with existing recruitment systems?
Semantic search tools can be integrated with Applicant Tracking Systems (ATS) and Human Resource Information Systems (HRIS) to streamline the recruitment process.
Are there any specific semantic search tools for recruitment?
Yes, tools like LinkedIn Talent Insights, Hiretual, and Textkernel offer semantic search capabilities specifically designed for recruitment.
What role does AI play in semantic search?
AI powers semantic search by analyzing and understanding large volumes of textual data, learning from patterns, and improving search accuracy over time.
How does natural language processing (NLP) enhance semantic search?
NLP allows semantic search to understand and interpret human language, recognizing the context and meaning behind words and phrases.
Can semantic search be used for internal talent mobility?
Yes, semantic search can match current employees with new roles and development opportunities within the organization, facilitating internal talent mobility.
How does semantic search support diversity and inclusion in recruitment?
Semantic search can help identify diverse candidates by recognizing a wider range of skills and experiences, reducing bias in the hiring process.
What are the challenges of implementing semantic search in recruitment?
Challenges include ensuring data privacy and security, addressing algorithmic bias, and keeping up with rapid technological advancements.
How can recruiters maximize the benefits of semantic search?
Recruiters can maximize benefits by providing comprehensive training, regularly updating job descriptions, and continuously monitoring and optimizing search algorithms.
What is the future of semantic search in recruitment?
The future includes advancements in AI and ML, deeper contextual understanding, multilingual capabilities, and seamless integration with other HR technologies.
How does semantic search save time in the recruitment process?
Semantic search automates the initial screening of candidates, quickly identifying the best matches and reducing the time spent on manual resume reviews.
Can semantic search handle multilingual job searches?
Yes, advanced semantic search tools support multiple languages, making them suitable for global recruitment efforts.
How does semantic search improve the candidate experience?
Semantic search provides more accurate job recommendations and personalized interactions, enhancing the candidate’s journey and engagement.
What is the role of machine learning in semantic search?
Machine learning enables semantic search algorithms to learn from data, continuously improving their accuracy and effectiveness in matching candidates to jobs.
Can semantic search be customized for specific industries?
Yes, semantic search can be tailored to understand industry-specific terminology and requirements, improving relevance and accuracy for specialized roles.
How does semantic search contribute to strategic workforce planning?
Semantic search helps identify skills gaps, forecast future talent needs, and plan for workforce development, supporting strategic HR initiatives.
What metrics should be tracked to evaluate semantic search performance?
Key metrics include time-to-hire, quality of hire, candidate satisfaction, and diversity statistics, which help assess the effectiveness of semantic search.
How does semantic search support proactive talent acquisition?
Semantic search enables recruiters to identify and engage with passive candidates who are not actively looking for jobs but match the organization’s needs.
What are some common pitfalls to avoid with semantic search?
Avoid over-reliance on automation, ensure compliance with data privacy regulations, and regularly audit algorithms to prevent bias.
How can semantic search help with skill gap analysis?
Semantic search can analyze employee skills, identify gaps, and recommend training or recruitment strategies to address these gaps.
Is semantic search suitable for all types of job roles?
Yes, semantic search is versatile and can be applied to a wide range of job roles, from technical positions to managerial and executive roles.
How can organizations stay updated with the latest in semantic search technology?
Organizations can stay updated by following industry trends, attending relevant conferences, and investing in ongoing training and development for HR teams.
What impact does semantic search have on recruitment ROI?
Semantic search improves recruitment ROI by increasing hiring efficiency, reducing time-to-hire, and enhancing the quality of candidate matches, leading to better hiring outcomes.