Key Takeaways
- AI analysts are in high demand across industries, with roles expanding due to the rapid adoption of artificial intelligence technologies.
- Strong data skills, machine learning expertise, and ethical awareness are essential for success in the evolving AI analyst career landscape.
- Continuous learning and specialization opportunities make this a future-proof, high-growth career path with competitive salaries and global prospects.
In today’s rapidly evolving digital economy, the role of the AI Analyst has emerged as one of the most critical and transformative careers of the 21st century. As organizations across industries pivot towards automation, big data, and intelligent decision-making, the demand for professionals who can interpret, manage, and derive insights from artificial intelligence systems is skyrocketing. This blog, “The AI Analyst: A Comprehensive Career Report,” offers an in-depth exploration of the AI Analyst profession, dissecting its current landscape, future outlook, required skills, education pathways, industry applications, salary benchmarks, and more.
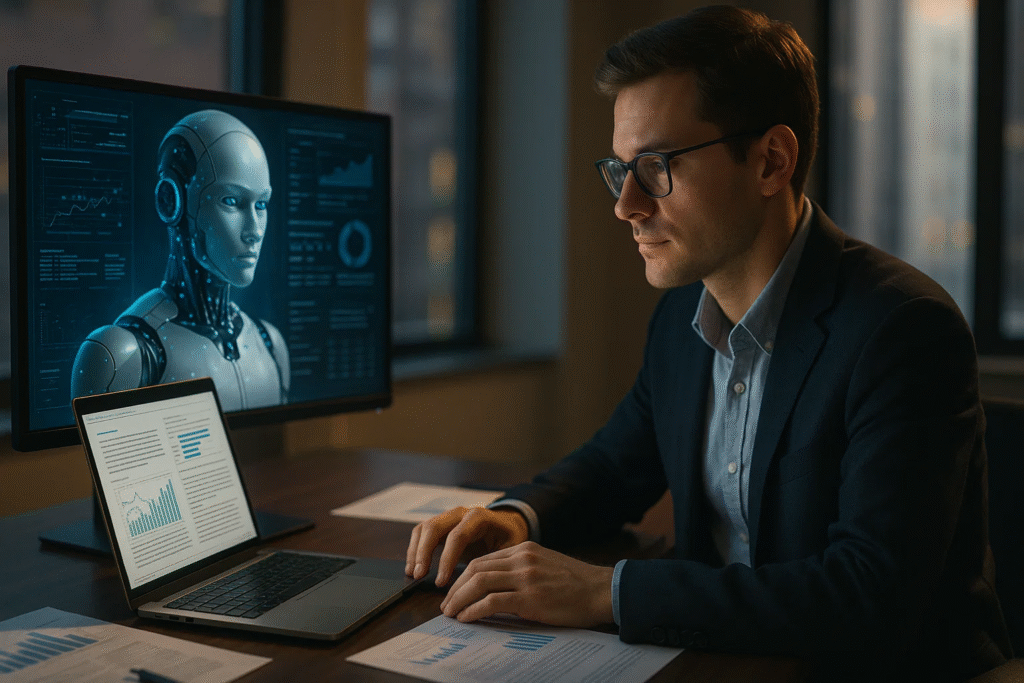
Artificial intelligence is no longer a futuristic concept—it is an operational reality that is redefining how businesses compete, innovate, and serve their customers. From finance and healthcare to manufacturing and e-commerce, AI technologies are being embedded into every aspect of enterprise strategy. However, the effectiveness of these technologies is only as good as the human intelligence that shapes and interprets them. This is where AI Analysts come into play. These professionals serve as the bridge between technical AI capabilities and actionable business insights, ensuring that data-driven strategies align with organizational goals.
With increasing volumes of structured and unstructured data being generated every second, companies need experts who can not only understand AI models and machine learning algorithms but also assess their performance, ethical implications, and business value. AI Analysts are expected to blend technical acumen with strategic thinking, providing crucial guidance on how AI initiatives can be designed, implemented, monitored, and improved. This multifaceted role requires a solid foundation in data science, statistical analysis, programming, and domain-specific knowledge—making it one of the most intellectually demanding yet rewarding careers in the digital era.
This report dives deep into the key responsibilities of AI Analysts, outlines the core and emerging competencies required to succeed, and highlights the educational and certification paths that can set aspiring professionals on the right trajectory. Additionally, we examine the sectors that are most aggressively hiring AI Analysts, from multinational tech firms to startups and government institutions. We also provide up-to-date salary data and career growth trends, offering a comprehensive view of what professionals can expect in terms of compensation and career development.
Furthermore, we explore how advancements in generative AI, natural language processing (NLP), and predictive analytics are reshaping the expectations and job descriptions of AI Analysts. As AI systems become more autonomous and sophisticated, the analyst’s role is shifting from traditional data interpretation to more nuanced functions such as bias detection, ethical governance, and human-AI collaboration. These changes underscore the importance of lifelong learning and adaptability in this field.
Whether you are a student exploring future career options, a working professional considering a pivot into AI, or an employer looking to understand the value an AI Analyst can bring to your organization, this blog serves as a definitive guide. By the end of this report, you will gain a well-rounded understanding of the AI Analyst’s role, its strategic importance in today’s business ecosystem, and the opportunities and challenges that come with it.
In a world increasingly driven by intelligent technologies, the AI Analyst stands at the forefront of innovation, decision-making, and digital transformation. This comprehensive career report aims to illuminate the path forward for individuals and organizations ready to embrace the AI-powered future.
Before we venture further into this article, we would like to share who we are and what we do.
About 9cv9
9cv9 is a business tech startup based in Singapore and Asia, with a strong presence all over the world.
With over nine years of startup and business experience, and being highly involved in connecting with thousands of companies and startups, the 9cv9 team has listed some important learning points in this overview of The AI Analyst: A Comprehensive Career Report.
If your company needs recruitment and headhunting services to hire top-quality employees, you can use 9cv9 headhunting and recruitment services to hire top talents and candidates. Find out more here, or send over an email to hello@9cv9.com.
Or just post 1 free job posting here at 9cv9 Hiring Portal in under 10 minutes.
The AI Analyst: A Comprehensive Career Report
- The Strategic Emergence of the AI Analyst in 2025
- Defining the AI Analyst Role in 2025: Scope, Functions, and Evolving Responsibilities
- Cracking the AI Analyst Interview in 2025
- Mapping the Future of the AI Analyst Career in 2025
- Decoding Compensation Trends
- The AI Analyst in Action
- The Expanding Career Horizon
- Key Challenges and Strategic Considerations for AI Analysts in 2025
- Embracing the AI Analyst Profession: A Future-Forward Career in 2025
1. The Strategic Emergence of the AI Analyst in 2025
As the global economy accelerates its adoption of artificial intelligence (AI), a new generation of professionals is rising to prominence—AI Analysts. These individuals are becoming indispensable to modern enterprises, acting as the crucial bridge between technical AI systems and business outcomes. “The AI Analyst: A Comprehensive Career Report in 2025” offers an authoritative, data-driven exploration of this rapidly evolving career path. This report dissects the AI Analyst’s function across industries, required qualifications, emerging technologies, and salary benchmarks while forecasting career growth and transition opportunities in a dynamic job market.
The Strategic Emergence of the AI Analyst in 2025
- AI as a Business Imperative:
- AI is now integral to digital transformation initiatives across virtually all industries.
- Organizations leverage AI to streamline operations, enhance decision-making, personalize customer experiences, and reduce human error.
- The gap between AI’s technical complexity and business utility necessitates skilled interpreters—enter the AI Analyst.
- The Core Role of the AI Analyst:
- Functions as a translator between AI models and business objectives.
- Evaluates algorithmic performance and adjusts models based on real-world feedback.
- Advises decision-makers by transforming raw AI-generated outputs into strategic insights.
Key Responsibilities and Core Functions
- Data-Centric Tasks:
- Extracts, preprocesses, and analyzes massive datasets to train and validate AI models.
- Develops dashboards, visualization tools, and reports to communicate trends.
- AI Model Oversight:
- Collaborates with data scientists and ML engineers to refine algorithms.
- Ensures ethical and unbiased AI application through model auditing and fairness analysis.
- Business Integration:
- Identifies high-impact areas for AI implementation within departments (e.g., marketing, logistics, customer support).
- Manages the lifecycle of AI products from development to deployment and monitoring.
