Key Takeaways
- Craft Clear Job Descriptions: Attract top data science talent by crafting detailed job descriptions that highlight key responsibilities and qualifications.
- Effective Screening Methods: Use tailored screening techniques, including technical assessments and cultural fit evaluations, to identify the best candidates.
- Structured Onboarding Process: Ensure successful integration with a structured onboarding process that includes comprehensive training, role clarity, and mentorship opportunities.
In today’s digital age, data has become the lifeblood of businesses across various industries.
From tech giants to small startups, companies are leveraging data to drive decisions, optimize operations, and gain a competitive edge.
However, harnessing the full potential of data requires more than just collecting it; it requires expert analysis and interpretation.
This is where data scientists come in.
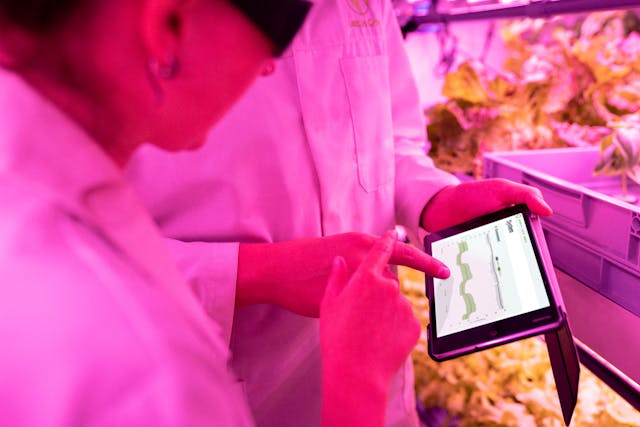
Data scientists are the modern-day alchemists, transforming raw data into valuable insights that can shape the future of a business.
Their unique blend of statistical knowledge, programming skills, and domain expertise makes them indispensable in the quest for data-driven success.
According to a report by the U.S. Bureau of Labor Statistics, the demand for data scientists is expected to grow by 31% over the next decade, highlighting the crucial role they play in today’s economy.
Despite their importance, hiring a data scientist can be a daunting task.
The role is relatively new and evolving, making it challenging for hiring managers to pinpoint exactly what they need.
Moreover, the competition for top talent is fierce, with companies across the globe vying for a limited pool of qualified professionals.
Understanding the nuances of the role and knowing where to find and how to attract the right candidates is essential for making a successful hire.
This comprehensive guide aims to demystify the process of hiring a data scientist.
Whether you are a seasoned HR professional or a business leader new to the field, this step-by-step guide will equip you with the knowledge and tools needed to make an informed hiring decision.
From defining the role and crafting the perfect job description to sourcing candidates and conducting effective interviews, we will walk you through each stage of the hiring process.
First, we’ll start by understanding the role of a data scientist in detail.
What exactly does a data scientist do, and what skills and qualifications should you look for?
We’ll then move on to defining your specific needs.
Every business is unique, and understanding your objectives and requirements is crucial in finding the right fit.
Next, we’ll guide you through crafting a job description that not only attracts top talent but also clearly communicates your expectations.
Sourcing candidates can be one of the most challenging parts of the process.
We’ll explore various avenues to find potential hires, from job boards and recruitment agencies to networking and university partnerships.
Once you have a pool of candidates, the screening and shortlisting phase begins.
We’ll provide tips on how to effectively evaluate resumes and conduct technical assessments to identify the most promising candidates.
Interviewing is a critical step where you get to delve deeper into a candidate’s technical abilities and cultural fit.
We’ll cover best practices for conducting both technical and behavioral interviews, as well as using case studies and practical tasks to assess real-world problem-solving skills.
After you’ve found the right candidate, making an attractive offer is key.
We’ll discuss strategies for competitive compensation and benefits, as well as negotiation tips to ensure a smooth hiring process.
Finally, we’ll address the onboarding and integration phase, ensuring your new hire has a smooth transition into your company.
From training and development to performance metrics and support systems, we’ll provide insights into creating a welcoming and productive environment for your new data scientist.
In conclusion, hiring a data scientist is a multifaceted process that requires careful planning and execution.
By following this step-by-step guide, you will be well-equipped to navigate the complexities of the hiring process and secure a talented data scientist who can drive your business forward.
Whether you are looking to build a data science team from scratch or expand your existing capabilities, this guide will serve as a valuable resource in your journey to harnessing the power of data.
Stay tuned as we delve into each of these steps in detail, starting with a deep dive into the role of a data scientist.
Let’s embark on this journey to make data-driven decisions and drive your business to new heights.
Before we venture further into this article, we like to share who we are and what we do.
About 9cv9
9cv9 is a business tech startup based in Singapore and Asia, with a strong presence all over the world.
With over eight years of startup and business experience, and being highly involved in connecting with thousands of companies and startups, the 9cv9 team has listed some important learning points in this overview of How to Hire a Data Scientist.
If your company needs recruitment and headhunting services to hire top-quality employees, you can use 9cv9 headhunting and recruitment services to hire top talents and candidates. Find out more here, or send over an email to hello@9cv9.com.
Or just post 1 free job posting here at 9cv9 Hiring Portal in under 10 minutes.
How to Hire a Data Scientist: A Step-By-Step Guide
- Understanding the Role of a Data Scientist
- Defining Your Needs
- Crafting the Perfect Job Description
- Sourcing Candidates
- Screening and Shortlisting Candidates
- Interviewing Candidates
- Making the Offer
- Onboarding and Integration
1. Understanding the Role of a Data Scientist
In this section, we will delve into the intricacies of the data scientist role, exploring their responsibilities, skills, and various specializations.
This comprehensive understanding will help you identify the right candidate for your business needs.
What is a Data Scientist?
- Definition:
- A data scientist is a professional who extracts insights from structured and unstructured data using scientific methods, processes, algorithms, and systems.
- They play a critical role in analyzing and interpreting complex data to help organizations make informed decisions.
- Evolution of the Role:
- Initially, data roles were limited to statisticians and data analysts.
- The advent of big data and advanced analytics has led to the emergence of the data scientist role, blending statistics, computer science, and domain expertise.
Key Responsibilities of a Data Scientist
- Data Collection and Cleaning:
- Example: Aggregating sales data from various sources to create a comprehensive dataset.
- Ensuring data accuracy and completeness through cleaning and preprocessing techniques.
- Data Exploration and Analysis:
- Example: Analyzing customer purchase patterns to identify trends and anomalies.
- Using statistical methods to explore data and uncover underlying patterns.
- Model Building and Evaluation:
- Example: Developing predictive models to forecast inventory requirements.
- Selecting appropriate machine learning algorithms and validating model performance.
- Data Visualization and Communication:
- Example: Creating dashboards to visualize key business metrics for stakeholders.
- Translating complex data findings into actionable insights and presenting them to non-technical audiences.
- Collaboration with Cross-Functional Teams:
- Example: Working with marketing teams to design data-driven campaigns.
- Partnering with IT, engineering, and business teams to implement data solutions.
Essential Skills and Qualifications
- Technical Skills:
- Programming Languages: Proficiency in Python, R, SQL, and Java.
- Example: Using Python for data manipulation and machine learning model development.
- Statistical Analysis: Strong foundation in statistical methods and concepts.
- Example: Applying regression analysis to predict customer churn.
- Machine Learning: Knowledge of supervised and unsupervised learning techniques.
- Example: Implementing clustering algorithms to segment customers based on behavior.
- Data Visualization Tools: Expertise in tools like Tableau, Power BI, and matplotlib.
- Example: Creating interactive dashboards in Tableau to monitor key performance indicators (KPIs).
- Big Data Technologies: Familiarity with Hadoop, Spark, and NoSQL databases.
- Example: Using Spark for distributed data processing and analysis.
- Programming Languages: Proficiency in Python, R, SQL, and Java.
- Soft Skills:
- Communication: Ability to convey complex data insights in a clear and concise manner.
- Problem-Solving: Strong analytical thinking and problem-solving capabilities.
- Collaboration: Effective teamwork and collaboration with cross-functional teams.
- Business Acumen: Understanding of the business context and ability to align data solutions with organizational goals.
- Educational Background:
- Degrees: Bachelor’s or Master’s degree in Computer Science, Statistics, Mathematics, or related fields.
- Certifications: Relevant certifications such as Certified Data Scientist, Google Professional Data Engineer, or Microsoft Certified: Azure Data Scientist Associate.
Different Types of Data Scientists
- Data Analysts:
- Focus on examining datasets to provide actionable insights.
- Example: Analyzing website traffic data to identify user behavior patterns.
- Machine Learning Engineers:
- Specialize in designing and implementing machine learning models.
- Example: Developing recommendation systems for e-commerce platforms.
- Data Engineers:
- Build and maintain the infrastructure required for data generation and storage.
- Example: Designing data pipelines to automate the ETL (Extract, Transform, Load) process.
- Business Intelligence (BI) Developers:
- Create and manage BI solutions to support data-driven decision-making.
