Key Takeaways
- Understanding Data-Driven Recruitment: Data-driven recruitment uses analytics, AI, and technology to streamline hiring, improve candidate selection, and enhance decision-making.
- How It Works: By leveraging tools like predictive analytics and ATS, organizations can optimize sourcing, reduce biases, and predict hiring success rates effectively.
- Future Trends and Benefits: Data-driven recruitment fosters efficiency, diversity, and cost-effectiveness while embracing trends like AI automation and personalized hiring strategies.
In today’s fast-paced, competitive job market, the recruitment process has evolved far beyond traditional methods.
The days of solely relying on resumes and gut instincts are being replaced by a new era of precision, efficiency, and informed decision-making.
This transformation is being led by data-driven recruitment, an approach that leverages technology, analytics, and actionable insights to streamline hiring processes, enhance decision-making, and ultimately connect the right talent with the right opportunities.
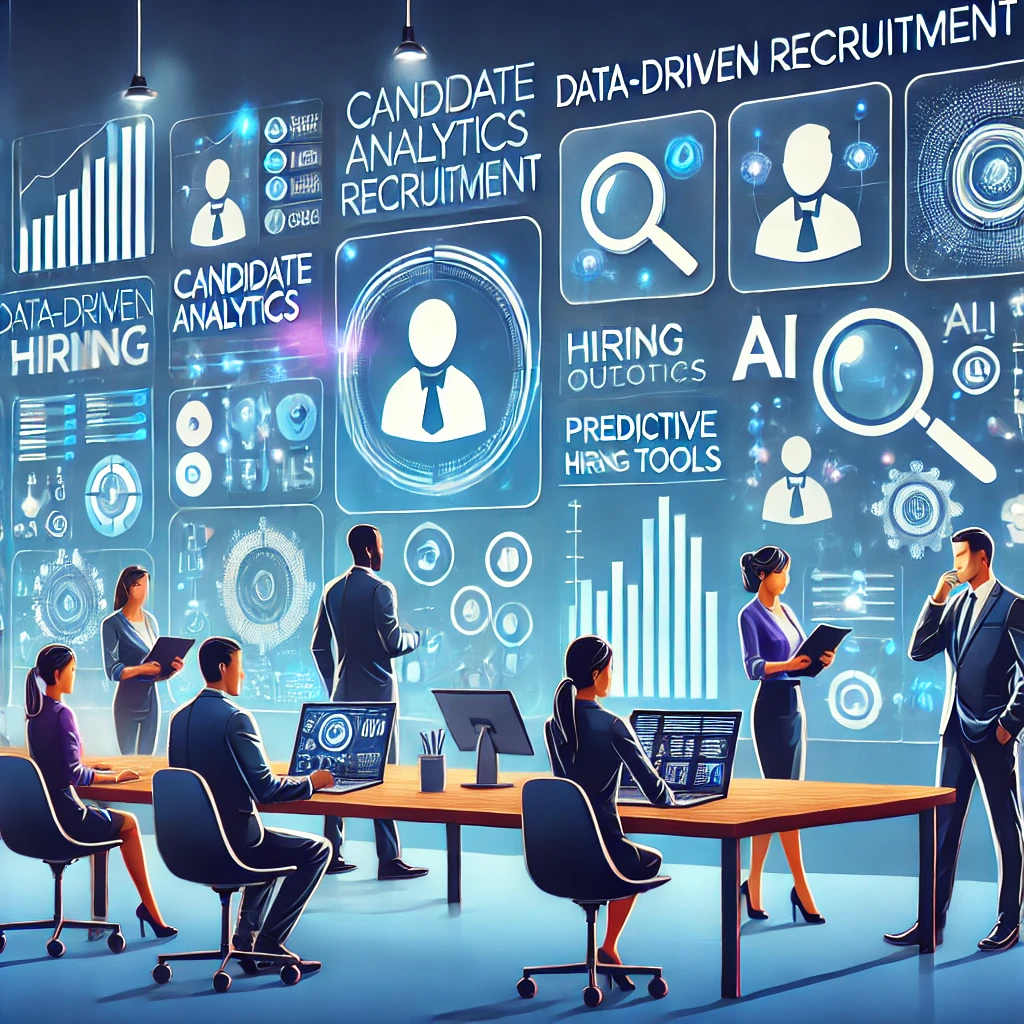
Data-driven recruitment is not just a buzzword; it is a revolution in how companies attract, evaluate, and hire candidates.
By integrating data and advanced analytics into recruitment strategies, organizations are better equipped to overcome common hiring challenges such as bias, inefficiency, and poor job-to-candidate matching.
It empowers employers to make decisions that are backed by facts, reducing guesswork and significantly improving outcomes for both recruiters and candidates.
As technology continues to advance, data-driven recruitment has become a critical part of a company’s overall talent acquisition strategy.
Tools such as applicant tracking systems (ATS), artificial intelligence (AI), and predictive analytics are now at the forefront of modern hiring practices.
These tools help organizations to sift through large volumes of applications, predict candidate success, and enhance the overall candidate experience.
In essence, this methodology is transforming recruitment into a science—where every decision is measured, analyzed, and optimized.
But how does this innovative approach work, and why is it crucial for businesses to adopt it? This blog dives deep into the concept of data-driven recruitment, exploring its definition, working process, and the technologies that make it possible.
From discussing the benefits it brings to both employers and candidates to addressing the challenges of implementation, this comprehensive guide will help you understand why data is the future of hiring.
Whether you’re an HR professional, a business leader, or someone looking to gain insights into modern recruitment trends, this blog will equip you with the knowledge to embrace data-driven strategies effectively. Let’s uncover how data-driven recruitment works and why it’s becoming the cornerstone of successful hiring in the digital age.
Before we venture further into this article, we would like to share who we are and what we do.