Technical and Interpersonal Skill Requirements
Skill Category | Key Skills Required |
---|---|
Technical Skills | Python, R, SQL, TensorFlow, Scikit-learn, data wrangling |
Analytical Skills | Statistics, hypothesis testing, model evaluation |
Communication Skills | Business storytelling, data visualization, stakeholder reporting |
Ethical & Regulatory Awareness | AI fairness, GDPR, explainability, data governance |
- Interdisciplinary Proficiency:
- Combines computer science expertise with business strategy acumen.
- Requires familiarity with both AI theory and the commercial implications of AI solutions.
AI Analyst Career Pathways and Advancement
- Entry-Level to Executive Progression:
- Starts typically with roles such as AI Research Assistant or Junior AI Analyst.
- Progresses to Senior AI Analyst, AI Strategy Consultant, or Head of AI Operations.
- Potential Transition Tracks:
- Into specialist fields: Machine Learning Engineer, Data Scientist.
- Into leadership: AI Product Manager, Director of AI Innovation, Chief Data Officer.
- Cross-Functional Mobility:
- Professionals from data science or data analytics backgrounds can pivot into AI analysis through targeted upskilling in AI/ML.
AI Analyst vs. Related Roles: A Comparative Overview
Role | Focus Area | Technical Depth | Business Strategy |
---|---|---|---|
AI Analyst | Bridging AI models and business insights | Medium-High | High |
Data Scientist | Building complex data models | High | Medium |
Data Analyst | Historical data trends and reporting | Medium | Low-Medium |
ML Engineer | Model deployment and optimization | Very High | Low |
- The AI Analyst uniquely balances both the technical rigor of AI/ML and the strategic orientation required by business leaders.
Industry Demand and Job Market Outlook in 2025
- Increased Market Demand:
- 78% of global enterprises report plans to expand AI investments by end of 2025 (source: McKinsey/Statista).
- AI Analysts are ranked among the top 10 fastest-growing jobs in the technology sector.
- Key Hiring Industries:
- Finance & Banking
- Healthcare & Pharmaceuticals
- Retail & E-commerce
- Manufacturing & Supply Chain
- Government & Public Services
Compensation Trends and Salary Benchmarks
Region | Entry-Level Salary (USD) | Mid-Level (USD) | Senior-Level (USD) |
---|---|---|---|
North America | $75,000 – $95,000 | $100,000 – $130,000 | $140,000 – $180,000 |
Europe | €55,000 – €70,000 | €75,000 – €95,000 | €100,000 – €130,000 |
Asia-Pacific | $40,000 – $60,000 | $65,000 – $90,000 | $95,000 – $120,000 |
Middle East | $45,000 – $65,000 | $70,000 – $90,000 | $95,000 – $115,000 |
- Salaries vary based on:
- Industry: Finance and tech sectors pay premium rates.
- Experience: Senior roles often include stock options or performance bonuses.
- Location: Urban hubs such as New York, London, Dubai, and Singapore offer higher pay scales.
Future Outlook and Upskilling Opportunities
- Key 2025 Trends:
- Growing importance of AI ethics and governance.
- Integration of generative AI and multimodal AI models into standard analytics pipelines.
- Expansion of low-code/no-code AI platforms, enhancing accessibility for business users.
- Recommended Certifications:
- IBM AI Analyst Professional Certificate
- Google Cloud Machine Learning Engineer
- Microsoft Certified: Azure AI Fundamentals
- Coursera/edX AI Specializations
- Upskilling Channels:
- Online learning platforms (Coursera, edX, Udacity)
- Specialized bootcamps in AI/ML
- Master’s degrees in Data Science, AI, or Business Analytics
Conclusion: Why This Report Matters in 2025
“The AI Analyst: A Comprehensive Career Report” is more than just an overview—it is an indispensable resource for professionals, educators, HR strategists, and business leaders who wish to understand and navigate the future of AI-integrated workforces. As AI continues to transform global commerce, the AI Analyst stands as a key architect of intelligent enterprise strategies.
2. Defining the AI Analyst Role in 2025: Scope, Functions, and Evolving Responsibilities
The role of the AI Analyst in 2025 is characterized by its intersectional blend of technical depth, strategic insight, and ethical oversight. As enterprises increasingly embed artificial intelligence into core business functions, AI analysts have emerged as the linchpin professionals who ensure these systems are both effective and aligned with organizational objectives. This section offers a detailed examination of the modern AI analyst’s functions, required skills, and how the role differs from or overlaps with adjacent professions in the data and AI ecosystem.
Core Functions and Responsibilities of an AI Analyst
AI analysts are instrumental in bridging the complex world of machine intelligence with real-world business applications. Their core responsibilities span the entire lifecycle of AI solution development and deployment.
AI Model Development & Implementation
- Analyzing large, complex datasets to identify patterns and opportunities for AI deployment.
- Designing, developing, and refining AI and machine learning models using statistical methods and advanced algorithms.
- Utilizing AI frameworks (e.g., TensorFlow, PyTorch, Keras) to implement intelligent solutions.
- Fine-tuning models to improve accuracy, efficiency, and performance relative to business KPIs.
Lifecycle Management of AI Projects
- Managing end-to-end AI project execution—from ideation to deployment and post-deployment monitoring.
- Collaborating with:
- Data scientists to enhance model sophistication.
- Software engineers to ensure production-ready implementation.
- Business leaders to align AI outputs with strategic objectives.
- Monitoring model performance over time and iterating based on new data or shifting requirements.
Business Translation and Communication
- Acting as an interpreter between technical teams and non-technical stakeholders.
- Producing clear, insightful reports and visualizations to support executive decision-making.
- Delivering stakeholder-specific presentations that contextualize AI outcomes in business terms.
Ethical AI Oversight
- Embedding ethical considerations into AI development, including:
- Bias detection and mitigation
- Fairness and transparency protocols
- Data privacy compliance (e.g., GDPR, HIPAA)
- Ensuring responsible AI practices are followed across the development pipeline.
Continuous Learning & Innovation
- Staying abreast of emerging AI technologies, frameworks, and methodologies.
- Proactively integrating state-of-the-art developments into existing AI solutions to maintain innovation edge.
Essential Skills and Technological Competencies
A modern AI analyst must possess a broad and deep skill set, spanning from algorithmic theory to business communication.
Technical Proficiencies
Category | Tools/Concepts |
---|---|
Programming Languages | Python, R, Java, C++ |
AI Frameworks | TensorFlow, PyTorch, Keras |
Data Tools | SQL, Hadoop, Spark |
Visualization Platforms | Power BI, Tableau, Matplotlib, Seaborn |
Cloud Platforms | AWS, Microsoft Azure, Google Cloud Platform |
Machine Learning Concepts | Supervised/unsupervised learning, neural networks, deep learning, NLP |
Soft and Strategic Skills
- Strong analytical reasoning and diagnostic thinking.
- Strategic problem-solving and decision support.
- Ability to communicate complex ideas in simple, impactful ways.
- Cross-functional collaboration and stakeholder management.
- Adaptability in fast-paced, innovation-driven environments.
AI Analyst vs. Related Roles: Key Differences
Understanding the distinction between AI analysts and adjacent roles is vital for organizations seeking the right talent, as well as for professionals planning their career trajectories.
Role | Primary Focus Area | Degree of AI Specialization | Business Strategy Focus |
---|---|---|---|
AI Analyst | Application of AI/ML to solve business problems | High | High |
Data Analyst | Descriptive data insights and reporting | Low to Moderate | Moderate |
Data Scientist | Experimental model development & research | Very High | Medium |
AI Engineer | System architecture and deployment engineering | High | Low |
Role Comparison: Core Differentiators
- AI Analyst:
- Applies ML/AI to solve practical business challenges.
- Involved in performance monitoring and ongoing optimization.
- Balances technical depth with strategic communication.
- Data Scientist:
- Focuses on experimental design and algorithm innovation.
- Often contributes to foundational AI research.
- AI Engineer:
- Concerned with operational scalability and system reliability.
- Builds backend infrastructure for AI integration.
- Data Analyst:
- Uses conventional statistical methods for data exploration.
- Limited use of AI-specific technologies.
The Growing Responsibility for Ethical AI in Practice
The AI analyst is increasingly tasked with implementing ethical guardrails in a space that’s rapidly expanding in complexity and societal impact.
Why Ethical AI Is Critical
- AI is being adopted in sensitive domains such as:
- Finance (e.g., credit scoring)
- Healthcare (e.g., diagnostics)
- Law enforcement (e.g., facial recognition)
- These use cases carry high stakes for fairness, bias, and accountability.
Ethical Responsibilities of AI Analysts
- Conducting audits for bias in data and models.
- Implementing explainability techniques to increase model transparency.