- Example: Developing BI reports to monitor sales performance and identify growth opportunities.
- Research Scientists:
- Conduct advanced research to develop new algorithms and methodologies.
- Example: Innovating new deep learning techniques for image recognition.
Understanding the various specializations within the field of data science can help you pinpoint the exact type of data scientist you need. Whether your focus is on predictive analytics, big data processing, or business intelligence, having clarity on these roles ensures you find the right fit for your organization’s goals.
Real-World Examples
- Retail Industry:
- Amazon: Utilizes data scientists to develop recommendation algorithms that personalize the shopping experience for customers, significantly boosting sales and customer satisfaction.
- Healthcare Sector:
- IBM Watson: Leverages data science to analyze vast amounts of medical data and assist in diagnosing diseases and recommending treatment plans.
- Finance:
- JPMorgan Chase: Employs data scientists to develop fraud detection systems that analyze transaction patterns and flag suspicious activities in real-time.
- Tech Companies:
- Google: Uses data science to improve search algorithms, enhance ad targeting, and refine product features like Google Assistant.
By understanding the multifaceted role of a data scientist and the specific skills and responsibilities required, you can make a more informed decision when hiring.
This clarity ensures that you not only attract top talent but also align their expertise with your business objectives, driving growth and innovation through data-driven insights.
2. Defining Your Needs
Before you embark on the journey of hiring a data scientist, it’s crucial to clearly define your needs.
Understanding what your business requires will help you attract the right talent and ensure that your data science initiatives are aligned with your organizational goals.
In this section, we’ll explore how to assess your business requirements, set clear goals, and determine the necessary skills and experience for your ideal candidate.
Assessing Your Business Requirements
- Identify Key Business Areas:
- Determine which areas of your business will benefit the most from data science.
- Example: In retail, focus areas might include inventory management, customer segmentation, and sales forecasting.
- Prioritize business functions that can leverage data for improved decision-making.
- Determine which areas of your business will benefit the most from data science.
- Understand Current Challenges:
- Pinpoint specific problems or challenges that data science can address.
- Example: A healthcare provider may need data science to predict patient readmission rates and improve care management.
- Evaluate how data-driven insights can solve these issues.
- Pinpoint specific problems or challenges that data science can address.
- Data Availability and Quality:
- Assess the availability and quality of your existing data.
- Example: Ensure that you have access to clean, structured data from various sources such as CRM systems, web analytics, and sales databases.
- Identify gaps in your data collection processes that need to be addressed.
- Assess the availability and quality of your existing data.
- Evaluate Technological Infrastructure:
- Review your current technology stack and its compatibility with data science tools.
- Example: Check if your existing systems can integrate with popular data science platforms like Hadoop, Spark, or cloud-based solutions like AWS and Google Cloud.
- Plan for any necessary upgrades or investments in technology.
- Review your current technology stack and its compatibility with data science tools.
Setting Clear Goals and Objectives
- Define Business Objectives:
- Establish clear and measurable business objectives that data science can help achieve.
- Example: Aim to increase customer retention by 20% over the next year through personalized marketing campaigns.
- Align data science goals with overall business strategy and KPIs.
- Establish clear and measurable business objectives that data science can help achieve.
- Short-Term vs. Long-Term Goals:
- Differentiate between short-term and long-term goals to guide your data science initiatives.
- Short-Term Goals: Immediate improvements such as optimizing marketing campaigns or enhancing product recommendations.
- Long-Term Goals: Strategic initiatives like developing predictive models for long-term sales forecasting or implementing AI-driven automation.
- Differentiate between short-term and long-term goals to guide your data science initiatives.
- Quantify Expected Outcomes:
- Set specific, quantifiable targets for your data science projects.
- Example: Reduce operational costs by 15% through data-driven process optimization within the next six months.
- Use these targets to measure the success of your data science initiatives.
- Set specific, quantifiable targets for your data science projects.
Determining Required Skills and Experience
- Technical Skills:
- Programming and Scripting Languages:
- Proficiency in Python, R, SQL, and Java.
- Example: Require Python for data manipulation and machine learning, SQL for database management, and R for statistical analysis.
- Proficiency in Python, R, SQL, and Java.
- Machine Learning and AI:
- Knowledge of machine learning algorithms and AI techniques.
- Example: Implement supervised learning algorithms for classification tasks and unsupervised learning for clustering customer segments.
- Knowledge of machine learning algorithms and AI techniques.
- Data Visualization:
- Expertise in tools like Tableau, Power BI, and matplotlib.
- Example: Create dashboards to visualize sales performance and customer behavior.
- Expertise in tools like Tableau, Power BI, and matplotlib.
- Big Data Technologies:
- Familiarity with Hadoop, Spark, and NoSQL databases.
- Example: Use Spark for processing large datasets and Hadoop for distributed storage and computation.
- Familiarity with Hadoop, Spark, and NoSQL databases.
- Programming and Scripting Languages:
- Domain-Specific Knowledge:
- Look for candidates with experience in your industry or a similar domain.
- Example: In the finance industry, prioritize candidates with experience in risk assessment, fraud detection, or financial modeling.
- Ensure they understand the specific challenges and opportunities within your sector.
- Look for candidates with experience in your industry or a similar domain.
- Soft Skills:
- Communication and Collaboration:
- Ability to convey complex data insights in a clear, actionable manner.
- Example: Present findings to non-technical stakeholders and collaborate with marketing, sales, and IT teams.
- Ability to convey complex data insights in a clear, actionable manner.
- Problem-Solving and Critical Thinking:
- Strong analytical thinking to address business challenges.
- Example: Identify root causes of customer churn and develop strategies to improve retention.
- Strong analytical thinking to address business challenges.
- Project Management:
- Experience managing data science projects from inception to completion.
- Example: Oversee the development and deployment of predictive models, ensuring they meet business objectives.
- Experience managing data science projects from inception to completion.
- Communication and Collaboration:
Matching Skills to Business Needs
- Role Specializations:
- Match the required skills and experience to the specific role you need.
- Example: For a role focused on predictive analytics, prioritize candidates with strong machine learning and statistical skills.
- Understand the different types of data scientists (e.g., data analysts, machine learning engineers) and their unique contributions.
- Match the required skills and experience to the specific role you need.
- Prioritize Critical Skills:
- Identify the most critical skills for your business and prioritize them in your hiring criteria.
- Example: If real-time data processing is crucial, prioritize expertise in big data technologies like Spark and Kafka.
- Ensure that the candidate’s skill set aligns with your top business priorities.
- Identify the most critical skills for your business and prioritize them in your hiring criteria.
Real-World Examples
- E-commerce:
- Amazon: Uses data science to optimize supply chain management, improve delivery times, and enhance customer recommendations.
- Objective: Increase operational efficiency and customer satisfaction.
- Healthcare:
- UnitedHealth Group: Leverages data science to predict patient outcomes, optimize treatment plans, and reduce healthcare costs.
- Objective: Improve patient care and reduce readmission rates.
- Financial Services:
- Goldman Sachs: Employs data scientists to develop trading algorithms, assess credit risk, and detect fraudulent activities.
- Objective: Enhance trading strategies and mitigate financial risks.
By clearly defining your business needs, setting measurable goals, and identifying the required skills and experience, you can streamline the hiring process and ensure that you find the right data scientist for your organization.
This clarity not only helps attract top talent but also ensures that your data science initiatives are aligned with your strategic objectives, driving meaningful business outcomes.
3. Crafting the Perfect Job Description
A well-crafted job description is essential to attract top talent for your data science position.
It not only defines the role and its responsibilities but also sets the expectations for potential candidates.
In this section, we’ll explore how to create a compelling job description that stands out to highly qualified data scientists.
Also, read more on our top guide on how to create the best job descriptions.
Job Title and Overview
- Clear and Concise Title:
- Ensure the job title is clear and accurately reflects the role.
- Example: Use “Senior Data Scientist” instead of vague titles like “Data Wizard” or “Data Guru.”
- Include any relevant specializations.
- Example: “Machine Learning Data Scientist” or “Data Scientist – Natural Language Processing (NLP).”
- Ensure the job title is clear and accurately reflects the role.
- Brief Overview:
- Provide a snapshot of the role in 2-3 sentences.
- Example: “We are seeking a Senior Data Scientist to join our analytics team. The ideal candidate will have a strong background in machine learning, data analysis, and statistical modeling to drive data-driven decisions and solutions for our business.”
- Provide a snapshot of the role in 2-3 sentences.
Detailed Responsibilities
- Data Collection and Processing:
- Oversee the acquisition, cleaning, and preprocessing of data from various sources.
- Example: “Collect, clean, and preprocess data from internal databases and external sources to ensure data quality and accuracy.”
- Oversee the acquisition, cleaning, and preprocessing of data from various sources.
- Exploratory Data Analysis:
- Perform exploratory data analysis to uncover patterns and insights.