About 9cv9
9cv9 is a business tech startup based in Singapore and Asia, with a strong presence all over the world.
With over eight years of startup and business experience, and being highly involved in connecting with thousands of companies and startups, the 9cv9 team has listed some important learning points in this overview of What is Data-Driven Recruitment and How It Works.
If your company needs recruitment and headhunting services to hire top-quality employees, you can use 9cv9 headhunting and recruitment services to hire top talents and candidates. Find out more here, or send over an email to hello@9cv9.com.
Or just post 1 free job posting here at 9cv9 Hiring Portal in under 10 minutes.
What is Data-Driven Recruitment and How It Works
- What is Data-Driven Recruitment?
- How Does Data-Driven Recruitment Work?
- Benefits of Data-Driven Recruitment
- Challenges in Implementing Data-Driven Recruitment
- Best Practices for Implementing Data-Driven Recruitment
- Future Trends in Data-Driven Recruitment
1. What is Data-Driven Recruitment?
Data-driven recruitment is a modern approach to hiring that relies on data, technology, and analytics to enhance decision-making throughout the recruitment process. By analyzing a variety of recruitment metrics, such as candidate profiles, application trends, and hiring performance, organizations can improve efficiency, minimize bias, and achieve better hiring outcomes. Below is an in-depth exploration of what data-driven recruitment entails, its core elements, and how it stands apart from traditional hiring methods.
Understanding Data-Driven Recruitment
- Definition:
- A hiring strategy that uses data, analytics, and technology to optimize recruitment decisions.
- Focuses on gathering actionable insights to ensure more precise hiring processes.
- Core Objective:
- To find the best candidates for a role by analyzing data points rather than relying solely on intuition or experience.
Key Features of Data-Driven Recruitment
- Data Collection:
- Involves collecting large volumes of data from multiple sources, including:
- Applicant tracking systems (ATS).
- Employee performance reviews.
- Social media platforms (LinkedIn, Twitter).
- Example: A company uses ATS to track the number of applicants, time-to-hire, and job offer acceptance rates to identify bottlenecks.
- Involves collecting large volumes of data from multiple sources, including:
- Analytics and Insights:
- Data is analyzed to extract patterns and insights.
- Common analytics tools include predictive analytics, resume screening software, and data visualization platforms.
- Example: Predictive analytics might indicate that candidates with experience in a particular software tool have a 30% higher chance of excelling in a specific role.
- Performance-Based Decisions:
- Hiring decisions are based on measurable data points rather than subjective judgments.
- Includes candidate scoring models that rank applicants based on skills, experience, and compatibility.
- Example: A recruitment platform assigns a “fit score” to applicants, helping employers prioritize candidates with higher compatibility.
Advantages Over Traditional Recruitment
- Traditional Recruitment:
- Often relies on resumes, interviews, and intuition.
- Subject to personal bias and inefficiencies in the screening process.
- Data-Driven Recruitment:
- Relies on objective data to improve hiring outcomes.
- Reduces time-to-hire, enhances candidate experience, and minimizes the risk of bad hires.
- Example: A company replaced manual resume reviews with AI-powered software and reduced their hiring time by 50%.
Core Components of Data-Driven Recruitment
1. Use of Technology
- Applicant Tracking Systems (ATS):
- Automates the sorting and filtering of candidate applications.
- Tracks metrics like application completion rates, time-to-fill, and source effectiveness.
- Example: Google for Jobs uses ATS integration to provide insights into job posting performance.
- Artificial Intelligence (AI):
- Automates tasks like resume screening and initial candidate engagement.
- AI chatbots conduct pre-screening interviews to filter unqualified candidates.
- Example: Unilever uses AI for its recruitment process, which resulted in a 70% time savings for recruiters.
- Data Visualization Tools:
- Helps recruiters interpret complex hiring data through charts, graphs, and dashboards.
- Example: Tableau and Power BI are commonly used to visualize recruitment KPIs.
2. Metrics and KPIs (Key Performance Indicators)
- Quality of Hire:
- Measures the performance and retention of new hires.
- Example: A company tracks the six-month performance reviews of hires to assess hiring success.
- Source Effectiveness:
- Tracks which platforms (e.g., job boards, LinkedIn) bring in the most successful candidates.
- Example: LinkedIn might yield higher-quality candidates for technical roles, while Indeed works better for customer service jobs.
- Candidate Experience:
- Surveys and feedback tools are used to measure how candidates perceive the recruitment process.
- Example: A company uses Glassdoor reviews to improve candidate satisfaction.
Examples of Data-Driven Recruitment in Action
1. Netflix’s Data-Driven Hiring
- Netflix uses data to screen candidates and predict cultural fit.
- Analyzes candidate skills, past performance, and alignment with company values using proprietary tools.
- Result: Netflix hires high-performing employees with a strong cultural match.
2. Amazon’s AI-Powered Recruitment
- Amazon implemented AI to analyze job applications at scale.
- AI systems predict a candidate’s likelihood of succeeding based on historical hiring data.
- Result: Reduced time-to-hire and increased efficiency in candidate selection.
3. Leveraging Social Media Insights
- Companies analyze engagement on platforms like LinkedIn to identify active job seekers.
- Example: A recruitment agency tracks candidate engagement with job postings to gauge interest levels and suitability.
Why Data-Driven Recruitment is Essential in Modern Hiring
- Addresses Bias:
- Reduces unconscious bias by relying on objective data rather than subjective opinions.
- Improves Efficiency:
- Automates repetitive tasks, allowing recruiters to focus on strategic decision-making.
- Enhances Retention:
- Data insights help identify candidates who are more likely to succeed and stay longer in the role.
- Example: An analytics platform flagged candidates with a history of frequent job changes, leading to more stable hires.
Data-driven recruitment is transforming the way organizations hire talent. By integrating advanced analytics, leveraging technology, and focusing on measurable outcomes, companies can ensure better hiring success and enhance their overall talent acquisition strategies.