- Ensuring privacy and regulatory compliance (e.g., anonymizing personal data).
- Aligning technical solutions with corporate governance standards and social impact policies.
The Communication Imperative in AI Analytics
Technical acumen alone is not sufficient. The value AI analysts deliver is only realized when their insights are:
- Understood by decision-makers.
- Integrated into business strategy.
- Trusted across organizational levels.
Core Communication Functions
- Translating algorithmic findings into actionable business strategies.
- Developing dashboards that enable executive-level visibility into AI performance.
- Leading presentations that clarify the implications and ROI of AI projects.
Conclusion: A Mission-Critical Role in a Data-Driven Future
The AI Analyst is not simply a data practitioner—they are a strategic innovator, ethical steward, and business communicator. In 2025, as AI cements itself as a foundational element of enterprise strategy, the AI Analyst stands at the intersection of technological capability and business value.
Professionals entering or evolving into this role must demonstrate fluency across a diverse spectrum of skills—from neural network configuration to ethical evaluation, from data architecture to executive storytelling. For companies aiming to drive meaningful transformation through AI, investing in skilled AI analysts will be critical to achieving sustainable, responsible, and scalable innovation.
3. Cracking the AI Analyst Interview in 2025
As organizations continue to invest heavily in artificial intelligence to drive innovation and streamline decision-making processes, the demand for qualified AI analysts has soared. Consequently, interviews for AI analyst roles have become increasingly rigorous, testing not only theoretical knowledge and practical expertise but also communication skills, ethical awareness, and real-world problem-solving capabilities. This section provides an in-depth overview of how candidates can effectively prepare to navigate the multifaceted AI analyst interview process in 2025.
Technical Interview Focus Areas: Key AI Concepts and Their Applications
Modern AI analyst interviews are built to assess a candidate’s foundational understanding of artificial intelligence, machine learning, and deep learning, alongside their ability to apply this knowledge practically.
Core Topics to Master:
- Artificial Intelligence Hierarchy:
- Differentiate between Artificial Intelligence (AI), Machine Learning (ML), and Deep Learning (DL).
- Understand AI categories: Reactive Machines, Limited Memory, Theory of Mind, and Self-Aware AI.
- Know the distinctions between Narrow AI vs. General AI.
- Types of Machine Learning:
- Supervised Learning: Models trained using labeled datasets.
- Unsupervised Learning: Algorithms designed to identify hidden structures in unlabeled data.
- Reinforcement Learning: Decision-making models based on trial-and-error strategies.
- Neural Network Architecture:
- Basics of feedforward neural networks and backpropagation.
- Understanding of Convolutional Neural Networks (CNNs) and their use in image recognition.
- Knowledge of Recurrent Neural Networks (RNNs) for sequence-based data.
- Model Optimization Concepts:
- Bias-Variance Tradeoff: Balancing model complexity vs. generalization.
- Overfitting & Underfitting: Identification and mitigation strategies.
- Loss Functions: Role in training and performance evaluation.
Algorithmic Proficiency: Commonly Assessed AI Models and Techniques
A candidate’s familiarity with a diverse range of machine learning algorithms is essential. Interviews may require explanation, comparison, and application of the following:
Common Algorithms Covered:
- Regression & Classification:
- Linear and Logistic Regression
- Decision Trees and Random Forests
- Support Vector Machines (SVMs)
- Clustering Techniques:
- K-Means Clustering
- Hierarchical Clustering
- DBSCAN for density-based clustering
- Advanced Models:
- Generative Adversarial Networks (GANs)
- Concepts: Generator, Discriminator, Latent Space
- Reinforcement Learning Methods
- Q-Learning
- Markov Decision Processes (MDPs)
- Hidden Markov Models (HMMs)
- Generative Adversarial Networks (GANs)
Evaluating Model Performance: Metrics and Diagnostic Tools
A critical component of AI analyst responsibilities is measuring and improving model effectiveness. Interviews often include deep dives into performance assessment.
Classification Metrics:
- Accuracy, Precision, Recall, F1-Score
- Confusion Matrix Analysis
- ROC-AUC Curve
Regression Metrics:
- Mean Squared Error (MSE)
- Root Mean Squared Error (RMSE)
- R-Squared (Coefficient of Determination)
Generative Model Evaluation:
- Inception Score (IS)
- Fréchet Inception Distance (FID)
Handling Imbalanced Datasets:
- Oversampling and Undersampling
- SMOTE (Synthetic Minority Over-sampling Technique)
- Precision-Recall Tradeoff Analysis
Essential Tools & Technologies for AI Analysts
To succeed in technical interviews, candidates must demonstrate proficiency with industry-standard tools used for AI development, deployment, and analysis.
Programming Languages:
- Python (primary language for AI development)
- R (statistical computing)
- SQL (data querying)
AI Frameworks & Libraries:
- TensorFlow and PyTorch (model building)
- Keras (user-friendly neural network API)
- Scikit-learn (machine learning algorithms)
Cloud AI Services:
- AWS SageMaker
- Microsoft Azure Machine Learning
- Google Cloud AI Platform
Visualization & Reporting Tools:
- Power BI
- Tableau
- Matplotlib and Seaborn (Python libraries)
Behavioral Interview Strategy: Soft Skills That Define Success
Behavioral interviews are designed to evaluate how candidates approach team collaboration, decision-making, and communication in a business context.
Typical Behavioral Questions:
- “Describe a time you led a challenging AI project and how you resolved issues.”
- “How do you manage conflicting priorities in a fast-paced AI environment?”
- “Share an example of explaining AI concepts to a non-technical stakeholder.”
- “How do you stay current with AI advancements?”
- “Describe a situation where your attention to detail prevented a major mistake.”
Key Traits Assessed:
- Communication and storytelling
- Emotional intelligence and conflict resolution
- Curiosity and commitment to continuous learning
- Adaptability and quick learning
- Data-driven decision-making
Case Studies and Problem Scenarios: Applying AI in Real-World Business Challenges
Many interviewers now include role-specific case studies to assess a candidate’s critical thinking, business acumen, and AI implementation capabilities.
Sample Scenarios:
Business Challenge | AI Application Strategy |
---|---|
E-Commerce Recommendations | Develop a content-based or collaborative filtering system using user interaction data. |
Fraud Detection in Finance | Build a classification model using transaction data with anomaly detection. |
Churn Prediction in Telecom | Apply logistic regression or decision trees on user activity and support logs. |
Bias Mitigation in Recruitment AI | Audit model fairness using demographic parity and apply de-biasing techniques. |
Poor Model Performance | Diagnose using learning curves, re-train with hyperparameter tuning, and assess feature selection. |
Final Thoughts: What Employers Seek in 2025 AI Analyst Candidates
The AI analyst interview process in 2025 is designed to identify professionals who combine deep theoretical grounding with practical, strategic thinking. Employers prioritize:
- Strong Foundational Knowledge: Especially in core ML/AI concepts.
- Real-World Application Skills: Ability to translate theory into tangible results.
- Clear Communication: Essential for stakeholder alignment and business integration.
- Collaborative Mindset: Working across data science, engineering, and business teams.
- Ethical Awareness: Ensuring AI solutions are transparent, fair, and accountable.
4. Mapping the Future of the AI Analyst Career in 2025
In 2025, the role of the AI Analyst stands not only as a gateway into the dynamic field of artificial intelligence but also as a versatile platform from which professionals can evolve into a range of high-impact roles. As organizations deepen their reliance on AI-driven insights for strategic and operational decision-making, the career path of the AI Analyst offers extensive opportunities for advancement, specialization, and leadership.
Career Progression: From Entry-Level to Executive Leadership
The AI Analyst career ladder supports steady upward mobility, with opportunities to transition from technical execution to strategic oversight.
Entry-Level and Intermediate Roles
- Junior AI Analyst / AI Analyst:
- Focus on foundational tasks such as data cleaning, exploratory data analysis (EDA), and supporting senior analysts with model evaluations.
- Collaborate with data engineering teams to acquire and prepare datasets.
- Senior AI Analyst / AI & ML Analyst:
- Lead more complex AI projects.
- Specialize in machine learning pipelines and deploy production-ready models.
- Provide mentorship to junior analysts and contribute to strategic discussions.
Advanced and Strategic Roles
- AI Consultant:
- Act as a bridge between technical teams and business stakeholders.
- Provide AI strategy guidance, often working across various clients or business units.
- AI Lead / AI Manager:
- Manage cross-functional teams and oversee project execution from conception to deployment.
- Ensure models align with organizational goals and compliance standards.