- Example: “Conduct exploratory data analysis to identify trends, anomalies, and correlations within large datasets.”
- Perform exploratory data analysis to uncover patterns and insights.
- Model Development and Implementation:
- Develop, validate, and deploy predictive models and machine learning algorithms.
- Example: “Design and implement predictive models and machine learning algorithms to solve complex business problems.”
- Develop, validate, and deploy predictive models and machine learning algorithms.
- Data Visualization and Reporting:
- Create visualizations and reports to communicate findings to stakeholders.
- Example: “Develop dashboards and reports using tools like Tableau or Power BI to present data insights to non-technical stakeholders.”
- Create visualizations and reports to communicate findings to stakeholders.
- Collaboration with Cross-Functional Teams:
- Work closely with other departments to integrate data solutions.
- Example: “Collaborate with marketing, sales, and IT teams to implement data-driven solutions and strategies.”
- Work closely with other departments to integrate data solutions.
- Staying Updated with Industry Trends:
- Keep abreast of the latest developments in data science and machine learning.
- Example: “Stay current with advancements in data science, machine learning, and AI to continuously enhance our data capabilities.”
- Keep abreast of the latest developments in data science and machine learning.
Required Skills and Qualifications
- Educational Background:
- Specify the necessary educational qualifications.
- Example: “Bachelor’s or Master’s degree in Computer Science, Statistics, Mathematics, or a related field. PhD preferred.”
- Specify the necessary educational qualifications.
- Technical Skills:
- Programming Languages: Proficiency in Python, R, SQL, and other relevant languages.
- Example: “Proficient in Python and R for data analysis and machine learning.”
- Machine Learning and AI: Experience with machine learning frameworks and libraries.
- Example: “Hands-on experience with machine learning frameworks like TensorFlow, PyTorch, and Scikit-Learn.”
- Data Visualization: Expertise in data visualization tools.
- Example: “Skilled in using data visualization tools such as Tableau, Power BI, and matplotlib.”
- Big Data Technologies: Familiarity with big data platforms.
- Example: “Experience with big data technologies like Hadoop, Spark, and NoSQL databases.”
- Programming Languages: Proficiency in Python, R, SQL, and other relevant languages.
- Experience:
- Outline the required years of experience and relevant fields.
- Example: “5+ years of experience in data science, with a focus on machine learning and statistical modeling.”
- Outline the required years of experience and relevant fields.
- Soft Skills:
- Communication: Ability to explain complex concepts to non-technical audiences.
- Example: “Excellent communication skills to present data insights clearly to stakeholders.”
- Problem-Solving: Strong analytical and problem-solving abilities.
- Example: “Proven problem-solving skills to tackle complex business challenges using data-driven approaches.”
- Collaboration: Ability to work effectively in cross-functional teams.
- Example: “Demonstrated ability to collaborate with diverse teams to achieve business objectives.”
- Communication: Ability to explain complex concepts to non-technical audiences.
Company Overview
- Company Description:
- Provide a brief overview of your company, its mission, and its values.
- Example: “XYZ Corp is a leading e-commerce company dedicated to providing exceptional online shopping experiences. We value innovation, customer satisfaction, and continuous improvement.”
- Provide a brief overview of your company, its mission, and its values.
- Company Culture:
- Highlight your company culture and work environment.
- Example: “Our collaborative and inclusive work environment encourages creativity and fosters professional growth. We believe in empowering our employees to drive positive change.”
- Highlight your company culture and work environment.
- Unique Selling Points:
- Mention any unique benefits or perks your company offers.
- Example: “We offer competitive salaries, comprehensive health benefits, flexible work arrangements, and opportunities for continuous learning and development.”
- Mention any unique benefits or perks your company offers.
Application Process
- How to Apply:
- Provide clear instructions on how to apply for the position.
- Example: “To apply, please submit your resume, cover letter, and any relevant project portfolios to careers@xyzcorp.com.”
- Provide clear instructions on how to apply for the position.
- Application Deadline:
- Specify any deadlines for application submissions.
- Example: “Applications are accepted until August 31, 2024.”
- Specify any deadlines for application submissions.
- Selection Process:
- Outline the stages of the selection process.
- Example: “Our selection process includes an initial resume screening, a technical assessment, and interviews with the hiring manager and team members.”
- Outline the stages of the selection process.
Example Job Description
Job Title: Senior Data Scientist – Machine Learning
Overview: We are seeking a Senior Data Scientist to join our analytics team. The ideal candidate will have a strong background in machine learning, data analysis, and statistical modeling to drive data-driven decisions and solutions for our business.
Responsibilities:
- Collect, clean, and preprocess data from internal databases and external sources to ensure data quality and accuracy.
- Conduct exploratory data analysis to identify trends, anomalies, and correlations within large datasets.
- Design and implement predictive models and machine learning algorithms to solve complex business problems.
- Develop dashboards and reports using tools like Tableau or Power BI to present data insights to non-technical stakeholders.
- Collaborate with marketing, sales, and IT teams to implement data-driven solutions and strategies.
- Stay current with advancements in data science, machine learning, and AI to continuously enhance our data capabilities.
Qualifications:
- Bachelor’s or Master’s degree in Computer Science, Statistics, Mathematics, or a related field. PhD preferred.
- Proficient in Python and R for data analysis and machine learning.
- Hands-on experience with machine learning frameworks like TensorFlow, PyTorch, and Scikit-Learn.
- Skilled in using data visualization tools such as Tableau, Power BI, and matplotlib.
- Experience with big data technologies like Hadoop, Spark, and NoSQL databases.
- 5+ years of experience in data science, with a focus on machine learning and statistical modeling.
- Excellent communication skills to present data insights clearly to stakeholders.
- Proven problem-solving skills to tackle complex business challenges using data-driven approaches.
- Demonstrated ability to collaborate with diverse teams to achieve business objectives.
Company Overview: XYZ Corp is a leading e-commerce company dedicated to providing exceptional online shopping experiences. We value innovation, customer satisfaction, and continuous improvement. Our collaborative and inclusive work environment encourages creativity and fosters professional growth. We believe in empowering our employees to drive positive change.
How to Apply: To apply, please submit your resume, cover letter, and any relevant project portfolios to careers@xyzcorp.com. Applications are accepted until August 31, 2024.
Also, read our in-depth guide on creating the best data scientist job description here: Lead Data Scientist Job Description: A Complete Guide for Hiring Managers to Use.
By crafting a detailed and compelling job description, you set clear expectations for potential candidates and attract highly qualified data scientists who are well-suited to meet your business needs.
A well-defined job description not only helps in attracting the right talent but also ensures that candidates have a clear understanding of their roles and responsibilities, leading to a more efficient hiring process and successful integration into your organization.
4. Sourcing Candidates
Finding the right data scientist requires a strategic approach to sourcing candidates.
With the demand for data science talent on the rise, it’s essential to utilize a variety of channels and techniques to attract the best candidates.
In this section, we’ll explore effective strategies for sourcing data scientists, including leveraging online platforms, networking, and working with recruitment agencies.
Online Job Portals and Platforms
- Popular Job Boards:
- 9cv9: Leverage 9cv9’s vast network to post job openings and search for potential candidates.
- Example: Use 9cv9 advanced search filters to find data scientists with specific skills and experience.
- Indeed: Post your job description on Indeed to reach a large audience.
- Example: Utilize Indeed’s resume database to search for qualified candidates actively looking for new opportunities.
- Glassdoor: Post job listings and take advantage of company reviews to attract top talent.
- Example: Highlight positive company reviews and employee testimonials to build your employer brand.
- 9cv9: Leverage 9cv9’s vast network to post job openings and search for potential candidates.
- Specialized Job Boards:
- 9cv9: A platform for data scientists
- Example: Post job listings and reach out to top-performing competitors in 9cv9.
- AngelList: Focuses on startups and tech roles, including data science.
- Example: Post job openings to attract candidates interested in working in dynamic startup environments.
- DataJobs: A niche job board specifically for data science and analytics roles.
- Example: Post detailed job descriptions to target candidates with specialized data science skills.
- 9cv9: A platform for data scientists
University and Educational Partnerships
- University Recruitment Programs:
- Partner with universities known for strong data science programs.
- Example: Collaborate with institutions like Stanford, MIT, and Carnegie Mellon to participate in career fairs and recruitment events.
- Offer internships and co-op programs to students.
- Example: Provide opportunities for students to gain practical experience while you evaluate their potential for full-time roles.
- Partner with universities known for strong data science programs.
- Guest Lectures and Workshops:
- Conduct guest lectures or workshops at universities to engage with students.
- Example: Host a workshop on machine learning applications in your industry to attract and identify promising candidates.
- Conduct guest lectures or workshops at universities to engage with students.
- Capstone Projects and Competitions:
- Sponsor capstone projects or data science competitions at universities.
- Example: Partner with universities to create real-world data challenges, offering winners potential job opportunities.
- Sponsor capstone projects or data science competitions at universities.