2. How Does Data-Driven Recruitment Work?
Data-driven recruitment combines technology, analytics, and actionable insights to create a structured and optimized hiring process. By leveraging data at every stage of recruitment—from job posting to candidate evaluation and onboarding—employers can make smarter, faster, and more accurate decisions. Below, we explore the key steps and methodologies of data-driven recruitment in detail, including how each stage works, the tools involved, and real-world examples.
Step 1: Gathering Data
1. Collecting Candidate Information
- Sources of Data:
- Resumes and cover letters.
- Online profiles (LinkedIn, GitHub, portfolios).
- Application forms on job boards and company websites.
- Example: A recruiter uses a LinkedIn scraper to collect information on candidates’ skills, experience, and certifications.
- Behavioral Data:
- Tracks how candidates interact with job postings, such as:
- Time spent on the careers page.
- Click-through rates (CTR) on job ads.
- Example: A company identifies a high CTR on remote job postings, leading to adjustments in its hiring strategy.
- Tracks how candidates interact with job postings, such as:
2. Internal Organizational Data
- Historical Data:
- Employee performance metrics and turnover rates.
- Past recruitment data, including time-to-hire and cost-per-hire.
- Example: A company uses data from past hires to identify common traits in successful employees.
- Feedback Data:
- Collects insights from employees and candidates about the recruitment process.
- Example: Post-interview surveys reveal whether the process was efficient and fair.
Step 2: Analyzing Data
1. Use of Recruitment Analytics Tools
- Predictive Analytics:
- Analyzes historical data to predict future hiring trends and candidate success.
- Example: A predictive tool suggests that candidates with certifications in cloud computing have a 40% higher success rate in tech roles.
- Sentiment Analysis:
- Evaluates candidate responses to assess motivation, confidence, and cultural alignment.
- Example: AI-powered tools analyze interview transcripts for positive and negative sentiment indicators.
- Skill Gap Analysis:
- Identifies skills missing in current employees and uses this information to tailor job postings.
- Example: A company finds a shortage of data visualization expertise and prioritizes hiring candidates with Tableau experience.
2. Candidate Scoring Systems
- Weighted Scoring Models:
- Assign scores to candidates based on criteria such as:
- Educational qualifications.
- Relevant skills and certifications.
- Cultural fit assessments.
- Example: A candidate scoring 85/100 based on these parameters gets prioritized for interviews.
- Assign scores to candidates based on criteria such as:
- ATS Integration:
- Uses algorithms to rank candidates automatically.
- Example: Google’s ATS highlights top applicants by matching resumes against job descriptions.
Step 3: Optimizing Job Advertisements and Sourcing
1. Data-Driven Job Descriptions
- Keyword Optimization:
- Analyzes job description performance metrics such as views and applications.
- Adjusts keywords and content to attract better candidates.
- Example: A company adds “remote work” to job titles after noticing high demand for flexible roles.
- A/B Testing Job Posts:
- Tests multiple versions of job ads to find the most effective ones.
- Example: Job postings emphasizing salary transparency outperform generic ads by 25%.
2. Sourcing Strategy
- Tracking Source Effectiveness:
- Identifies the most successful recruitment channels, such as LinkedIn, Indeed, or niche platforms.
- Example: Data reveals that referrals produce the highest-quality hires, leading to an expansion of the referral program.
Step 4: Candidate Screening and Shortlisting
1. AI-Powered Resume Screening
- Automated Resume Parsing:
- Extracts and analyzes key information from resumes to identify top candidates.
- Example: AI software filters applicants based on keywords like “Python,” “Machine Learning,” and “Data Analysis.”
- Bias Reduction:
- Removes identifiers like names, gender, and ethnicity to ensure fair evaluation.
- Example: Blind screening helps a company increase diversity in its engineering team.
2. Pre-Employment Assessments
- Skill-Based Tests:
- Measures candidate expertise through practical assignments or online tests.
- Example: A marketing company uses a content creation test to evaluate applicants’ writing skills.
- Psychometric Evaluations:
- Gauges personality traits and cultural fit through data-driven personality assessments.
- Example: Candidates for leadership roles undergo personality assessments to test decision-making skills.
Step 5: Decision-Making and Selection
1. Data-Driven Interview Processes
- Structured Interviews:
- Standardized interview questions reduce bias and improve reliability.
- Example: Data shows that structured interviews lead to better predictions of job performance.
- Interview Scoring:
- Assigns numerical ratings to interview responses.
- Example: A recruiter uses scoring rubrics for consistency across interviewers.
2. Final Selection Based on Data
- Candidate Fit Scores:
- Combines data from all stages—resume screening, tests, and interviews—to select the best candidate.
- Example: A candidate with a high score across assessments gets prioritized for an offer.
- Offer Prediction Tools:
- Predicts the likelihood of a candidate accepting an offer based on previous data.
- Example: Data suggests offering flexible work hours to increase acceptance rates by 15%.
Step 6: Post-Hire Analytics and Feedback
1. Onboarding Metrics
- Tracks new hire performance during the onboarding phase.
- Example: A company measures time-to-productivity to refine its onboarding program.
2. Long-Term Performance Tracking
- Evaluates how well hires perform over time to improve future recruitment strategies.
- Example: Employees hired through data-driven methods have a 20% higher retention rate than those hired traditionally.
Examples of Data-Driven Recruitment in Action
1. IBM
- Uses AI-powered recruitment tools to analyze candidate skills and predict job fit.
- Results: Reduced time-to-hire by 30% and increased diversity.
2. Spotify
- Implements structured interview scoring and predictive analytics to identify top talent.
- Results: Improved hiring success rate for creative roles.
By systematically collecting, analyzing, and applying data, data-driven recruitment transforms hiring into a measurable, efficient, and outcome-driven process. This approach ensures organizations not only find the best talent but also build sustainable recruitment practices for long-term success.