- AI Director / Chief AI Officer (CAIO):
- Responsible for defining and executing the organization’s AI vision.
- Drive enterprise-level innovation, investment in AI infrastructure, and ethical governance.
Cross-Functional Career Transitions: Specialization Beyond the Analyst Role
The transferable expertise developed as an AI Analyst opens pathways into several specialized and technical domains within AI and data science.
Specialization Opportunities
Role Title | Focus Area | Description |
---|---|---|
Data Scientist (AI-Focused) | Predictive Modeling | Specializes in statistical analysis, predictive algorithms, and hypothesis testing. |
Machine Learning Engineer | Model Deployment | Implements, tests, and optimizes ML models in production environments. |
AI Engineer | System Design | Designs scalable AI infrastructure and APIs to support enterprise use cases. |
AI Architect | Strategic Oversight | Develops high-level AI systems architecture and integration strategies. |
Natural Language Processing (NLP) Engineer | Text & Language Data | Focuses on sentiment analysis, chatbots, translation, and speech recognition. |
Computer Vision Engineer | Visual Data | Specializes in image recognition, facial detection, and video analysis algorithms. |
AI Product Manager | Business & Tech Alignment | Oversees the development and delivery of AI-driven products, translating business needs into technical requirements. |
AI Ethics Specialist | Responsible AI | Addresses fairness, transparency, and bias in AI systems; ensures ethical AI deployment. |
Business Intelligence Analyst (AI-Enhanced) | Data-Driven Strategy | Leverages AI tools to generate business insights, forecasting, and dashboards. |
Data Engineer | Data Infrastructure | Builds and maintains robust data pipelines that support scalable AI applications. |
Upskilling and Continuous Learning: Staying Ahead in a Rapidly Evolving Field
In a field as fast-paced as artificial intelligence, continuous learning is not optional—it’s essential for long-term success and relevance.
Professional Certifications for AI Analysts
Earning industry-recognized certifications can significantly enhance an AI analyst’s credibility and technical expertise.
Certification Title | Issuing Body | Focus Area |
---|---|---|
Certified Artificial Intelligence Professional (CAIP) | Global Tech Councils | General AI and ML principles |
IBM AI Engineering Professional Certificate | IBM / Coursera | AI pipelines, deep learning, NLP |
AWS Certified Machine Learning – Specialty | Amazon Web Services | ML on cloud infrastructure |
Microsoft Azure AI Engineer Associate | Microsoft | AI services on Azure cloud |
Google Professional Machine Learning Engineer | Google Cloud | Production-level ML solutions |
Advanced Academic Qualifications
- Master’s Degree (M.Sc.) in AI, Machine Learning, or Data Science:
- Emphasizes theoretical depth, research skills, and applied knowledge.
- Ph.D. in AI or Computer Science:
- Focuses on AI innovation, new algorithm design, and academic or R&D leadership roles.
The Versatility of the AI Analyst Role: A Launchpad to Diverse Career Domains
One of the most valuable aspects of the AI Analyst position is its adaptability across domains, industries, and disciplines.
Adaptive Career Scenarios
- An analyst fascinated by human-computer language interactions may transition into NLP Engineering, working on sentiment analysis or chatbots.
- One intrigued by real-world physical systems might move into Robotics Engineering, integrating AI into autonomous machines.
- A data-focused professional with strong strategic thinking could evolve into an AI Product Manager, steering AI tools from conception to launch.
- Those concerned with the ethical implications of AI may pivot to AI Policy and Ethics, advocating for fair and explainable AI systems in public and private sectors.
Key Takeaways: Building a Sustainable and Impactful AI Career in 2025
The AI Analyst role in 2025 is far more than a stepping-stone—it is a foundational position that builds the critical thinking, technical proficiency, and business acumen necessary for advancement in the broader artificial intelligence ecosystem.
Career Planning Recommendations:
- Define Specialization Areas Early: Based on interests in NLP, CV, deployment, or strategy.
- Invest in Continuous Learning: Stay current with AI research, open-source frameworks, and emerging regulations.
- Build a Portfolio: Document and publish AI projects, Kaggle competitions, or contributions to GitHub.
- Cultivate Soft Skills: Leadership, communication, and ethical reasoning are increasingly valued.
- Pursue Certifications: To validate your expertise and remain competitive in a credential-driven market.
5. Decoding Compensation Trends
As the artificial intelligence sector continues its rapid expansion, the compensation structure for AI Analysts in 2025 reflects both the increasing demand for AI expertise and the broad range of responsibilities associated with this evolving role. Salary variations are influenced by experience level, job scope, specialization, geography, and the maturity of the employing organization’s AI strategy.
Average Salary Benchmarks for AI Analysts in 2025
AI Analysts in the United States earn competitive compensation packages that vary widely depending on role expectations and expertise.
Core Salary Ranges (2025 Data)
Role Type | Average Annual Salary | 25th Percentile | 75th Percentile | 90th Percentile |
---|---|---|---|---|
General AI Analyst | $100,058 | $77,000 | $120,500 | $137,000 |
AI Data Analyst | $82,640 | $62,500 | $97,000 | $120,500 |
Alternative Estimate | $66,684 (avg.) | $57,178 | $77,255 | N/A |
Pecan AI Estimate | $90,000 – $310,000 | Varies by role | Mid–High | Senior Expert |
Robert Half (AI/ML Analyst) | $119,250 – $169,000 | Location-based | Higher in metro areas | Up to $206,180 in Chicago |
Insight: The wide disparity in reported salary figures underscores how the “AI Analyst” title encompasses various roles—from entry-level analysts to highly specialized professionals contributing to strategic AI initiatives.
Salary Comparisons: Related AI & ML Roles
To understand where AI Analysts stand in the broader AI job market, it is helpful to compare compensation data with adjacent, more technically demanding roles.
Salary Comparison Table
Position Title | 25th Percentile | Median Salary | 75th Percentile |
---|---|---|---|
AI Architect | $139,250 | $171,000 | $193,000 |
Machine Learning Engineer | $128,750 | $163,500 | $185,750 |
Data Scientist | $121,750 | $147,750 | $171,000 |
General AI Roles (Talent.com) | N/A | $154,273 | N/A |
Interpretation: AI Analysts are often positioned as entry or mid-level contributors, whereas roles such as AI Architect and Machine Learning Engineer demand deeper technical expertise and command significantly higher salaries.
Key Factors Influencing AI Analyst Compensation
Several variables shape compensation packages within the AI domain. These factors extend beyond years of experience to include geography, educational background, industry specialization, and technical skillset.
Influential Salary Drivers
- Professional Experience
- Entry-level analysts often start at lower salaries.
- Professionals with 5–10 years of experience can command mid-to-high six-figure packages.
- Geographic Location
- Salaries are significantly higher in tech hubs such as:
- San Francisco Bay Area
- New York City
- Seattle
- Chicago (AI Analyst salaries range from $145,485 to $206,180)
- Salaries are significantly higher in tech hubs such as:
- Industry Sector
- AI roles in finance, defense, healthcare, and big tech pay more due to reliance on AI innovation and data processing.
- Startups may offer lower salaries but provide equity or fast career advancement.
- Specialized Skillsets
- Expertise in the following areas typically commands a premium:
- Natural Language Processing (NLP)
- Deep Learning
- Computer Vision
- Model Deployment and MLOps
- Generative AI
- Expertise in the following areas typically commands a premium:
- Education and Certification
- Holding a Master’s or Ph.D. in Data Science, Computer Science, or AI often leads to higher compensation.
- Industry-recognized certifications (e.g., AWS ML, Google ML Engineer, IBM AI) enhance credibility and negotiating power.
Variability in Role Definitions and Salary Expectations
The “AI Analyst” job title lacks standardization across industries, leading to significant disparities in job scope and compensation. This variability is critical for professionals to consider when evaluating salary benchmarks.
Role Scope Variance
- Entry-Level Data Analyst with AI Exposure:
- Tasks limited to data cleaning, basic visualization, or assisting in algorithm evaluation.
- Lower salary bands.
- Advanced AI Analyst / ML Specialist:
- Involved in model development, deployment, and strategic implementation.
- Salaries aligned with Machine Learning Engineers or Data Scientists.
Organizational Maturity
- Startups may use the title “AI Analyst” for high-level responsibilities, while larger enterprises may restrict the role to support functions.
- Career progression typically leads to high-paying roles such as:
- AI Architect
- AI Engineer
- ML Engineer
- Chief AI Officer (CAIO)
Strategic Insights for AI Career Planning
For aspiring and current AI Analysts, understanding how compensation aligns with evolving responsibilities is essential for long-term career strategy.