Networking and Professional Communities
- Data Science Meetups and Conferences:
- Attend and participate in data science meetups and conferences.
- Example: Network with data science professionals at events like Strata Data Conference, PyData, and local meetups.
- Sponsor or host events to showcase your company’s data initiatives.
- Example: Organize a data science hackathon to attract and engage with potential candidates.
- Attend and participate in data science meetups and conferences.
- Online Communities and Forums:
- Engage with online communities such as GitHub, Stack Overflow, and Reddit.
- Example: Participate in discussions, share your company’s projects, and identify active contributors as potential candidates.
- Join specialized forums and groups focused on data science and machine learning.
- Example: Participate in discussions on platforms like Data Science Central and Towards Data Science.
- Engage with online communities such as GitHub, Stack Overflow, and Reddit.
Leveraging Social Media
- Social Media Recruitment:
- Use social media platforms like Twitter, Facebook, and Instagram to post job openings and company updates.
- Example: Share job listings and behind-the-scenes content to attract candidates interested in your company culture.
- Engage with data science communities on these platforms.
- Example: Follow and interact with influential data scientists and participate in relevant conversations.
- Use social media platforms like Twitter, Facebook, and Instagram to post job openings and company updates.
- Employee Advocacy:
- Encourage your current employees to share job openings on their social media profiles.
- Example: Implement an employee referral program to incentivize sharing job posts and referring candidates.
- Highlight employee testimonials and success stories to showcase your company culture.
- Example: Share stories of your data science team’s achievements and career growth on social media.
- Encourage your current employees to share job openings on their social media profiles.
Working with Recruitment Agencies
- Specialized Recruitment Agencies:
- Partner with recruitment agencies that specialize in data science and technology roles.
- Example: Agencies like 9cv9 have expertise in sourcing data science talent.
- Provide detailed job descriptions and candidate profiles to ensure the agency understands your needs.
- Example: Work closely with the agency to refine the search criteria and identify the best candidates.
- Partner with recruitment agencies that specialize in data science and technology roles.
- Contingency vs. Retained Search Firms:
- Decide between contingency firms (paid upon successful hire) and retained search firms (paid upfront for a dedicated search).
- Example: Use retained search firms for executive-level data science roles where a thorough and dedicated search is necessary.
- Decide between contingency firms (paid upon successful hire) and retained search firms (paid upfront for a dedicated search).
- Contract and Freelance Talent:
- Consider hiring contract or freelance data scientists for short-term projects.
- Example: Use platforms like Upwork and Toptal to find highly skilled freelance data scientists for specific tasks.
- Evaluate their work for potential long-term employment opportunities.
- Example: Offer contract-to-hire positions to assess candidates’ fit before committing to a full-time role.
- Consider hiring contract or freelance data scientists for short-term projects.
Using 9cv9 Recruitment Agency to Hire Top Data Scientists
9cv9 Recruitment Agency stands out as a strategic partner for organizations seeking to hire top-tier data scientists.
Specializing in tech recruitment, 9cv9 brings a wealth of expertise and a robust network within the data science community.
Here’s why partnering with 9cv9 can streamline your hiring process and attract top data science talent:
Expertise in Data Science Recruitment
With a deep understanding of the data science landscape, 9cv9 Recruitment Agency employs specialized recruiters who are well-versed in the skills, qualifications, and market demands specific to data scientists. They can effectively identify and evaluate candidates with the right blend of technical prowess and industry experience.
Access to a Wide Talent Pool
9cv9 Recruitment Agency maintains an extensive network of data science professionals, including passive candidates who may not be actively searching but are open to exceptional career opportunities. This broad reach ensures access to a diverse pool of talent, increasing the chances of finding the perfect fit for your organization.
Tailored Recruitment Strategies
Understanding that each organization has unique hiring needs, 9cv9 Recruitment Agency tailors its recruitment strategies accordingly. Whether you require candidates with expertise in machine learning, artificial intelligence, big data analytics, or specific industry knowledge, they customize their approach to align with your requirements.
Rigorous Screening and Selection Process
9cv9 employs a rigorous screening process to assess candidates thoroughly. This includes technical assessments, behavioral interviews, and evaluating cultural fit to ensure candidates not only meet but exceed your expectations. They present you with highly qualified candidates who are well-prepared for the challenges of your role.
Expedited Time-to-Hire
By leveraging their expertise and network, 9cv9 Recruitment Agency accelerates the hiring process. They streamline candidate sourcing, screening, and interviewing, reducing time-to-hire while maintaining quality standards. This efficiency is crucial in securing top data science talent before competitors.
Comprehensive Support Throughout the Hiring Journey
From initial consultation to post-placement follow-up, 9cv9 Recruitment Agency provides comprehensive support. They guide you through job profiling, market analysis, offer negotiation, and onboarding, ensuring a seamless experience for both the employer and the candidate.
Industry Insights and Market Trends
Staying ahead in data science recruitment requires staying informed about industry trends and market dynamics. 9cv9 Recruitment Agency offers valuable insights into emerging technologies, salary benchmarks, and competitor analysis, enabling informed decision-making throughout the hiring process.
Commitment to Diversity and Inclusion
Diversity is crucial for innovation and success in today’s workforce. 9cv9 Recruitment Agency prioritizes diversity and inclusion in their candidate sourcing and selection process. They strive to present a diverse slate of candidates, promoting an inclusive workplace culture for your organization.
Long-term Partnership and Success
Partnering with 9cv9 Recruitment Agency isn’t just about filling a position; it’s about building a long-term partnership for success.
They focus on understanding your organizational goals and aligning recruitment strategies to support your growth and development in data science capabilities.
In summary, 9cv9 Recruitment Agency offers specialized expertise, a vast talent network, and tailored recruitment strategies to help organizations hire top data scientists effectively and efficiently.
Whether you’re scaling your data science team or looking to fill a critical role, partnering with 9cv9 can lead to finding the ideal candidate who will drive innovation and business success in data-driven decision-making.
Utilizing Your Company’s Talent Pool
- Internal Talent Development:
- Identify current employees with potential for data science roles and provide training and development opportunities.
- Example: Offer data science courses and workshops to upskill employees in related roles, such as data analysts or software engineers.
- Encourage employees to pursue certifications in data science.
- Example: Support employees in obtaining certifications like the Certified Data Scientist (CDS) or Google Professional Data Engineer.
- Identify current employees with potential for data science roles and provide training and development opportunities.
- Employee Referrals:
- Implement an employee referral program to leverage your employees’ networks.
- Example: Offer bonuses or other incentives for employees who refer successful candidates for data science positions.
- Promote open positions internally and encourage employees to share them with their connections.
- Example: Regularly update employees on open roles and encourage them to refer qualified candidates.
- Implement an employee referral program to leverage your employees’ networks.
Engaging Passive Candidates
- Proactive Outreach:
- Identify and reach out to passive candidates who may not be actively looking for a new job.
- Example: Use LinkedIn Recruiter to search for profiles that match your criteria and send personalized messages.
- Highlight your company’s unique selling points and the opportunities available.
- Example: Emphasize career growth, innovative projects, and a positive work culture in your outreach messages.
- Identify and reach out to passive candidates who may not be actively looking for a new job.
- Talent Pipelines:
- Build and maintain a talent pipeline for future hiring needs.
- Example: Keep a database of potential candidates who have shown interest in your company or attended your events.
- Engage with these candidates periodically to keep them informed about new opportunities.
- Example: Send newsletters or updates about your company’s data science initiatives and open positions.
- Build and maintain a talent pipeline for future hiring needs.
By employing a diverse range of sourcing strategies, you can broaden your reach and attract highly qualified data scientists.
Combining online platforms, university partnerships, networking, and recruitment agencies ensures a comprehensive approach to finding the right candidates for your organization.
This multifaceted strategy not only increases your chances of attracting top talent but also helps build a robust talent pipeline for future hiring needs.
5. Screening and Shortlisting Candidates
Screening and shortlisting candidates is a crucial step in the hiring process for data scientists.
This phase involves evaluating applicants to ensure they meet the required qualifications and skills, and identifying the best candidates to move forward to the interview stage.
A thorough and strategic approach to screening can save time and resources, and ensure you find the right fit for your organization.
In this section, we’ll discuss effective methods for screening resumes, conducting initial assessments, and creating a shortlist of top candidates.
Initial Resume Screening
- Keyword Matching:
- Use applicant tracking systems (ATS) to filter resumes based on relevant keywords.
- Example: Look for keywords such as “machine learning,” “Python,” “data analysis,” and “predictive modeling.”
- Ensure that candidates have mentioned key technical skills and qualifications in their resumes.
- Use applicant tracking systems (ATS) to filter resumes based on relevant keywords.
- Educational Background:
- Check for relevant educational qualifications, such as degrees in Computer Science, Statistics, Mathematics, or related fields.
- Example: Prioritize candidates with a Bachelor’s, Master’s, or PhD in data-intensive disciplines.
- Look for additional certifications in data science or machine learning.