3. Benefits of Data-Driven Recruitment
Data-driven recruitment offers numerous advantages that revolutionize the traditional hiring process, making it more efficient, fair, and strategic. By leveraging data, organizations can streamline their recruitment efforts, reduce costs, and ensure they attract the most suitable talent. Below, we break down the key benefits of data-driven recruitment with relevant examples to illustrate its impact.
1. Enhanced Decision-Making
1.1. Improved Candidate Evaluation
- Data-driven tools help recruiters assess candidates based on objective metrics rather than subjective opinions.
- Examples:
- AI-powered Applicant Tracking Systems (ATS) rank candidates by matching resumes to job descriptions.
- Predictive analytics determine which candidates are likely to excel based on historical performance data.
1.2. Reduction of Human Bias
- Blind screening tools eliminate identifiers such as names, gender, or ethnicity, ensuring fair evaluations.
- Examples:
- Companies using blind screening see a 20% increase in diverse hires.
- Software like Textio detects and removes biased language in job descriptions.
1.3. Strategic Decision-Making
- Recruitment decisions are supported by data, such as talent availability and industry benchmarks, to craft effective hiring strategies.
- Example: A tech company uses regional talent data to identify cities with a higher concentration of software developers, focusing recruitment efforts accordingly.
2. Increased Efficiency in Recruitment Processes
2.1. Faster Hiring Cycles
- Data-driven recruitment significantly reduces the time-to-hire by automating repetitive tasks like resume screening and interview scheduling.
- Examples:
- Automation tools filter through thousands of resumes in minutes.
- A company reduced its average hiring time from 30 days to 15 days by using AI screening tools.
2.2. Cost Reduction
- Optimized recruitment processes help lower costs by minimizing wasted effort and resources.
- Examples:
- Using data to identify high-performing recruitment channels can cut job advertising costs by 25%.
- Automated sourcing tools reduce reliance on costly external recruitment agencies.
2.3. Targeted Candidate Sourcing
- Analyzing sourcing channel effectiveness allows recruiters to focus on platforms delivering the best results.
- Examples:
- Data reveals LinkedIn delivers the highest-quality candidates for executive roles, while GitHub is more effective for developers.
- A recruitment agency uses A/B testing on job ads to increase application rates by 40%.
3. Improved Candidate Experience
3.1. Personalized Engagement
- Data enables tailored communication based on candidates’ preferences and behavior.
- Examples:
- Sending personalized emails based on candidate profiles results in higher response rates.
- Chatbots provide 24/7 support, answering FAQs about the hiring process.
3.2. Streamlined Application Process
- Data-driven systems simplify applications by auto-filling forms and minimizing repetitive steps.
- Examples:
- Job platforms use autofill technology, reducing application time by 50%.
- Companies track drop-off rates to identify and eliminate cumbersome steps in the application process.
3.3. Transparent and Timely Feedback
- Recruiters use data to provide candidates with real-time updates about their application status.
- Examples:
- Automated systems notify candidates of interview results faster, increasing satisfaction.
- Post-interview surveys show that transparency improves candidate experience by 30%.
4. Higher Quality of Hires
4.1. Better Cultural Fit
- Data-driven personality assessments ensure hires align with company culture.
- Examples:
- A marketing agency uses psychometric tests to measure collaboration and creativity, leading to a more cohesive team.
- Data reveals that candidates who excel in teamwork assessments have a 25% lower turnover rate.
4.2. Skill Matching and Predictive Success
- Tools analyze skillsets to match candidates to roles where they are most likely to succeed.
- Examples:
- Predictive analytics reveal candidates with certifications in cybersecurity have a 50% success rate in IT roles.
- Machine learning algorithms suggest specific training for new hires to fill skill gaps, increasing productivity.
5. Greater Workforce Diversity
5.1. Objective Recruitment Practices
- Data-driven methods eliminate bias in candidate sourcing and evaluation.
- Examples:
- Companies implementing data-driven hiring practices see a 15% increase in underrepresented groups in leadership roles.
- Tools like Entelo Diversity use AI to identify diverse candidates while ensuring qualifications are met.
5.2. Tracking Diversity Metrics
- Organizations can measure diversity in recruitment and adjust strategies accordingly.
- Examples:
- Analyzing gender and ethnicity ratios among applicants helps companies meet diversity goals.
- Tracking internal promotions ensures equal opportunities for all employees.
6. Data-Driven Recruitment Metrics
6.1. Monitoring Key Performance Indicators (KPIs)
- Data allows companies to measure and track recruitment metrics like:
- Time-to-hire.
- Cost-per-hire.
- Quality-of-hire.
- Examples:
- A company reduces time-to-hire by 20% after identifying bottlenecks in the interview process.
- Recruitment campaigns using cost-per-hire metrics adjust budgets effectively.
6.2. Continuous Improvement
- Data insights enable ongoing refinement of recruitment strategies.
- Examples:
- Post-hire data shows candidates from specific channels perform better, leading to increased investment in those platforms.
- Regularly analyzing feedback ensures the recruitment process remains efficient and candidate-friendly.
Real-World Example: How Amazon Benefits from Data-Driven Recruitment
Amazon employs data-driven strategies to optimize its hiring process:
- Uses machine learning models to screen resumes and predict job fit.
- Implements recruitment dashboards to monitor hiring performance in real-time.
- Tracks long-term employee performance, using data insights to refine hiring criteria.
- Result: Amazon reduces time-to-hire while maintaining a high standard for quality-of-hire.
Conclusion
By focusing on objective metrics, automation, and continuous improvement, data-driven recruitment delivers a wealth of benefits. It enhances decision-making, improves efficiency, elevates candidate experience, and ensures better alignment with organizational goals. Companies adopting this approach are better equipped to build high-performing, diverse, and resilient teams in today’s competitive job market.