Recommendations for Maximizing Compensation
- Clarify Job Descriptions: Analyze responsibilities, not just titles, when comparing offers.
- Research Market Rates: Use multiple data sources (e.g., Robert Half, Glassdoor, Talent.com) for informed negotiations.
- Invest in Continuous Learning: Enroll in certification programs and stay updated with AI advancements.
- Negotiate Intelligently: Tailor your pitch around specific AI tools, past impact, and domain expertise.
- Target Strategic Locations or High-Growth Industries: Consider relocating to AI-focused metro areas or industries with active digital transformation agendas.
Visual Snapshot: AI Analyst Salary Ladder in 2025
$60,000 ───────── Entry-Level AI Analyst
$82,640 ───────── AI Data Analyst Average
$100,000 ──────── General AI Analyst Median
$120,500 ──────── 75th Percentile
$137,000 ──────── 90th Percentile
$169,000 ──────── Top AI/ML Analyst (Robert Half)
$193,000+ ─────── AI Architect / ML Engineer Peak
$310,000 ──────── Elite Level (Pecan AI estimate)
Conclusion:
The AI Analyst role in 2025 serves as both a stable entry point and a springboard to more lucrative, technically intensive positions. As the AI landscape matures, understanding salary dynamics and strategically aligning one’s skills with market needs will be instrumental for maximizing compensation and career satisfaction.
6. The AI Analyst in Action
As artificial intelligence becomes a transformative force across industries, the role of the AI Analyst has evolved into a dynamic, multidisciplinary position. In 2025, AI Analysts operate at the intersection of data science, machine learning, and business strategy—translating raw data into intelligent solutions that drive innovation and efficiency.
Core Daily Responsibilities of an AI Analyst
The daily workflow of an AI Analyst integrates a balance of technical execution, collaborative teamwork, and strategic insight. Though the title centers on artificial intelligence, a substantial part of the role focuses on foundational data practices essential to successful AI outcomes.
Key Daily Tasks
- Data Acquisition & Preparation
- Identify and extract data from diverse sources: internal databases, APIs, web platforms, and spreadsheets.
- Perform thorough data cleansing to remove duplicates, handle missing values, and correct inconsistencies.
- Structure data using preprocessing techniques to ensure compatibility with ML algorithms.
- Data Analysis & Pattern Recognition
- Conduct exploratory data analysis (EDA) using statistical tools and AI models.
- Detect patterns, anomalies, and key trends to inform business decisions.
- Utilize tools such as Python, R, SQL, and AI libraries (TensorFlow, Scikit-learn, PyTorch).
- Machine Learning Model Development
- Design and implement predictive models tailored to business objectives.
- Train and validate models using supervised, unsupervised, or reinforcement learning methods.
- Fine-tune parameters to optimize accuracy and performance metrics (e.g., precision, recall, F1 score).
- Cross-Functional Collaboration
- Engage with stakeholders, software engineers, product teams, and data scientists to align objectives.
- Translate complex technical results into actionable insights for non-technical audiences.
- Model Deployment & Monitoring
- Integrate machine learning models into production environments or business systems.
- Continuously monitor model performance; retrain or recalibrate as needed.
- Address model drift and data pipeline issues to ensure reliability over time.
- Reporting & Communication
- Develop dashboards, visualizations, and documentation for internal reporting.
- Present findings in stakeholder meetings, using storytelling and data-driven narratives.
- Document modeling decisions and experimental methodologies for reproducibility.
- Research & Upskilling
- Stay abreast of emerging trends in AI tools, model architectures, and ethical frameworks.
- Experiment with cutting-edge AI applications (e.g., large language models, explainable AI).
Typical AI Analyst Projects Across Industries
The versatility of AI technologies enables analysts to contribute meaningfully across sectors. Their projects address a range of organizational challenges, from operational efficiency to customer engagement and risk mitigation.
Common Use Cases by Industry
Industry | Project Type | Objective |
---|---|---|
Finance | Fraud detection systems | Identify unusual transaction patterns |
Stock price forecasting models | Predict market trends using historical data | |
E-Commerce | Product recommendation engines | Enhance customer experience and increase sales |
Sentiment analysis from customer reviews | Understand brand perception | |
Healthcare | Predictive diagnostic models | Assist with early disease detection |
NLP models for medical transcription | Automate documentation processes | |
Manufacturing | Predictive maintenance algorithms | Prevent equipment failure and reduce downtime |
Supply chain optimization models | Improve logistics and cost-efficiency | |
Government & Urban Planning | Traffic flow prediction systems | Optimize city infrastructure and reduce congestion |
Media & Tech | Fake news detection tools | Combat misinformation on digital platforms |
AI-powered language translation | Enable multilingual communication | |
Human Resources | Resume parsers and candidate ranking algorithms | Streamline recruitment and reduce bias |
Customer Service | Intelligent chatbots and virtual assistants | Automate and scale customer support |
Visual Summary: Scope of AI Analyst Projects
+---------------------+---------------------------+
| Industry | AI Project Type |
+---------------------+---------------------------+
| Finance | Fraud Detection, Forecast |
| E-commerce | Recommendations, Sentiment|
| Manufacturing | Maintenance, Logistics |
| Healthcare | Diagnostics, NLP Models |
| HR & Recruitment | Resume Parsing |
| Public Sector | Traffic Prediction |
| Tech & Media | Chatbots, Fake News AI |
+---------------------+---------------------------+
The Crucial Role of Data Wrangling
Despite the AI-centric title, a significant proportion of an AI Analyst’s workload involves data wrangling—the transformation of raw data into structured, usable formats that can support machine learning models.
Data Preparation Workflow
- Source Identification: Determine relevant data repositories aligned with project goals.
- Data Extraction: Pull structured, semi-structured, and unstructured data.
- Cleaning & Quality Assurance:
- Remove null values and outliers
- Address inconsistencies in data formatting
- Feature Engineering: Derive new variables that enhance model performance.
- Normalization & Encoding: Standardize data for algorithm compatibility.
Insight: High-quality data preparation is not only time-intensive but also essential for ensuring the accuracy, relevance, and generalizability of AI models.
The Value AI Analysts Bring to Modern Organizations
AI Analysts are pivotal in bridging the gap between complex AI technologies and practical business applications. Their ability to convert raw data into intelligent, scalable solutions drives operational efficiency and competitive advantage.
Strategic Impact of the Role
- Enable data-driven decision-making across departments.
- Reduce manual workload through AI automation tools.
- Improve customer engagement with hyper-personalized experiences.
- Increase predictive accuracy in strategic forecasting initiatives.
- Enhance organizational agility through continuous learning and optimization loops.
Conclusion: A Profession Defined by Diversity and Innovation
In 2025, the AI Analyst role is characterized by a robust combination of technical fluency, problem-solving acumen, and business awareness. As AI continues to permeate diverse sectors, the projects undertaken by these professionals highlight the broad applicability and transformative potential of artificial intelligence.
The expansive and evolving nature of this career provides AI Analysts with continuous opportunities to innovate, collaborate, and contribute to meaningful progress across industries.
7. The Expanding Career Horizon
In 2025, the job market for AI Analysts reflects the accelerating integration of artificial intelligence across global industries. As businesses increasingly adopt intelligent systems to gain strategic advantages, demand for professionals skilled in AI analysis, data interpretation, and machine learning has surged. This section offers a comprehensive look into the employment trends, sector-specific demand, and future career prospects shaping the AI Analyst profession.
Accelerating Market Growth for AI Roles
The global proliferation of AI technologies has catalyzed an unprecedented expansion in employment opportunities for AI specialists, with the role of the AI Analyst being a central pillar.
Global Job Market Indicators
- Explosive Growth in AI-Related Job Postings:
- AI-related job listings grew by 21% from 2018 to mid-2024, indicating sustained interest in AI talent across industries.
- LinkedIn reported a 38% increase in AI-related job postings from 2020 to 2024, driven by demand for data-driven decision-making.
- Projected AI Employment Surge:
- According to the World Economic Forum, 97 million new AI-related jobs are expected to emerge by 2025, outpacing the number of roles displaced by automation.
- U.S. Market Trends:
- In the United States, AI-related job postings represented 1.62% of all online listings in the first eight months of 2024.
- AI hiring demand has experienced steady growth since 2010, with notable acceleration over the past two years.