- Example: Certifications like Certified Data Scientist (CDS) or Google Professional Data Engineer can be strong indicators of expertise.
- Check for relevant educational qualifications, such as degrees in Computer Science, Statistics, Mathematics, or related fields.
- Professional Experience:
- Evaluate the candidate’s professional experience in data science roles.
- Example: Consider the number of years of experience and relevance to your industry.
- Look for experience in specific domains relevant to your business.
- Example: For a healthcare company, prioritize candidates with experience in health data analytics or bioinformatics.
- Evaluate the candidate’s professional experience in data science roles.
- Project Experience:
- Assess the candidate’s project experience and the impact of their work.
- Example: Look for details on specific projects, such as developing a predictive model for customer churn or implementing a recommendation system.
- Check for experience with relevant tools and technologies.
- Example: Ensure they have worked with tools like TensorFlow, PyTorch, Hadoop, and Spark.
- Assess the candidate’s project experience and the impact of their work.
Technical Skills Assessment
- Online Assessments and Coding Challenges:
- Use online platforms to administer coding challenges and technical assessments.
- Example: Platforms like HackerRank, Codility, and LeetCode can be used to evaluate coding skills and problem-solving abilities.
- Focus on challenges that test relevant skills, such as data manipulation, algorithm development, and machine learning.
- Use online platforms to administer coding challenges and technical assessments.
- Data Science Competitions:
- Consider candidates’ performance in data science competitions.
- Example: Review their rankings and solutions in competitions on platforms like Kaggle.
- Evaluate their ability to solve real-world data problems and innovate.
- Consider candidates’ performance in data science competitions.
- Technical Screening Interviews:
- Conduct initial technical screening interviews to assess core competencies.
- Example: Use phone or video interviews to discuss candidates’ technical skills, project experience, and problem-solving approaches.
- Ask questions that test their understanding of data science concepts and methodologies.
- Example: Inquire about their approach to feature engineering, model validation, and handling imbalanced datasets.
- Conduct initial technical screening interviews to assess core competencies.
Soft Skills and Cultural Fit
- Communication Skills:
- Evaluate the candidate’s ability to explain complex technical concepts in simple terms.
- Example: Ask them to describe a technical project to a non-technical audience.
- Assess their written communication skills through their resume and cover letter.
- Evaluate the candidate’s ability to explain complex technical concepts in simple terms.
- Collaboration and Teamwork:
- Look for evidence of effective collaboration with cross-functional teams.
- Example: Ask about their experience working with marketing, sales, or product teams to implement data-driven solutions.
- Evaluate their ability to work in a team-oriented environment.
- Look for evidence of effective collaboration with cross-functional teams.
- Problem-Solving and Critical Thinking:
- Assess their problem-solving skills through scenario-based questions.
- Example: Present a business problem and ask them to outline their approach to solving it using data science techniques.
- Look for examples of innovative solutions they have implemented in past roles.
- Assess their problem-solving skills through scenario-based questions.
- Adaptability and Continuous Learning:
- Evaluate their willingness to learn and adapt to new technologies and methodologies.
- Example: Ask about recent courses, certifications, or projects they have undertaken to stay current in the field.
- Look for a growth mindset and enthusiasm for continuous improvement.
- Evaluate their willingness to learn and adapt to new technologies and methodologies.
Creating a Shortlist
- Scorecard Method:
- Use a scorecard to rate candidates based on predefined criteria.
- Example: Assign scores for technical skills, project experience, communication skills, and cultural fit.
- Ensure consistency in evaluating all candidates.
- Use a scorecard to rate candidates based on predefined criteria.
- Ranking and Prioritization:
- Rank candidates based on their total scores and overall fit for the role.
- Example: Prioritize candidates who excel in key areas and align well with your company’s needs and culture.
- Create a shortlist of the top candidates to move forward to the next stage.
- Rank candidates based on their total scores and overall fit for the role.
- Diversity and Inclusion:
- Consider diversity and inclusion in your shortlisting process.
- Example: Ensure a diverse pool of candidates in terms of gender, ethnicity, and background.
- Strive for a balanced and inclusive team.
- Consider diversity and inclusion in your shortlisting process.
Tools and Platforms for Screening
- Applicant Tracking Systems (ATS):
- Greenhouse: An ATS that helps manage the recruitment process, from job posting to candidate evaluation.
- Lever: Provides tools for sourcing, managing, and nurturing candidates throughout the hiring process.
- Technical Assessment Platforms:
- HackerRank: Offers coding challenges and technical assessments for evaluating data science skills.
- Codility: Provides a platform for coding tests and technical interviews.
- Interviewing Platforms:
- HireVue: Uses video interviewing technology to conduct and record interviews for later review.
- Spark Hire: Allows for one-way video interviews, where candidates record responses to predefined questions.
By implementing a structured and thorough approach to screening and shortlisting candidates, you can efficiently identify the most qualified and suitable individuals for your data science roles.
This process not only ensures that you select candidates with the right technical skills and experience but also evaluates their soft skills and cultural fit, leading to successful hires who can contribute effectively to your organization.
6. Interviewing Candidates
Interviewing candidates is a pivotal stage in the hiring process for data scientists.
This step involves a deeper assessment of the candidates’ technical skills, problem-solving abilities, cultural fit, and overall suitability for the role.
Conducting effective interviews requires a structured approach, clear objectives, and well-prepared interviewers.
In this section, we’ll explore best practices for interviewing data science candidates, including types of interviews, key areas to assess, and example questions.
Types of Interviews
- Phone Screenings:
- Purpose: To conduct an initial assessment of the candidate’s background and communication skills.
- Duration: Typically lasts 20-30 minutes.
- Focus Areas:
- Brief overview of the candidate’s resume and experience.
- Initial discussion of technical skills and relevant projects.
- Evaluation of communication skills and cultural fit.
- Example Question: “Can you give us a brief overview of your most recent data science project and your role in it?”
- Technical Interviews:
- Purpose: To evaluate the candidate’s technical competencies in depth.
- Duration: Usually 1-2 hours, can be divided into multiple sessions.
- Focus Areas:
- Coding skills and proficiency in programming languages like Python or R.
- Understanding of machine learning algorithms and data processing techniques.
- Practical problem-solving using real-world scenarios.
- Example Question: “Given a dataset with missing values, how would you approach cleaning and preprocessing the data for analysis?”
- Behavioral Interviews:
- Purpose: To assess the candidate’s soft skills, cultural fit, and alignment with company values.
- Duration: Typically 30-45 minutes.
- Focus Areas:
- Communication, teamwork, and leadership abilities.
- Adaptability, resilience, and continuous learning.
- Example Question: “Describe a time when you had to explain a complex technical concept to a non-technical stakeholder. How did you ensure they understood it?”
- Case Study Interviews:
- Purpose: To evaluate the candidate’s analytical and problem-solving skills through practical scenarios.
- Duration: Usually 1-2 hours, depending on the complexity of the case.
- Focus Areas:
- Data analysis and interpretation.
- Model development and validation.
- Presentation of findings and recommendations.
- Example Question: “Here is a dataset related to customer churn. Can you develop a model to predict churn and explain your approach and results?”
- Panel Interviews:
- Purpose: To get a holistic view of the candidate by involving multiple interviewers from different departments.
- Duration: Typically 1-2 hours.
- Focus Areas:
- Comprehensive assessment of technical, analytical, and interpersonal skills.
- Evaluation from multiple perspectives to ensure a well-rounded decision.
- Example Question: “Can you walk us through a project where you had to collaborate with cross-functional teams to achieve a common goal?”
Key Areas to Assess
- Technical Proficiency:
- Programming Skills: Assess proficiency in languages like Python, R, SQL, and familiarity with big data technologies like Hadoop and Spark.
- Example Question: “How do you optimize your Python code for performance in data-intensive tasks?”
- Machine Learning Knowledge: Evaluate understanding of algorithms, model building, and validation techniques.
- Example Question: “Can you explain the difference between supervised and unsupervised learning, and provide examples of each?”
- Data Manipulation and Analysis: Check for skills in data cleaning, preprocessing, and exploratory data analysis.
- Example Question: “How do you handle outliers in your dataset? Can you provide a specific example?”
- Programming Skills: Assess proficiency in languages like Python, R, SQL, and familiarity with big data technologies like Hadoop and Spark.
- Problem-Solving Abilities:
- Analytical Thinking: Assess the candidate’s approach to breaking down complex problems and developing solutions.
- Example Question: “Given a sudden drop in user engagement on our platform, how would you investigate and identify the root cause?”
- Creativity and Innovation: Evaluate their ability to come up with novel solutions to challenging problems.
- Example Question: “Can you describe a time when you used an unconventional method to solve a data science problem?”
- Practical Application: Check their ability to apply theoretical knowledge to real-world scenarios.
- Example Question: “How would you approach building a recommendation system for an e-commerce platform?”
- Analytical Thinking: Assess the candidate’s approach to breaking down complex problems and developing solutions.