4. Challenges in Implementing Data-Driven Recruitment
While data-driven recruitment offers significant advantages, implementing it comes with several challenges. Organizations must address these obstacles to fully leverage the potential of data in their hiring processes. This section explores the most common challenges, supported by relevant examples, and provides a structured breakdown for better understanding.
1. Data Accessibility and Quality
1.1. Insufficient Data Availability
- Many organizations lack the volume of historical recruitment data needed for meaningful analysis.
- Examples:
- A startup with limited hiring history may struggle to build predictive models for candidate success.
- Smaller businesses often face challenges in collecting comprehensive candidate data.
1.2. Poor Data Quality
- Inaccurate, incomplete, or outdated data can lead to flawed insights and decisions.
- Examples:
- An organization using old candidate databases may overlook qualified individuals who have updated skills.
- Incorrect job title mappings in ATS systems can result in misaligned candidate recommendations.
1.3. Fragmented Data Sources
- Recruitment data may reside in multiple platforms, making integration and analysis challenging.
- Examples:
- Candidate profiles scattered across job boards, LinkedIn, and in-house databases hinder comprehensive evaluation.
- Siloed data systems prevent recruiters from having a unified view of the talent pipeline.
2. High Implementation Costs
2.1. Expensive Technology Investments
- Implementing advanced tools such as AI-powered ATS or predictive analytics software can be cost-prohibitive for many organizations.
- Examples:
- A mid-sized firm delays adopting recruitment AI due to its high upfront licensing costs.
- Cloud-based platforms with subscription fees pose budgetary challenges for small businesses.
2.2. Cost of Training and Expertise
- Recruiters need specialized training to effectively use data-driven tools, which can be time-consuming and costly.
- Examples:
- An organization spends months training staff on new recruitment analytics software.
- Companies hiring data analysts specifically for recruitment processes face additional payroll expenses.
3. Resistance to Change
3.1. Organizational Pushback
- Employees accustomed to traditional hiring methods may resist adopting data-driven approaches.
- Examples:
- Hiring managers skeptical about AI-based decisions may override algorithmic recommendations.
- Recruiters preferring gut instincts over data struggle to adapt to automated systems.
3.2. Lack of Stakeholder Buy-In
- Leadership and stakeholders may hesitate to invest in data-driven recruitment without clear ROI demonstrations.
- Examples:
- An HR team fails to convince executives about the long-term benefits of data analytics in hiring.
- Budget approval delays due to doubts about the effectiveness of predictive hiring tools.
4. Ethical and Legal Concerns
4.1. Data Privacy Issues
- Collecting and analyzing candidate data can raise privacy concerns, particularly in regions with strict regulations.
- Examples:
- General Data Protection Regulation (GDPR) in Europe requires companies to secure explicit consent before storing candidate data.
- Organizations may face penalties for non-compliance with local data protection laws.
4.2. Risk of Discrimination
- Poorly designed algorithms may inadvertently perpetuate biases, leading to unfair hiring practices.
- Examples:
- AI trained on biased historical hiring data may prioritize male candidates over equally qualified female candidates.
- A tech company discovers its recruitment algorithm unfairly disadvantages applicants from certain socioeconomic backgrounds.
4.3. Ethical Transparency
- Candidates and stakeholders demand clarity on how data is used in recruitment decisions.
- Examples:
- Lack of transparency about AI processes causes mistrust among job applicants.
- Companies fail to explain how predictive analytics influence hiring outcomes, leading to concerns about fairness.
5. Technical Challenges
5.1. Integration Issues
- Integrating data-driven recruitment tools with existing HR systems can be complex.
- Examples:
- An ATS may not seamlessly integrate with an organization’s performance tracking software, leading to data gaps.
- Data migration during system upgrades can result in the loss of valuable candidate information.
5.2. Maintenance and Upgrades
- Keeping recruitment systems updated and functional requires continuous investment and expertise.
- Examples:
- Frequent software updates disrupt workflows, causing temporary inefficiencies.
- An outdated recruitment platform fails to support new AI features, limiting its capabilities.
5.3. Data Security Concerns
- Protecting candidate data from breaches is critical but challenging.
- Examples:
- A major data breach exposes sensitive candidate information, damaging the organization’s reputation.
- Recruitment platforms with weak encryption become targets for cyberattacks.
6. Over-Reliance on Technology
6.1. Ignoring Human Judgment
- Over-reliance on data may lead recruiters to undervalue human intuition and experience.
- Examples:
- Automated systems might overlook soft skills that are crucial for specific roles.
- A candidate with unconventional experience is filtered out by rigid algorithms, despite their potential fit for the role.
6.2. Automation Errors
- Errors in algorithms can result in false negatives or false positives in candidate screening.
- Examples:
- A flawed scoring model mistakenly ranks unsuitable candidates higher than qualified ones.
- Resume parsing errors lead to the exclusion of candidates with non-standard formatting.
7. Scalability and Adaptability Issues
7.1. Challenges in Scaling Processes
- Systems designed for smaller organizations may struggle to handle large volumes of data as the company grows.
- Examples:
- A fast-growing business experiences delays in recruitment due to an overloaded ATS.
- Existing tools fail to meet the demands of global recruitment campaigns.
7.2. Adapting to Industry Trends
- Data-driven recruitment requires continuous adaptation to evolving technologies and workforce trends.
- Examples:
- Emerging technologies like natural language processing (NLP) necessitate constant system upgrades.
- Companies unable to track shifting candidate preferences risk falling behind competitors.
Real-World Example: Challenges Faced by a Leading E-Commerce Firm
A prominent e-commerce company aimed to implement data-driven recruitment across its global operations but encountered significant hurdles:
- Data fragmentation across regional offices delayed the unification process.
- Leadership was initially hesitant to invest in expensive analytics tools without guaranteed returns.