Market Size and Economic Outlook
Metric | Value | Timeline |
---|---|---|
Global AI Market Size | $1.8 trillion (projected) | By 2030 |
Compound Annual Growth Rate (CAGR) | 37.3% | 2023–2030 |
AI Jobs Expected by 2025 | 97 million | Global |
Insight: The exponential growth of the AI market signals enduring, high-volume demand for AI analysts, positioning the role as a long-term career with high strategic value.
High-Demand Roles Within AI
Among the wide spectrum of positions within the AI ecosystem, certain titles consistently top recruitment priorities:
- Machine Learning Engineers: Design, build, and optimize machine learning systems.
- AI Analysts: Interpret complex data, uncover patterns, and help guide strategic decisions through AI.
- Data Scientists: Employ advanced analytics and predictive modeling techniques.
- Natural Language Processing Specialists: Focus on AI applications involving human language.
Industries Driving AI Analyst Demand
AI adoption is no longer confined to tech companies. Organizations in virtually every sector are integrating AI into their workflows to enhance efficiency, improve customer experience, and gain a competitive edge.
Sector-Wise AI Analyst Demand
Industry | Primary Applications of AI |
---|---|
Technology & Software | System optimization, AI product development |
Healthcare | Predictive diagnostics, medical imaging, patient data analytics |
Finance & Banking | Fraud detection, algorithmic trading, risk modeling |
Retail & E-commerce | Customer behavior analysis, recommendation systems, inventory control |
Marketing & Advertising | Customer segmentation, campaign optimization, sentiment analysis |
Manufacturing | Predictive maintenance, process automation, supply chain AI |
Automotive | Autonomous vehicle systems, predictive safety models |
Telecommunications | Network optimization, customer churn prediction |
Management Consulting | AI advisory for digital transformation |
Staffing & Recruitment | AI-based candidate screening, HR analytics |
Trends in Sectoral Hiring
- Healthcare: With AI transforming diagnostics and clinical workflows, demand for analysts who can interpret medical data is rapidly increasing.
- Finance: AI Analysts play a critical role in fraud detection, credit risk evaluation, and financial forecasting.
- Retail: Personalized AI systems are revolutionizing customer engagement, leading to strong hiring momentum in e-commerce.
- Manufacturing & Logistics: AI is streamlining operations and predictive systems, requiring continuous analyst support for optimization.
Transformative Factors Shaping AI Analyst Careers
Several structural and technological developments are influencing the evolution of the AI analyst profession:
Emerging Dynamics
- Growth of Generative AI:
- Tools such as GPT, DALL·E, and Codex are not only automating tasks but creating new roles focused on prompt engineering, model training, and AI safety.
- Shift Toward Specialization:
- Employers increasingly prefer deeply specialized experts (e.g., in NLP, computer vision, or predictive analytics) over generalists.
- A rise in demand for professionals with domain-specific expertise, such as healthcare or finance, coupled with technical fluency.
- Hybrid Skill Requirements:
- Successful AI Analysts are expected to master the full data lifecycle: from ingestion to model deployment.
- Emphasis on cross-functional skills combining statistics, engineering, business strategy, and communication.
The Future Outlook: A Career Defined by Scale and Impact
With AI now embedded in the core strategies of global organizations, the AI Analyst role is forecasted to maintain high relevance and strategic importance for the foreseeable future.
Key Growth Drivers
- Increased R&D Investment:
- Governments and corporations alike are funneling substantial resources into AI innovation, expanding job opportunities.
- Cross-Sector Integration of AI:
- Adoption beyond the tech sector includes healthcare, education, logistics, real estate, and more.
- Business Reliance on Predictive Intelligence:
- AI Analysts are central to helping companies forecast trends, detect anomalies, and automate decision-making.
Expanding Career Pathways
- Professionals entering the AI space in 2025 can choose from a diverse array of career tracks, such as:
- Industry-specialized analyst roles (e.g., Financial AI Analyst, Healthcare AI Analyst)
- Transition to leadership (AI Product Manager, AI Strategy Consultant)
- Advanced research and development in AI ethics, fairness, and explainability
Conclusion: The AI Analyst’s career path is no longer niche—it is foundational to the digital transformation agendas of global enterprises. Those who equip themselves with specialized, high-demand skills in data, AI, and machine learning will be best positioned to capitalize on this ongoing revolution.
8. Key Challenges and Strategic Considerations for AI Analysts in 2025
While the role of an AI Analyst is rich with opportunity, it also presents a complex array of challenges. These obstacles span technical, ethical, operational, and strategic dimensions. The effectiveness of AI initiatives—and by extension, the impact of AI Analysts—depends heavily on their ability to navigate and resolve these multifaceted issues.
Data Quality and Reliability: The Foundation of Effective AI
One of the most critical challenges in AI analysis is ensuring the quality and consistency of input data. Poor data undermines the integrity of AI models, regardless of the sophistication of the algorithms applied.
Common Data Quality Challenges
- Incomplete Data: Missing values and inconsistent formats hinder accurate modeling.
- Irrelevant or Noisy Inputs: Unfiltered or non-representative data introduces inaccuracies.
- Data Bias: Historical or systemic biases embedded in data can result in discriminatory outcomes.
- Data Preparation Overhead:
- A significant portion (up to 80% in some AI projects) of time is consumed by data wrangling and cleaning, delaying time to insights.
Conclusion: Strong data governance and engineering capabilities are non-negotiable for AI analysts. Data preprocessing remains the backbone of reliable AI outputs.
Model Bias and Ethical AI: The Rising Demand for Responsible Practices
As AI systems are deployed in decision-critical domains, ensuring fairness and transparency is paramount.
Key Ethical and Bias-Related Challenges
- Embedded Societal Biases:
- Algorithms trained on biased datasets can amplify social inequalities.
- Opaque Decision-Making:
- Complex deep learning models often lack interpretability, reducing trust among stakeholders.
- Ethical Concerns:
- Issues related to data privacy, accountability, and transparency have attracted significant scrutiny.
- Regulatory Pressures:
- Governments and institutions are increasingly enforcing responsible AI frameworks, requiring compliance with ethical standards.
Insight: AI analysts must not only be technically proficient but also well-versed in AI ethics, fairness auditing, and transparency methodologies.
Model Performance and Interpretability
Building high-performance AI models is not just about algorithm selection; it’s about achieving balance between accuracy, generalizability, and transparency.
Technical Performance Challenges
- Overfitting:
- Models perform well on training data but fail to generalize to unseen scenarios.
- Underfitting:
- Models fail to learn key patterns, producing weak predictions.
- Explainability Limitations:
- Deep neural networks often function as “black boxes,” hindering business adoption.
Recommended Best Practices
Challenge | Strategic Solution |
---|---|
Overfitting | Cross-validation, dropout layers, regularization |
Underfitting | Feature engineering, model complexity tuning |
Lack of transparency | SHAP, LIME, model distillation for interpretability |
Actionable Tip: Leveraging explainable AI (XAI) tools helps bridge the gap between model complexity and stakeholder understanding.
Organizational Integration and Business Alignment
For AI to deliver tangible value, its solutions must align seamlessly with existing business goals and infrastructure.
Organizational Hurdles
- Integration Complexity:
- Existing legacy systems often lack the flexibility to accommodate advanced AI pipelines.
- ROI Justification:
- Demonstrating the measurable impact of AI (e.g., cost reduction, revenue lift, operational efficiency) remains a frequent barrier.
- Skill Gaps Within Teams:
- Lack of cross-functional fluency between AI teams and business leaders can stall project implementation.
Strategic Alignment Tactics
- Develop a strong understanding of core business metrics and link model outputs to these KPIs.
- Collaborate with IT departments early in the design phase to ensure infrastructure compatibility.
- Communicate AI value using visual dashboards, projections, and case-based ROI frameworks.
Continuous Learning and Adaptability in a Fast-Evolving Domain
The pace of advancement in AI tools, frameworks, and methodologies is relentless. Staying current is a fundamental requirement.
Rapid Technological Evolution
- New algorithms, open-source libraries, and platforms emerge weekly.
- Staying ahead requires ongoing education in areas like:
- Generative AI
- Federated Learning
- Reinforcement Learning
- AI Governance and Compliance
Professional Development Recommendations
- Pursue certifications in AI, data science, cloud platforms (AWS, GCP).
- Engage in continuous learning through MOOCs, industry whitepapers, and peer-reviewed research.
- Contribute to open-source projects and Kaggle competitions to refine practical skills.
Talent Gaps and the Need for Hybrid Expertise
While demand for AI analysts remains robust, employers frequently report difficulty in finding candidates with the ideal blend of technical, business, and domain-specific skills.