- Communication Skills:
- Clarity and Articulation: Assess the candidate’s ability to communicate complex ideas clearly and concisely.
- Example Question: “How do you ensure that your technical reports are understandable to non-technical stakeholders?”
- Presentation Skills: Evaluate their ability to present data insights and recommendations effectively.
- Example Question: “Can you present a recent project where you had to deliver your findings to a diverse audience?”
- Listening Skills: Check their ability to understand and respond to questions and feedback.
- Example Question: “How do you handle feedback on your analysis or recommendations from colleagues or clients?”
- Clarity and Articulation: Assess the candidate’s ability to communicate complex ideas clearly and concisely.
- Cultural Fit and Soft Skills:
- Teamwork and Collaboration: Assess their experience and comfort level working in team environments.
- Example Question: “Can you describe a time when you had to collaborate with a difficult team member? How did you handle it?”
- Adaptability and Learning: Evaluate their willingness and ability to adapt to new technologies and methodologies.
- Example Question: “How do you stay current with the latest developments in data science and machine learning?”
- Leadership and Initiative: Check for leadership qualities and the ability to take initiative on projects.
- Example Question: “Can you provide an example of a time when you led a project or took initiative to solve a problem?”
- Teamwork and Collaboration: Assess their experience and comfort level working in team environments.
Structuring the Interview Process
- Preparation:
- Develop a clear interview plan and criteria for assessment.
- Example: Create a detailed interview guide with specific questions and evaluation metrics.
- Ensure all interviewers are aligned on the objectives and criteria.
- Example: Conduct a pre-interview briefing to discuss the candidate’s resume and key areas to focus on.
- Develop a clear interview plan and criteria for assessment.
- Conducting the Interview:
- Start with an introduction and brief overview of the interview process.
- Example: “Thank you for joining us today. We’ll start with some questions about your background and experience, followed by technical and behavioral questions.”
- Ask a mix of open-ended and specific questions to get a comprehensive view of the candidate.
- Example: “Can you walk us through a challenging data science project you’ve worked on recently? What were the key challenges and how did you overcome them?”
- Take notes and use a scorecard to rate the candidate on different criteria.
- Example: Use a standardized rating scale to evaluate technical skills, problem-solving abilities, communication, and cultural fit.
- Start with an introduction and brief overview of the interview process.
- Post-Interview Evaluation:
- Hold a debrief session with all interviewers to discuss their impressions and ratings.
- Example: Share notes and scores, and discuss any discrepancies or concerns.
- Compare candidates based on their overall performance and fit for the role.
- Example: Use the scorecards to create a ranking and make a decision on who to move forward in the process.
- Hold a debrief session with all interviewers to discuss their impressions and ratings.
Example Questions and Scenarios
- Technical Questions:
- “How do you handle missing data in a dataset? What techniques do you use to impute missing values?”
- “Explain the bias-variance tradeoff and how you balance it when building machine learning models.”
- “Describe a situation where you had to optimize a machine learning model. What steps did you take and what was the outcome?”
- Behavioral Questions:
- “Describe a time when you had to work under a tight deadline. How did you manage your time and resources to meet the deadline?”
- “Tell us about a project where you faced significant data quality issues. How did you address these challenges?”
- “Can you provide an example of a time when you had to persuade a stakeholder to adopt a data-driven approach? How did you approach the situation?”
- Case Study Scenarios:
- “You are given a dataset of customer transactions. How would you identify and segment the most valuable customers for a targeted marketing campaign?”
- “Imagine you are working for a healthcare company. How would you develop a predictive model to identify patients at risk of readmission?”
- “You have been asked to improve the accuracy of a recommendation system for an online retailer. What steps would you take to achieve this goal?”
By adopting a structured and thorough approach to interviewing candidates, you can effectively evaluate their technical skills, problem-solving abilities, and cultural fit.
This ensures that you select the best candidates for your data science roles, leading to successful hires who can contribute significantly to your organization’s data-driven initiatives.
7. Making the Offer
Making the offer is a critical step in the hiring process for data scientists.
It’s essential to present a compelling offer that not only attracts top talent but also aligns with the candidate’s career goals and expectations.
This section will guide you through the steps of making an effective job offer, including offer components, negotiation strategies, and best practices for ensuring acceptance.
Components of the Job Offer
- Salary:
- Competitive Compensation:
- Research industry standards and salary benchmarks for data scientists.
- Ensure the offered salary is competitive within your industry and location.
- Example: Use resources like Glassdoor, Payscale, and industry-specific salary surveys to gather data.
- Consider the candidate’s experience, education, and specific skill set.
- Example: Offer a higher salary for candidates with specialized skills in machine learning or big data analytics.
- Transparency:
- Clearly outline the base salary and any additional compensation components.
- Provide a breakdown of how the salary was determined.
- Competitive Compensation:
- Bonuses and Incentives:
- Performance-Based Bonuses:
- Include details about annual or quarterly performance bonuses.
- Example: A bonus structure tied to achieving specific project milestones or company performance metrics.
- Include details about annual or quarterly performance bonuses.
- Sign-On Bonuses:
- Offer a sign-on bonus to attract top talent.
- Example: A one-time payment upon joining the company.
- Offer a sign-on bonus to attract top talent.
- Equity and Stock Options:
- Include equity or stock options as part of the compensation package.
- Example: Offer stock options that vest over a period of time to incentivize long-term commitment.
- Include equity or stock options as part of the compensation package.
- Performance-Based Bonuses:
- Benefits:
- Health and Wellness:
- Outline health insurance options, including medical, dental, and vision coverage.
- Provide details about wellness programs and initiatives.
- Example: Gym memberships, mental health support, and wellness reimbursements.
- Retirement Plans:
- Describe retirement plan options, such as 401(k) matching or pension plans.
- Include information about company contributions and vesting schedules.
- Work-Life Balance:
- Highlight policies on remote work, flexible hours, and paid time off.
- Example: Offer generous vacation days, parental leave, and personal days.
- Include details about work-from-home equipment and support.
- Example: Provide stipends for home office setups or reimbursements for internet expenses.
- Highlight policies on remote work, flexible hours, and paid time off.
- Health and Wellness:
- Professional Development:
- Training and Education:
- Offer opportunities for ongoing education and professional development.
- Example: Cover the costs of relevant courses, certifications, or conferences.
- Include details about internal training programs and workshops.
- Offer opportunities for ongoing education and professional development.
- Career Advancement:
- Outline potential career paths and advancement opportunities within the company.
- Provide information about mentorship programs and leadership development.
- Tuition Reimbursement:
- Include tuition reimbursement programs for advanced degrees or relevant courses.
- Example: Reimburse up to a certain amount per year for courses related to data science or other relevant fields.
- Include tuition reimbursement programs for advanced degrees or relevant courses.
- Training and Education:
- Work Environment and Culture:
- Company Culture:
- Highlight the company’s values, mission, and culture.
- Example: Emphasize collaborative work environment, innovation, and inclusivity.
- Share examples of team-building activities and company events.
- Highlight the company’s values, mission, and culture.
- Diversity and Inclusion:
- Outline the company’s commitment to diversity and inclusion.
- Example: Describe diversity initiatives, employee resource groups, and inclusive policies.
- Outline the company’s commitment to diversity and inclusion.
- Work Environment:
- Provide details about the office environment and amenities.
- Example: Modern office spaces, on-site cafeterias, and relaxation areas.
- Provide details about the office environment and amenities.
- Company Culture:
Negotiation Strategies
- Preparation:
- Research:
- Gather information about the candidate’s current compensation and benefits package.
- Understand industry standards and benchmarks.
- Flexibility:
- Be prepared to negotiate on various aspects of the offer, not just salary.
- Identify areas where you can be flexible and areas where you cannot.
- Example: If budget constraints limit salary increases, consider offering additional benefits or professional development opportunities.
- Research:
- Communication:
- Clear and Transparent Communication:
- Clearly articulate the components of the offer and how they align with the candidate’s expectations.
- Address any concerns or questions the candidate may have.
- Active Listening:
- Listen to the candidate’s needs and preferences.
- Show empathy and understanding towards their career goals and personal circumstances.
- Clear and Transparent Communication:
- Counteroffers:
- Evaluate Counteroffers:
- Be prepared for the possibility of a counteroffer from the candidate.
- Evaluate the feasibility of meeting their demands.
- Response Strategy:
- Respond to counteroffers promptly and professionally.
- Consider the overall fit of the candidate and the long-term benefits of securing their acceptance.
- Evaluate Counteroffers:
- Non-Monetary Negotiations:
- Flexible Work Arrangements:
- Offer flexibility in work hours, remote work options, or hybrid work models.
- Example: Allow candidates to work from home a few days a week or provide flexible start and end times.
- Offer flexibility in work hours, remote work options, or hybrid work models.
- Professional Development:
- Negotiate additional training or education opportunities.
- Example: Agree to cover the cost of a specific certification or course the candidate is interested in.