- Legal teams flagged compliance risks related to data privacy in different markets.
- Despite these challenges, the company overcame them by hiring specialized data analysts and partnering with a compliance consultancy.
Conclusion
Implementing data-driven recruitment requires organizations to navigate a range of challenges, from technical barriers and costs to ethical concerns and organizational resistance. Addressing these issues proactively ensures that businesses can unlock the full potential of data in hiring, resulting in a more efficient, fair, and impactful recruitment process.
5. Best Practices for Implementing Data-Driven Recruitment
Implementing data-driven recruitment successfully requires strategic planning, technological investments, and organizational alignment. By adopting best practices, companies can maximize the benefits of this approach while minimizing potential challenges. Below is a detailed exploration of the best practices, supported by examples and actionable insights.
1. Establish Clear Goals and KPIs
1.1. Define Recruitment Objectives
- Set specific, measurable goals for using data in recruitment processes.
- Examples:
- Aim to reduce the time-to-hire by 20% using automated candidate screening.
- Increase the diversity of hires by 15% within a year through bias detection in recruitment algorithms.
1.2. Identify Key Performance Indicators (KPIs)
- Use relevant metrics to measure success and track progress.
- Examples of KPIs:
- Time-to-hire: Evaluate how long it takes to fill a position.
- Quality of hire: Assess the long-term performance of new hires.
- Candidate satisfaction: Gather feedback from applicants about their recruitment experience.
1.3. Align Goals with Business Strategy
- Ensure recruitment objectives support broader organizational goals.
- Example:
- A tech company focusing on innovation prioritizes hiring candidates with cutting-edge skills in AI and machine learning.
2. Invest in the Right Tools and Technologies
2.1. Choose an Advanced Applicant Tracking System (ATS)
- Use ATS platforms that offer robust data analytics capabilities.
- Examples:
- Lever or Greenhouse: These ATS solutions provide real-time data insights on candidate pipelines.
- SmartRecruiters: A tool known for its predictive analytics features.
2.2. Leverage AI-Powered Tools
- Incorporate AI tools to automate and enhance decision-making processes.
- Examples:
- Pymetrics: Uses neuroscience-based games and AI to match candidates to roles.
- HireVue: Provides video interview analysis using AI to evaluate non-verbal cues and communication skills.
2.3. Integrate Data Across Platforms
- Consolidate data from various sources (job boards, ATS, CRM) for a unified view.
- Example:
- Integrating LinkedIn Recruiter data with in-house ATS to create a centralized talent database.
3. Ensure Data Accuracy and Quality
3.1. Maintain Clean and Updated Data
- Regularly audit databases to remove duplicates, outdated profiles, and incomplete records.
- Examples:
- Quarterly data clean-ups in the ATS to ensure accuracy.
- Use data validation tools to verify candidate details during onboarding.
3.2. Standardize Data Collection
- Establish consistent methods for collecting and storing candidate data.
- Example:
- Implement structured application forms with mandatory fields for education, skills, and experience.
3.3. Monitor Data Quality Regularly
- Conduct periodic checks to identify errors or inconsistencies in the data.
- Example:
- A recruitment team uses dashboards to monitor real-time data quality metrics like completeness and accuracy.
4. Train Teams and Foster a Data-Driven Culture
4.1. Provide Training for Recruiters
- Ensure HR teams understand how to use data-driven tools effectively.
- Examples:
- Workshops on interpreting recruitment analytics dashboards.
- Hands-on training for using AI-based tools like predictive analytics software.
4.2. Encourage a Data-First Mindset
- Promote the value of data-driven decision-making across the organization.
- Example:
- Conduct regular meetings to showcase successful hiring outcomes achieved through data-driven methods.
4.3. Collaborate Across Departments
- Work closely with IT, legal, and compliance teams to ensure seamless implementation.
- Example:
- HR teams partner with IT to address data integration challenges during ATS implementation.
5. Prioritize Ethical and Legal Compliance
5.1. Adhere to Data Privacy Regulations
- Comply with laws such as GDPR, CCPA, or local data protection acts.
- Examples:
- Include privacy policies on career pages explaining how candidate data will be used.
- Obtain explicit consent before collecting personal data during the application process.
5.2. Mitigate Algorithmic Bias
- Regularly audit AI tools to identify and eliminate biases.
- Examples:
- Adjust recruitment algorithms to avoid favoring specific demographics.
- Use diverse datasets to train AI tools and reduce bias in candidate selection.
5.3. Maintain Transparency with Candidates
- Be open about how data influences recruitment decisions.
- Example:
- Share insights from candidate assessments with applicants to build trust and encourage feedback.
6. Continuously Monitor and Optimize
6.1. Analyze Recruitment Performance Regularly
- Use dashboards and reports to identify trends and areas for improvement.
- Example:
- Monthly reviews of candidate drop-off rates in the application process to pinpoint pain points.
6.2. Conduct A/B Testing
- Experiment with different approaches to identify the most effective strategies.
- Example:
- Test two different job ad formats to see which generates more qualified applications.
6.3. Incorporate Feedback Loops
- Gather insights from candidates and hiring managers to refine processes.
- Example:
- Use post-interview surveys to understand candidate experiences and improve the recruitment process.
7. Use Data for Proactive Workforce Planning
7.1. Forecast Future Hiring Needs
- Use historical data to predict workforce requirements.
- Example:
- An e-commerce company analyzes seasonal hiring trends to prepare for peak periods like Black Friday.
7.2. Build Talent Pipelines
- Identify and engage with potential candidates before positions open.
- Example:
- A software company creates a talent pool of top developers identified through data analytics.
7.3. Leverage Market Insights
- Use external data to understand industry trends and adjust recruitment strategies.
- Example:
- Recruitment teams analyze job market reports to benchmark salaries and attract top talent.