In-Demand Skills Employers Seek
Skill Category | Examples |
---|---|
Technical Skills | Python, SQL, TensorFlow, PyTorch, Scikit-learn |
Analytical Thinking | Statistical analysis, hypothesis testing |
Business Acumen | KPI alignment, ROI modeling, stakeholder comms |
Domain Expertise | Finance, healthcare, logistics, legal |
Advice: A diverse personal portfolio demonstrating applied AI skills in real-world use cases significantly enhances credibility and employability.
Summary: Strategic Imperatives for Future-Ready AI Analysts
To succeed in 2025 and beyond, AI Analysts must:
- Prioritize data quality through rigorous governance protocols.
- Champion ethical AI by identifying and mitigating bias.
- Demonstrate model explainability to foster trust.
- Quantify and communicate business value effectively.
- Remain adaptive through lifelong learning and upskilling.
- Bridge gaps between AI solutions and operational systems.
- Build a strong, relevant project portfolio tailored to current market needs.
9. Embracing the AI Analyst Profession: A Future-Forward Career in 2025
As organizations across the globe accelerate their adoption of artificial intelligence (AI) technologies, the role of the AI Analyst has emerged as a linchpin of innovation, strategy, and data-driven decision-making. This profession offers a highly dynamic and intellectually stimulating pathway for those with a strong analytical mindset, technical fluency, and a passion for solving complex problems.
Core Role Overview: What Does an AI Analyst Do?
An AI Analyst serves as a strategic intermediary between raw data and actionable insights, using artificial intelligence techniques to interpret patterns, automate processes, and optimize decision-making frameworks across industries.
Key Responsibilities
- Data Analysis and Feature Engineering:
- Extracting, cleaning, transforming, and analyzing large-scale datasets.
- Designing input features that optimize model accuracy and performance.
- Model Development and Evaluation:
- Collaborating with data scientists and engineers to build machine learning models.
- Testing, tuning, and validating algorithms using robust statistical techniques.
- Insight Generation and Communication:
- Translating complex technical outcomes into clear, business-relevant insights.
- Presenting findings through reports, dashboards, and data visualizations.
- Ethical Oversight and Bias Mitigation:
- Monitoring AI systems for fairness, transparency, and compliance with regulations.
- Implementing strategies to reduce algorithmic and data-driven bias.
Required Skill Set: The Blend of Technical and Interpersonal Excellence
A career in AI analysis requires not only mastery of data science principles but also the ability to communicate and collaborate effectively within interdisciplinary teams.
Technical Competencies
Skill Domain | Relevant Tools and Techniques |
---|---|
Programming Languages | Python, R, SQL |
Machine Learning | Scikit-learn, TensorFlow, PyTorch, XGBoost |
Data Visualization | Tableau, Power BI, Matplotlib, Seaborn |
Statistical Modeling | Regression analysis, hypothesis testing, clustering |
Data Engineering | ETL pipelines, big data platforms (Spark, Hadoop) |
Soft Skills in High Demand
- Critical Thinking: Framing the right questions and identifying meaningful patterns.
- Communication: Translating technical outputs into business impact.
- Collaboration: Working across departments, including marketing, finance, and IT.
- Adaptability: Embracing continuous learning in a fast-evolving landscape.
Career Pathways and Growth Opportunities
The AI Analyst role is not static—it offers several avenues for specialization, leadership, and strategic influence.
Typical Career Progression
Career Stage | Title | Primary Focus |
---|---|---|
Entry-Level | Junior AI Analyst | Foundational data tasks, assisting with model testing |
Mid-Level | AI Analyst / Data Analyst | Independent analysis, insight generation |
Advanced | Senior AI Analyst | Strategic modeling, project leadership |
Executive / Specialized Track | AI Strategist / ML Engineer / Chief Data Officer | Oversight of enterprise AI strategy and systems |
Compensation Trends: High Demand Meets High Rewards
With AI skillsets in high demand and relatively short supply, the compensation for AI Analysts reflects this competitive landscape.
Estimated Salary Ranges (Global Average, 2025)
Experience Level | Annual Salary (USD) |
---|---|
Entry-Level | $60,000 – $85,000 |
Mid-Level | $90,000 – $120,000 |
Senior-Level | $130,000 – $170,000+ |
Note: Salary figures vary by industry, geography, and organization size. Roles in finance, tech, and healthcare typically command premium compensation.
Daily Work Environment and Industry Applications
The daily routine of an AI Analyst is marked by variety, collaboration, and problem-solving across multiple domains.
Typical Daily Activities
- Designing and executing machine learning experiments.
- Collaborating with stakeholders to refine project goals.
- Developing analytical dashboards and model reports.
- Participating in model review and validation sessions.
Industry Applications
Sector | Common AI Use Cases |
---|---|
Healthcare | Predictive diagnostics, patient risk profiling |
Finance | Fraud detection, credit scoring, algorithmic trading |
Retail & E-Commerce | Personalized recommendations, inventory forecasting |
Manufacturing | Predictive maintenance, process optimization |
Government | Public policy modeling, citizen service automation |
Navigating Challenges: Staying Resilient in a Demanding Field
Despite the rewarding nature of the profession, AI Analysts must confront and adapt to a series of challenges inherent in the discipline.
Major Obstacles
- Data Quality Issues: Poor or inconsistent data hampers model performance.
- Model Interpretability: Explaining black-box algorithms to non-technical stakeholders.
- Ethical Risks: Managing AI’s societal impact, from bias to privacy concerns.
- Rapid Technological Evolution: Constant upskilling is essential to remain relevant.
Strategies for Overcoming Challenges
- Commit to continuous education and certification.
- Build a strong foundation in ethical AI principles.
- Develop explainability techniques for stakeholder engagement.
- Curate a diverse portfolio to showcase applied capabilities.
Future Outlook: A Career of Enduring Relevance and Influence
The trajectory of AI adoption across global industries suggests that the role of the AI Analyst will only grow in importance.
Forecasts for 2025 and Beyond
- AI-related job postings are expected to grow by 30–40% annually.
- Organizations will increasingly require data-to-decision pipelines, with AI Analysts at the core.
- Regulatory oversight will demand greater transparency, positioning ethical AI practitioners in high demand.
Final Takeaway: Is AI Analysis the Right Path?
A career as an AI Analyst in 2025 represents more than just a job—it is a dynamic journey at the confluence of technology, strategy, and human insight. Professionals who cultivate a balanced skill set across analytics, ethics, and communication stand to make meaningful contributions to organizations, societies, and global innovation.
Recommended Next Steps for Aspiring AI Analysts:
- Master technical tools and programming foundations.
- Gain real-world experience through internships and projects.
- Stay informed on emerging AI trends and policies.
- Build a network in AI and data science communities.
Conclusion
In the rapidly evolving digital economy of 2025 and beyond, The AI Analyst emerges as a pivotal profession that bridges the gap between raw data and intelligent decision-making. As artificial intelligence reshapes every sector—from healthcare and finance to retail, manufacturing, and public services—the demand for skilled AI analysts has not only surged but has become essential to sustaining innovation, driving business value, and ensuring ethical deployment of machine intelligence.
This comprehensive career report underscores that an AI analyst is far more than a back-end data technician. They are strategic thinkers, problem solvers, and ethical gatekeepers of tomorrow’s digital infrastructure. Their responsibilities extend across data exploration, model interpretation, stakeholder engagement, and continuous system refinement. Unlike traditional roles limited by static outputs, AI analysts operate in a dynamic, iterative environment where agility, adaptability, and advanced analytical acumen are constantly in demand.
A High-Impact Role at the Crossroads of Innovation and Responsibility
AI analysts serve as architects of intelligent solutions, shaping how organizations automate processes, personalize customer experiences, mitigate risks, and unlock new sources of competitive advantage. Their influence spans:
- Data Governance and Quality Control: Ensuring the foundation of AI models is built on reliable, clean, and representative data.
- Model Accuracy and Bias Mitigation: Designing fair, transparent systems that align with ethical AI practices and regulatory expectations.
- Business Alignment and ROI Tracking: Translating model outputs into actionable insights that directly contribute to key performance indicators (KPIs) and strategic goals.
- Cross-Functional Collaboration: Acting as liaisons between data scientists, business stakeholders, IT departments, and executive leadership.
Career Growth, Compensation, and Opportunities in 2025
The AI analyst career path is characterized by a diverse range of growth trajectories, competitive salaries, and sector-wide relevance. From entry-level positions to senior analytical roles and even executive leadership, professionals in this field are positioned for long-term upward mobility. As AI becomes more embedded in core business functions, organizations are not only increasing their investment in AI talent but are also restructuring teams around these capabilities.