- Negotiate additional training or education opportunities.
- Work-Life Balance:
- Provide additional paid time off or personal days.
- Example: Offer extra vacation days as part of the negotiation.
- Provide additional paid time off or personal days.
- Flexible Work Arrangements:
Best Practices for Ensuring Acceptance
- Personalized Approach:
- Tailored Offers:
- Personalize the offer to align with the candidate’s career goals and preferences.
- Example: If the candidate values professional development, emphasize opportunities for growth and learning.
- Personalize the offer to align with the candidate’s career goals and preferences.
- Build Relationships:
- Establish a strong relationship with the candidate throughout the interview process.
- Maintain regular communication and express genuine interest in their success.
- Tailored Offers:
- Clear and Detailed Offer Letters:
- Comprehensive Documentation:
- Provide a clear and detailed offer letter that outlines all components of the offer.
- Example: Include information about salary, bonuses, benefits, and other incentives.
- Provide a clear and detailed offer letter that outlines all components of the offer.
- Legal Compliance:
- Ensure the offer letter complies with local labor laws and regulations.
- Include any necessary legal disclaimers or clauses.
- Comprehensive Documentation:
- Timely Follow-Up:
- Prompt Communication:
- Follow up with the candidate promptly after extending the offer.
- Example: Call the candidate to discuss the offer and answer any immediate questions they may have.
- Follow up with the candidate promptly after extending the offer.
- Regular Updates:
- Maintain regular communication with the candidate until they accept the offer.
- Example: Send periodic emails to check in and address any concerns or questions.
- Maintain regular communication with the candidate until they accept the offer.
- Prompt Communication:
- Onboarding Preparation:
- Seamless Transition:
- Prepare a comprehensive onboarding plan to ensure a smooth transition for the candidate.
- Example: Provide a detailed onboarding schedule and introduce them to key team members.
- Prepare a comprehensive onboarding plan to ensure a smooth transition for the candidate.
- Welcome Package:
- Send a welcome package that includes company swag, onboarding materials, and a personalized welcome note.
- Example: Include items like a company-branded notebook, a welcome letter from the CEO, and a guide to the company’s culture and values.
- Send a welcome package that includes company swag, onboarding materials, and a personalized welcome note.
- Seamless Transition:
By following these best practices and strategies for making an offer, you can effectively attract and secure top data science talent.
A well-crafted offer that aligns with the candidate’s expectations and career goals, combined with clear communication and timely follow-up, will increase the likelihood of acceptance and set the foundation for a successful working relationship.
8. Onboarding and Integration
Effective onboarding and integration are crucial for ensuring that new data scientists feel welcomed, supported, and equipped to contribute to your organization from day one.
A well-structured onboarding process can significantly enhance employee satisfaction, productivity, and retention.
This section will explore best practices for onboarding and integrating new data science hires, including preparation, orientation, training, and ongoing support.
Preparation for Onboarding
- Pre-Arrival Preparation:
- Welcome Package:
- Send a personalized welcome package before the start date.
- Example: Include company swag, a welcome letter, and an onboarding schedule.
- Send a personalized welcome package before the start date.
- IT Setup:
- Ensure all necessary hardware and software are ready.
- Example: Provide a laptop, necessary peripherals, and access to relevant tools and systems.
- Ensure all necessary hardware and software are ready.
- Workspace Preparation:
- Prepare the new hire’s workspace, whether in-office or remote.
- Example: Set up a comfortable and functional workspace with all required equipment.
- Prepare the new hire’s workspace, whether in-office or remote.
- Welcome Package:
- Documentation and Paperwork:
- HR Documents:
- Send all necessary HR paperwork for the new hire to complete.
- Example: Employment contracts, tax forms, and benefits enrollment forms.
- Send all necessary HR paperwork for the new hire to complete.
- Policy Manuals:
- Provide access to company policies and employee handbooks.
- Example: Include information on workplace policies, code of conduct, and data privacy guidelines.
- Provide access to company policies and employee handbooks.
- HR Documents:
- Communication Plan:
- Introduction Email:
- Send an introduction email to the team announcing the new hire.
- Example: Share the new hire’s background, role, and start date.
- Send an introduction email to the team announcing the new hire.
- Buddy System:
- Assign a buddy or mentor to help the new hire acclimate.
- Example: Choose a seasoned employee who can provide guidance and answer questions.
- Assign a buddy or mentor to help the new hire acclimate.
- Introduction Email:
Orientation
- Company Overview:
- Mission and Values:
- Introduce the company’s mission, values, and culture.
- Example: Share stories and examples that illustrate the company’s core values in action.
- Introduce the company’s mission, values, and culture.
- Organizational Structure:
- Provide an overview of the company’s organizational structure.
- Example: Explain the different departments and how they interact with the data science team.
- Provide an overview of the company’s organizational structure.
- Mission and Values:
- Role-Specific Information:
- Job Responsibilities:
- Clarify the new hire’s specific job responsibilities and expectations.
- Example: Provide a detailed job description and outline key projects they will be working on.
- Clarify the new hire’s specific job responsibilities and expectations.
- Team Introductions:
- Facilitate introductions to team members and key stakeholders.
- Example: Arrange one-on-one meetings or team lunches to foster relationships.
- Facilitate introductions to team members and key stakeholders.
- Job Responsibilities:
- Tools and Resources:
- Technical Tools:
- Introduce the new hire to the tools and technologies they will be using.
- Example: Provide training on data analysis tools like Python, R, SQL, and big data platforms.
- Introduce the new hire to the tools and technologies they will be using.
- Documentation:
- Share access to relevant documentation and resources.
- Example: Provide access to internal wikis, project documentation, and data repositories.
- Share access to relevant documentation and resources.
- Technical Tools:
Training and Development
- Initial Training:
- Technical Training:
- Offer training sessions on key technical skills and tools.
- Example: Organize workshops on machine learning algorithms, data visualization techniques, and statistical analysis.
- Offer training sessions on key technical skills and tools.
- Process Training:
- Educate the new hire on the company’s data processes and workflows.
- Example: Provide training on data collection, cleaning, analysis, and reporting protocols.
- Educate the new hire on the company’s data processes and workflows.
- Technical Training:
- Ongoing Development:
- Mentorship Programs:
- Establish a mentorship program for continuous learning and development.
- Example: Pair the new hire with an experienced mentor who can provide guidance and support.
- Establish a mentorship program for continuous learning and development.
- Professional Development Opportunities:
- Offer opportunities for ongoing education and skill enhancement.
- Example: Provide access to online courses, certifications, and industry conferences.
- Offer opportunities for ongoing education and skill enhancement.
- Regular Check-Ins:
- Schedule regular check-ins to discuss progress, challenges, and development needs.
- Example: Arrange bi-weekly meetings with the manager to review goals and provide feedback.
- Schedule regular check-ins to discuss progress, challenges, and development needs.
- Mentorship Programs:
Integration into the Team
- Building Relationships:
- Team-Building Activities:
- Organize team-building activities to foster camaraderie.
- Example: Plan team lunches, off-site events, or virtual game nights.
- Organize team-building activities to foster camaraderie.
- Collaboration Tools:
- Introduce collaboration tools and platforms.
- Example: Train the new hire on using Slack, Microsoft Teams, or other communication tools.
- Introduce collaboration tools and platforms.
- Team-Building Activities:
- Understanding Company Culture:
- Cultural Immersion:
- Involve the new hire in company traditions and cultural activities.
- Example: Include them in regular team meetings, company-wide town halls, and social events.
- Involve the new hire in company traditions and cultural activities.
- Feedback and Adaptation:
- Encourage the new hire to provide feedback on their integration experience.
- Example: Use surveys or one-on-one meetings to gather insights and make necessary adjustments.
- Encourage the new hire to provide feedback on their integration experience.
- Cultural Immersion:
Performance and Feedback
- Setting Goals and Expectations:
- SMART Goals:
- Establish specific, measurable, achievable, relevant, and time-bound (SMART) goals.
- Example: Set clear performance targets and project milestones for the first six months.
- Establish specific, measurable, achievable, relevant, and time-bound (SMART) goals.
- Regular Performance Reviews:
- Conduct regular performance reviews to assess progress and provide feedback.
- Example: Schedule quarterly reviews to discuss achievements, challenges, and development areas.
- Conduct regular performance reviews to assess progress and provide feedback.
- SMART Goals:
- Providing Feedback:
- Constructive Feedback:
- Provide constructive feedback to help the new hire improve and grow.
- Example: Highlight strengths and areas for improvement in a supportive manner.
- Provide constructive feedback to help the new hire improve and grow.
- Continuous Improvement:
- Encourage a culture of continuous improvement and learning.
- Example: Promote a growth mindset and provide resources for skill development.
- Encourage a culture of continuous improvement and learning.
- Constructive Feedback:
By following these best practices and strategies for onboarding and integrating new data science hires, you can ensure they feel welcomed, supported, and equipped to contribute effectively to your organization.