Real-World Example: Success in Data-Driven Recruitment
A global retail giant implemented a data-driven recruitment strategy to improve hiring efficiency and candidate quality:
- Steps Taken:
- Invested in a predictive analytics platform to identify high-potential candidates.
- Conducted recruiter training on data interpretation and AI tool usage.
- Implemented A/B testing on job postings to improve click-through rates.
- Results:
- Reduced time-to-hire by 30%.
- Improved quality-of-hire scores by 25% based on new hire performance evaluations.
Conclusion
By following these best practices, organizations can navigate the complexities of data-driven recruitment and create a more efficient, fair, and strategic hiring process. From investing in the right tools to fostering a data-centric culture, these strategies ensure that companies fully leverage the power of data in recruitment.
6. Future Trends in Data-Driven Recruitment
As technology and data analytics continue to evolve, the field of data-driven recruitment is poised to undergo significant advancements. These trends promise to make recruitment processes faster, more efficient, and highly personalized, enabling organizations to secure top talent while staying competitive. Below are the most notable future trends in data-driven recruitment, broken into sub-sections for clarity.
1. Enhanced Use of Artificial Intelligence (AI) in Recruitment
1.1. AI-Powered Candidate Sourcing
- AI tools are becoming increasingly adept at analyzing vast data sets to identify the best candidates.
- Examples:
- AI platforms like SeekOut and HireEZ enable recruiters to source passive candidates by scanning social media profiles, resumes, and job boards.
- Machine learning models suggest candidates based on similar profiles that performed well in the past.
1.2. Intelligent Chatbots for Screening
- AI chatbots will handle initial interactions with candidates, asking questions and providing real-time feedback.
- Benefits:
- Saves recruiters’ time by pre-screening candidates.
- Ensures round-the-clock availability for queries.
- Example:
- Olivia by Paradox helps automate early-stage recruitment tasks like answering FAQs and scheduling interviews.
1.3. Predictive Analytics for Talent Matching
- AI-driven predictive analytics will assess candidates’ likelihood of success based on historical data.
- Example:
- Using predictive algorithms, a tech company identifies candidates who are more likely to excel in remote work settings based on previous hires’ performance.
2. Integration of Advanced Analytics for Deeper Insights
2.1. Sentiment Analysis in Candidate Evaluation
- Sentiment analysis tools analyze candidates’ tone and communication during interviews or in written applications.
- Benefits:
- Helps assess cultural fit and soft skills.
- Example:
- HR analytics tools evaluate email correspondence or interview transcripts for indicators of motivation and team alignment.
2.2. Real-Time Analytics Dashboards
- Recruiters will rely on dynamic dashboards to monitor recruitment metrics in real time.
- Metrics tracked include:
- Application drop-off rates.
- Candidate engagement on job portals.
- Effectiveness of sourcing channels.
2.3. Talent Market Mapping
- Analytics tools will provide insights into industry trends, salary benchmarks, and skill demand.
- Example:
- A company uses data-driven insights to identify emerging skill gaps in the IT sector and proactively hires cloud computing specialists.
3. Greater Adoption of Virtual and Augmented Reality in Recruitment
3.1. Virtual Reality (VR) for Immersive Assessments
- VR will create simulated environments where candidates can showcase skills.
- Example:
- A construction firm uses VR simulations to assess candidates’ ability to navigate safety challenges on a virtual construction site.
3.2. Augmented Reality (AR) for Employer Branding
- AR tools will enhance job postings by offering interactive experiences to candidates.
- Example:
- An automotive company uses AR to allow candidates to explore a 3D model of its latest electric car, highlighting the innovation and culture of the organization.
4. Personalized Recruitment with Big Data
4.1. Hyper-Personalized Candidate Experiences
- Big data will allow recruiters to create tailored experiences based on candidate preferences and profiles.
- Examples:
- Personalizing job recommendations based on past applications and browsing history.
- Sending customized interview prep materials aligned with the candidate’s skills and interests.
4.2. Data-Driven Diversity and Inclusion
- Big data analytics will identify biases and recommend strategies to promote inclusivity.
- Example:
- A company uses diversity analytics to assess hiring trends, ensuring equal opportunities for underrepresented groups.
5. Automation of Recruitment Processes
5.1. Fully Automated Candidate Engagement
- Automation tools will send reminders, schedule interviews, and provide updates automatically.
- Example:
- An automated system follows up with candidates to reduce drop-offs in later recruitment stages.
5.2. Programmatic Advertising for Job Openings
- Recruitment marketing platforms will use automation to place job ads in the right locations based on candidate data.
- Example:
- Using programmatic job advertising, a retail chain targets job ads to candidates in specific geographies with relevant skills.
6. Focus on Skills-Based Hiring
6.1. Transition from Resumes to Skills Portfolios
- Data-driven recruitment tools will assess skills through tests and portfolios rather than traditional resumes.
- Example:
- Platforms like CodeSignal evaluate programming skills via live coding challenges, bypassing the need for detailed resumes.
6.2. Rise of Micro-Credentials
- Candidates will showcase micro-credentials (short, specialized certifications) verified by recruitment systems.
- Example:
- A marketing candidate highlights their Google Ads certification, which is automatically validated by the company’s ATS.
7. Increased Use of Blockchain for Recruitment Transparency
7.1. Verifiable Digital Resumes
- Blockchain technology will ensure that resumes and certifications are tamper-proof.
- Example:
- A blockchain-powered platform allows employers to instantly verify academic credentials and professional certifications.
7.2. Secure Data Sharing
- Blockchain ensures secure sharing of candidate data across recruitment platforms.
- Benefit:
- Enhances data privacy while enabling seamless collaboration between recruiters and candidates.
8. Emphasis on Sustainability and Ethical Recruitment
8.1. Data-Driven Sustainability Metrics
- Companies will use data to evaluate the environmental and social impact of their recruitment efforts.