The high compensation associated with this role reflects both the complexity of the skill set required and the transformative value that AI analysts deliver. With continual technological advancements—from generative AI to real-time analytics—those who stay current and continuously enhance their skills are likely to remain in high demand across multiple industries.
Overcoming Challenges: The Road to Excellence
Despite its many advantages, the AI analyst role is not without challenges. Data quality issues, model explainability, ethical dilemmas, and the pressure to consistently demonstrate business impact are ongoing concerns. However, professionals who adopt a proactive mindset—focusing on upskilling, ethical awareness, and strong communication—can not only overcome these hurdles but thrive because of them.
The need for responsible AI development has never been more urgent. As AI systems play larger roles in high-stakes areas such as hiring, lending, public safety, and healthcare diagnostics, the role of the AI analyst becomes more socially consequential. Analysts who commit to building fair, transparent, and human-centered AI solutions will be critical to fostering trust and legitimacy in AI technologies.
Future Outlook: Why the AI Analyst Will Remain Indispensable
Looking ahead, AI analysts are set to become integral players in digital transformation strategies worldwide. As organizations seek to scale AI across departments and use cases, the demand for professionals who can both implement and interpret these systems will intensify. Key future trends that will shape the profession include:
- Regulatory Expansion: As governments introduce AI-specific legislation, analysts will be responsible for ensuring compliance and ethical alignment.
- Real-Time Analytics and Edge AI: The shift toward instant decision-making will increase the need for analysts skilled in streaming data analysis and deployment on edge devices.
- Human-AI Collaboration: Rather than replacing human workers, AI will augment roles across organizations, with analysts facilitating effective human-machine partnerships.
Final Thoughts: The AI Analyst as a Career of Purpose and Progress
In conclusion, becoming an AI analyst in 2025 offers more than a lucrative job—it offers the chance to be at the forefront of technological and societal progress. It is a profession that demands intellectual curiosity, technical proficiency, ethical mindfulness, and strategic foresight. For those willing to invest in continuous learning and adapt to the shifting landscape of artificial intelligence, the AI analyst role provides unmatched opportunities to shape the future of work, industry, and human innovation.
Whether you are a student exploring future careers, a professional considering a pivot into AI, or a decision-maker looking to understand the value of this role in your organization, the message is clear: The AI Analyst is not just a job—it is a mission-critical profession for the next generation of intelligent systems and data-driven transformation.
If you find this article useful, why not share it with your hiring manager and C-level suite friends and also leave a nice comment below?
We, at the 9cv9 Research Team, strive to bring the latest and most meaningful data, guides, and statistics to your doorstep.
To get access to top-quality guides, click over to 9cv9 Blog.
People Also Ask
What does an AI Analyst do?
An AI Analyst interprets complex data using AI tools, develops machine learning models, and provides insights to help businesses make data-driven decisions.
What skills are required to become an AI Analyst?
Key skills include Python, SQL, machine learning, data visualization, statistics, problem-solving, and knowledge of AI frameworks like TensorFlow or PyTorch.
Is AI analysis a good career in 2025?
Yes, AI analysis is a high-demand, future-proof career with growing opportunities across industries and excellent salary prospects in 2025.
What industries hire AI Analysts?
Industries such as finance, healthcare, retail, manufacturing, tech, marketing, and logistics actively hire AI Analysts for strategic insights and automation.
How much does an AI Analyst earn in 2025?
In 2025, AI Analysts typically earn between $80,000 to $140,000 annually, depending on experience, industry, and geographical location.
What is the difference between an AI Analyst and a Data Scientist?
AI Analysts focus on applying AI techniques to solve problems, while Data Scientists have broader roles including statistical modeling, data engineering, and predictive analytics.
Do AI Analysts need a degree in computer science?
A degree in computer science, data science, or a related field is often preferred, but relevant certifications and experience can also open doors.
Can you become an AI Analyst without a degree?
Yes, with strong technical skills, a solid project portfolio, and certifications in AI and machine learning, it’s possible to become an AI Analyst without a degree.
What programming languages do AI Analysts use?
Python is the most commonly used language, followed by R, SQL, and sometimes Java or C++ for specific AI or ML tasks.
What tools do AI Analysts commonly use?
AI Analysts use tools like Python, TensorFlow, PyTorch, Jupyter Notebooks, Power BI, Tableau, and cloud platforms like AWS or Google Cloud.
Is AI analysis a stressful job?
Like any tech role, AI analysis can be demanding, but those passionate about AI and data typically find it engaging and intellectually rewarding.
How do I prepare for an AI Analyst interview?
Prepare by reviewing machine learning concepts, practicing coding challenges, building end-to-end AI projects, and understanding AI ethics and deployment.
What certifications help in becoming an AI Analyst?
Useful certifications include Google Professional ML Engineer, Microsoft Azure AI Engineer, IBM AI Engineering, and Coursera or edX AI specializations.
Are AI Analysts in high demand in 2025?
Yes, AI Analysts are in high demand in 2025 due to rapid AI adoption in business operations, automation, and decision-making processes.
Do AI Analysts work remotely?
Many AI Analyst roles offer remote or hybrid work options, especially within the tech, finance, and consulting industries.
What are the career growth opportunities for AI Analysts?
AI Analysts can advance to roles such as Senior Analyst, Machine Learning Engineer, Data Scientist, AI Architect, or Chief Data Officer.
What is a typical day like for an AI Analyst?
A typical day involves data cleaning, model development, analyzing results, team collaboration, reporting findings, and learning new AI trends.
What are the biggest challenges for AI Analysts?
Key challenges include handling biased or poor-quality data, keeping up with fast-evolving tools, ensuring model transparency, and ethical AI deployment.
What soft skills are important for AI Analysts?
Communication, critical thinking, teamwork, business acumen, and problem-solving are essential soft skills for effective AI analysis.
Is AI analysis suitable for beginners in tech?
It can be challenging for beginners, but with proper training, mentorship, and consistent practice, it’s an attainable and rewarding path.
How long does it take to become an AI Analyst?
With focused learning, certifications, and project experience, one can become job-ready as an AI Analyst within 12 to 24 months.
Which companies hire AI Analysts?
Tech giants like Google, Amazon, Microsoft, IBM, as well as banks, healthcare companies, startups, and consultancies actively hire AI Analysts.
Do AI Analysts need knowledge of cloud computing?
Yes, familiarity with cloud platforms like AWS, Azure, or GCP is increasingly important for deploying and scaling AI solutions.
Is AI analysis future-proof?
AI analysis is considered future-proof due to the rising demand for AI-driven insights across nearly every sector worldwide.
What educational background is best for AI Analysts?
Degrees in computer science, data science, mathematics, or engineering provide a strong foundation for AI analyst roles.
Can AI Analysts transition into data science roles?
Yes, with added expertise in statistics and advanced analytics, AI Analysts can easily transition into data science positions.
Are internships important for AI Analyst careers?
Internships provide hands-on experience and industry exposure, significantly boosting employability and practical understanding.
What projects should AI Analysts include in their portfolio?
Include projects on predictive modeling, NLP, computer vision, recommender systems, and ethical AI practices to showcase versatility.
How do AI Analysts contribute to business value?
They translate complex data into actionable insights, optimize operations, and drive innovation using machine learning and AI tools.
What is the future of AI Analysts in the next 5 years?
The role will become more strategic, with increased involvement in AI product design, governance, and cross-functional decision-making.
Sources
Robert Half
AI Degree Guide
Pecan AI
Towards AI Jobs
Indeed
Careers360
Bain & Company
DRI Healthcare
Berkeley Research Group
Workable
Coursera
DataCamp
Simplilearn
DEV Community
InterviewBit
Interview Query
TestGorilla
DigitalDefynd
Braintrust
Pickl.AI
Quora
Final Round AI
K21Academy
Kforce
Intuit
Springboard
California Miramar University
4DayWeek.io
eWEEK
Caltech Bootcamps
Dice
Euphoria XR
ProjectPro
upGrad
Aura Intelligence
Software Oasis
European Commission
Magnet ABA
Exploding Topics
NC Commerce
OnHires
University of San Diego
YouTube
ZipRecruiter
Salary.com
Talent.com
365 Data Science
Federal Reserve Bank of Atlanta
Career Group Companies
AIM Research
World Economic Forum
Noble Desktop
Career Village
Pure Search
CareerFoundry
NineTwoThree
Oracle
B EYE
DATAVERSITY
UNU (United Nations University)
Brookings Institution