A well-structured onboarding process not only enhances employee satisfaction and productivity but also sets the foundation for long-term success and retention.
Conclusion
Hiring a data scientist is a pivotal decision for any organization aiming to harness the power of data-driven insights.
This comprehensive guide has walked you through the step-by-step process of hiring a data scientist, from defining your needs to onboarding and integration.
By following these structured steps, you can streamline your hiring process, attract top talent, and build a data-driven culture that drives innovation and growth.
Recap of Key Steps
- Understanding the Role: We began by exploring the crucial aspects of a data scientist’s role, including key skills, qualifications, and responsibilities. By defining the role clearly, you ensure alignment between your expectations and the candidate’s capabilities.
- Crafting the Perfect Job Description: A well-crafted job description serves as a magnet for qualified candidates. We discussed how to structure job descriptions effectively to attract the right talent pool and set realistic expectations.
- Sourcing and Screening Candidates: Strategies for sourcing candidates, whether through job boards, networking, or recruitment agencies, were covered in detail. Screening techniques, including technical assessments and cultural fit evaluations, were highlighted to ensure you select candidates who not only meet but exceed your expectations.
- Interviewing Candidates: Effective interview techniques, from behavioral questions to technical assessments, were explored to assess a candidate’s skills, problem-solving abilities, and cultural fit accurately.
- Making the Offer: Crafting a compelling job offer that includes competitive compensation, benefits, and professional development opportunities is essential to securing top talent. Negotiation strategies and best practices for ensuring acceptance were also discussed.
- Onboarding and Integration: Finally, we emphasized the importance of a structured onboarding process to integrate new hires seamlessly into your organization. Providing comprehensive training, clarifying roles and expectations, and fostering a supportive environment are key to retaining talent and maximizing their contributions.
Importance of Data Scientists
Data scientists play a critical role in driving business strategy, optimizing operations, and enhancing customer experiences through data analysis and insights.
As businesses increasingly rely on data to make informed decisions, hiring skilled data scientists becomes paramount to maintaining a competitive edge in today’s digital landscape.
Future Trends in Data Science Hiring
Looking ahead, the field of data science continues to evolve with advancements in AI, machine learning, and big data technologies.
Organizations must adapt their hiring strategies to attract data scientists who possess not only technical expertise but also the ability to innovate and adapt to emerging trends.
Conclusion
In conclusion, hiring a data scientist requires a strategic approach that aligns with your organization’s goals and values.
By following the steps outlined in this guide, you can navigate the complexities of hiring data scientists with confidence and precision.
Remember, investing in the right talent today can lead to transformative outcomes tomorrow, propelling your organization towards data-driven success.
Whether you’re building a data science team from scratch or expanding an existing one, this guide equips you with the tools and knowledge to make informed decisions at every stage of the hiring process. Embrace the opportunities that data science offers and leverage the expertise of talented data scientists to drive innovation and growth in your organization.
If your company needs HR, hiring, or corporate services, you can use 9cv9 hiring and recruitment services. Book a consultation slot here, or send over an email to hello@9cv9.com.
If you find this article useful, why not share it with your hiring manager and C-level suite friends and also leave a nice comment below?
We, at the 9cv9 Research Team, strive to bring the latest and most meaningful data, guides, and statistics to your doorstep.
To get access to top-quality guides, click over to 9cv9 Blog.
People Also Ask
How do I define the role of a data scientist for my company?
Understanding the specific needs and goals of your organization is crucial. Define the responsibilities, required skills, and how the role fits into your overall business strategy.
What are the key skills to look for in a data scientist?
Look for proficiency in programming languages like Python or R, expertise in statistical analysis and machine learning, data visualization skills, and a strong understanding of database systems and big data technologies.
How can I craft an effective job description for a data scientist?
Craft a job description that clearly outlines responsibilities, qualifications, and expectations. Highlight key projects, technologies used, and opportunities for growth within your company.
What are the best sources for sourcing data science candidates?
Explore online job boards, professional networking platforms like LinkedIn, industry-specific forums, recruitment agencies specializing in tech roles, and attending data science conferences or meetups.
What screening techniques can I use to evaluate data science candidates?
Use technical assessments, coding challenges, case studies, and data analysis exercises to gauge technical proficiency. Assess cultural fit through behavioral interviews and team-based scenarios.
How should I structure the interview process for hiring a data scientist?
Structure interviews to assess technical skills, problem-solving abilities, and cultural fit. Include initial screenings, technical interviews, and discussions with key stakeholders to evaluate alignment with company values.
What should I include in a data scientist’s compensation package?
Offer competitive salaries based on industry standards and candidate experience. Include bonuses tied to performance, equity options, comprehensive health benefits, professional development opportunities, and work-life balance perks.
How can I effectively negotiate with data science candidates?
Understand candidate expectations and market rates. Be prepared to negotiate on salary, benefits, remote work options, and career development opportunities while ensuring alignment with company policies and budget constraints.
What role does cultural fit play in hiring a data scientist?
Cultural fit ensures alignment with your company’s values, work environment, and team dynamics. Evaluate candidates’ collaboration skills, communication style, and adaptability to company culture.
How important is experience in specific industries or domains for a data scientist?
Industry-specific experience can bring valuable insights and understanding of domain-specific challenges and data nuances. Consider candidates who can apply their skills effectively in your industry context.
What onboarding strategies are effective for integrating data scientists into my team?
Implement a structured onboarding program that includes orientation on company culture, introduction to team members and stakeholders, technical training on tools and systems, and ongoing mentorship and support.
How can I provide continuous learning opportunities for data scientists?
Offer access to online courses, workshops, certifications, and conferences relevant to data science and analytics. Support participation in industry events and encourage knowledge sharing within the team.
What are the common challenges in hiring data scientists?
Challenges include competition for top talent, evolving skill requirements, aligning candidate expectations with company offerings, and ensuring cultural fit within the organization.
How can I evaluate a data scientist’s ability to handle real-world data challenges?
Use case studies or real-world scenarios during interviews to assess problem-solving skills, analytical thinking, and the ability to derive meaningful insights from complex datasets.
What are some red flags to watch for during the hiring process?
Watch for candidates lacking clarity on their past projects or technical skills, inconsistent communication, and a lack of alignment with your company’s values or culture.
How should I assess a data scientist’s communication skills?
Evaluate their ability to explain technical concepts to non-technical stakeholders, present findings clearly and concisely, and collaborate effectively within cross-functional teams.
What strategies can I use to retain data science talent?
Offer competitive compensation, opportunities for career advancement, a supportive work environment, ongoing learning and development, recognition for achievements, and work-life balance initiatives.
How do I ensure diversity and inclusion in my data science hiring process?
Implement inclusive job descriptions, diverse candidate sourcing strategies, unbiased screening and interview processes, and foster an inclusive workplace culture that values diverse perspectives and backgrounds.
What metrics should I use to measure the success of my data science hires?
Measure success based on project outcomes, impact on business KPIs, contribution to data-driven decision-making, feedback from team members and stakeholders, and professional growth within the organization.
How can I promote collaboration between data scientists and other teams?
Encourage cross-functional collaboration through regular meetings, shared project goals, and open communication channels. Foster a collaborative culture that values knowledge sharing and teamwork.
What are the ethical considerations when hiring data scientists?
Ensure data privacy and security compliance, ethical handling of data, transparency in algorithms and decision-making processes, and promote ethical conduct in data analysis and reporting.
How should I handle candidate rejections respectfully?
Provide constructive feedback to candidates, communicate promptly about the decision, and maintain a positive relationship for potential future opportunities or referrals.
How can I leverage recruitment technology in hiring data scientists?
Use applicant tracking systems (ATS) for streamlined candidate management, AI-powered tools for resume screening and sourcing, and virtual interview platforms for remote hiring processes.
How do I stay updated with trends in data science hiring?
Stay informed through industry publications, attending conferences and webinars, networking with professionals in the field, and participating in online communities focused on data science and analytics.
What are the career growth opportunities for data scientists in my organization?
Outline career paths that include opportunities for specialization, leadership roles, and interdisciplinary collaboration. Offer mentoring, continuous learning, and professional development initiatives.
How can I assess a data scientist’s potential for leadership roles?
Look for leadership qualities such as strategic thinking, mentorship capabilities, effective decision-making, and the ability to influence and inspire others within the team.
How can I create a positive candidate experience throughout the hiring process?
Communicate transparently, provide timely updates, offer a seamless interview experience, and ensure respectful treatment of candidates to enhance their overall experience with your organization.
How do I measure the ROI of hiring a data scientist?
Calculate ROI based on improved business insights, operational efficiencies, cost savings, and revenue growth attributed to data-driven initiatives led by the data scientist.
What are the emerging skills and technologies in data science hiring?
Stay updated on emerging skills like AI and machine learning, advanced analytics techniques, cloud computing platforms, and data visualization tools to remain competitive in hiring top data science talent.