- Example:
- Measuring the carbon footprint of in-person interviews and shifting to virtual interviews to reduce emissions.
8.2. Ethical Use of Recruitment Data
- Recruiters will adopt stringent data privacy measures to build trust with candidates.
- Tip:
- Always disclose how candidate data will be used, ensuring compliance with global regulations like GDPR.
9. Cross-Industry Collaboration and Crowdsourcing Talent
9.1. Talent Sharing Ecosystems
- Companies in different industries will collaborate by sharing talent pools.
- Example:
- A hospitality chain partners with a retail company to hire candidates during seasonal surges.
9.2. Open Talent Marketplaces
- Crowdsourcing platforms will allow freelancers and part-time workers to connect with organizations more efficiently.
- Example:
- Platforms like Upwork and Toptal leverage AI to match highly skilled freelancers with specific project needs.
Conclusion
The future of data-driven recruitment is both exciting and transformative, offering unprecedented opportunities for innovation and efficiency. By staying ahead of these trends, organizations can enhance their recruitment strategies, attract top talent, and build a workforce aligned with their goals. These developments promise not only to make recruitment smarter but also more inclusive, transparent, and sustainable.
Conclusion
Data-driven recruitment is no longer just an optional innovation for modern hiring—it is a strategic necessity in today’s competitive job market. By leveraging the vast amounts of data available, organizations can significantly enhance the efficiency, accuracy, and fairness of their recruitment processes. From sourcing candidates and streamlining interviews to predicting future hiring needs, data-driven recruitment enables companies to build high-performing teams while minimizing biases and inefficiencies.
By understanding what data-driven recruitment is and how it works, businesses can take proactive steps toward creating a recruitment strategy that is both results-oriented and aligned with organizational goals. Integrating tools such as applicant tracking systems (ATS), artificial intelligence (AI), and analytics dashboards transforms hiring into a precise, objective, and insightful process, ensuring that every decision is backed by meaningful data.
Key Benefits Highlighted
- Enhanced efficiency: Automation and AI reduce time-to-hire by streamlining labor-intensive processes such as resume screening and interview scheduling.
- Better candidate matching: Predictive analytics ensure that organizations can identify candidates with the highest likelihood of success.
- Improved diversity and inclusion: Data analysis helps organizations uncover and address unconscious biases in hiring.
- Cost-effectiveness: Data-driven approaches allow companies to allocate resources more effectively, reducing overall recruitment costs.
Challenges and Opportunities
While data-driven recruitment offers immense potential, its implementation is not without challenges. Companies must address concerns such as:
- Ensuring data privacy and compliance with regulations like GDPR.
- Balancing human intuition with algorithmic recommendations.
- Managing the costs and complexities of adopting new technologies.
By prioritizing these considerations and adopting best practices, businesses can overcome these hurdles to reap the full benefits of data-driven recruitment. For instance, implementing robust data governance policies and fostering collaboration between recruiters and technology teams can ensure smooth integration of advanced tools.
The Future of Data-Driven Recruitment
The future of hiring lies in a fully integrated and intelligent ecosystem where data, automation, and human insights work together seamlessly. Emerging trends such as AI-driven sentiment analysis, blockchain for data security, and virtual reality assessments are set to redefine the recruitment landscape further. These innovations will continue to push the boundaries of how talent is identified, engaged, and retained.
Organizations that embrace these advancements early will not only secure top talent more effectively but also gain a competitive edge in their industries. Whether it’s leveraging big data for hyper-personalized experiences or using analytics to drive diversity initiatives, the potential for data-driven recruitment is immense and ever-evolving.
Final Thoughts
To succeed in the age of data-driven recruitment, businesses must combine a forward-thinking mindset with a willingness to adapt to new tools and technologies. By placing data at the heart of their hiring strategies, organizations can ensure that their recruitment processes are not just efficient but also equitable, scalable, and future-ready.
The question is no longer whether to adopt data-driven recruitment but how quickly businesses can embrace it to unlock its full potential. As more companies recognize the transformative impact of data on hiring, those that lead the way will set new benchmarks for excellence in talent acquisition.
If you find this article useful, why not share it with your hiring manager and C-level suite friends and also leave a nice comment below?
We, at the 9cv9 Research Team, strive to bring the latest and most meaningful data, guides, and statistics to your doorstep.
To get access to top-quality guides, click over to 9cv9 Blog.
People Also Ask
h business goals?
It ensures that hiring strategies are aligned with organizational objectives by providing insights into workforce needs and skills gaps.
What is the impact of data-driven recruitment on employer branding?
It strengthens employer branding by ensuring a seamless and efficient hiring process that reflects positively on the company.
How do recruitment teams get started with data-driven hiring?
Start by adopting basic tools like ATS, analyzing current recruitment data, and training teams on how to use analytics effectively.
Are there any risks in data-driven recruitment?
Potential risks include over-reliance on algorithms, inaccuracies in data interpretation, and the misuse of sensitive candidate information.
What is the cost of implementing data-driven recruitment tools?
Costs vary widely depending on the tools used, but many platforms offer scalable pricing options suitable for businesses of all sizes.
Can data-driven recruitment help with workforce planning?
Yes, by analyzing data trends, it helps organizations forecast workforce needs and plan for future hiring requirements.
How does data-driven recruitment support remote hiring?
It enables recruiters to assess candidates virtually, analyze data for remote compatibility, and streamline global talent acquisition.
What skills are needed to implement data-driven recruitment?
Skills include data analysis, familiarity with recruitment tools, understanding of compliance laws, and knowledge of hiring trends.
What is the future of data-driven recruitment?
The future includes greater use of AI, blockchain for data security, predictive analytics, and VR for candidate assessments.
Why is data-driven recruitment essential in today’s market?
It ensures organizations stay competitive by optimizing hiring processes, improving decision-making, and attracting top talent efficiently.